What is a degree of freedom?
TLDRIn this educational video, Dr. Jack explores the concept of statistical power and its relationship with degrees of freedom in the context of statistical models. He explains degrees of freedom as the number of cells in a dataset that can freely vary and how applying statistical models, such as means or regressions, reduces this variability. Dr. Jack illustrates how more complex models, while fitting the data more closely, can decrease statistical power by using up degrees of freedom. The video emphasizes the importance of balancing model complexity with the ability to make accurate predictions about data that was free to vary, highlighting the significance of statistical power in validating the robustness of a model.
Takeaways
- ๐ **Degrees of Freedom**: The number of cells in a dataset that can vary independently. It's reduced when a statistical model is applied because the model uses up some of the variability.
- ๐ข **Statistical Models**: Simple models use fewer degrees of freedom, while complex models use more, impacting the statistical power of the analysis.
- โ๏ธ **Mean as a Model**: Knowing the mean reduces the degrees of freedom because it allows you to calculate the value of a missing data point.
- ๐ **Model Complexity vs. Power**: More complex models fit the data better but can decrease statistical power because they use up more degrees of freedom.
- ๐ **Statistical Power**: The ability of a model to make accurate predictions about data that was free to vary. It's crucial for making reliable predictions about future samples.
- ๐ **Linear Regression**: A statistical model that uses two degrees of freedom (the slope and y-intercept) to describe the relationship between two variables.
- ๐ **Polynomial Models**: More complex than linear models, polynomials use more degrees of freedom for each additional term in the model, reducing the data's variability.
- ๐ง **Model Robustness**: A model's robustness is tied to its ability to predict on data that was allowed to vary freely; overfitting reduces this ability.
- ๐ **Coefficients in Models**: In multiple regression, each variable has a coefficient that represents its contribution to the model, using up degrees of freedom.
- ๐งฎ **Statistical Formulas**: Statisticians use flipped formulas (ฮฒ0, ฮฒ1, ฮฒ2, etc.) to easily add more variables to a model, which is essential for complex analyses.
- ๐ **Understanding Statistics**: Focus on understanding the concepts of explained vs. unexplained variation and the importance of degrees of freedom for statistical power, rather than memorizing formulas.
Q & A
What is the concept of statistical power in the context of statistical models?
-Statistical power refers to the probability that a statistical test will reject the null hypothesis when the null hypothesis is false. It is deeply intertwined with degrees of freedom and is a measure of the test's ability to detect an effect if there is one.
What are degrees of freedom and why are they important in statistics?
-Degrees of freedom represent the number of values in a dataset that are free to vary independently. They are crucial because they determine the number of independent pieces of information and are used in calculating standard deviations and variances. In the context of a statistical model, the degrees of freedom can influence the model's complexity and its statistical power.
How does applying a statistical model affect the degrees of freedom in a dataset?
-Applying a statistical model reduces the degrees of freedom in a dataset. This is because the model imposes constraints on the data, meaning that some values can be calculated from others. For instance, knowing the mean and five out of six data points allows you to calculate the sixth, thus reducing the degrees of freedom by one.
Why might a simpler statistical model be chosen over a more complex one?
-A simpler statistical model might be chosen because it retains more degrees of freedom, which can lead to higher statistical power. Simple models are also often easier to interpret and less prone to overfitting, where the model describes random error or noise instead of the underlying relationship.
What is the relationship between the complexity of a statistical model and its statistical power?
-As the complexity of a statistical model increases, it tends to fit the data better, reducing unexplained variation. However, this increased complexity also uses up more degrees of freedom, which can decrease the model's statistical power. A balance must be struck between model complexity and the ability to make robust predictions.
How does the concept of a linear model relate to other statistical tests like t-tests and ANOVAs?
-Linear models are fundamental to many statistical tests. A t-test and ANOVA are essentially special cases of linear models where the relationship between the dependent and independent variables is assumed to be linear. Understanding the linear model concept helps in grasping the principles behind these tests.
What is the formula for a linear regression model and how does it relate to degrees of freedom?
-The formula for a simple linear regression model is y = ฮฒ0 + ฮฒ1x + ฮต, where y is the dependent variable, x is the independent variable, ฮฒ0 is the y-intercept, ฮฒ1 is the slope, and ฮต is the error term. Each parameter (ฮฒ0, ฮฒ1) in the model uses up degrees of freedom, as they are estimated from the data.
How does the introduction of more variables into a model affect its degrees of freedom?
-Introducing more variables into a model increases its complexity and the number of coefficients needed to estimate the model. Each additional variable adds another degree of freedom to the model, which reduces the degrees of freedom available for the data, potentially decreasing the model's statistical power.
What is the role of statistical power in making predictions about future samples?
-Statistical power is crucial for making accurate predictions about future samples. A model with high statistical power can predict a large amount of variation in the data, and if the data was free to vary, the model is considered robust and reliable for predictions.
Why is it important to consider both explained and unexplained variation when evaluating a statistical model?
-Explained variation shows how much of the data the model can account for, while unexplained variation is what remains. A good model will have a significant amount of explained variation, indicating that it has captured the underlying relationship. However, there should also be some unexplained variation to ensure the model is not overfitting the data.
What is the significance of the y-intercept (ฮฒ0) and the slope (ฮฒ1) in a linear regression model?
-The y-intercept (ฮฒ0) represents the expected value of y when all the independent variables in the model are zero. The slope (ฮฒ1) indicates the change in the dependent variable for a one-unit change in the independent variable. Together, they define the line of best fit for the data in the model.
Outlines
๐ Introduction to Statistical Power and Degrees of Freedom
Dr. Jack Order introduces the concept of statistical power and the trade-off between complex and simple statistical models. He explains that statistical power is linked to degrees of freedom, which is often discussed without a clear definition. Using an Excel spreadsheet analogy, he clarifies that a degree of freedom is a cell that can take any value, and the number of such cells represents the degrees of freedom in a dataset. By applying a statistical model, such as calculating the mean, the dataset loses degrees of freedom, which impacts the power of the model to make predictions about future data.
๐ The Impact of Model Complexity on Degrees of Freedom and Statistical Power
This paragraph delves into how applying more complex statistical models, such as calculating means for different treatment groups, reduces the degrees of freedom in the data. Dr. Order illustrates that as the model becomes more complex, the data becomes less free to vary, which in turn affects the model's ability to make accurate predictions about new samples. He emphasizes the importance of statistical power in determining the robustness of a model and its ability to explain variation in the data that was free to vary.
๐ Understanding Linear Regression and Polynomial Models in Terms of Degrees of Freedom
Dr. Order explains the concept of linear regression as a statistical model that uses degrees of freedom. He uses the formula y = mx + c to demonstrate that each data point in a linear model has one degree of freedom, and the model itself uses up degrees of freedom based on the number of coefficients it requires. He further discusses polynomial models, which are more complex and require more coefficients, thus using up more degrees of freedom and reducing the model's statistical power. The paragraph highlights the trade-off between model fit and power due to the consumption of degrees of freedom.
๐ The Importance of Statistical Models, Degrees of Freedom, and Statistical Power in Data Analysis
In the final paragraph, Dr. Order summarizes the importance of understanding statistical models, degrees of freedom, and statistical power. He stresses that the goal of statistical analysis is to explain variation in the data and that a good model is one that explains a significant amount of variation with data that was free to vary. He also points out that complex models, while fitting the data better, can decrease statistical power due to the reduction in degrees of freedom. The paragraph concludes with a preview of upcoming content that will further explore the concept that all statistical tests are essentially linear models.
Mindmap
Keywords
๐กStatistical Power
๐กDegrees of Freedom
๐กStatistical Models
๐กMean
๐กVariation
๐กLinear Regression
๐กCoefficients
๐กModel Complexity
๐กPolynomial Model
๐กUnexplained Variation
Highlights
Introduction of the concept of statistical power and its relation to simple versus complex statistical models.
Explanation of statistical power being intertwined with degrees of freedom.
Clarification on the term 'degrees of freedom' and its common misuse in statistical discussions.
Illustration of degrees of freedom using an Excel spreadsheet analogy.
Demonstration of how applying a statistical model like the mean reduces the degrees of freedom in data.
Discussion on the trade-off between model complexity and the ability to make strong predictions about future samples.
Example of how increasing the number of means in a statistical model (e.g., by group) reduces data's degrees of freedom.
Importance of data being free to vary for a model to have statistical power.
Exploration of how a more complex model with treatment and gender groups affects degrees of freedom and statistical power.
The concept that a model with zero degrees of freedom has zero statistical power, making it unable to predict future data.
Introduction to the linear regression model and its relation to degrees of freedom.
Explanation of the linear regression formula y = mx + c and its components' impact on degrees of freedom.
Transition to using coefficients (beta0, beta1, etc.) in statistical models to accommodate multiple variables.
Introduction of polynomial models and their increased use of degrees of freedom due to complexity.
Statistical expression and notation for models with multiple coefficients.
The impact of model complexity on fit and statistical power, emphasizing the importance of a balance.
Emphasis on the importance of understanding statistical models in terms of explained and unexplained variation.
The significance of degrees of freedom in ensuring a model's robustness and predictive power.
Upcoming discussion on the universality of linear models in various statistical tests and methods.
Transcripts
Browse More Related Video
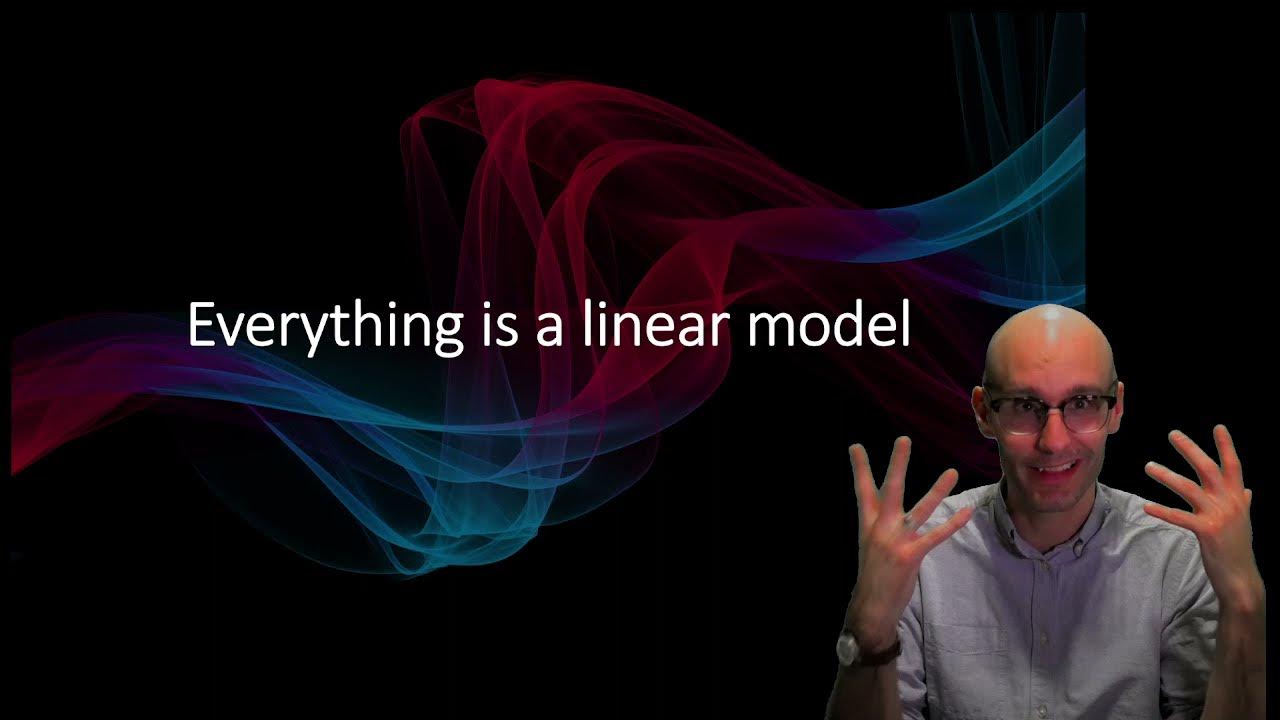
Everything is a linear model (nearly)
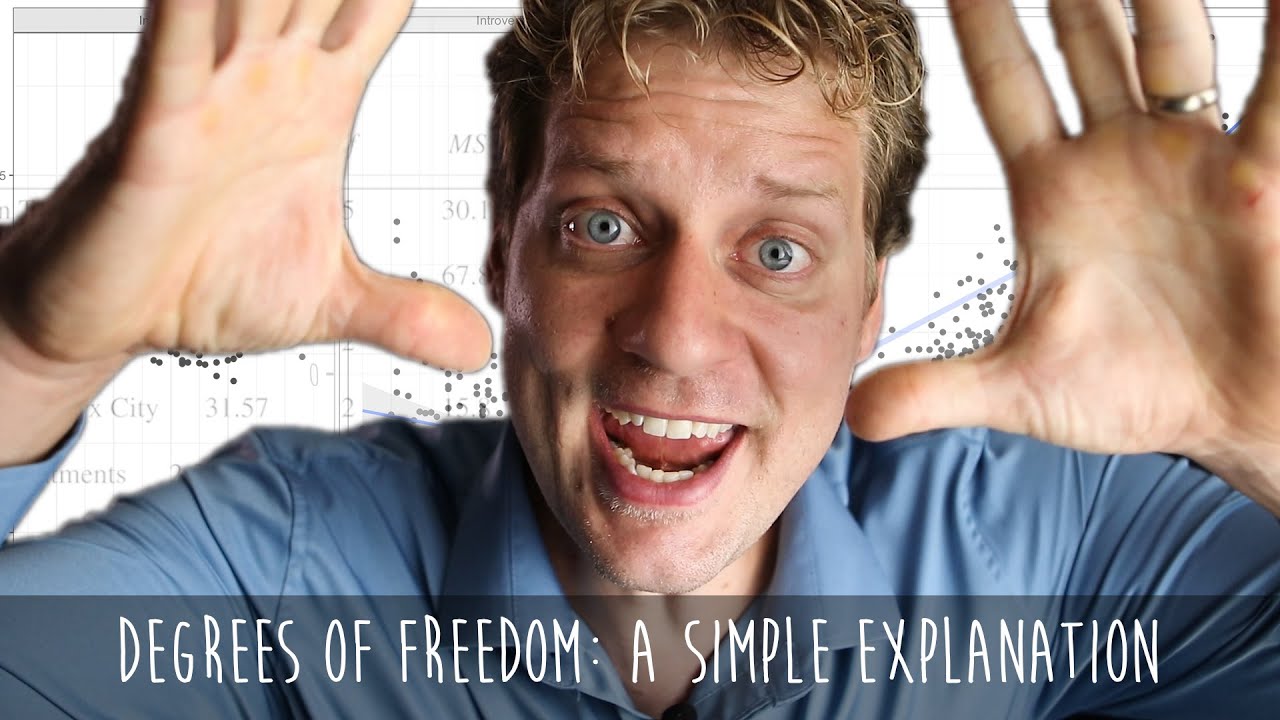
What are degrees of freedom in statistics? A simple explanation.
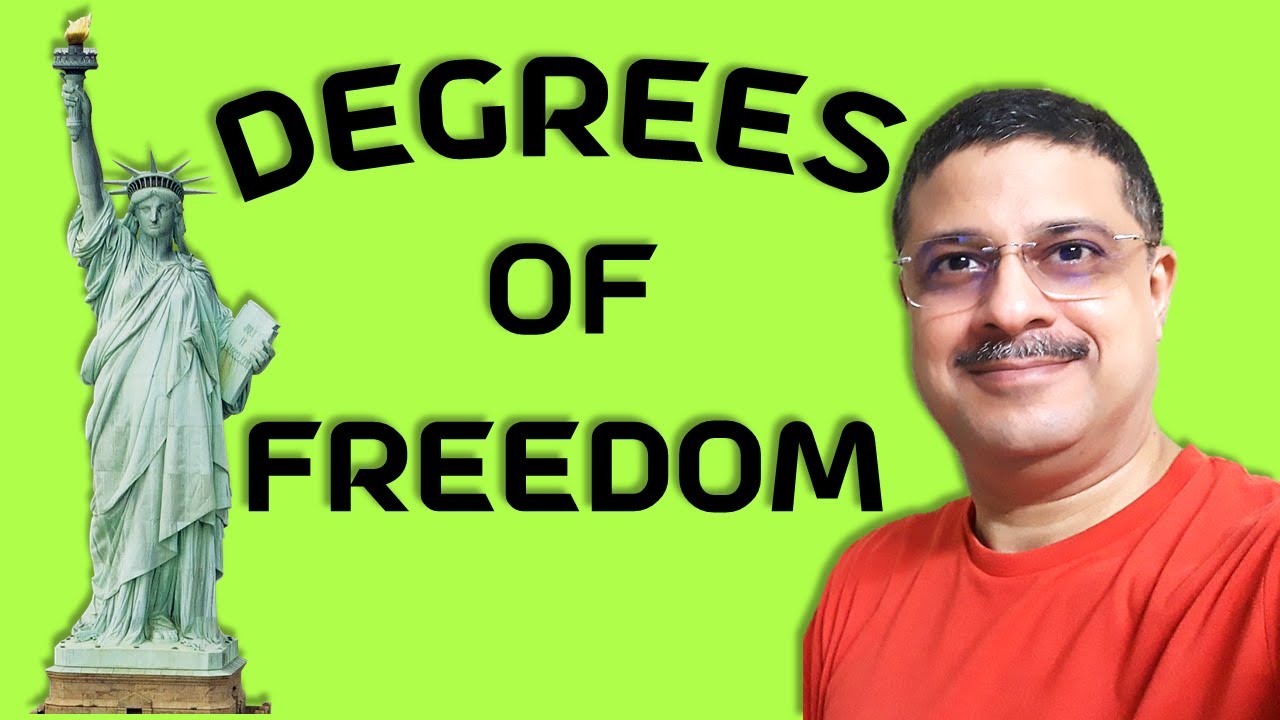
What is Degrees Of Freedom in Statistics? Degrees of freedom in Statistics Explained!
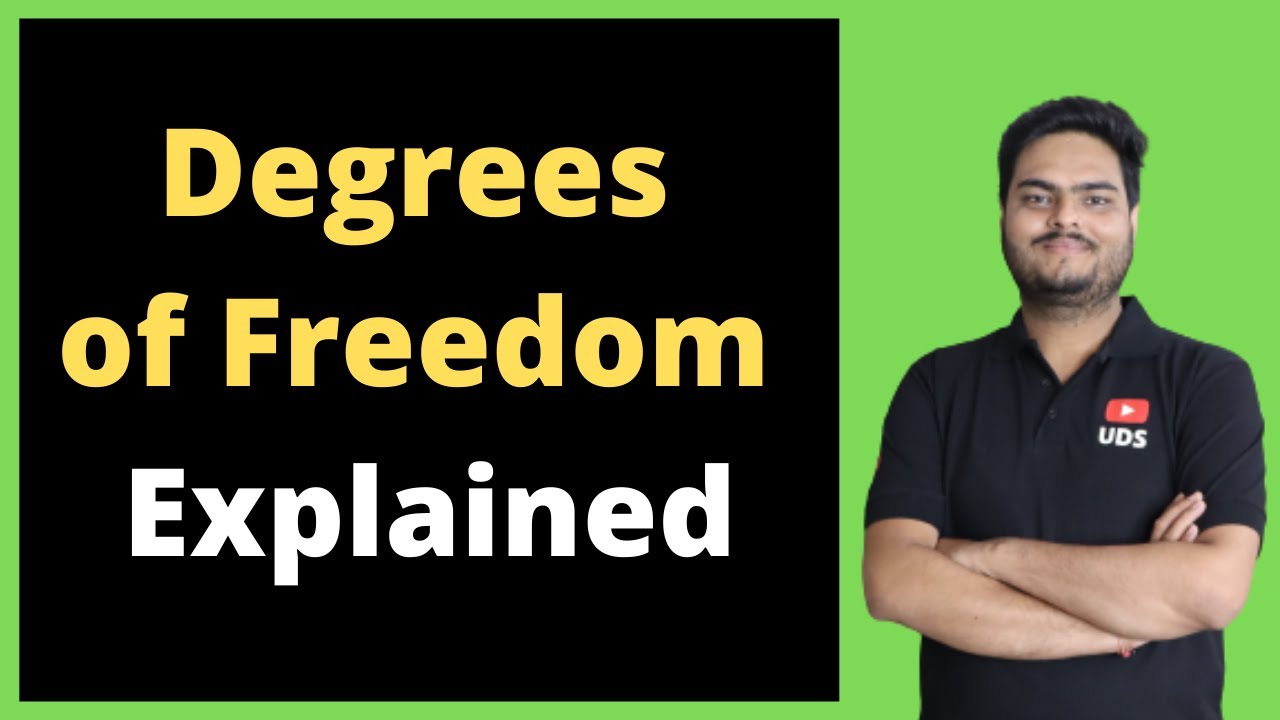
Degrees Of Freedom Explained | What is Degrees of freedom | Degrees of freedom in statistics
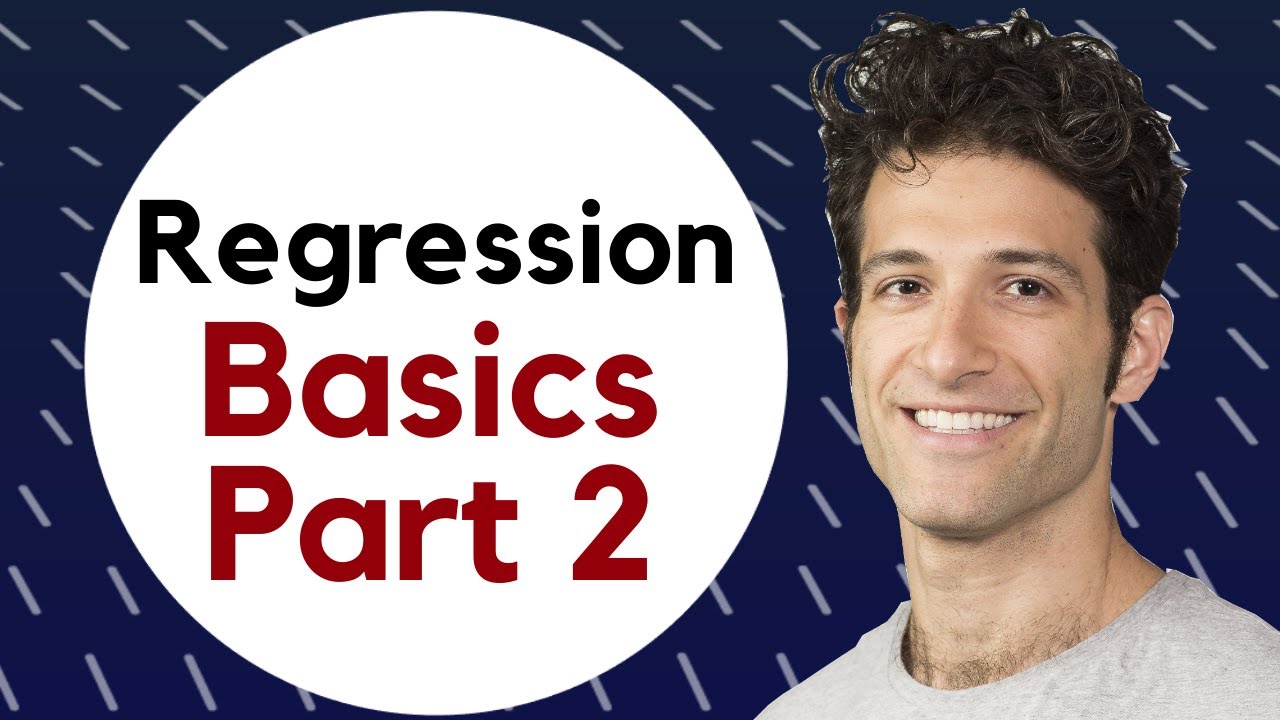
Regression II - Degrees of Freedom EXPLAINED | Adjusted R-Squared
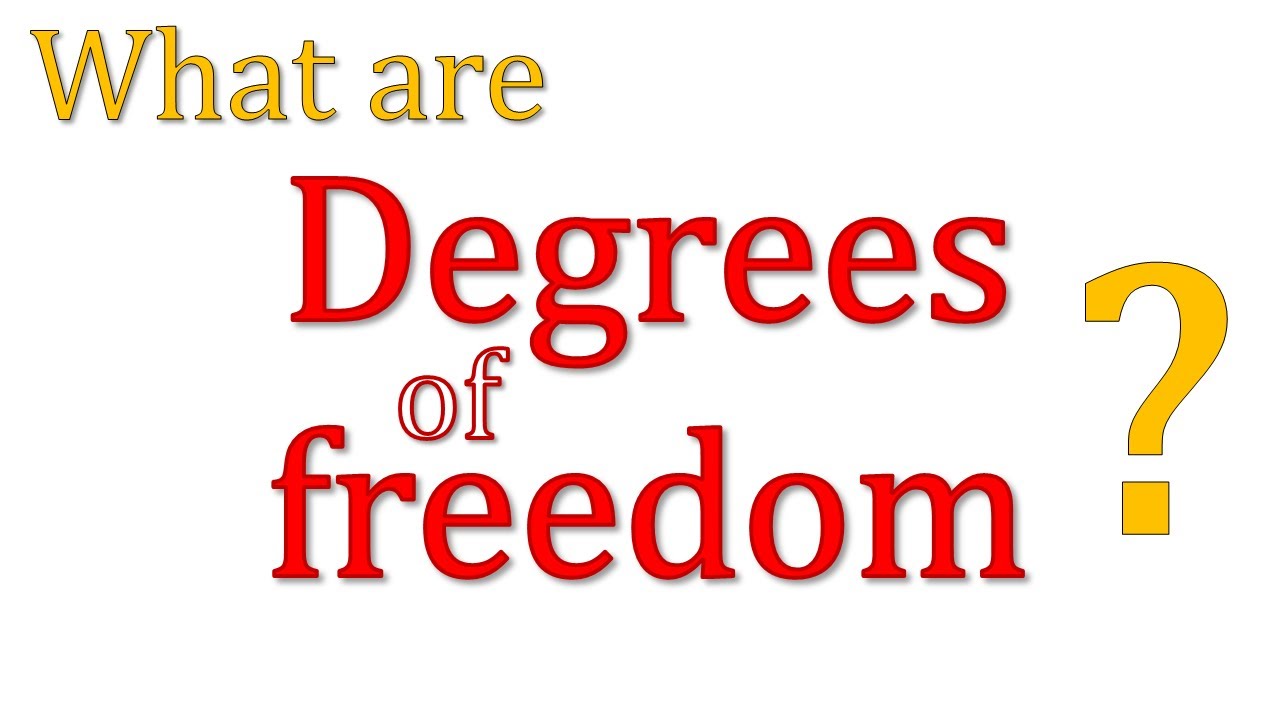
Statistical degrees of freedom - What are they REALLY?
5.0 / 5 (0 votes)
Thanks for rating: