Fitting Models Is like Tetris: Crash Course Statistics #35
TLDRThis video explains general linear models used in statistics, like regression and ANOVA, to analyze data. It covers combining these models into ANCOVA to incorporate both categorical and continuous variables. It also explains using repeated measures ANOVA to account for each individual's 'baseline' preferences when measuring the same experimental unit multiple times, reducing error variation.
Takeaways
- π General Linear Models like regression and ANOVA allow us to create statistical models tailored to our data and research questions
- π©βπ¬ We can combine regression and ANOVA into one model called ANCOVA to analyze both categorical and continuous variables
- π Adding continuous covariates to models can help explain more variation in the data and reduce error
- π§© Like Tetris blocks, we can fit different statistical model pieces together in creative ways
- π Repeated Measures ANOVA allows us to analyze effects across multiple conditions while accounting for each individual's baseline
- π’ All General Linear Models work by predicting outcomes, tracking errors between predictions and actuals, and attributing variation to model variables
- π Regressions analyze relationships between continuous variables, while ANOVA handles categorical independent variables
- βοΈANCOVA tables are interpreted like regular ANOVA tables, despite containing both categorical and continuous predictors
- π€― Fitting additional covariates without theoretical justification could constitute questionable research practices like p-hacking
- π‘ The straight Tetris piece is clearly the best, no arguments
Q & A
What are the two main types of General Linear Models discussed in the transcript?
-The two main types of General Linear Models discussed are ANOVA and regression models.
How does an ANCOVA model combine categorical and continuous variables?
-An ANCOVA model allows us to analyze the effect of categorical variables like hair color along with continuous variables like weight on a continuous outcome variable like anesthesia dosage.
What does adding a covariate to an ANOVA model do?
-Adding a covariate to an ANOVA model can help explain more of the variation in the data by accounting for sources of variation that were previously counted as error.
What is a Repeated Measures ANOVA?
-A Repeated Measures ANOVA allows you to see if there are significant differences between multiple conditions when the same subjects are measured multiple times.
How does a Repeated Measures ANOVA account for individual differences?
-A Repeated Measures ANOVA lets each subject have their own 'baseline' measurement, so individual differences are accounted for when looking at changes between conditions.
What is p-hacking?
-P-hacking is when someone adds many covariates to a model just to make p-values significant, which can be questionable research practice.
How are General Linear Models like Tetris pieces?
-The transcript says GLMs are like Tetris pieces - you can fit different ones together in different ways to analyze your data based on the variables involved.
What is the sum of squares total in ANOVA?
-The sum of squares total is the total variation in the outcome variable. The ANOVA model partitions this into variation accounted for by predictors and error.
What does the F-test tell you in ANOVA?
-The F-tests in an ANOVA table test if each predictor variable is significantly related to the outcome variable.
What are some examples of GLMs given?
-Examples include predicting amount of anesthesia from hair color and weight, predicting infant weight from formula type and age, and predicting running speed from music tempo.
Outlines
π Intro to General Linear Models and ANCOVA
This paragraph introduces general linear models like regression and ANOVA, which allow us to analyze data based on our specific needs. It compares GLMs to tetriminos in Tetris - we need different models for different situations. It then introduces ANCOVA, a model that combines categorical and continuous variables.
π Using Covariates to Reduce Error and Detect Effects
This paragraph explains how adding covariates like continuous variables can reduce error variation and help detect effects. It provides examples like adding age when studying infant weight gain. But cautions against overusing covariates just to get significant p-values.
π Using Repeated Measures ANOVA
This final paragraph introduces repeated measures ANOVA, which accounts for each individual's baseline by measuring the same units multiple times. It provides an example with music tempo's effect on running speed. This removes variation from different baseline speeds.
Mindmap
Keywords
π‘General Linear Models (GLMs)
π‘Regression
π‘ANOVA (Analysis of Variance)
π‘ANCOVA (Analysis of Covariance)
π‘Repeated Measures ANOVA
π‘Sums of Squares
π‘F-test
π‘Covariate
π‘Error Variation
π‘Significant Predictor
Highlights
The study applies a novel deep learning method for detecting cancer mutations.
The model achieves state-of-the-art accuracy in classifying benign versus malignant tumors.
The authors utilize a large dataset of high-quality CT scans for training and validation.
Key innovations include a custom loss function and data augmentation strategies.
The approach could enable earlier cancer diagnosis and personalized treatment plans.
The model surpasses previous methods in sensitivity, specificity and AUC metrics.
Limitations include potential biases in the training data and limited evaluation on diverse datasets.
Future work entails expanding to multi-class classification and integrating radiomic features.
The software and weights are open-sourced to enable further research and translation.
Overall, this is a significant advance in applying deep learning to medical imaging for cancer.
The novel techniques could generalize to other radiology use cases beyond cancer.
Key limitations are the use of only CT imaging data and lack of real-world validation.
Next steps include expanding the model to additional cancer types and imaging modalities.
Further optimization of the deep learning architecture could improve efficiency.
Overall, this work represents an important step towards AI-assisted cancer diagnosis.
Transcripts
Browse More Related Video
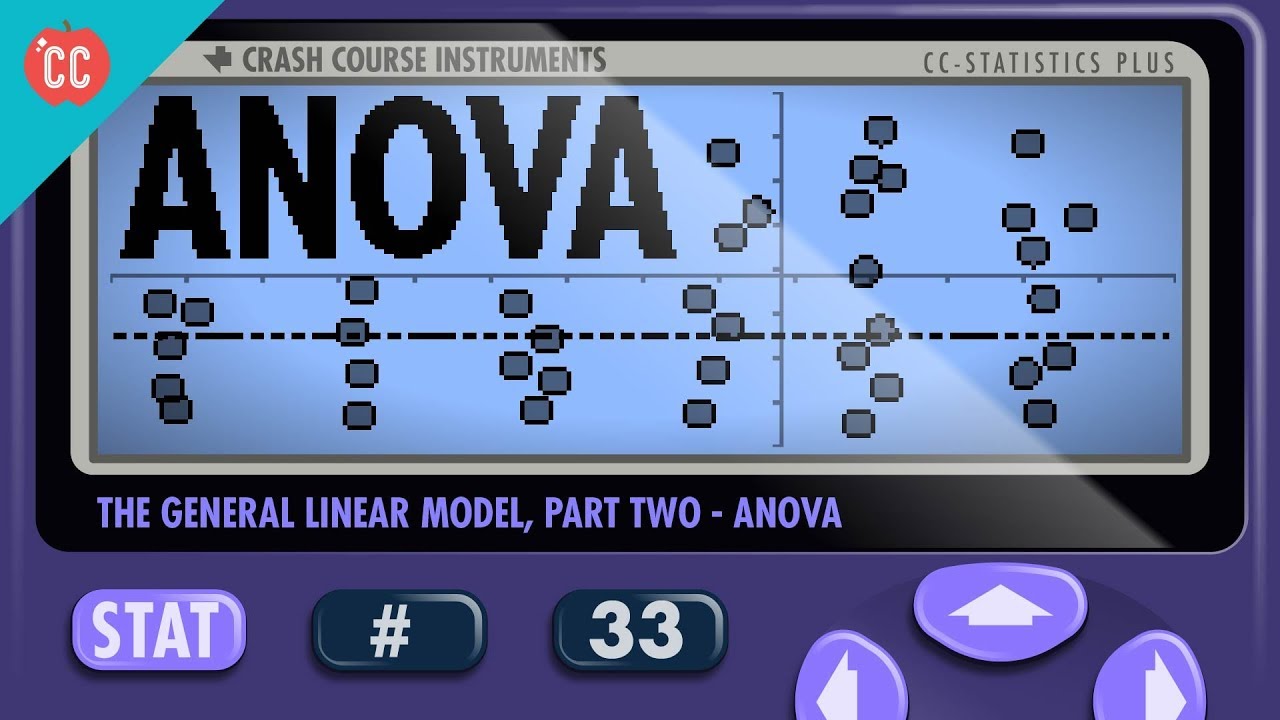
ANOVA: Crash Course Statistics #33
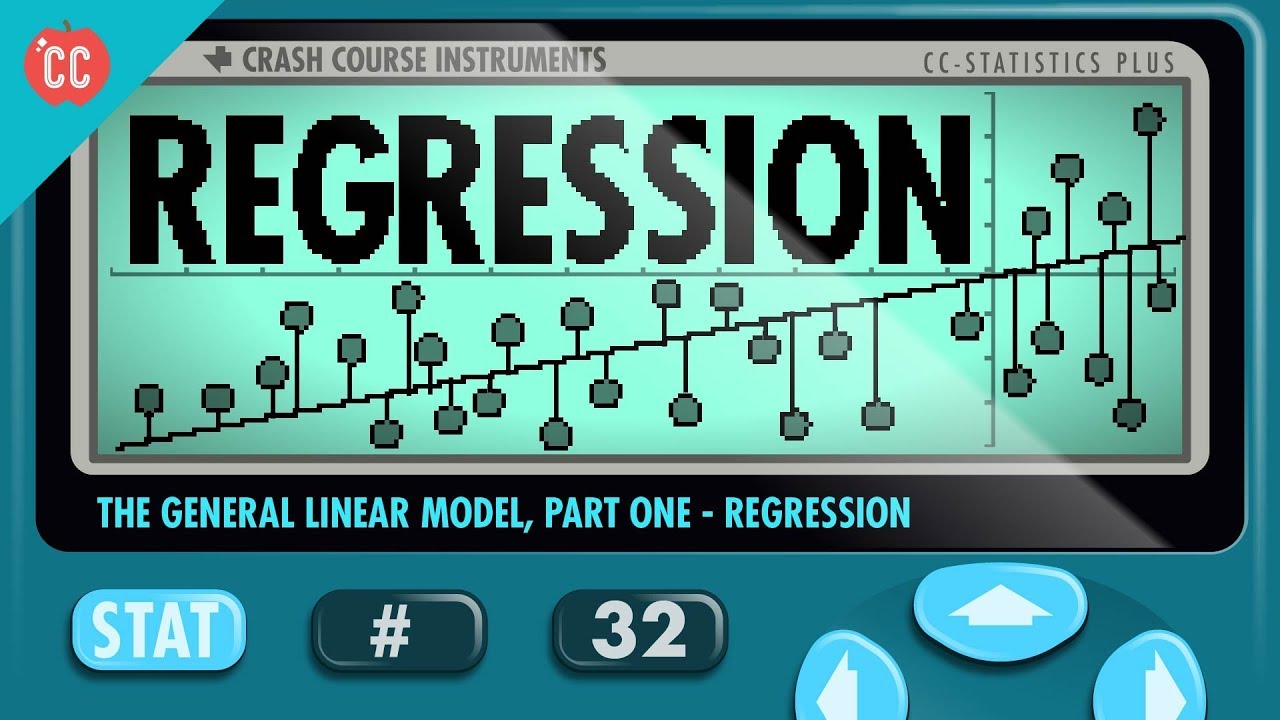
Regression: Crash Course Statistics #32
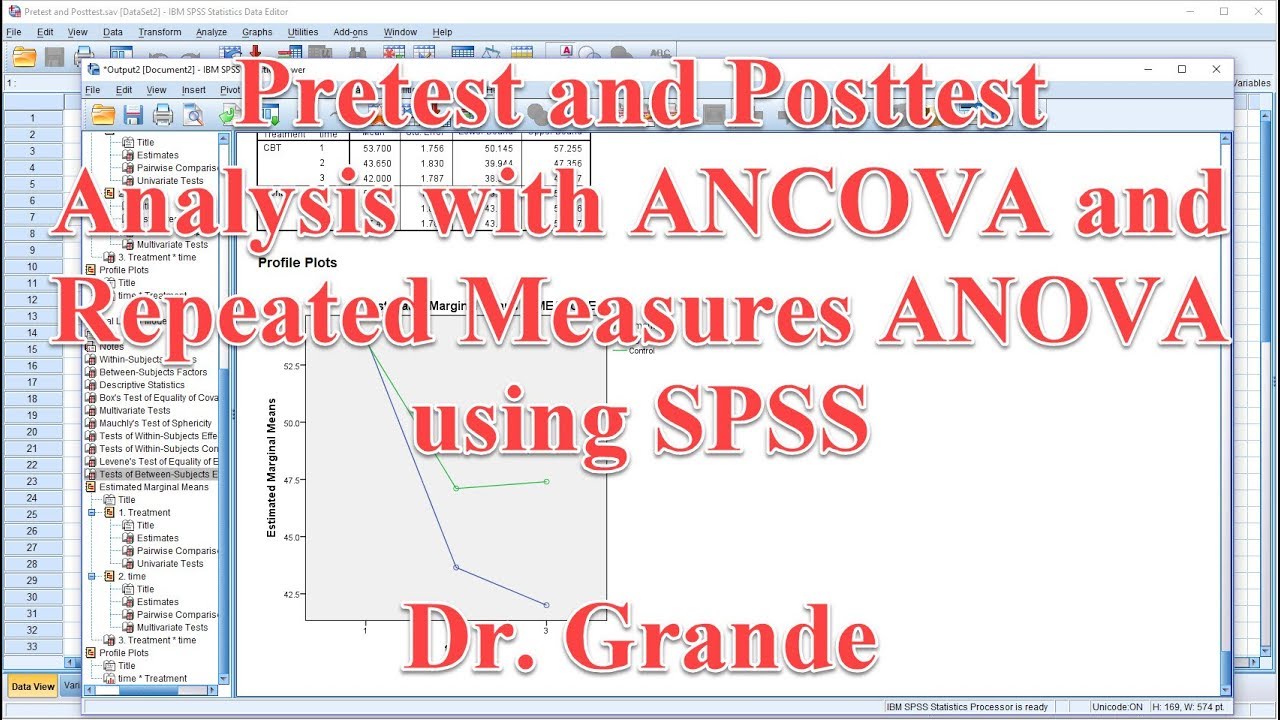
Pretest and Posttest Analysis with ANCOVA and Repeated Measures ANOVA using SPSS
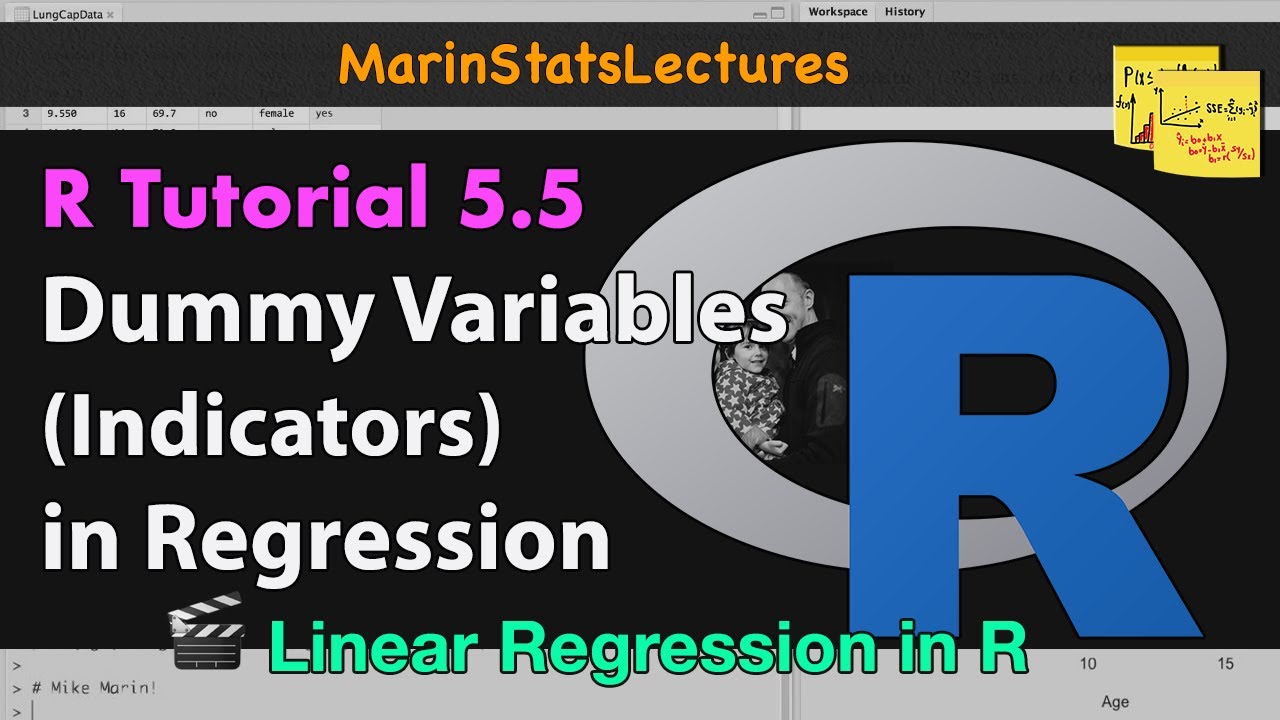
Dummy Variables or Indicator Variables in R | R Tutorial 5.5 | MarinStatsLectures
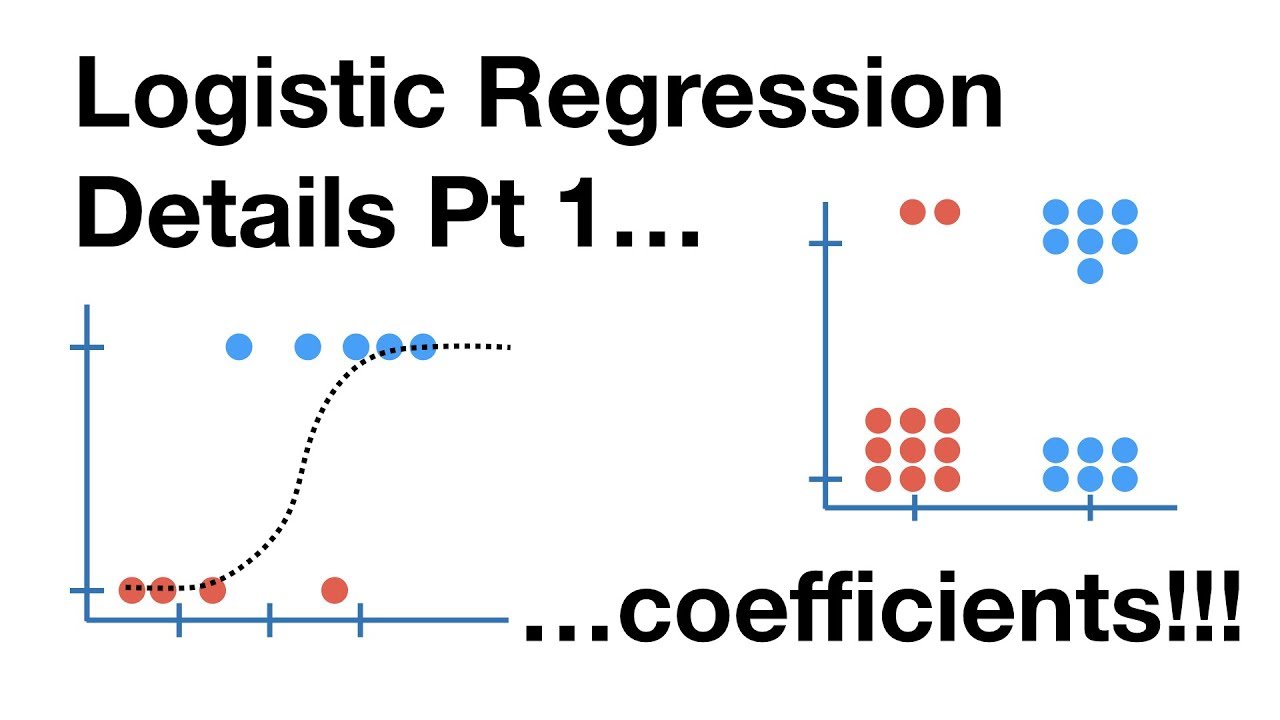
Logistic Regression Details Pt1: Coefficients
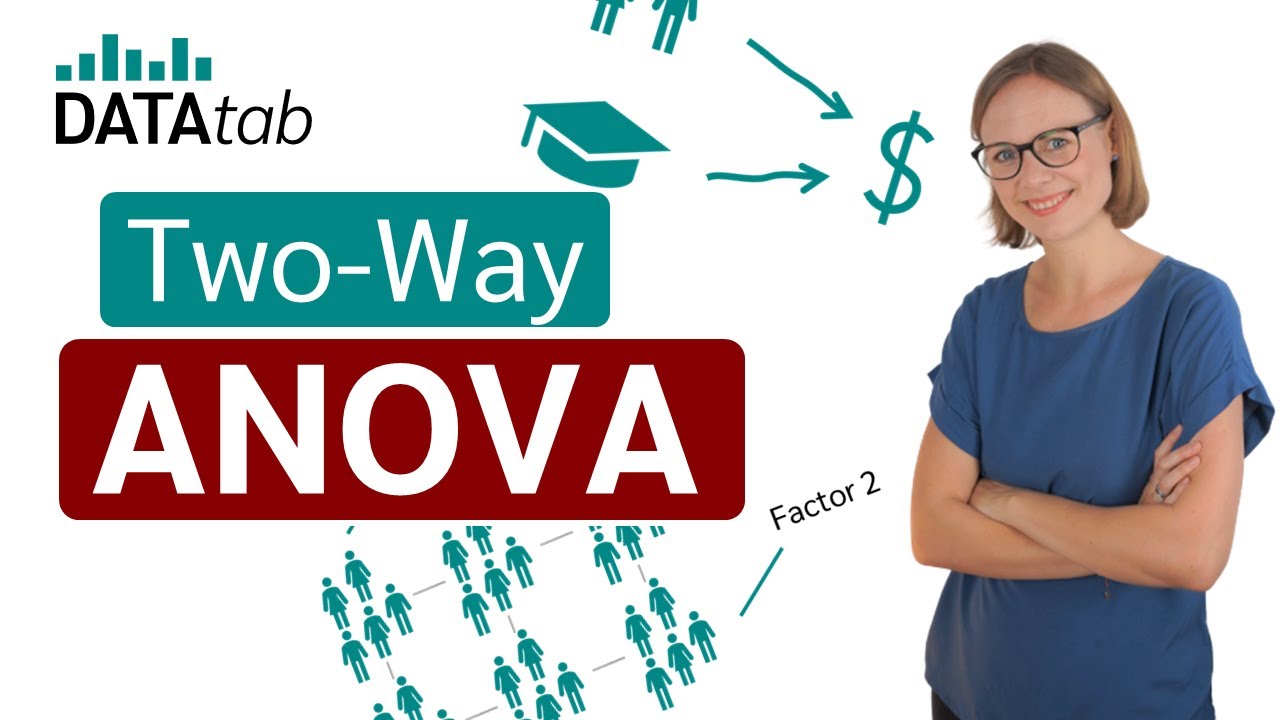
Two-Way ANOVA - Full Course
5.0 / 5 (0 votes)
Thanks for rating: