Two Factor ANOVA [without repeated measures]
TLDRThis video script delves into the intricacies of two-factor analysis of variance (ANOVA) without repeated measures. It explains the necessity of two-factor ANOVA when examining the impact of two categorical variables on a metric dependent variable. The script clarifies the differences between one-factor and two-factor ANOVA, discusses hypotheses, assumptions, and demonstrates online calculation and interpretation of results. It also elucidates the concept of interaction effects and provides a step-by-step guide to understanding the formulas involved in ANOVA.
Takeaways
- 📚 The video script introduces the concept of Two-Factor Analysis of Variance (ANOVA) without repeated measures, explaining its purpose and how it differs from One-Factor ANOVA.
- 🔍 A factor is defined as a categorical variable, such as gender, type of therapy, or field of study, which can be tested for its influence on a dependent variable like salary, blood pressure, or study duration.
- 📈 One-Factor ANOVA is used when testing the influence of a single categorical variable on a dependent variable, whereas Two-Factor ANOVA is employed when two categorical variables are considered for their impact.
- 🤔 The script discusses the importance of including additional variables in the analysis, such as the potential influence of education level on salary in addition to gender.
- 🧐 Three hypotheses are tested in Two-Factor ANOVA: the influence of the first factor, the second factor, and the interaction effect between the two factors on the dependent variable.
- 📝 The assumptions of Two-Factor ANOVA include the metric level of the dependent variable, nominal level of the independent variables, independence of measurements, homogeneity of variances, and normal distribution within groups.
- 📊 The script provides a step-by-step guide on how to perform Two-Factor ANOVA using an online tool, DataTab, including data entry, hypothesis testing, and interpretation of results.
- 📉 The results of ANOVA, including descriptive statistics and the Levene's test for equality of variances, are explained, with a focus on the significance of p-values in hypothesis testing.
- 🔄 The concept of interaction effects is explored through diagrams, illustrating scenarios with and without interaction between factors, and how it affects the dependent variable.
- 📘 Formulas for calculating ANOVA components, such as total sum of squares, sum of squares between, sum of squares for factors, interaction, and error, are explained in detail.
- 🔑 The final section of the script delves into how to calculate F-values and p-values, which are crucial for determining the statistical significance of the factors and their interaction in influencing the dependent variable.
Q & A
What is the main topic of the video?
-The main topic of the video is two-factor analysis of variance without repeated measures, discussing its requirements, differences from one-factor analysis, hypotheses, assumptions, and how to calculate and interpret the results.
What is the purpose of two-factor analysis of variance?
-The purpose of two-factor analysis of variance is to test whether there are differences between more than two independent samples divided between two variables or factors, and to determine if there is an interaction effect between these factors.
What is a factor in the context of analysis of variance?
-A factor in the context of analysis of variance is a categorical variable, such as gender, form of therapy, or field of study, which is used to categorize the samples in the study.
What is the difference between one-factor and two-factor analysis of variance?
-One-factor analysis of variance is used when there is only one categorical variable influencing the dependent variable, while two-factor analysis of variance is used when there are two categorical variables, allowing for the examination of their individual effects and any interaction between them.
What are the three null hypotheses tested in two-factor analysis of variance?
-The three null hypotheses tested are: 1) There are no significant differences between the groups of the first factor, 2) There are no significant differences between the groups of the second factor, and 3) There is no interaction effect between the two factors.
What assumptions are made in two-factor analysis of variance regarding the scale level of variables?
-The dependent variable should be measured on a metric scale, while the independent variables (factors) should be nominal or ordinal. Ordinal rank order is not used for ordinal variables to avoid losing information.
How is the independence assumption addressed in two-factor analysis of variance?
-The independence assumption is addressed by ensuring that measurements from one group are not influenced by measurements from another group. If measurements are not independent, an analysis of variance with repeated measures would be required.
What statistical test is used to check the assumption of homogeneity of variances?
-The Levene's test is used to check the assumption of homogeneity of variances, ensuring that the variances within the groups are approximately equal.
How can one calculate two-factor analysis of variance online?
-One can calculate two-factor analysis of variance online using platforms like DataDept.net by entering the data into the provided table and selecting the appropriate hypothesis test for the variables.
What does an interaction effect between two factors mean in the context of two-factor analysis of variance?
-An interaction effect between two factors means that the influence of one factor on the dependent variable is dependent on the level of the other factor. It indicates that the effect of one factor varies across the levels of the second factor.
How can one interpret the results of two-factor analysis of variance?
-The results of two-factor analysis of variance are interpreted by examining the p-values associated with each factor and their interaction. If the p-value is less than the significance level (commonly 0.05), the null hypothesis is rejected, indicating a significant effect or interaction. If the p-value is greater, the null hypothesis is retained, indicating no significant effect or interaction.
What is the significance of parallel lines in interaction effect diagrams?
-Parallel lines in interaction effect diagrams indicate that there is no interaction effect between the two factors. The influence of one factor on the dependent variable is consistent across the levels of the other factor.
How are the sums of squares calculated in two-factor analysis of variance?
-The sums of squares are calculated by determining the variance within each factor, the interaction between factors, and the error. This involves calculating the mean values of individual groups, the mean values of categories within each factor, and then using these to compute the sums of squares for each component.
What is the role of F-values in two-factor analysis of variance?
-F-values are used to determine the statistical significance of the variance explained by each factor and their interaction. They are calculated by dividing the variance attributed to each factor or interaction by the error variance. Higher F-values indicate a greater likelihood of rejecting the null hypothesis.
How are p-values determined in two-factor analysis of variance?
-P-values are determined using the F-values, the degrees of freedom for each factor and their interaction, and the F-distribution. They can be found using statistical tables or software, and are used to decide whether to reject or retain the null hypotheses.
Outlines
📊 Introduction to Two-Factor ANOVA
This paragraph introduces the concept of two-factor analysis of variance (ANOVA) without repeated measures, contrasting it with one-factor ANOVA. It explains the need for two-factor ANOVA when there are more than two independent samples categorized by two variables or factors. The paragraph outlines the purpose of the video, which includes explaining the hypotheses and assumptions of two-factor ANOVA, demonstrating how to calculate it using an online tool, interpreting results, and discussing interaction effects. It also differentiates between one-factor and two-factor ANOVA, emphasizing the role of categorical variables in forming groups for analysis.
🧐 Hypotheses and Assumptions in Two-Factor ANOVA
This section delves into the null and alternative hypotheses associated with two-factor ANOVA, which are three in each case, corresponding to the main effects of each factor and their interaction. It discusses the assumptions underlying ANOVA, such as the metric level of the dependent variable, the nominal scale of the independent variables, the independence of measurements, homogeneity of variances across groups, and normal distribution of data within groups. The paragraph also provides a practical example from a marketing department context to illustrate the application of two-factor ANOVA.
📈 Calculating and Interpreting Two-Factor ANOVA Results
The paragraph demonstrates how to perform a two-factor ANOVA using an online tool, DataTab, with a step-by-step guide. It explains the process of entering data, selecting variables, and conducting the analysis. The results, including descriptive statistics and Levene's test for equality of variances, are discussed. The significance of p-values in hypothesis testing is highlighted, with a focus on retaining or rejecting null hypotheses based on these values. The concept of interaction effects is introduced, with an explanation of how they can be visualized and interpreted in the context of the results.
🤔 Understanding Interaction Effects in ANOVA
This section provides a deeper understanding of interaction effects in two-factor ANOVA. It uses diagrams to illustrate how interaction effects can be observed when the influence of one factor on the dependent variable depends on the level of the other factor. The paragraph explains the concept of parallel lines indicating no interaction and how changes in the dependent variable associated with one factor vary depending on the level of the second factor, signifying an interaction effect.
🔢 Formulas and Calculations in Two-Factor ANOVA
The final paragraph focuses on the mathematical aspect of two-factor ANOVA, explaining the formulas used to calculate sums of squares for total, between, within, interaction, and error. It details the process of calculating means, variances, and F-values, which are essential for determining the statistical significance of the results. The paragraph also discusses the interpretation of these values in the context of the research question and how they can be used to explain the variance in the dependent variable.
Mindmap
Keywords
💡Two-Factor Analysis of Variance
💡Factor
💡Dependent Variable
💡Independent Samples
💡Null Hypothesis
💡Alternative Hypothesis
💡Interaction Effect
💡Assumptions
💡Data Tab
💡Significance Level
💡Sum of Squares (SS)
Highlights
The video discusses the differences between one-factor and two-factor analysis of variance without repeated measures.
Two-factor analysis of variance is used to test differences between more than two independent samples divided by two variables or factors.
A factor is a categorical variable, such as gender, therapy type, or field of study.
Analysis of variance is used to test the influence of categorical variables on a dependent variable like salary, blood pressure, or study duration.
In two-factor analysis, the group results come from the combination of the expressions of two factors.
The video explains the three null hypotheses and alternative hypotheses tested in two-factor analysis of variance.
Assumptions for two-factor analysis include metric level for the dependent variable and nominal for the independent variables.
The video demonstrates how to calculate two-factor analysis of variance online using DataTab.
Descriptive statistics and the Levine test for variance equality are presented as part of the analysis.
The interpretation of results, including p-values and their relation to the null hypotheses, is explained.
The concept of interaction effects between factors is introduced and explained.
An example from the marketing department of a bank is used to illustrate the application of two-factor analysis.
The video shows how to input data and select variables for hypothesis testing in DataTab.
Graphical representation of interaction effects and non-interaction effects is provided.
The video explains the calculation of sums of squares, variance, and F-values in two-factor analysis.
The importance of understanding the total variance, variance of factors, interaction, and error variance is discussed.
Formulas for calculating the different sums of squares and variances in two-factor analysis are provided.
The video concludes with a summary of the key points and an invitation to the next video.
Transcripts
Browse More Related Video
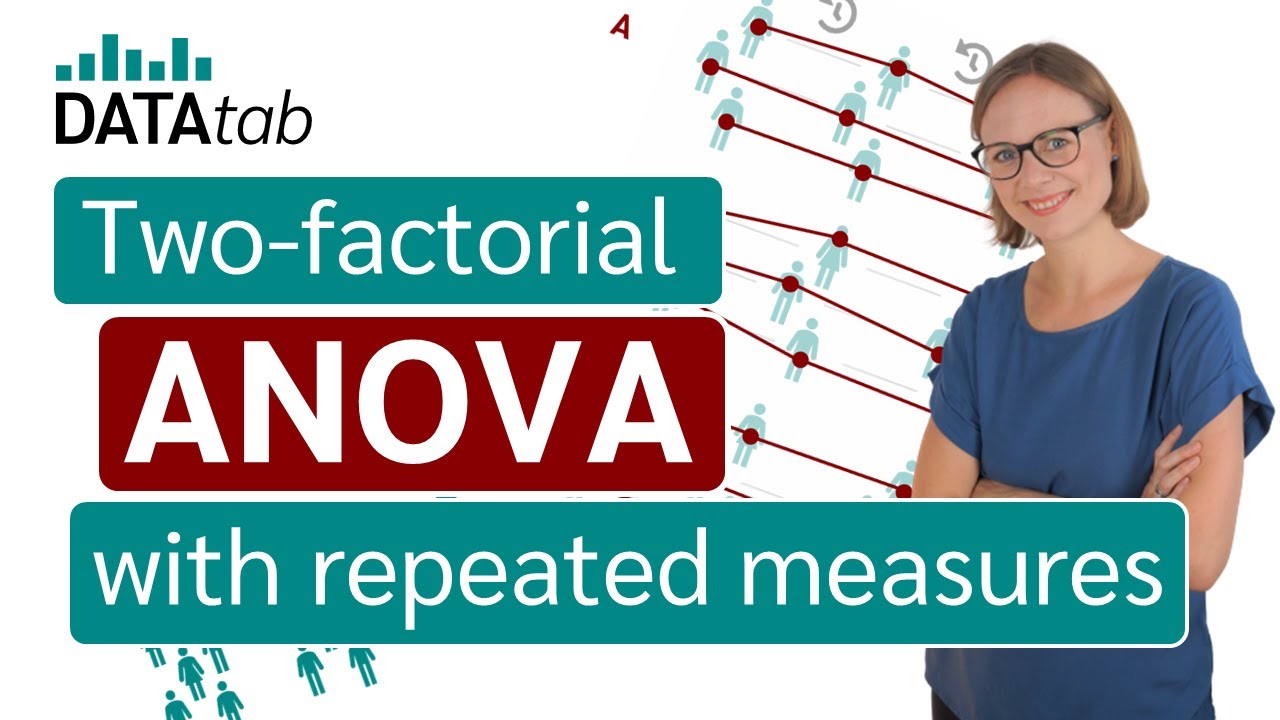
Two factor ANOVA with repeated measures
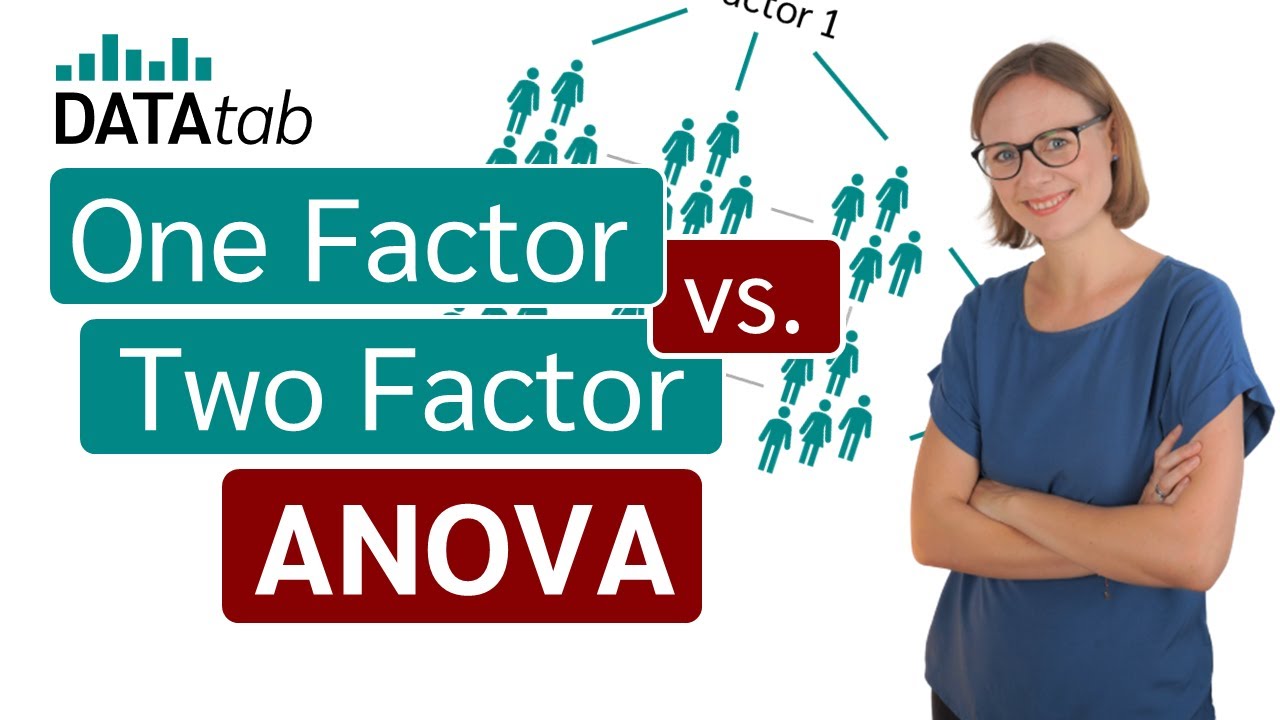
One Factor vs. Two Factor ANOVA [One Way vs Two Way]
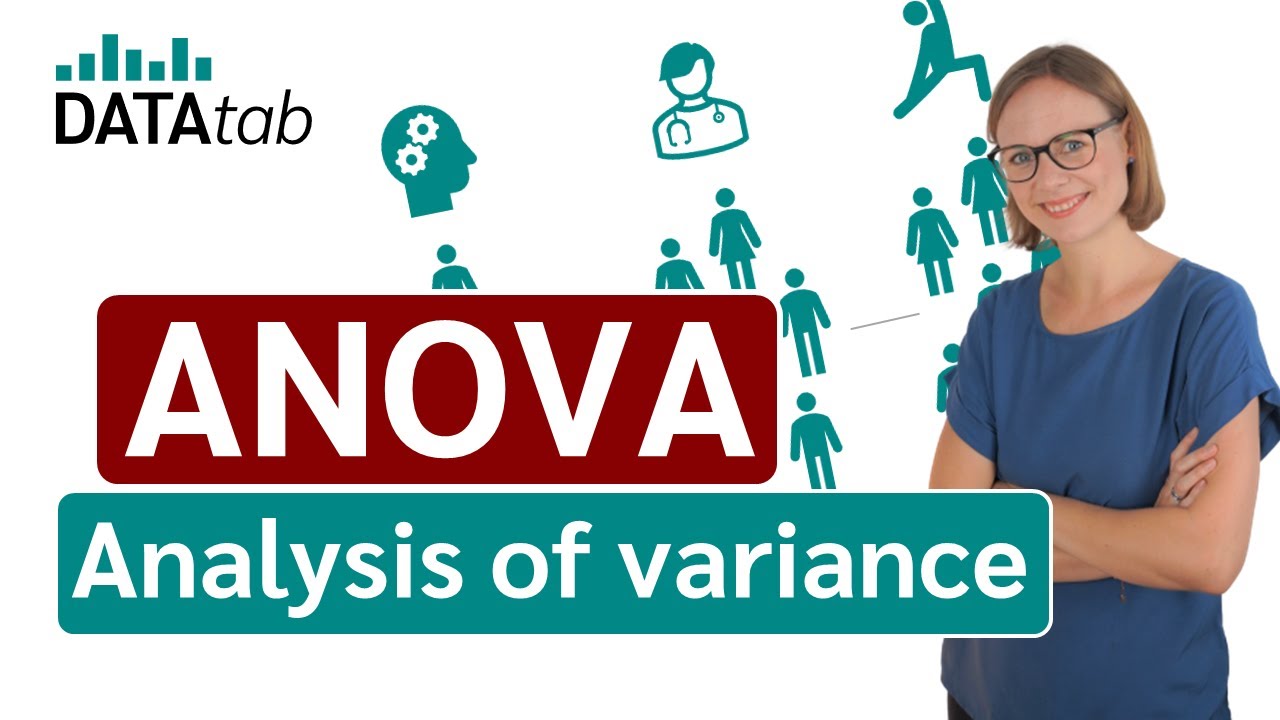
ANOVA (Analysis of variance) simply explained
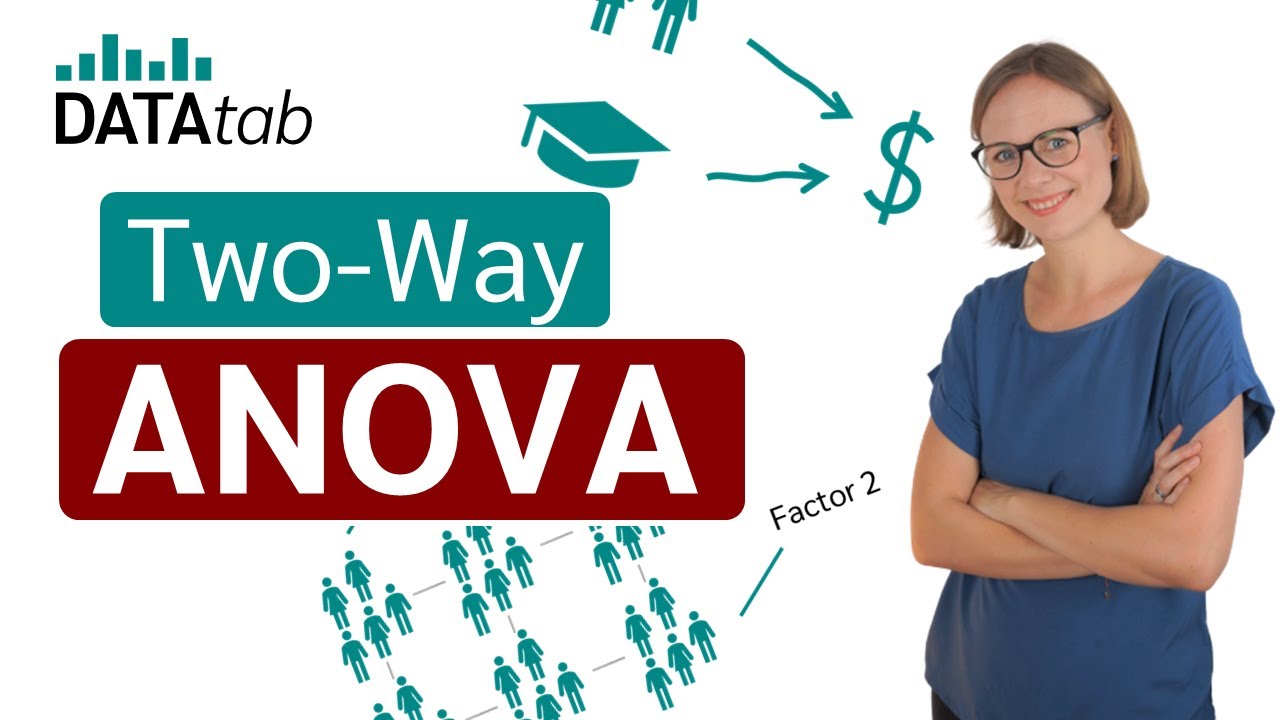
Two-Way ANOVA - Full Course

Two-way repeated measures ANOVA in SPSS: one-within, one-between (March 2020)
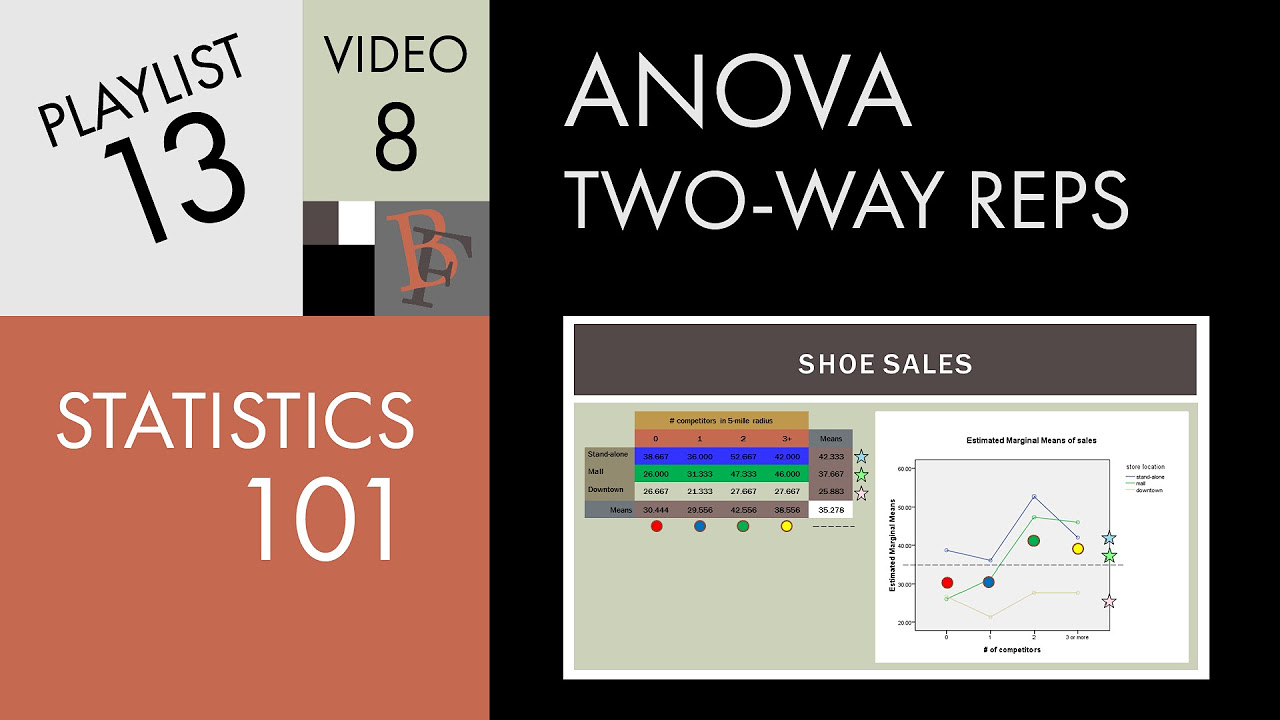
Statistics 101: Two-way ANOVA with Replication, Marginal Means Graphs
5.0 / 5 (0 votes)
Thanks for rating: