Plots for Two Variables | Statistics Tutorial | MarinStatsLectures
TLDRThis script discusses various plots for visualizing relationships between different types of variables: categorical and numeric, two categorical, and two numeric. It explains side-by-side box plots for one categorical and one numeric variable, highlighting an example with skin cancer and sun exposure. For two categorical variables, it describes side-by-side bar charts, stacked bar charts, and mosaic plots, using smoking and lung cancer as an example. Finally, for two numeric variables, scatter plots are introduced, with age and height as an example. The script also briefly mentions analytical techniques like t-tests, ANOVA, chi-squared tests, and correlations for different variable types.
Takeaways
- π The video discusses various plots to visualize relationships between different types of variables: categorical and numeric, two categorical, and two numeric.
- π For one categorical and one numeric variable, side-by-side box plots can be used to explore associations, such as the hours spent in the sun related to skin cancer.
- π Analytic techniques like two-sample t-tests and analysis of variance are applicable for analyzing relationships between one categorical and one numeric variable.
- π― When there's no association, as with biological sex and body temperature, side-by-side plots will show similar distributions.
- π For two categorical variables, side-by-side bar charts, stacked bar charts, and mosaic plots are effective in illustrating associations, such as the link between smoking and lung cancer.
- π Chi-squared tests, Fisher's tests, and calculating odds ratios and rate ratios are methods used to analyze relationships between two categorical variables.
- π Mosaic plots are particularly useful as they convey additional information, such as the proportion of the sample that belongs to each category.
- π― A mosaic plot without association would form a cross pattern, indicating similar proportions across categories.
- π Scatter plots (XY plots) are used to visualize relationships between two numeric variables, showing trends or correlations, like age and height in children.
- π For two numeric variables, analytic techniques include Pearson's or Spearman's correlation, and simple linear regression.
- π In adults, there's typically no association between age (20-65) and height, resulting in a scatter plot with no discernible pattern.
- π The video encourages viewers to subscribe for more content and provides a brief overview of various data visualization and analysis methods.
Q & A
What is the purpose of using plots to describe the relationship between variables?
-The purpose of using plots is to visualize and explore whether or not two variables are associated and the nature of that association.
What are the two types of variables that can be analyzed with plots?
-The two types of variables that can be analyzed with plots are categorical and numeric.
What is a side by side box plot and when is it used?
-A side by side box plot is used to compare the distribution of a numeric variable across different categories of another categorical variable. It is used when there is one categorical and one numeric variable.
How can a side by side box plot reveal an association between variables?
-A side by side box plot can reveal an association by showing differences in the distribution of the numeric variable among the categories of the categorical variable.
What are some plots that can be used to describe the relationship between two categorical variables?
-Some plots that can be used for two categorical variables include side by side bar charts, stacked bar charts, and mosaic plots.
What does a mosaic plot add in terms of information compared to a stacked bar chart?
-A mosaic plot adds the extra information of the proportion of the sample that belongs to each category of the categorical variable, which is shown by the width of the bars in the plot.
How does a scatter plot (XY plot) help in understanding the relationship between two numeric variables?
-A scatter plot helps by displaying the individual data points and revealing patterns such as correlations, trends, or absence of association between the two numeric variables.
What statistical tests can be used to analyze the relationship between one categorical and one numeric variable?
-Statistical tests for one categorical and one numeric variable include two sample t-tests and analysis of variance.
What are some analytical methods for examining the relationship between two categorical variables?
-Analytical methods for two categorical variables include chi-squared tests, Fisher's exact tests, odds ratios, and rate ratios.
What types of analyses are appropriate for two numeric variables?
-For two numeric variables, analyses such as Pearson's or Spearman's correlation, and simple linear regression can be used.
How does the distribution of body temperatures for males and females illustrate a lack of association?
-If the distribution of body temperatures for males and females looks pretty similar, it suggests that there is no significant difference between the two groups, indicating a lack of association between biological sex and body temperature.
Outlines
π Exploring Relationships with Plots
This paragraph introduces the concept of using plots to visualize the relationship between two variables, one categorical and one numeric, and between two categorical variables. It emphasizes the importance of plots in understanding associations and provides an example of side by side box plots to illustrate the association between skin cancer and sun exposure. The paragraph also briefly mentions upcoming discussions on analytic techniques and the dependency of the type of plots and analysis methods on the variable types.
π Comparing Categorical Variables with Mosaic Plots
This paragraph delves into the details of comparing two categorical variables using different types of plots, such as side by side bar charts and stacked bar charts. It provides a detailed example using smoking habits and lung cancer incidence to demonstrate how these plots can reveal associations. The paragraph then introduces mosaic plots as a preferred method due to their ability to convey additional information about the proportion of the sample, as well as the association between variables. The example illustrates the difference between smokers and non-smokers in terms of lung cancer rates, and contrasts this with a scenario where no association is present.
Mindmap
Keywords
π‘plots
π‘categorical variables
π‘numeric variables
π‘association
π‘side by side box plots
π‘stacked bar charts
π‘mosaic plots
π‘scatter plots
π‘chi-squared test
π‘Pearson's correlation
π‘analysis methods
Highlights
Overview of different plots for describing relationships between variables.
Importance of subscribing and enabling notifications for new videos.
Association between variables can be visualized through plots.
Analytic techniques for analyzing relationships between variables.
Categorical vs. numeric variables and their respective plots.
Side by side box plots for one categorical and one numeric variable.
Example of skin cancer and sun exposure hours.
No association example with biological sex and body temperature.
Side by side bar charts for two categorical variables.
Association example with smoking and lung cancer.
Stacked bar chart as an alternative to side by side bar charts.
Mosaic plot for additional information on associations.
Mosaic plot example with smoking, lung cancer, and sample proportions.
No association mosaic plot appearance.
Scatter plot (XY plot) for two numeric variables.
Association example with age and height in children.
No association in adults' age and height between 20 to 65.
Analytic methods for one categorical and one numeric: t-tests, ANOVA.
Analytic methods for two categorical: chi-squared, Fisher's test, odds ratios.
Analytic methods for two numeric: Pearson's, Spearman's correlation, regression.
Transcripts
Browse More Related Video

Stacked and Grouped Bar Charts and Mosaic Plots in R |R Tutorial 2.6| MarinStatsLectures
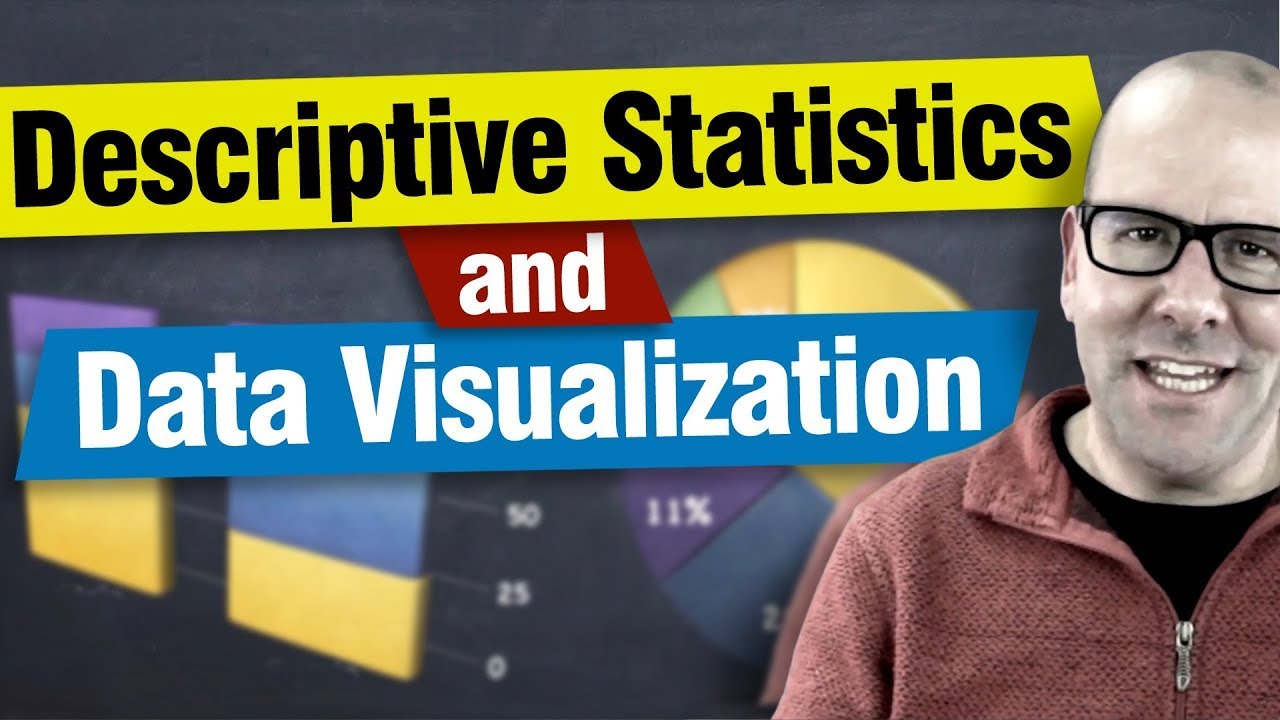
Descriptive statistics and data visualisation. An introduction to statistics and working with data
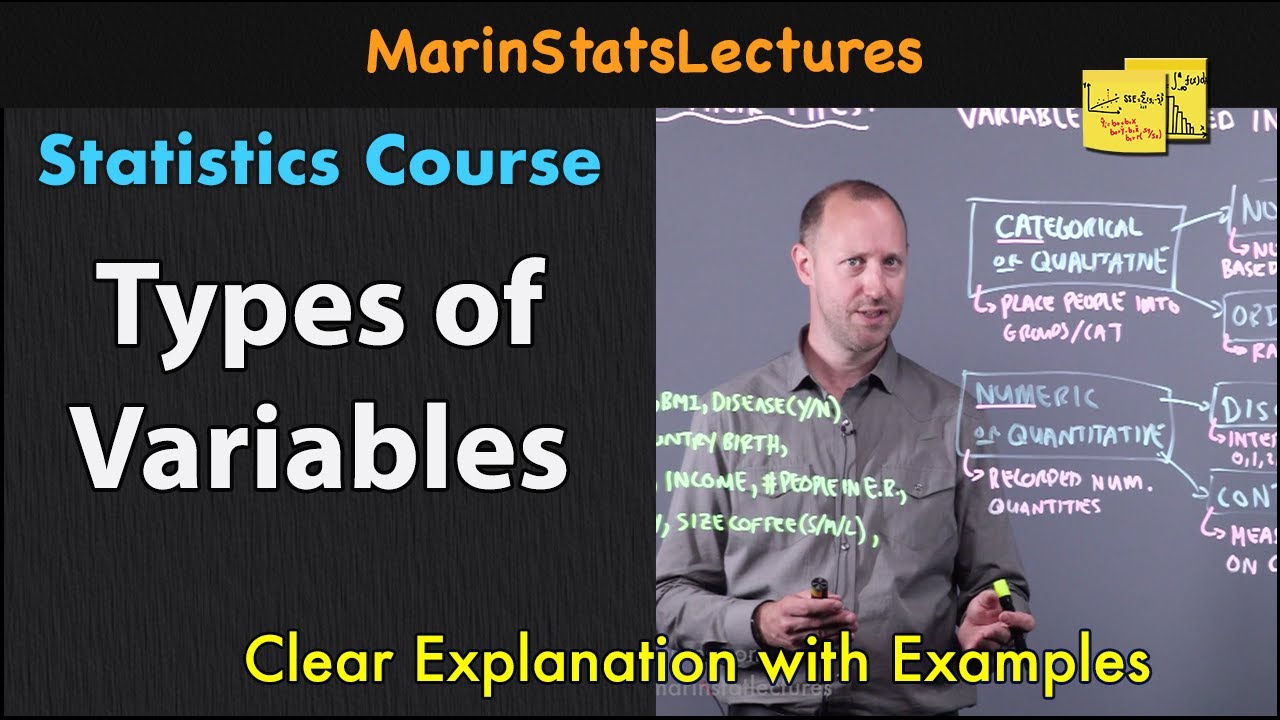
Variables and Types of Variables | Statistics Tutorial | MarinStatsLectures
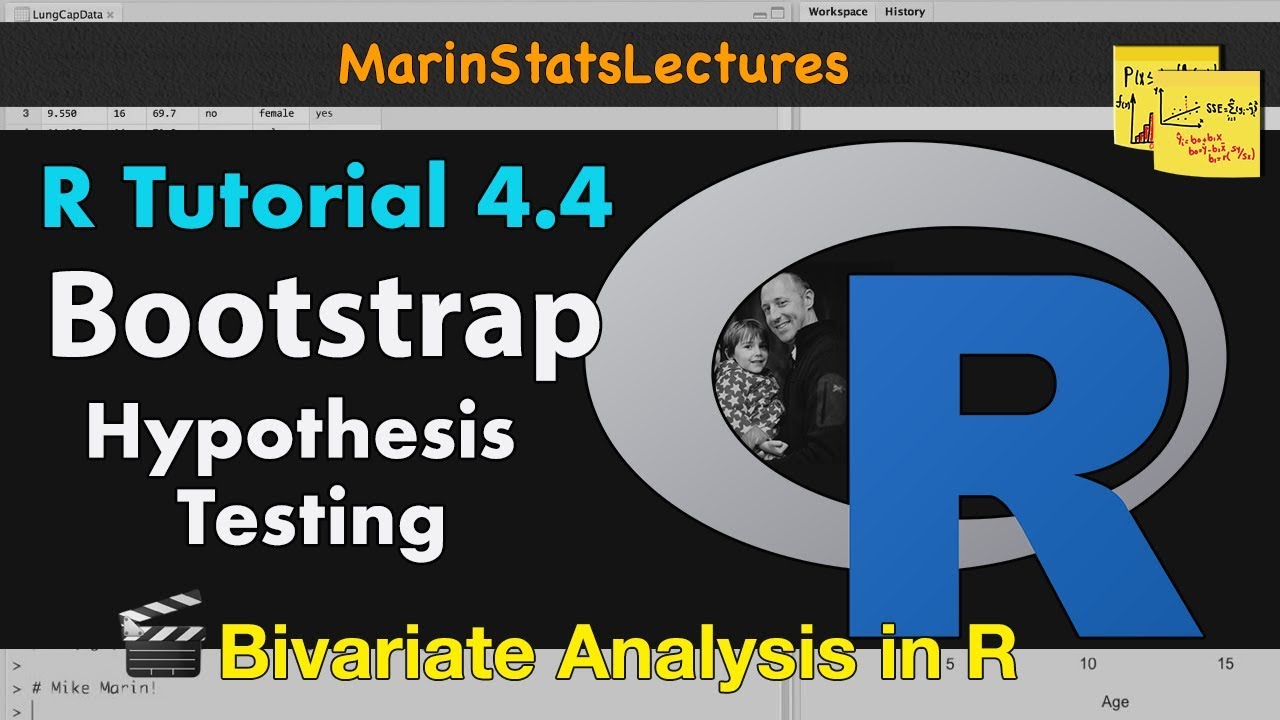
Bootstrap Hypothesis Testing in R with Example | R Video Tutorial 4.4 | MarinStatsLecutres

Histograms and Density Plots for Numeric Variables | Statistics Tutorial | MarinStatsLectures
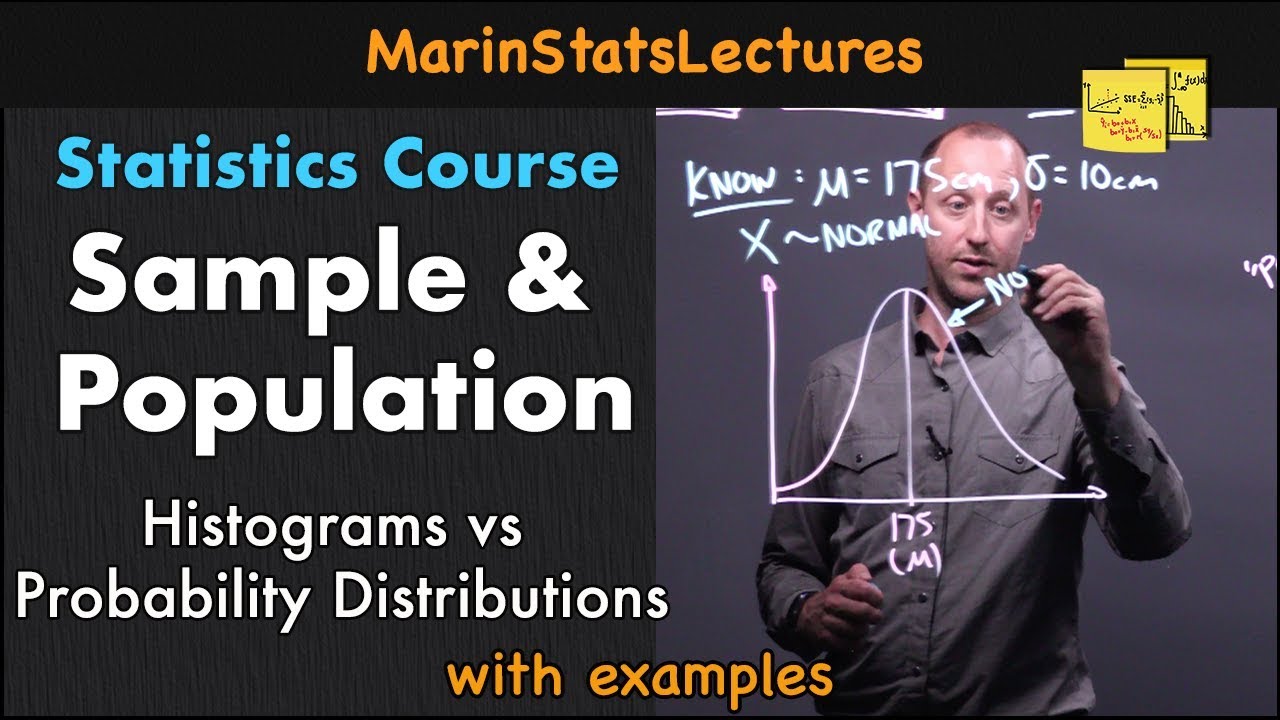
Sample and Population in Statistics | Statistics Tutorial | MarinStatsLectures
5.0 / 5 (0 votes)
Thanks for rating: