Calculus Chapter 4 Lecture 43 Probability Densities
TLDRIn this calculus lecture, Professor Greist explores the concept of probability densities, moving beyond the assumption of fair probability to address scenarios with inherent biases. He introduces probability density functions (PDFs), which quantify the likelihood of outcomes, using examples like light bulb failures and customer service wait times. The lecture delves into exponential and uniform PDFs, highlighting their mathematical properties and applications. It also touches on the Gaussian or normal PDF, a ubiquitous function in statistics, and hints at its significance in representing the convergence of numerous individual decisions.
Takeaways
- 📚 The lecture introduces the concept of probability density functions (PDFs) as a way to represent non-uniform probabilities where certain outcomes are more likely than others.
- 📉 A PDF, denoted by \( \rho \), is a function that must be non-negative and integrate to 1 over its domain, representing the likelihood of outcomes.
- 💡 The integral of a PDF over its domain equals 1, which is a crucial property for calculating probabilities of outcomes within subsets of the domain.
- 🕒 An example of a PDF is the exponential function \( e^{-\alpha t} \), where \( t \) is time and \( \alpha \) is a positive constant, representing the likelihood of an event occurring over time.
- 🔍 The concept of fairness in probability is contrasted with non-uniform probabilities, with fairness implying a uniform PDF where all outcomes are equally likely.
- 🎲 In the context of discrete outcomes, such as rolling a die, the uniform PDF is a constant value representing the probability of each outcome, which is 1 divided by the number of possible outcomes.
- ⏳ For continuous outcomes, the probability of a specific point is 0, but the probability of a range of outcomes can be calculated by integrating the PDF over that range.
- 📊 The probability of an outcome within a subset of the domain is the ratio of the integral of the PDF over that subset to the integral over the entire domain.
- 🍦 The Gaussian or normal PDF is a special type of PDF that is widely applicable, characterized by its bell-shaped curve and given by the formula \( \frac{1}{\sqrt{2\pi}} e^{-\frac{x^2}{2}} \).
- 🧩 Gaussian distributions can be translated and rescaled, and they arise naturally as limits of certain binomial processes, such as the distribution of binomial coefficients in Pascal's triangle.
- 🔮 The lecture concludes by emphasizing the importance of PDFs in probability theory and their role in calculating probabilities through integration.
Q & A
What is a probability density function (PDF)?
-A probability density function (PDF) is a function over a domain that tells you what outcomes are more likely than others. It satisfies two criteria: the function is non-negative, and the integral of the function over its domain equals 1.
How is a fair or uniform probability different from a biased probability?
-A fair or uniform probability implies that any point is as likely as any other point to be chosen at random, leading to a uniform distribution. A biased probability indicates that some outcomes are more likely than others, which is represented by a non-uniform PDF.
What does the integral of a PDF over its domain represent?
-The integral of a PDF over its domain represents the total probability, which must equal 1.
Can you give an example of a PDF for a biased scenario?
-Yes, an example of a PDF for a biased scenario is an exponential function representing the likelihood of light bulbs failing over time. The PDF might be of the form Rho(T) = e^(-alpha*T), where T is time and alpha is a positive constant.
What must be true about the constant in a uniform PDF over a domain D?
-In a uniform PDF over a domain D, the constant must be 1 divided by the volume of the domain, ensuring that the integral of the PDF over D equals 1.
How do you calculate the probability of a discrete outcome, such as rolling a die?
-For a discrete outcome like rolling a die, the probability of each outcome is 1 divided by the number of possible outcomes. For a six-sided die, each outcome has a probability of 1/6.
What is the probability of a randomly chosen point in a continuous domain landing exactly at a specific point?
-The probability of a randomly chosen point in a continuous domain landing exactly at a specific point is 0.
How do you determine the probability of a randomly chosen point lying within a subset of a continuous domain?
-The probability of a randomly chosen point lying within a subset of a continuous domain is determined by integrating the PDF over that subset.
What is a Gaussian (or normal) PDF, and why is it important?
-A Gaussian (or normal) PDF is of the form Rho(x) = (1/sqrt(2*pi)) * e^(-x^2/2). It is important because it appears frequently in statistics and natural phenomena, often representing the distribution of values around a mean.
How do you compute the probability of an event given a non-constant PDF?
-To compute the probability of an event given a non-constant PDF, you integrate the PDF over the domain of the event.
Outlines
📚 Introduction to Probability Densities
Professor Greist begins lecture 43 by introducing the concept of probability densities, which are used to represent the likelihood of outcomes when probabilities are not uniform. He explains that a probability density function (PDF), such as the exponential distribution for light bulb failures, must be non-negative and integrate to 1 over its domain. The lecture uses the exponential PDF as an example, showing how to adjust it to meet the criteria of a PDF. The professor also contrasts this with the uniform PDF, which assigns equal probability to all outcomes within a domain, and explains how the integral of the PDF over a subset of the domain can be used to calculate the probability of an event occurring within that subset.
🎲 Understanding Discrete and Continuous Probability Densities
In this section, the professor explores the differences between discrete and continuous probability densities. He uses examples such as rolling a die and flipping a coin to illustrate discrete probabilities, where the PDF is a constant value representing the equal likelihood of each outcome. For continuous cases, he explains that the probability of a single point is not meaningful, but the probability of a range of outcomes can be calculated using the integral of the PDF over that range. The professor also discusses how the integral of the PDF over the entire domain must equal 1, which allows for the calculation of probabilities of subsets within the domain.
📉 Exponential and Gaussian PDFs in Practical Scenarios
The professor delves into specific types of PDFs, starting with the exponential PDF, using a customer service wait time example to demonstrate how to calculate the probability of waiting times. He shows how to determine the constant 'alpha' from given probabilities and then uses it to find the probability of longer wait times. The Gaussian or normal PDF is introduced as a crucial PDF that is widely applicable, with its bell curve shape representing outcomes centered around a mean. The professor emphasizes the ubiquity of the Gaussian distribution and its appearance in various mathematical contexts, such as binomial coefficients converging to a Gaussian shape.
🔮 The Significance of Gaussian Distributions
In the final paragraph, the professor highlights the prevalence and importance of Gaussian distributions in various fields. He explains that Gaussian distributions can result from the accumulation of many small, independent decisions, hinting at the central limit theorem. The professor also mentions that Gaussians appear in unexpected places, such as in the rows of Pascal's triangle, which resemble a shifted Gaussian when normalized. The lecture concludes with a preview of upcoming lessons that will further explore the role of probability theory in calculus.
Mindmap
Keywords
💡Probability Density
💡Fairness
💡Bias
💡Exponential Function
💡Integral
💡Uniform Density Function
💡Discrete Probability
💡Gaussian PDF
💡Binomial Coefficients
💡Probability of an Event
Highlights
Introduction to probability densities and their deviation from fair or uniform probabilities.
Definition and description of probability density functions (PDFs).
Criteria for a function to be considered a PDF: non-negativity and integral equal to 1.
Example of an exponential PDF and its verification as a valid PDF.
Explanation of uniform density functions and their relation to fairness.
Calculation of probabilities in discrete and continuous domains using PDFs.
Interpretation of the value of a PDF at a point versus the probability of a specific outcome.
General formula for computing the probability of landing in a subset using a PDF.
Application of the concept of PDFs to the context of customer service wait times.
Calculation of the probability of waiting times using an exponential PDF.
Introduction to the Gaussian or normal PDF and its characteristics.
Verification of the Gaussian PDF's integral over the real line being equal to 1.
Discussion on the ubiquity of Gaussian distributions in various fields.
Connection between binomial coefficients and Gaussian distributions in Pascal's triangle.
The concept that Gaussians are limits of individual decisions converging to a distribution.
Anticipation of further exploration of probability theory characters in upcoming lessons.
Transcripts
Browse More Related Video
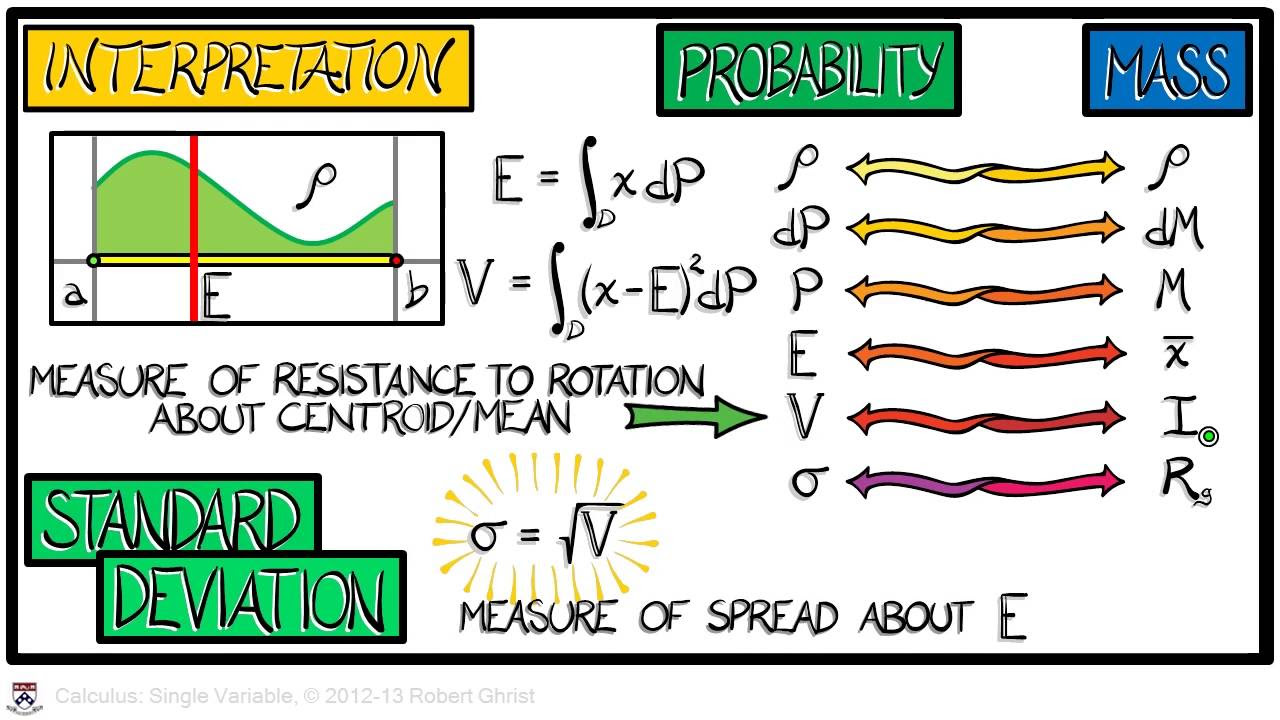
Calculus Chapter 4 Lecture 44 Expectation and Variance
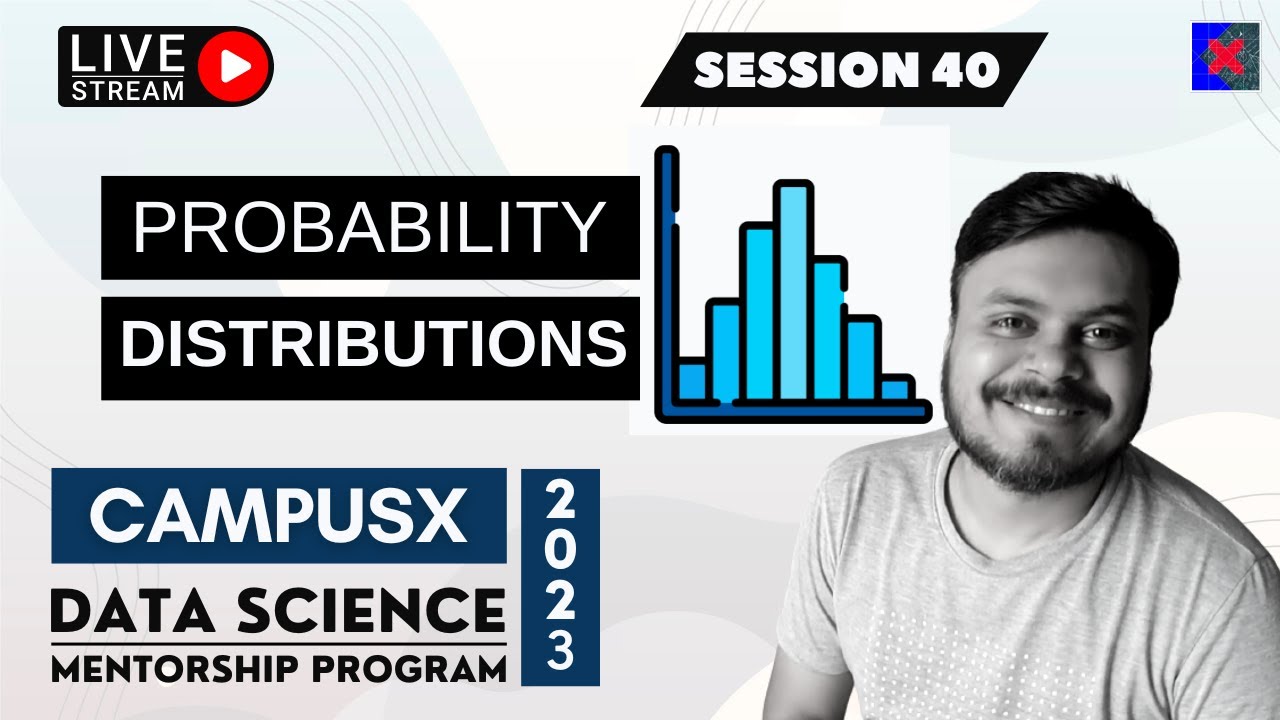
Session 40 - Probability Distribution Functions - PDF, PMF & CDF | DSMP 2023
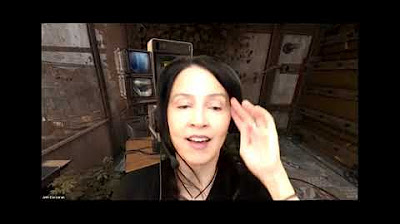
Mathematical Statistics, Lecture 1

Why “probability of 0” does not mean “impossible” | Probabilities of probabilities, part 2
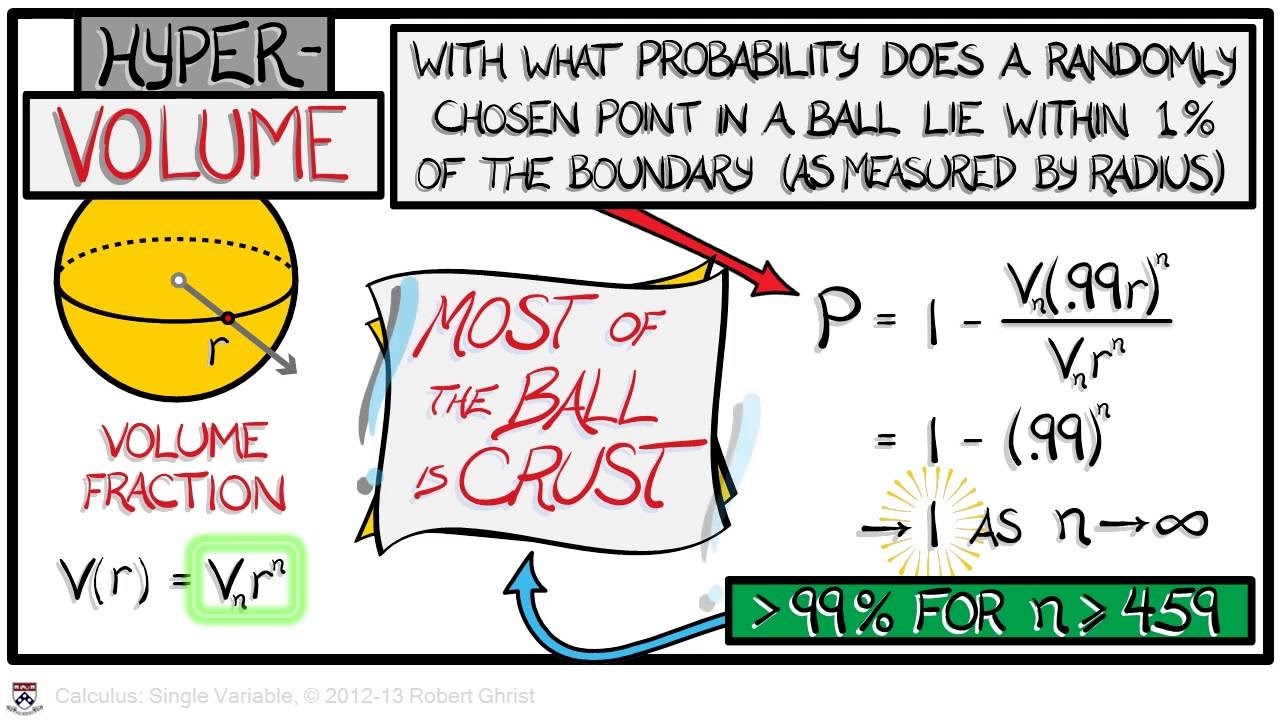
Calculus Chapter 4 Lecture 42 Fair Probability

Lec 36 | MIT 18.01 Single Variable Calculus, Fall 2007
5.0 / 5 (0 votes)
Thanks for rating: