How To Identify Type I and Type II Errors In Statistics
TLDRThis educational video explores the fundamental concepts of Type 1 and Type 2 errors in statistics, crucial for understanding hypothesis testing. Type 1 error occurs when a true null hypothesis is incorrectly rejected, symbolized by alpha, while Type 2 error happens when a false null hypothesis is not rejected, represented by beta. The video also introduces the concept of test power, illustrated through a detailed table and examples involving scenarios like assessing a used car's safety and a criminal court case, to differentiate between these errors effectively. By examining the consequences of each error type through practical examples, the video aims to clarify these statistical concepts, enhancing viewers' grasp of hypothesis testing's nuances.
Takeaways
- π Type 1 error occurs when the null hypothesis is incorrectly rejected, even though it's true.
- π Type 2 error happens when failing to reject a false null hypothesis, essentially accepting a falsehood.
- π The probability of making a Type 1 error is denoted by alpha (Ξ±), while the probability of making a Type 2 error is denoted by beta (Ξ²).
- π The power of a test, represented as 1 - Ξ², indicates its ability to correctly reject a false null hypothesis.
- π A table can effectively summarize the outcomes and errors associated with hypothesis testing, aiding comprehension.
- π§ Through examples, distinguishing between Type 1 and Type 2 errors becomes clearer, especially in practical scenarios.
- π The consequences of Type 1 and Type 2 errors can vary significantly depending on the context, with some having more severe implications.
- π In legal or safety-related decisions, the distinction between Type 1 and Type 2 errors highlights the importance of accurate judgment.
- π Analyzing hypothetical situations can help understand the nuances of these errors and the critical role of hypothesis testing in decision-making.
- π» Engaging with example problems is a practical approach to grasp the concepts of Type 1 and Type 2 errors in statistics.
Q & A
What is a Type 1 error in statistics?
-A Type 1 error occurs when the null hypothesis is incorrectly rejected when it is actually true.
What does a Type 2 error represent in statistical analysis?
-A Type 2 error occurs when the null hypothesis is not rejected when it is actually false.
What is the symbol used to represent the probability of making a Type 1 error?
-The symbol Ξ± (alpha) represents the probability of making a Type 1 error.
How is the probability of making a Type 2 error represented?
-The probability of making a Type 2 error is represented by the symbol Ξ² (beta).
What is the power of a test in statistical terms?
-The power of a test is the probability of correctly rejecting a false null hypothesis, calculated as 1 - Ξ².
In the context of the provided example, what would be a Type 1 error regarding John's used car?
-A Type 1 error would be believing John's car is unsafe to drive when, in fact, it is safe.
What example is given for a Type 2 error in the context of John's used car?
-A Type 2 error example is thinking John's car is safe when it is actually not safe to drive.
Which error is considered to have a greater consequence in the example of John's used car?
-The Type 2 error has a greater consequence because it can potentially cost John his life if he believes his unsafe car is safe to drive.
In the criminal court case example, what constitutes a Type 1 error?
-A Type 1 error in the criminal court case is believing the defendant is guilty when they are actually innocent.
Which type of error is deemed to have a greater consequence in the criminal court case example?
-The Type 1 error is considered to have a greater consequence because it involves punishing an innocent person, which is seen as a very serious mistake.
Outlines
π Introducing Type 1 and Type 2 Errors
This paragraph introduces the concepts of type 1 and type 2 errors that are important to understand in statistics. It explains that a type 1 error occurs when the null hypothesis is incorrectly rejected when it is actually true. A type 2 error occurs when the null hypothesis is not rejected when it is actually false. Key probabilities related to these errors are defined, including alpha, beta, and power.
π Distinguishing Between Type 1 and 2 Errors
This paragraph provides clear examples to distinguish between type 1 and type 2 errors. It emphasizes that a type 1 error occurs when the null hypothesis is true but incorrectly rejected, while a type 2 error occurs when the null hypothesis is false but fails to be rejected. An example problem is analyzed step-by-step to identify which statements represent each type of error.
π± Comparing the Consequences of Errors
This paragraph examines which type of error, 1 or 2, has greater consequences. It argues that a type 2 error often has more severe real-world impacts, using an example where incorrectly assuming a car is safe to drive could lead to a deadly accident. It also analyzes an example court case, concluding that wrongfully convicting an innocent defendant (type 1 error) is worse than failing to convict a guilty defendant (type 2 error).
Mindmap
Keywords
π‘Type 1 Error
π‘Type 2 Error
π‘Null Hypothesis
π‘Alpha (Ξ±)
π‘Beta (Ξ²)
π‘Power of the Test
π‘Decision Making
π‘Rejecting the Null Hypothesis
π‘Accepting the Null Hypothesis
π‘Consequences of Errors
Highlights
Explanation of Type 1 and Type 2 errors in statistics
Type 1 error occurs when null hypothesis is incorrectly rejected
Type 2 error happens when a false null hypothesis is not rejected
Alpha represents the probability of making a Type 1 error
Beta is the probability of making a Type 2 error
Power of the test is defined as 1 minus Beta
Creation of a table to summarize Type 1 and Type 2 errors
Illustration of decision outcomes when null hypothesis is true or false
Example problem illustrating Type 1 and Type 2 errors in a practical scenario
Distinguishing between good and bad decisions in hypothesis testing
Analysis of a Type 1 error scenario using an example
Explanation of Type 2 error with a practical example
Comparison of consequences between Type 1 and Type 2 errors
Application of Type 1 and Type 2 error concepts in a criminal court case example
Assessment of greater consequences between Type 1 and Type 2 errors in different contexts
Concluding remarks on the importance of understanding Type 1 and Type 2 errors in statistics
Transcripts
Browse More Related Video
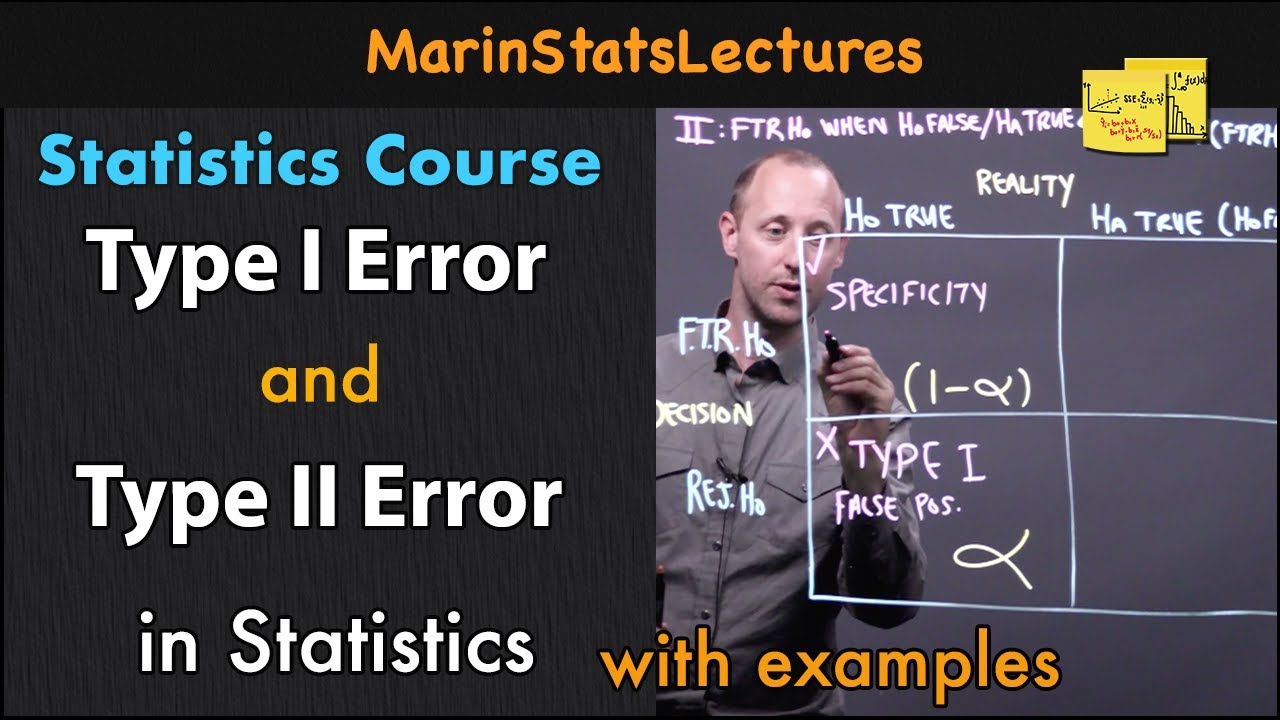
Errors and Power in Hypothesis Testing | Statistics Tutorial #16 | MarinStatsLectures
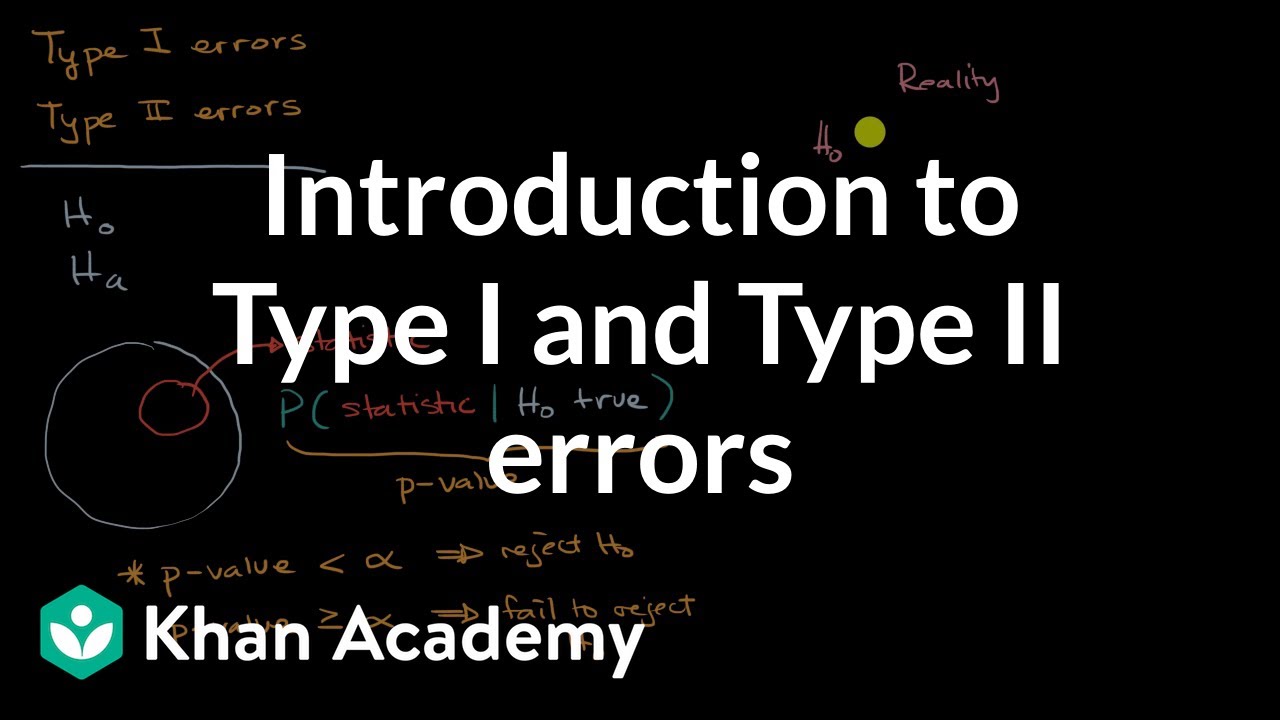
Introduction to Type I and Type II errors | AP Statistics | Khan Academy
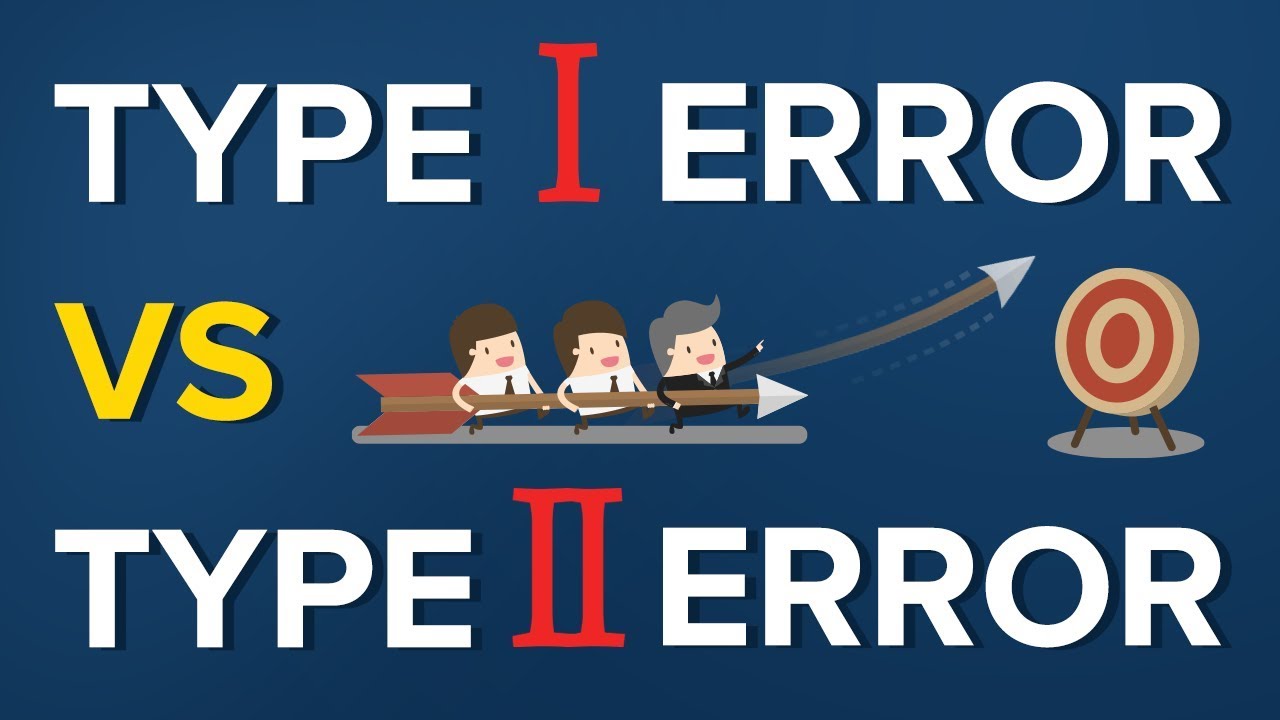
Type I error vs Type II error
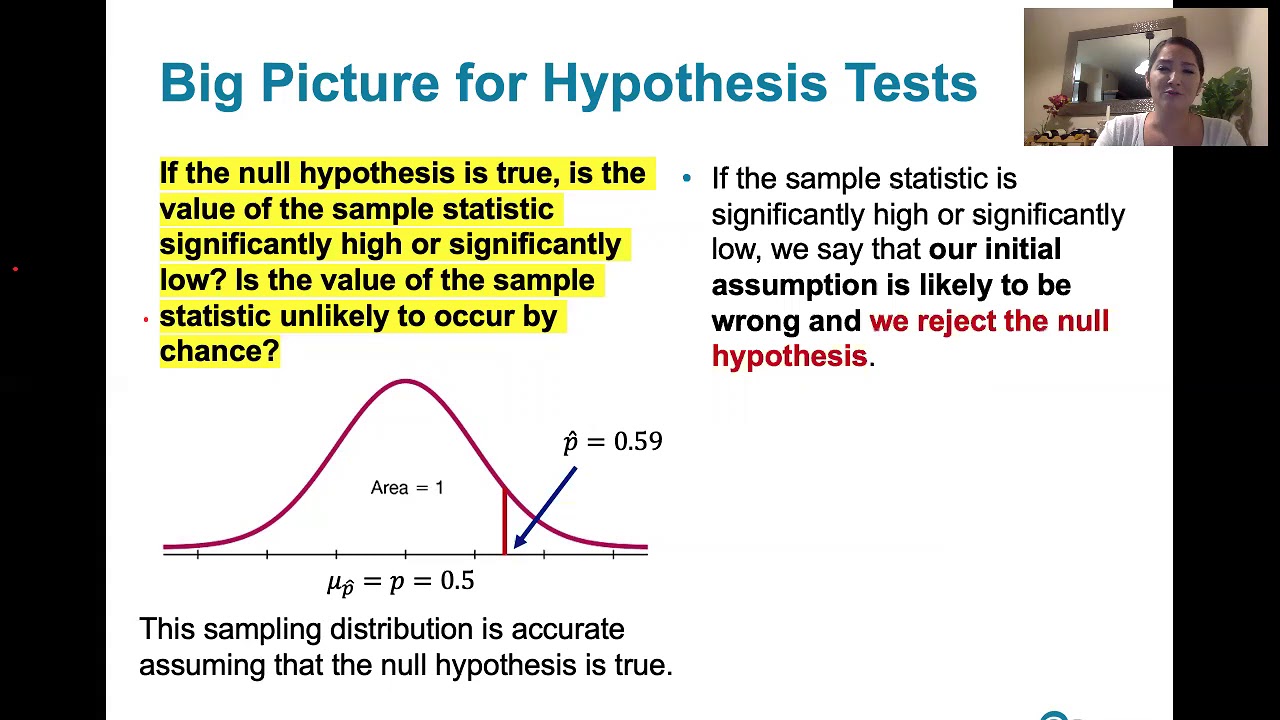
8.1.2 Basics of Hypothesis Testing - Significance Level, Type I and Type II Error
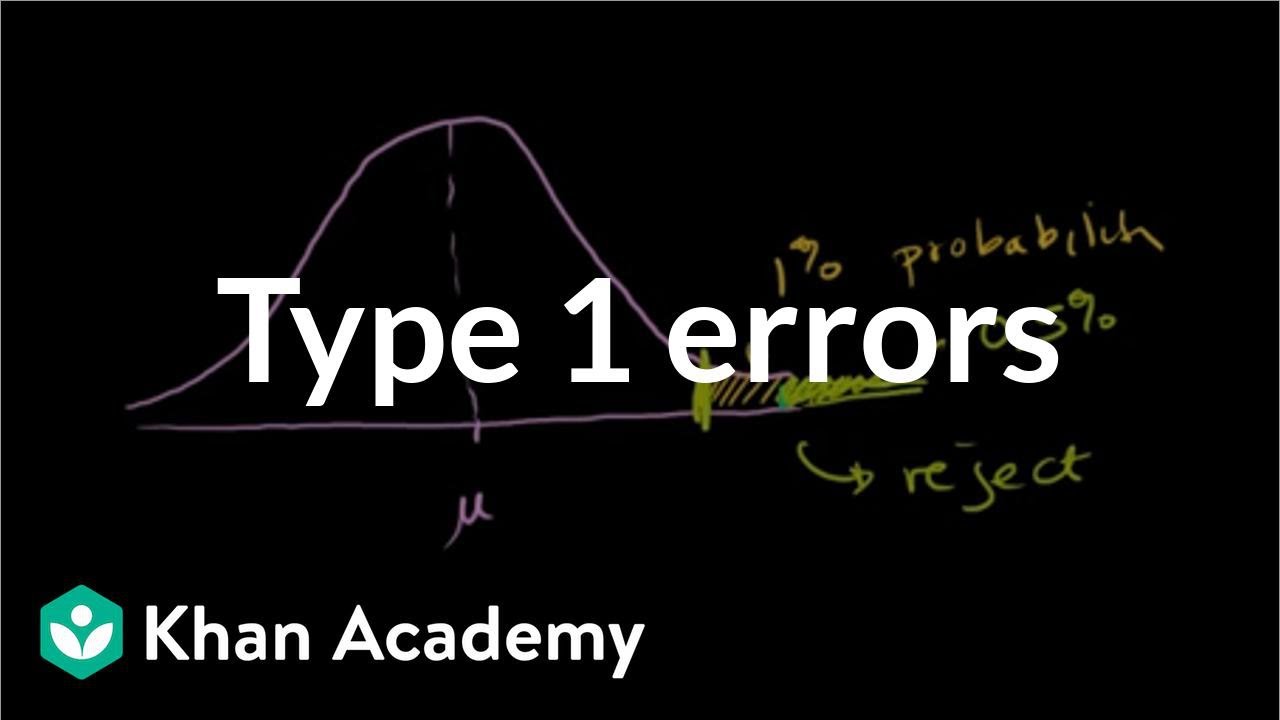
Type 1 errors | Inferential statistics | Probability and Statistics | Khan Academy

Type 1 (Alpha) vs. Type 2 (Beta) Error
5.0 / 5 (0 votes)
Thanks for rating: