Law of Large Numbers
TLDRThe law of large numbers states that as the number of trials or samples increases, the observed average will approach the expected theoretical average. This concept is illustrated through examples of flipping coins and measuring weights. With more coin flips, the observed probability of heads approaches 0.5. And by measuring more people's weights in a town, the observed average weight converges between 161-162 pounds. So taking larger samples leads to more accurate estimates of a population's true parameters.
Takeaways
- 😀 The law of large numbers states that as you increase the number of trials in an experiment, the observed average will approach the theoretical average.
- 😊 For example, when flipping a coin multiple times, the percentage of heads should approach 50% as you flip more coins.
- 🤓 You can use the law of large numbers to estimate characteristics about a population by taking larger and larger samples.
- 😎 For instance, you can estimate the average weight of people in a town by measuring more and more people.
- 🧐 As the sample size increases, the observed average weight converges towards the true average.
- 🤔 Selecting just one person may give an extreme weight that isn't representative.
- 😇 But increasing the sample size gives a better estimate of the true average weight.
- 🤠 The key is that increasing the number of trials or sample size makes the observed average closer to the expected.
- 😉 Even though chance plays a role, extremes get less likely in larger samples.
- 😃 So the more trials, flips, or people measured, the closer statistics will be to theoretical values.
Q & A
What is the law of large numbers?
-The law of large numbers states that as the number of trials or experiments increases, the average of the results obtained gets closer and closer to the expected theoretical average or true mean.
Why does tossing more coins lead to the observed probability getting closer to 0.5?
-There is a 50% probability of getting heads when tossing a fair coin. As more coins are tossed, the observed probability tends to get closer and closer to the true 50% probability according to the law of large numbers.
How can the law of large numbers be used to estimate population parameters?
-By taking larger and larger samples from a population, the sample averages get closer and closer to the true population parameter according to the law of large numbers. This allows population parameters to be estimated from samples.
What was the observed probability after tossing 10 and 100 coins?
-After tossing 10 coins, the observed probability was 0.4 or 40%. After tossing 100 coins, the observed probability was 0.49 or 49%.
Why is taking just one sample not enough to estimate the average weight?
-A single sample may give an extreme outlier value for the weight. More samples are needed for the average weight to converge to the true population average weight.
What was the range of average weights as more people were sampled?
-With samples of 5, 10, 50, 100, 200, 500, and 1000 people, the average weights ranged from 154 to 163 pounds, getting closer to 161-162 pounds with more samples.
What are the two possibilities when flipping a fair coin?
-The two possible outcomes when flipping a fair coin are heads or tails, with a 50% probability of each on any given flip.
Why does the observed probability get closer to 0.5 as more coins are tossed?
-According to the law of large numbers, as the sample size increases, the observed probability will approach the expected theoretical probability. For a fair coin it is 0.5 or 50% heads.
What is an example of an extreme weight value for one sample?
-Examples of extreme weight values from one sample could be someone who is obese at 230 pounds or underweight at 110 pounds, unlike the town's average.
How many people were assumed to live in the town?
-It was stated that there were 20,000 people assumed to be living in the town used as an example.
Outlines
📈 Explanation and Examples of Law of Large Numbers
This paragraph provides an explanation of the law of large numbers using an example with coin flips. As the number of coin flips increases, the observed probability of heads approaches the theoretical probability of 0.5. More examples are provided to show that as sample size increases, the sample average converges to the true population average.
📊 Using Law of Large Numbers to Estimate Population Parameters
This paragraph provides another example related to estimating the average weight of people in a town. As the sample size increases, the average weight converges to between 161-162 pounds. This demonstrates how the law of large numbers can be used to estimate population parameters from samples.
Mindmap
Keywords
💡probability
💡sample
💡converge
💡experiments
💡trials
💡outliers
💡parameters
💡observed average
💡theoretical average
💡sample size
Highlights
The law of large numbers states that as you increase the number of trials, the observed probability approaches the theoretical probability.
Flipping a fair coin has a 50% (0.5) chance of getting heads. But in a small number of trials you may not observe exactly 0.5.
As you flip more coins, the observed probability gets closer and closer to the theoretical 0.5 probability.
The more trials performed, the closer the observed results match the expected theoretical results. This is the essence of the law of large numbers.
You can use the law of large numbers to estimate characteristics of a whole population by taking samples.
With small samples, the average can vary significantly from sample to sample.
As the sample size increases, the average converges towards the true population average.
Large samples better approximate the actual population average than small samples.
Selecting just one individual could give an extreme outlier that misrepresents the population.
The more trials performed, the closer the observed probability gets to the expected theoretical probability.
The law of large numbers allows estimating unknown population parameters from samples.
Small samples lead to more variability in estimates compared to large samples.
As sample size grows, sample averages converge to the true population parameter.
Large samples provide better estimates of population characteristics than small samples.
Single observations may be unrepresentative extremes that skew results.
Transcripts
Browse More Related Video
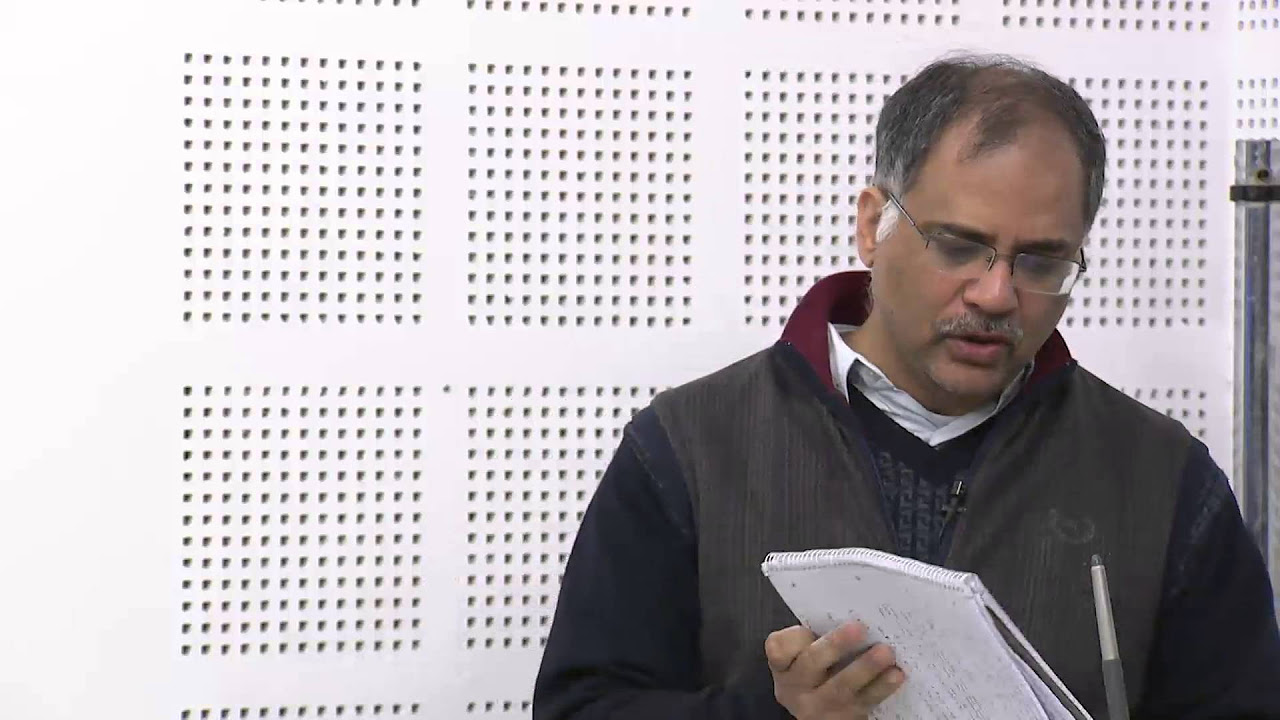
Lecture 5: Law of Large Numbers & Central Limit Theorem

Bunnies, Dragons and the 'Normal' World: Central Limit Theorem | The New York Times

Why “probability of 0” does not mean “impossible” | Probabilities of probabilities, part 2
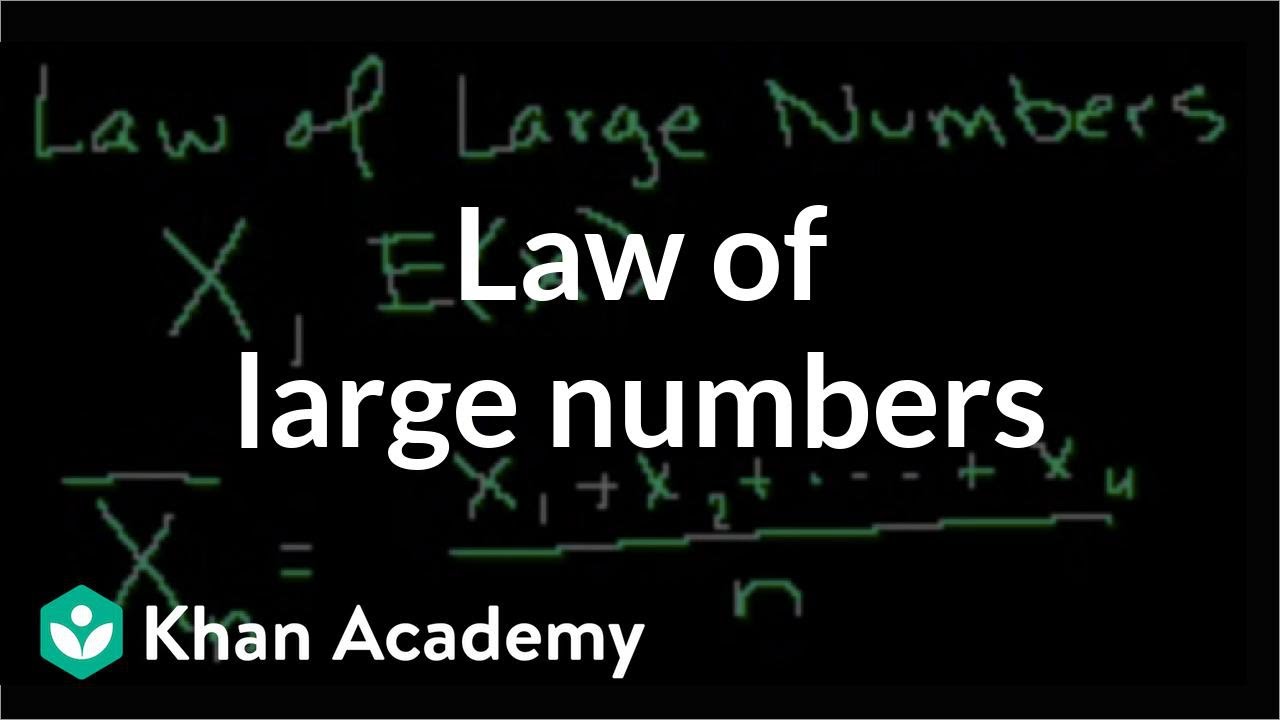
Law of large numbers | Probability and Statistics | Khan Academy

Compound Probability of Independent Events - Coins & 52 Playing Cards
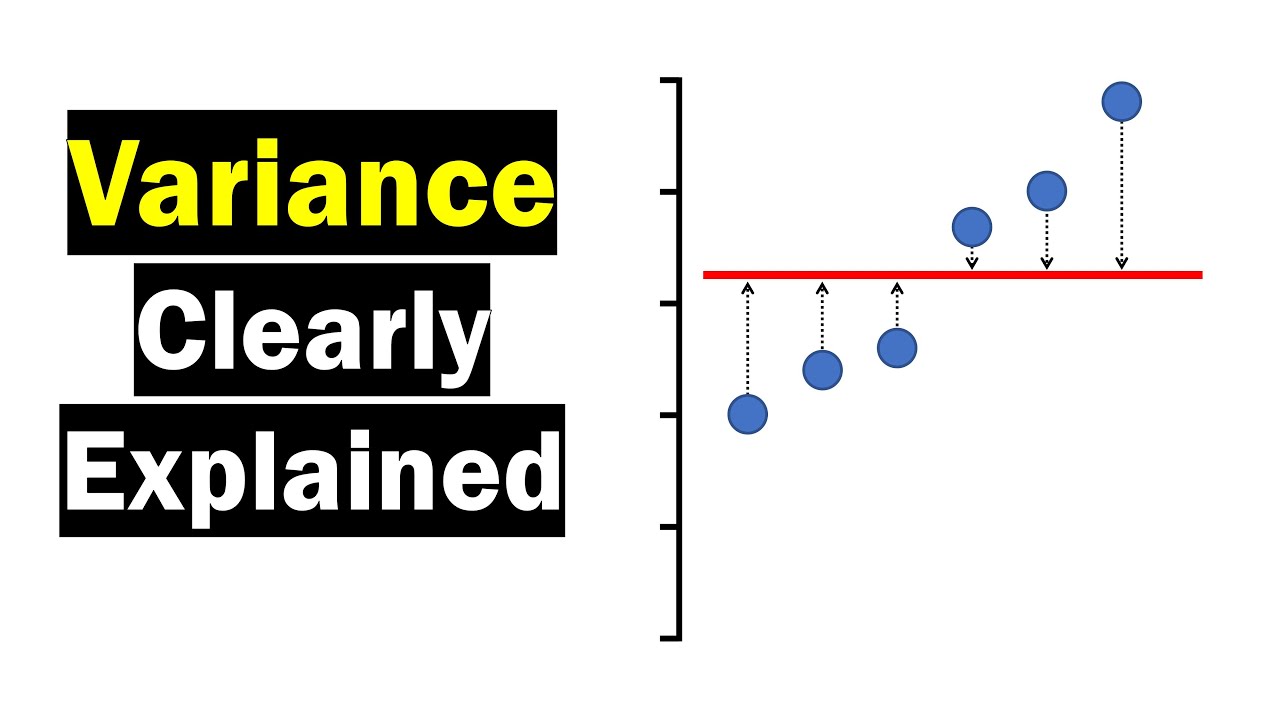
Variance - Clearly Explained (How To Calculate Variance)
5.0 / 5 (0 votes)
Thanks for rating: