Why “probability of 0” does not mean “impossible” | Probabilities of probabilities, part 2
TLDRThis video explores the concept of probability in the context of a weighted coin with an unknown bias, h. Through an example of flipping the coin ten times and observing seven heads, it delves into the paradox of assigning probabilities to continuous values. The key takeaway is that instead of focusing on individual probabilities, we should consider probability densities, which represent the likelihood of ranges of values. This approach resolves the paradox by using probability density functions (PDFs) and measure theory, which connect finite and continuous probability settings. The video sets the stage for understanding how to derive a PDF from observed data.
Takeaways
- 🎲 The script discusses a weighted coin with an unknown probability of landing heads, which could vary from 0% to 100%.
- 🤔 It raises the question of estimating the true probability of heads given observed outcomes, highlighting the complexity of probabilities of probabilities.
- 📊 The paradox of assigning a probability to continuous values is addressed, where individual probabilities for specific values approach zero but must sum to one.
- 🛠 The concept of probability density is introduced as a solution to the paradox, where the area under a curve represents the probability of a range of outcomes.
- 📚 The importance of measure theory is mentioned as a mathematical framework that unifies discrete and continuous probability distributions.
- 📉 The script explains that in continuous distributions, the probability of a specific value is zero, but the probability across a range is non-zero.
- 📈 The use of probability density functions (PDFs) is emphasized for understanding the likelihood of a random variable falling within a certain interval.
- 🔍 The process of refining the understanding of a distribution involves considering finer buckets and approaching a smooth curve as a limit.
- 📐 The total area under the PDF must equal one, reflecting the total probability of all possible outcomes.
- 🧩 The script suggests that in practice, using data to estimate the PDF can help answer complex probability questions about the underlying process.
- 🔑 The takeaway is that understanding the rules of probability in continuous settings is crucial for making meaningful inferences from data.
Q & A
What is the main point of the initial discussion about the weighted coin?
-The main point is to introduce the concept of a weighted coin where the probability of flipping heads is unknown and can vary, highlighting the challenge of determining this probability based on a limited number of flips.
Why is it problematic to ask about the probability of the true probability being exactly 0.7?
-It's problematic because it involves assigning a probability to a specific value within a continuous range, which can lead to paradoxes if not handled correctly.
How does the script propose to resolve the paradox of assigning probabilities to continuous values?
-The script resolves this paradox by focusing on ranges of values rather than individual values and using probability density instead of discrete probabilities.
What is the significance of using the area under the curve to represent probability?
-Using the area under the curve allows for meaningful representation of probabilities in a continuous setting, ensuring that the total probability sums to one and avoids paradoxes associated with individual probabilities.
What is a probability density function (PDF), and how is it used?
-A PDF is a function that describes the probability density of a continuous random variable. The probability of the variable falling within a certain range is given by the area under the curve of the PDF within that range.
How does measure theory help in understanding probabilities in continuous settings?
-Measure theory provides a rigorous mathematical framework for associating probabilities with subsets of possible outcomes, uniting finite and continuous settings and resolving paradoxes associated with continuous probabilities.
Why is it necessary to consider ranges of values rather than individual values when dealing with continuous probabilities?
-Considering ranges of values avoids the problem of assigning non-zero probabilities to an uncountably infinite number of individual values, which would lead to an infinite total probability.
What happens to the probabilities of individual values in the continuous setting, according to the script?
-In the continuous setting, the probability of any single specific value is zero, but the probabilities of ranges of values remain meaningful.
How do integrals relate to probabilities in continuous settings?
-In continuous settings, integrals are used to calculate the area under the curve of a probability density function, representing the probability of a random variable falling within a specific range.
What practical question does the script aim to address regarding the weighted coin?
-The script aims to address the practical question of how to use data to create meaningful answers to probabilities of probabilities questions, such as finding the probability density function for the true probability of flipping heads.
Outlines
🎲 Understanding Probabilities with a Biased Coin
This paragraph introduces a thought experiment involving a weighted coin with an unknown probability of landing heads. It discusses the difficulty of assigning a probability to the true probability of heads (denoted as 'h') and the paradox that arises when considering the infinitesimally small probabilities of each possible value of 'h'. The solution proposed is to consider ranges of values for 'h' rather than individual values, emphasizing the importance of using areas to represent probabilities in continuous distributions, which helps to resolve the paradox.
📊 The Concept of Probability Density Function (PDF)
The second paragraph delves into the concept of a Probability Density Function (PDF), explaining how it allows for the assignment of a meaningful probability to ranges of continuous values, thus avoiding the paradoxes associated with discrete probabilities. It clarifies that the probability of a specific value is zero in a continuous distribution, but the area under the PDF curve between two values represents the probability of the random variable falling within that range. The paragraph also touches on the shift in rules for combining probabilities in continuous versus discrete settings and briefly introduces measure theory as a mathematical framework that unifies these concepts.
Mindmap
Keywords
💡Weighted Coin
💡Probability
💡Continuous Values
💡Paradox
💡Probability Density
💡Probability Density Function (PDF)
💡Measure Theory
💡Integral
💡Random Variable
💡Dartboard
Highlights
Introduction of a hypothetical weighted coin with an unknown probability of landing heads.
Discussion of the paradoxical nature of assigning probabilities to continuous values.
The concept of a probability density function (PDF) as a solution to the paradox of continuous probabilities.
Explanation of how the area under a PDF curve represents the probability of a range of outcomes.
The importance of considering ranges of values rather than individual values in continuous probability.
The idea that as the width of probability buckets decreases, the height remains constant, leading to a smooth curve.
Clarification that the height of the PDF represents the probability density, not the probability itself.
The necessity for the total area under the PDF to equal one, reflecting a valid probability distribution.
The distinction between discrete and continuous probability settings and how they are handled differently.
Introduction to measure theory as a mathematical framework that unifies discrete and continuous probability.
The practical application of using data to estimate the probability density function of an unknown parameter.
The transition from discrete sums to continuous integrals as a common rule of thumb in probability.
The deeper theoretical underpinnings of integrals in relation to measure theory and probability.
The intuitive challenge of understanding how individual outcomes with zero probability can sum to one in continuous settings.
The realization that the rules for combining probabilities change between finite and continuous settings.
The practical question of estimating the true probability of flipping heads given observed outcomes.
The teaser for the next part of the video where the PDF will be derived from observed data.
Transcripts
Browse More Related Video
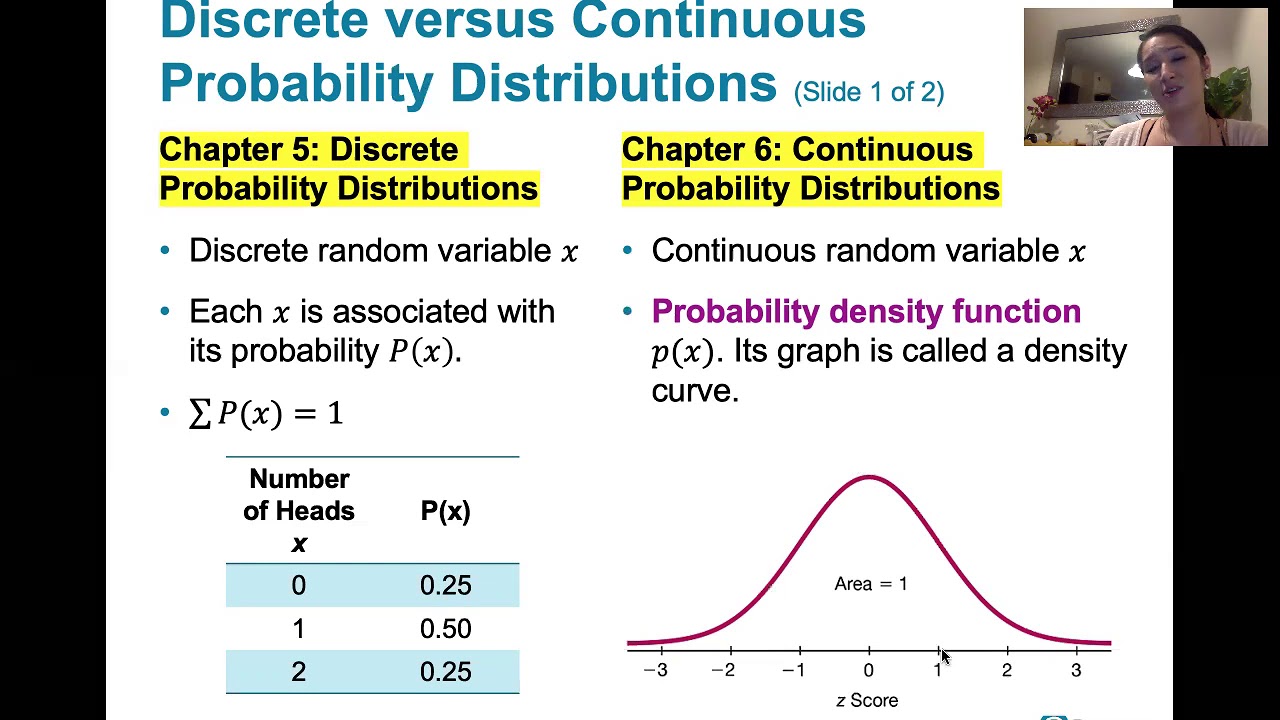
6.1.1 The Standard Normal Distribution - Discrete and Continuous Probability Distributions
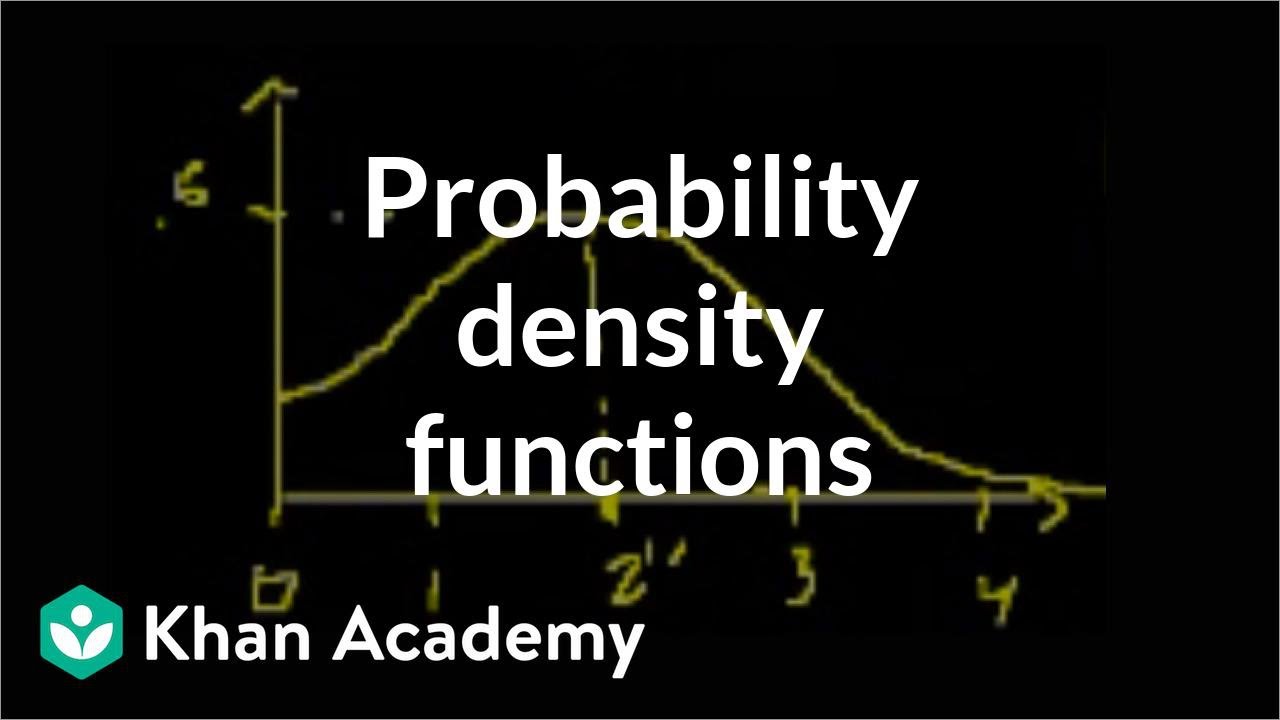
Probability density functions | Probability and Statistics | Khan Academy
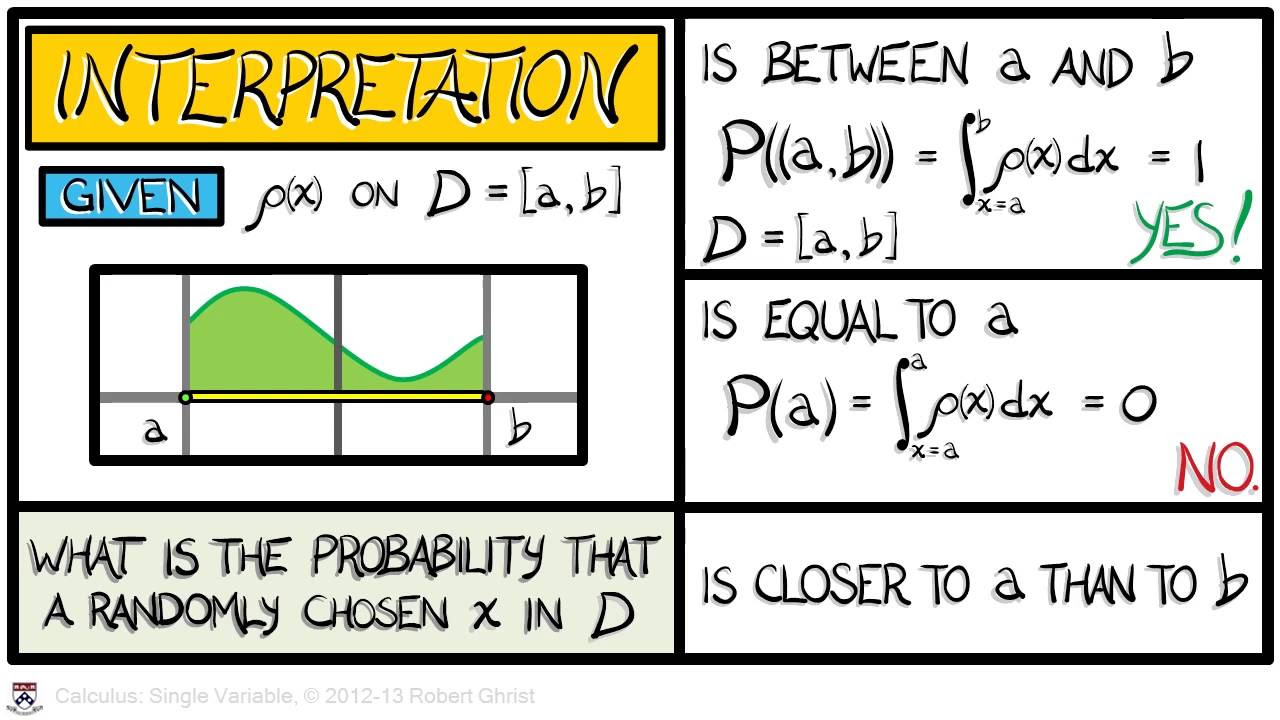
Calculus Chapter 4 Lecture 43 Probability Densities
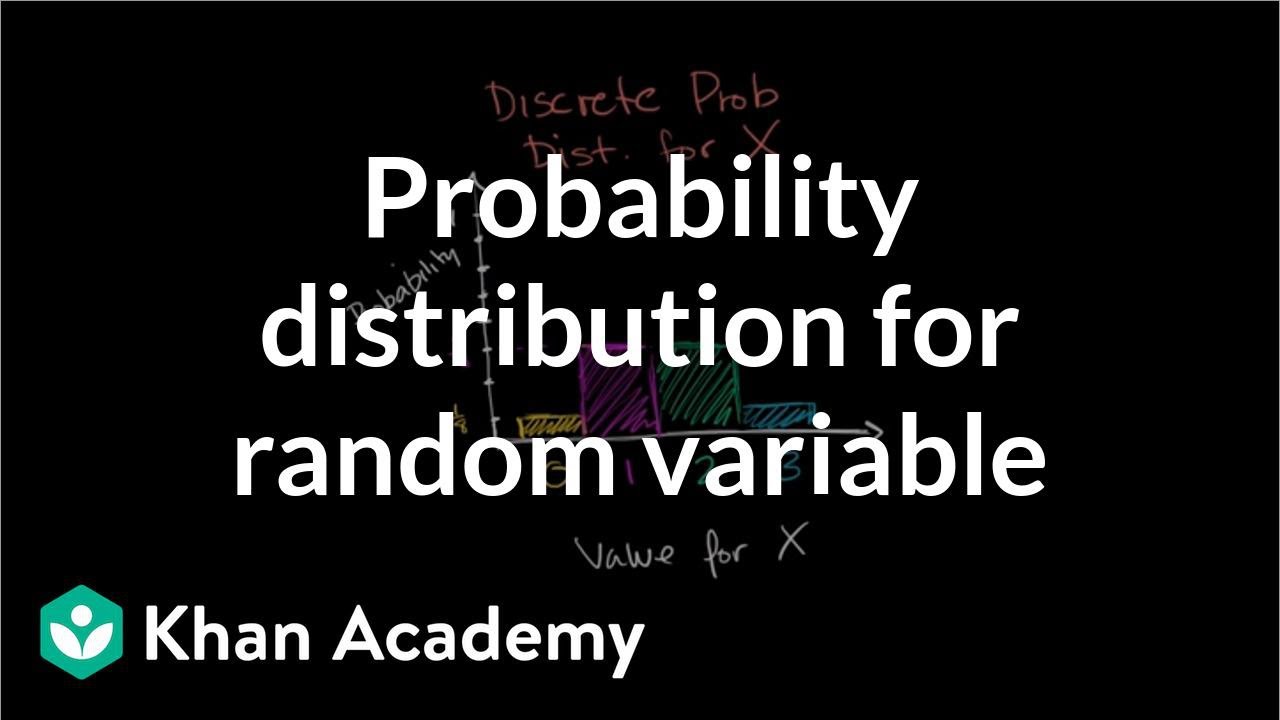
Constructing a probability distribution for random variable | Khan Academy
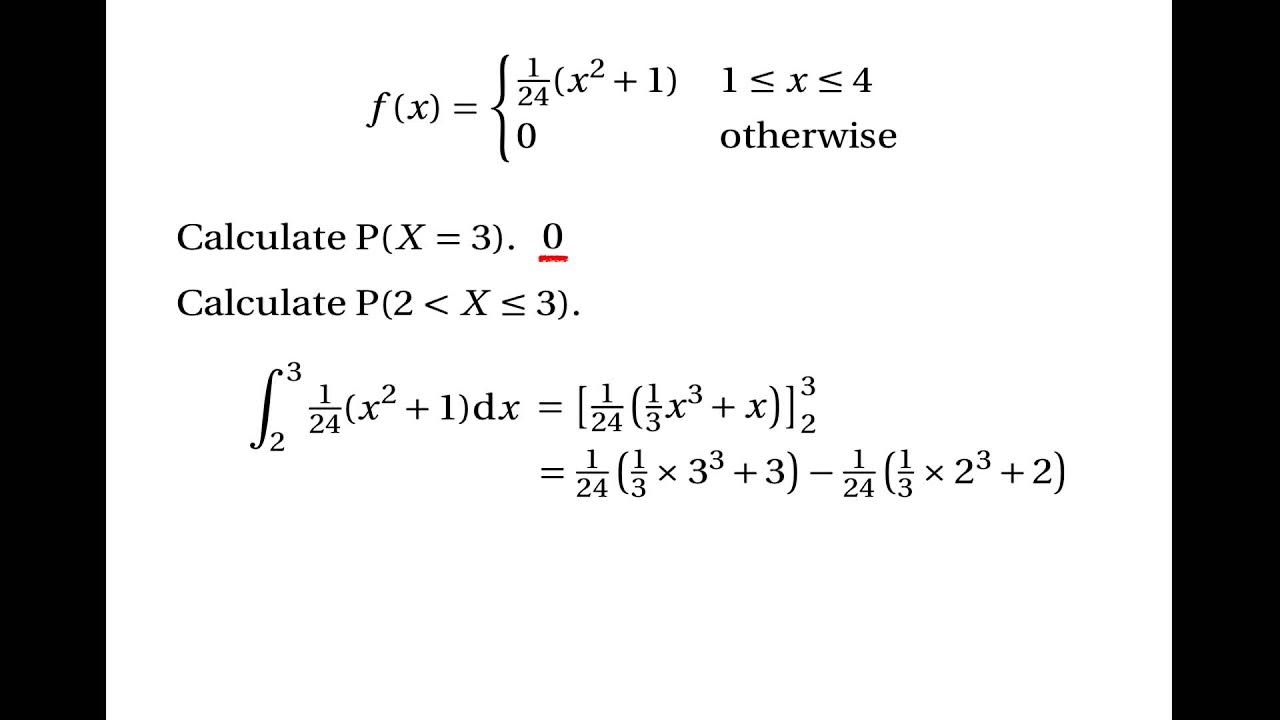
Continuous Random Variables: Probability Density Functions
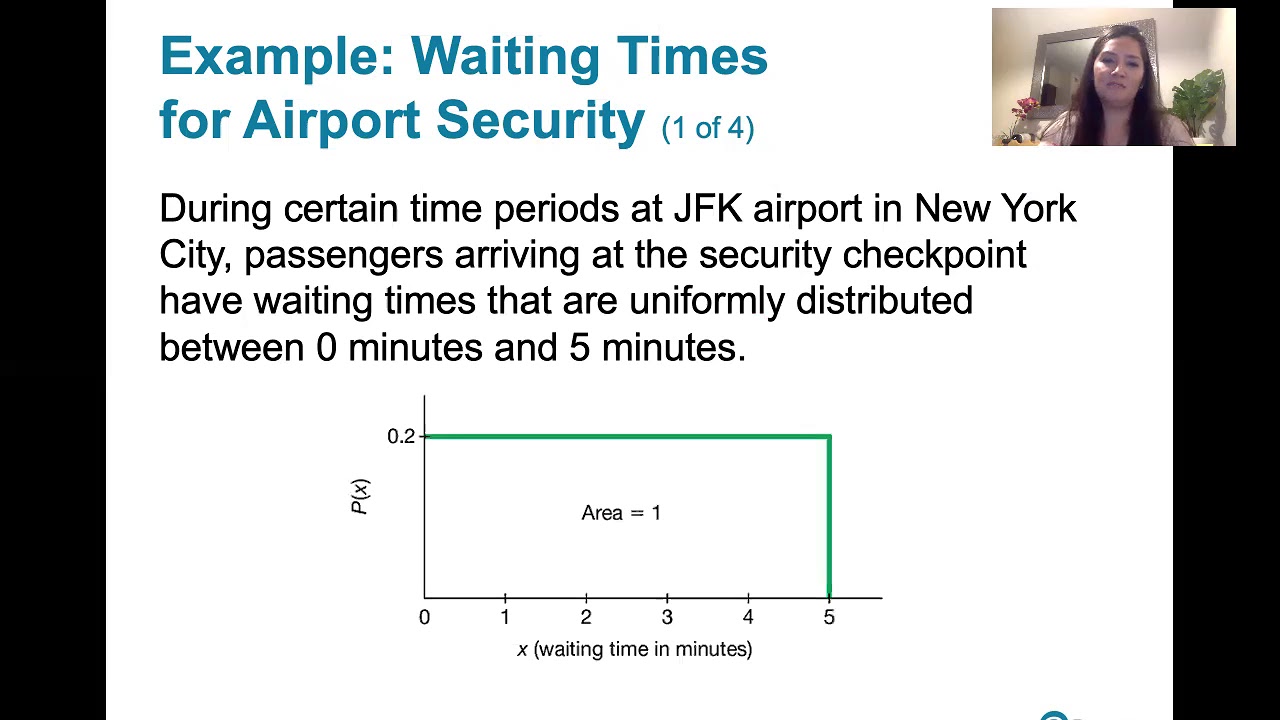
6.1.2 The Standard Normal Distribution - Uniform Distributions
5.0 / 5 (0 votes)
Thanks for rating: