What are degrees of freedom in statistics? A simple explanation.
TLDRIn this video, the concept of degrees of freedom in statistical models is explored through a metaphor of buying parameters with data, provided by Joseph Rogers. Degrees of freedom tell us how many parameters we've estimated (spent) and how many data points are left to test our model (remaining). The video uses examples and metaphors, such as working hours in a week and statistical models, to explain the importance of having leftover degrees of freedom for testing. The conclusion emphasizes the significance of reporting spent and remaining degrees of freedom clearly in statistical tables.
Takeaways
- π Degrees of freedom are a critical concept in statistics, indicating the number of independent pieces of information in a dataset.
- π‘ The video uses an accounting metaphor to explain degrees of freedom, comparing data points to money and parameters to commodities.
- π The purpose of degrees of freedom is to determine how many parameters can be estimated without exhausting all available data.
- π§© After estimating parameters, the remaining data should be used to test the model, which is akin to having leftover money after purchasing commodities.
- π A model that fits perfectly with no degrees of freedom left is not impressive, as it has no choice but to fit due to the limited data and parameters.
- π The video illustrates the concept with an example of a regression line fitted with only two data points, which must fit perfectly but doesn't demonstrate model quality.
- π― Impressive models are those that fit well even with many degrees of freedom remaining, indicating a good fit is not just due to parameter estimation.
- π Degrees of freedom can be thought of as 'spent' on estimating parameters and 'remaining' for testing the model's validity.
- π The video references Joseph Rogers' work, emphasizing the importance of distinguishing between spent and remaining degrees of freedom.
- π€ The current reporting of degrees of freedom in statistical tables, such as ANOVA, is criticized for not clearly distinguishing between spent and remaining degrees.
- π The video suggests that a reformed ANOVA summary table should explicitly show spent and remaining degrees of freedom for clarity.
Q & A
What is the main topic of the video?
-The main topic of the video is 'degrees of freedom' in the context of statistical models.
Why does the speaker delay revealing the answer to the main question?
-The speaker delays revealing the answer because it is complex and they want to engage the audience and explain what degrees of freedom tell us about statistical models first.
What is the metaphor used by the speaker to explain statistical models?
-The speaker uses the metaphor of accounting, where in the real world we buy commodities and in statistics we 'buy' parameters with data.
What does the term 'spent degrees of freedom' refer to according to the video?
-'Spent degrees of freedom' refers to the number of parameters estimated in a statistical model.
What does 'remaining degrees of freedom' indicate in the context of the video?
-'Remaining degrees of freedom' indicates the number of data points left to test the model after estimating parameters.
What is the significance of having 'remaining degrees of freedom' in a statistical model?
-Having 'remaining degrees of freedom' is significant because it allows for testing the model, providing an opportunity to see if the model actually fits the data beyond just estimating parameters.
What is the example given to illustrate the concept of 'spent' and 'remaining' degrees of freedom?
-The example given is about working a 40-hour week, where the total hours worked over six days constrain the hours that can be worked on the seventh day, illustrating the concept of 'spent' and 'remaining' degrees of freedom.
Why is it not impressive if a model fits perfectly with no degrees of freedom left?
-It is not impressive because the model has no choice but to fit perfectly given that all data points were used to estimate parameters, leaving none to test the model's performance.
What does the video suggest about the reporting of degrees of freedom in statistical tables?
-The video suggests that the current way of reporting degrees of freedom in statistical tables, such as ANOVA summary tables, is frustrating and should be reformulated to differentiate between 'spent' and 'remaining' degrees of freedom.
What is the speaker's final comment on the importance of degrees of freedom in statistical models?
-The speaker's final comment emphasizes that degrees of freedom are crucial as they tell us how many parameters have been estimated and how many data points are left to test the model, which is key to evaluating the model's effectiveness.
Outlines
π§ Degrees of Freedom in Statistical Models
The first paragraph introduces the concept of degrees of freedom in the context of statistical models. It uses an accounting metaphor to explain that in statistics, we 'buy' parameters such as mean, slope, or correlation using our data. Each data point allows us to estimate these parameters. The key takeaway is that after estimating parameters, we should have 'money' (data points) left to test our model. If we spend all our degrees of freedom on estimating parameters, we have nothing left to validate our model's performance. An example with two data points and two parameters (intercept and slope) illustrates that a perfect fit does not indicate a good modelβit's expected when the number of parameters equals the number of data points. The paragraph emphasizes that a model is impressive if it fits well even with many degrees of freedom remaining.
π Understanding Spent and Remaining Degrees of Freedom
The second paragraph delves deeper into the concept of degrees of freedom by differentiating between 'spent' degrees of freedom (used to estimate parameters) and 'remaining' degrees of freedom (available to test the model). It discusses the importance of having a substantial number of remaining degrees of freedom to assess the model's effectiveness. An example is provided comparing a model with no degrees of freedom left, which cannot be tested, to a model with many degrees of freedom left, allowing for testing and revealing the model's quality. The paragraph also critiques the way degrees of freedom are reported in ANOVA summary tables, suggesting they should distinguish between spent and remaining degrees for clarity. The speaker references a paper by Joe Rogers and mentions the Visual Modeling module in Jazz, which correctly separates spent and remaining degrees of freedom. The paragraph concludes by inviting questions and encouraging engagement with the content.
Mindmap
Keywords
π‘Degrees of Freedom
π‘Statistical Models
π‘Parameters
π‘Accounting Metaphor
π‘Regression Line
π‘Spent Degrees of Freedom
π‘Remaining Degrees of Freedom
π‘ANOVA Summary Table
π‘Unique Combination
π‘Visual Modeling Module
Highlights
The video discusses the concept of degrees of freedom in statistical models.
The presenter uses an accounting metaphor to explain degrees of freedom, comparing data points to money used to 'buy' parameters.
Parameters in statistical models, such as mean, slope, or correlation, are 'bought' with data.
The importance of having data left over after estimating parameters to test the model is emphasized.
An example is given where two data points are used to estimate a regression line with an intercept and slope, leaving no degrees of freedom for model testing.
Fitting a model perfectly with no degrees of freedom left is not impressive as the model has no choice but to fit.
A personal anecdote is shared about being a unique combination of a statistician and a photographer.
The presenter argues that fitting a model with many parameters is not impressive unless there are degrees of freedom left over.
A model that fits with many degrees of freedom left is considered impressive and the goal of statistical modeling.
Degrees of freedom indicate the number of parameters estimated (spent degrees of freedom) and data points left to test the model (remaining degrees of freedom).
A workweek example is used to illustrate degrees of freedom, comparing it to choosing work hours within a fixed total.
In statistical models, estimating parameters constrains the values they can take, similar to the workweek example.
The concept of degrees of freedom is central to understanding how well a model can be tested and validated.
A model with no degrees of freedom left cannot be tested for effectiveness.
A model with many degrees of freedom allows for testing and can reveal if the model fits well or poorly.
The presenter criticizes the way degrees of freedom are reported in ANOVA summary tables as confusing.
The video suggests reformulating ANOVA summary tables to distinguish between spent and remaining degrees of freedom.
The Jazz visual modeling module is mentioned as an example of software that differentiates between spent and remaining degrees of freedom.
Transcripts
Browse More Related Video
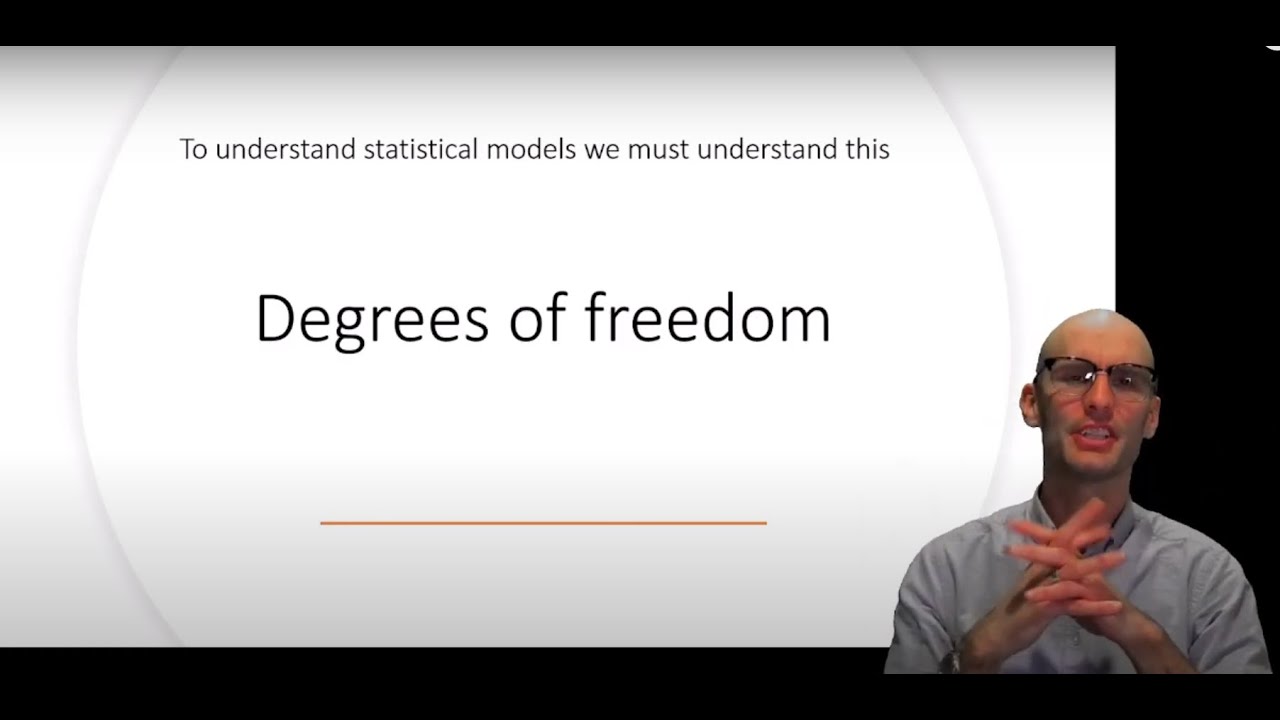
What is a degree of freedom?
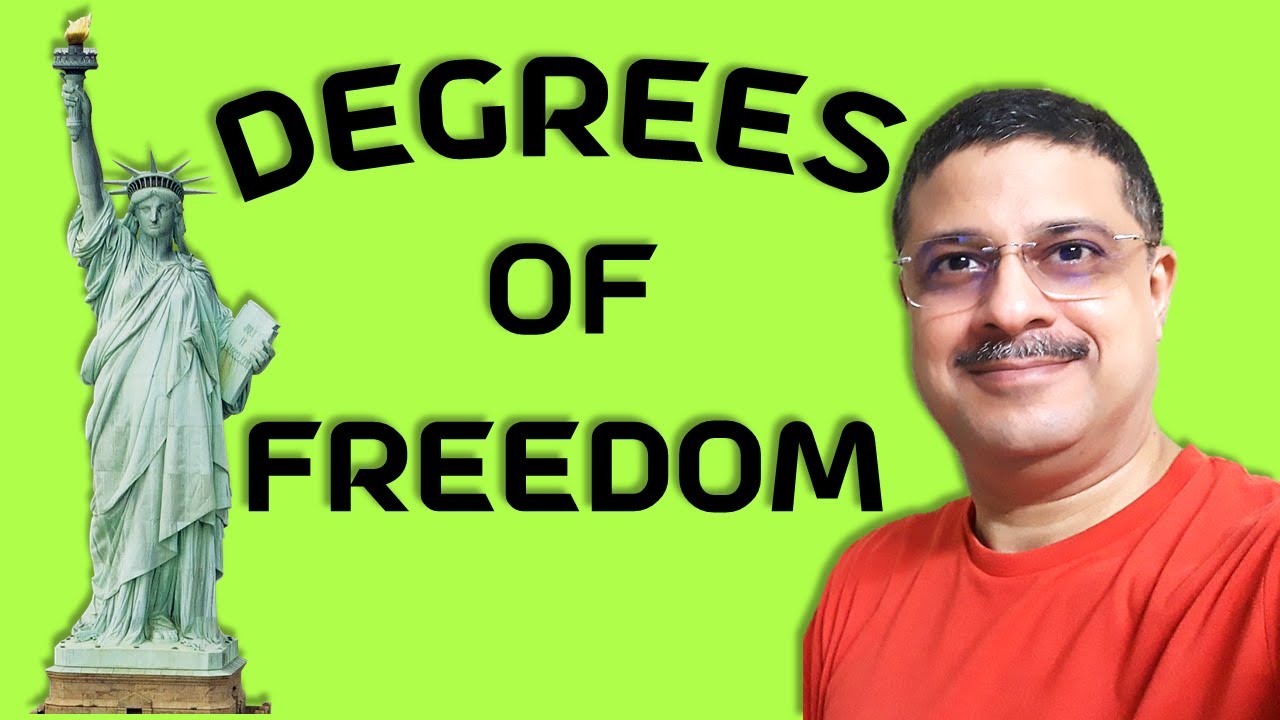
What is Degrees Of Freedom in Statistics? Degrees of freedom in Statistics Explained!
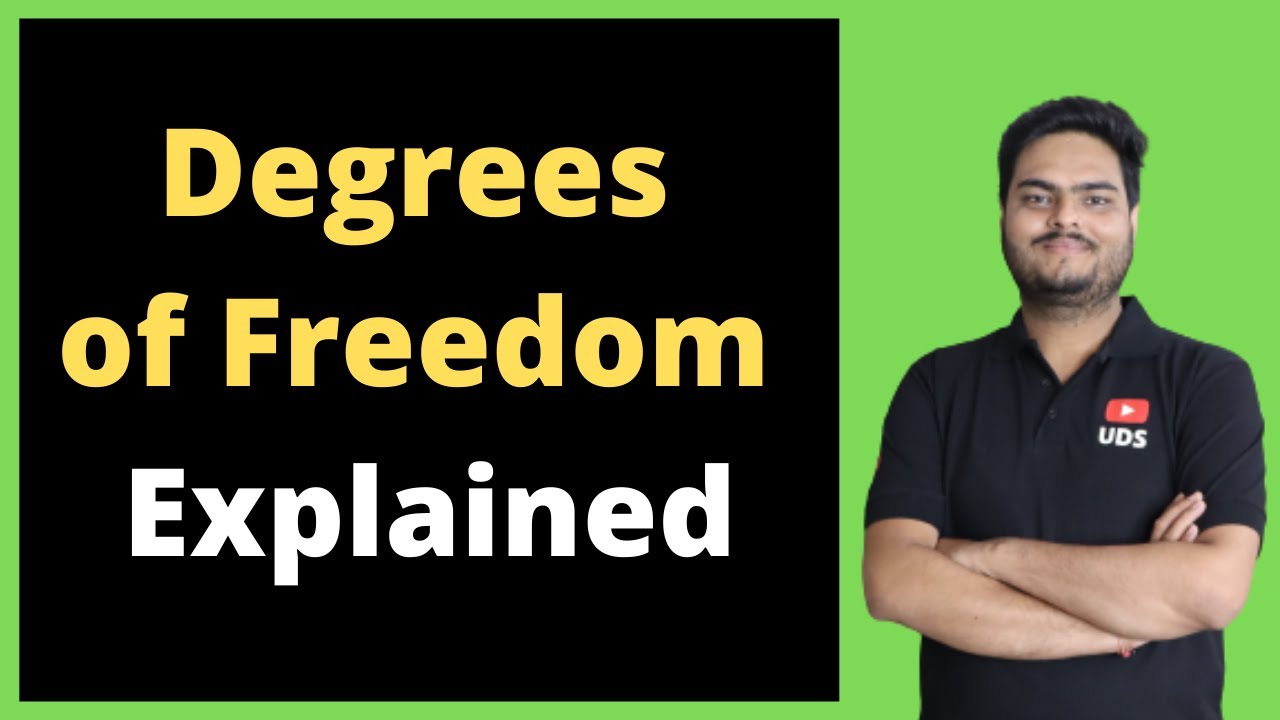
Degrees Of Freedom Explained | What is Degrees of freedom | Degrees of freedom in statistics
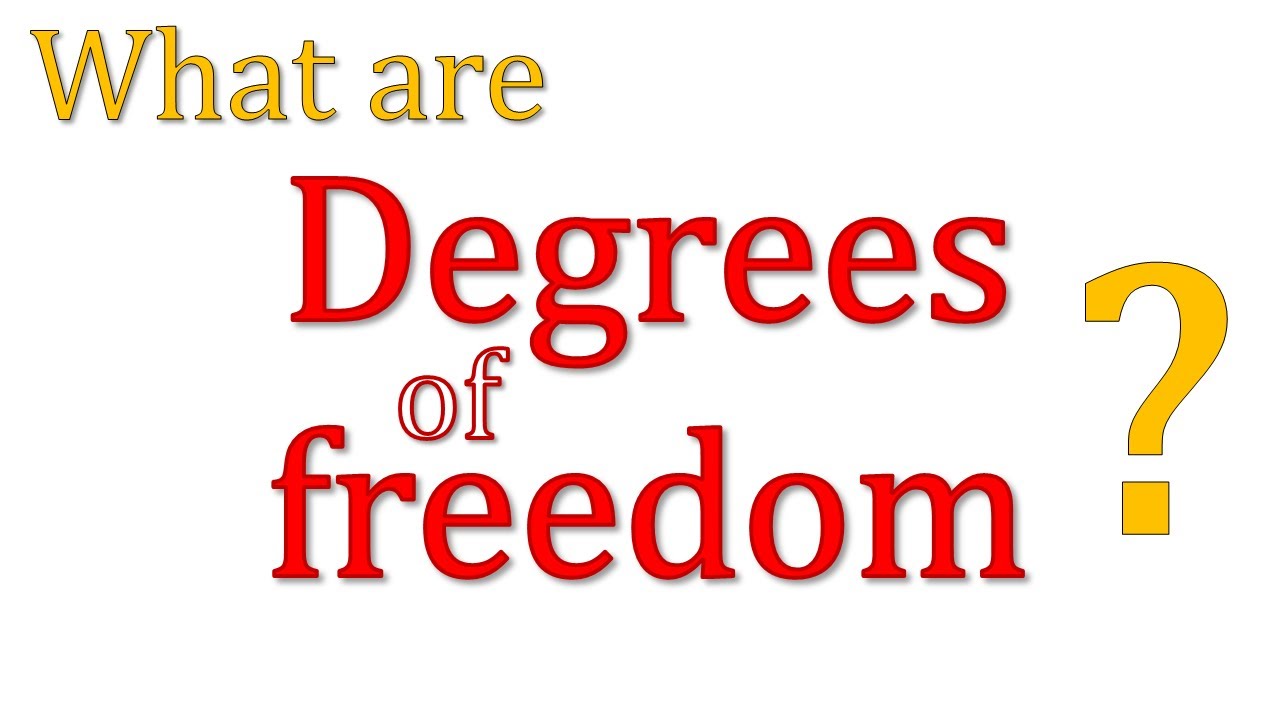
Statistical degrees of freedom - What are they REALLY?
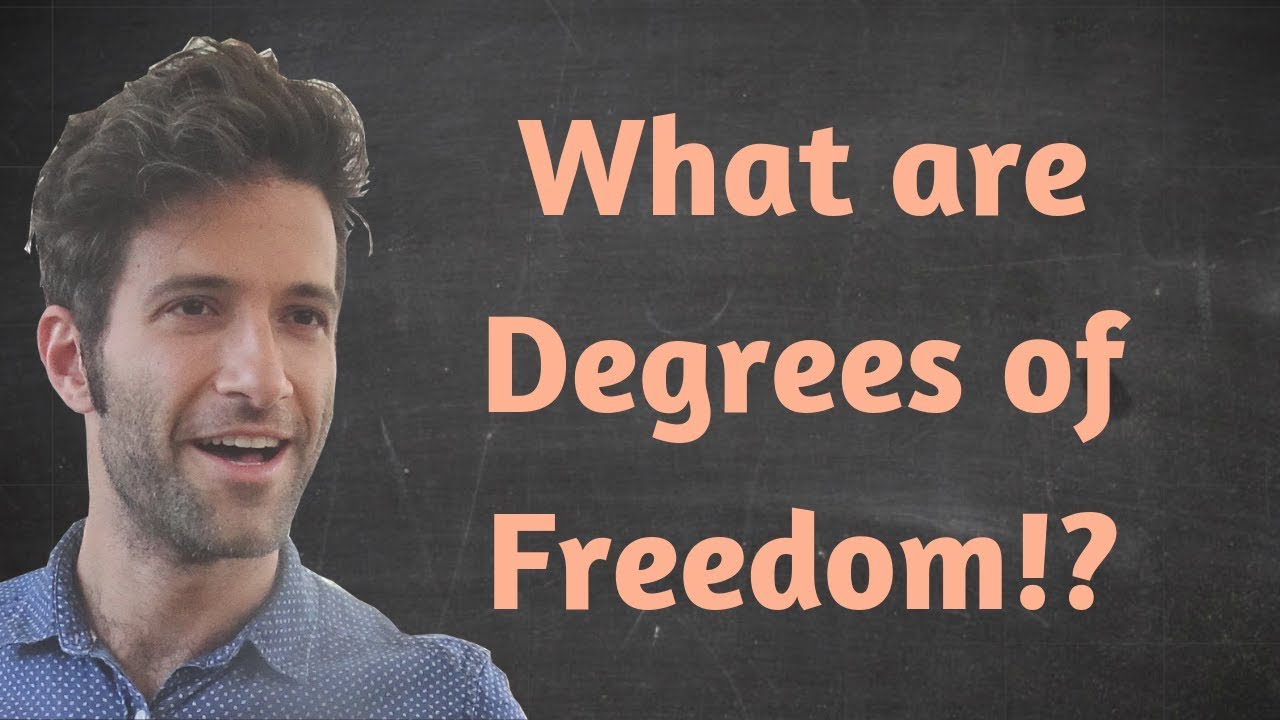
What are degrees of freedom?!? Seriously.
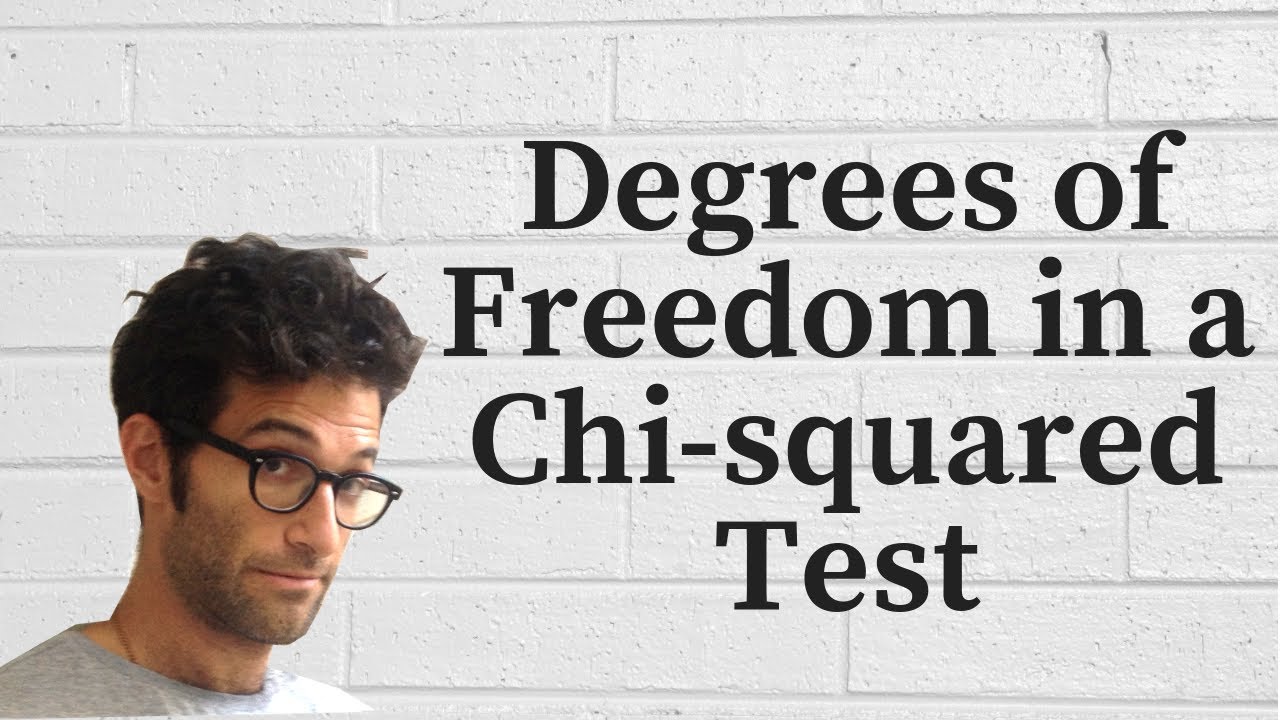
Degrees Of Freedom in a Chi-Squared Test
5.0 / 5 (0 votes)
Thanks for rating: