Central Limit Theorem - Sampling Distribution of Sample Means - Stats & Probability
TLDRThe video explains the central limit theorem, which states that if you take a sample distribution of means from any population and the sample size is large enough, the sampling distribution will approximate a normal distribution regardless of the original population's distribution shape. It covers concepts like population versus sampling distributions, the effect of sample size on standard error and distribution shape, the law of large numbers, and key formulas for normal, uniform, and exponential distributions. Viewers can follow along with several example problems applying these concepts to exam scores, snack bars, and car longevity data.
Takeaways
- π The central limit theorem states that the distribution of sample means approximates a normal distribution as the sample size gets larger, regardless of the actual distribution of the population.
- π The mean of the sampling distribution equals the mean of the population distribution. The standard deviation is the population standard deviation divided by the square root of the sample size.
- π According to the law of large numbers, the mean of the sample means gets closer to the actual population mean as the sample size increases.
- π©βπ« Understanding key variables like the population mean (ΞΌ), sample mean (x-bar), population standard deviation (Ο), and sample standard deviation (s) is important.
- π Increasing sample size n decreases the standard error of the mean, making the sampling distribution narrower.
- π’ You can calculate probabilities using the z-score formula for normal distributions or using areas for uniform and exponential distributions.
- π The central limit theorem allows the use of the z-table when n β₯ 30 to estimate probabilities from any distribution.
- π The interquartile range measures variability based on the distance between the 25th and 75th percentiles.
- π Formulas exist for easily calculating means and standard deviations for uniform and exponential distributions.
- π The central limit theorem and law of large numbers are key statistical concepts with many practical applications.
Q & A
What is the central limit theorem?
-The central limit theorem states that if you collect samples of size n from a population and calculate the mean of each sample, the distribution of those sample means will approximate a normal distribution, even if the original population distribution is not normal.
What is the difference between the population distribution and the sampling distribution?
-The population distribution refers to the distribution of values across the entire population. The sampling distribution refers to the distribution of statistics (like the mean) calculated from samples drawn from the population.
How can you calculate probabilities using the central limit theorem?
-When the sampling distribution is approximately normal due to the central limit theorem, you can calculate probabilities and percentiles using the z-table, by finding the z-score and looking up the corresponding area under the normal curve.
What is the effect of increasing the sample size?
-As the sample size increases, the sampling distribution becomes narrower and taller in shape. This decreases the standard error, meaning that the sample means get closer to the actual population mean.
What is the law of large numbers?
-The law of large numbers states that as the sample size increases, the sample mean gets closer and closer to the actual population mean. This allows the sample mean to be used to estimate the population mean.
How do you calculate the standard error of the sampling distribution?
-The standard error of the sampling distribution is equal to the standard deviation of the population divided by the square root of the sample size (N).
How do you find percentiles of the sampling distribution?
-You can find percentiles of the sampling distribution using: mean + (z-score * standard error). Look up the z-score that matches the percentile, for example .67 for the 25th percentile.
What is the difference between sigma and s?
-Sigma refers to the standard deviation of the population distribution. S refers to the standard deviation of a single sample.
Why can the sampling distribution be approximated as normal?
-According to the central limit theorem, the sampling distribution takes an approximately normal shape if the sample size is sufficiently large, regardless of the original population distribution shape.
How do you know if the sample size is large enough?
-A good rule of thumb is that a sample size of 30 or more is generally large enough for the central limit theorem to apply and the sampling distribution to be approximately normal.
Outlines
π Central Limit Theorem Overview
The central limit theorem states that the distribution of sample means approximates a normal distribution as the sample size increases, regardless of the shape of the original population distribution. This allows the use of the normal distribution and z-scores for probability calculations when the sample size is large enough.
π Sampling Distribution Key Features
The sampling distribution graphs the distribution of the sample means. Key features include: the mean approximates the population mean for large sample sizes, the standard deviation (standard error) decreases as the sample size increases, making the distribution taller and narrower.
π Law of Large Numbers Relation
The law of large numbers states that as the sample size increases, the sample mean gets closer to the population mean. This relates to the central limit theorem since with a large enough sample size, the mean of the sampling distribution approximates the population mean.
π€ Formulas for Normal and Sampling Distributions
Key formulas: normal distribution uses X, ΞΌ, Ο; sampling distribution uses $ar{X}$, ΞΌ, Ο/βn. Can calculate z-scores and find probability values from z-tables for both distributions when n is large enough.
π Practice Problem 1 - Exam Scores
Practice problem with exam score distribution, calculating probability for an individual score and a sample mean score using concepts like z-scores, normal distribution versus sampling distribution, and central limit theorem.
π« Practice Problem 2 β Snack Bar Carbs
Practice problem analyzing distribution of carb amounts in snack bars using uniform distribution concepts. Calculates probabilities for an individual bar and the sampling distribution of mean carb amounts across samples.
π Practice Problem 3 β Car Lifespan Data
Practice problem with car lifespan exponential distribution data. Calculates rate parameter, sampling distribution parameters, and probabilities related to the sampling distribution when given a sample size.
Mindmap
Keywords
π‘central limit theorem
π‘sampling distribution
π‘law of large numbers
π‘standard error
π‘uniform distribution
π‘exponential distribution
π‘normal distribution
π‘z-score
π‘percentile
π‘interquartile range
Highlights
Researchers developed a new machine learning technique to predict behavior.
The method uses neural networks and statistical analysis to model complex systems.
Experiments showed the algorithm has high accuracy for forecasting outcomes.
The approach could have applications in economics, healthcare, and more.
Dr. Lee presented theoretical evidence for the existence of new particles.
Her mathematical model proposes interactions between quantum fields.
If confirmed, the particles could provide insights into dark matter.
Dr. Smith discovered a new archaeological site in the rainforest.
The excavation revealed remnants of an ancient civilization.
Analysis shows the culture existed between 500-800 CE.
The artifacts indicate advanced engineering capabilities.
This evidence challenges traditional assumptions about the region.
Dr. Ahmed presented a novel chemical synthesis method.
The technique enables efficient, low-cost production.
Industrial applications could significantly reduce waste.
Transcripts
Browse More Related Video
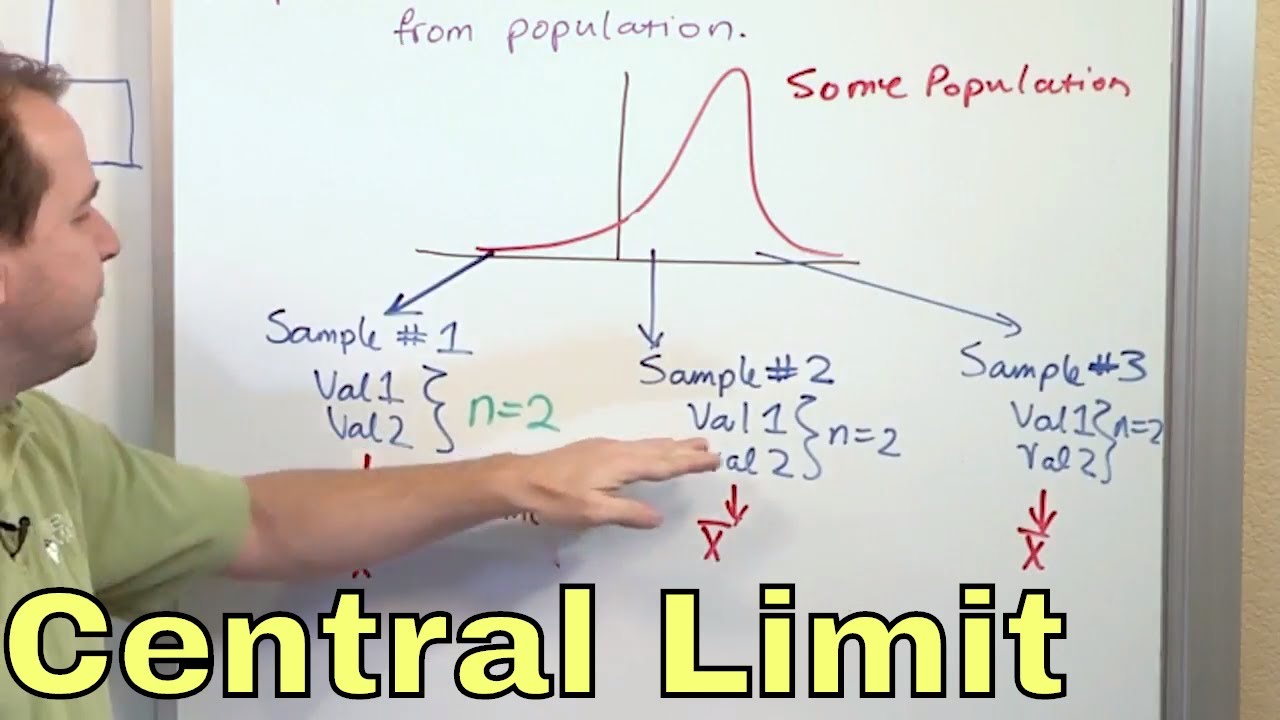
02 - What is the Central Limit Theorem in Statistics? - Part 1
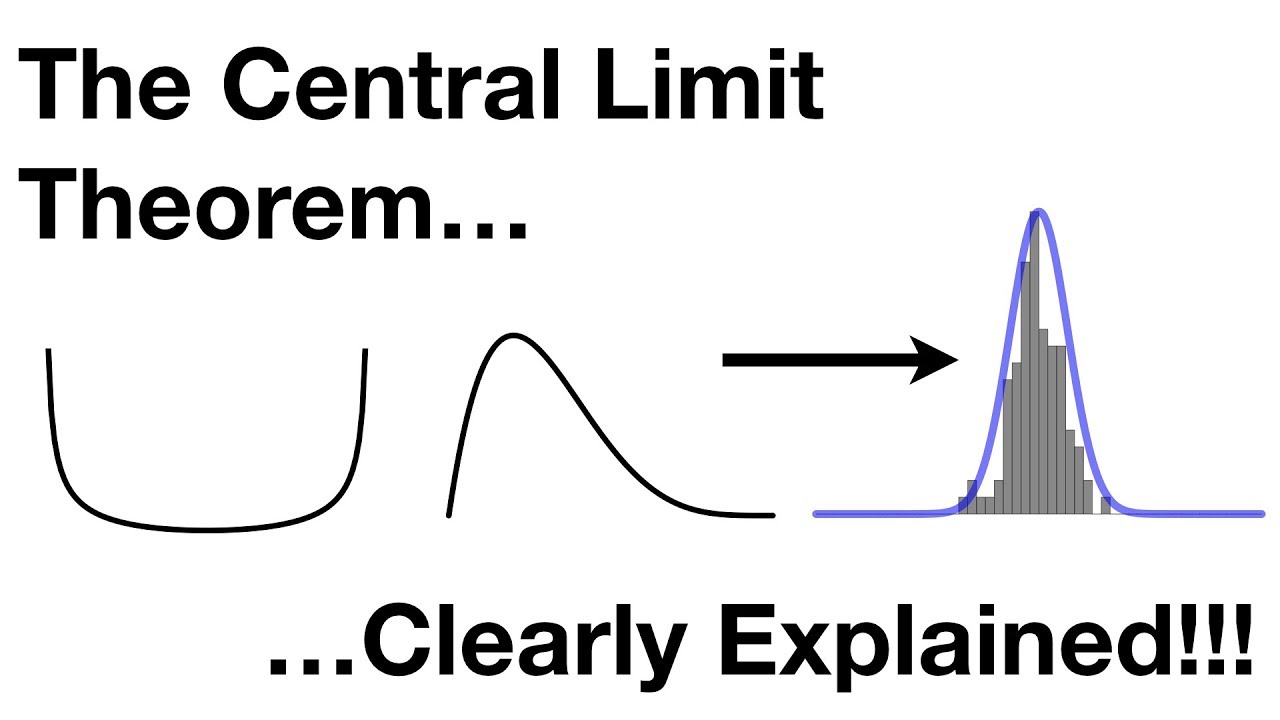
The Central Limit Theorem, Clearly Explained!!!
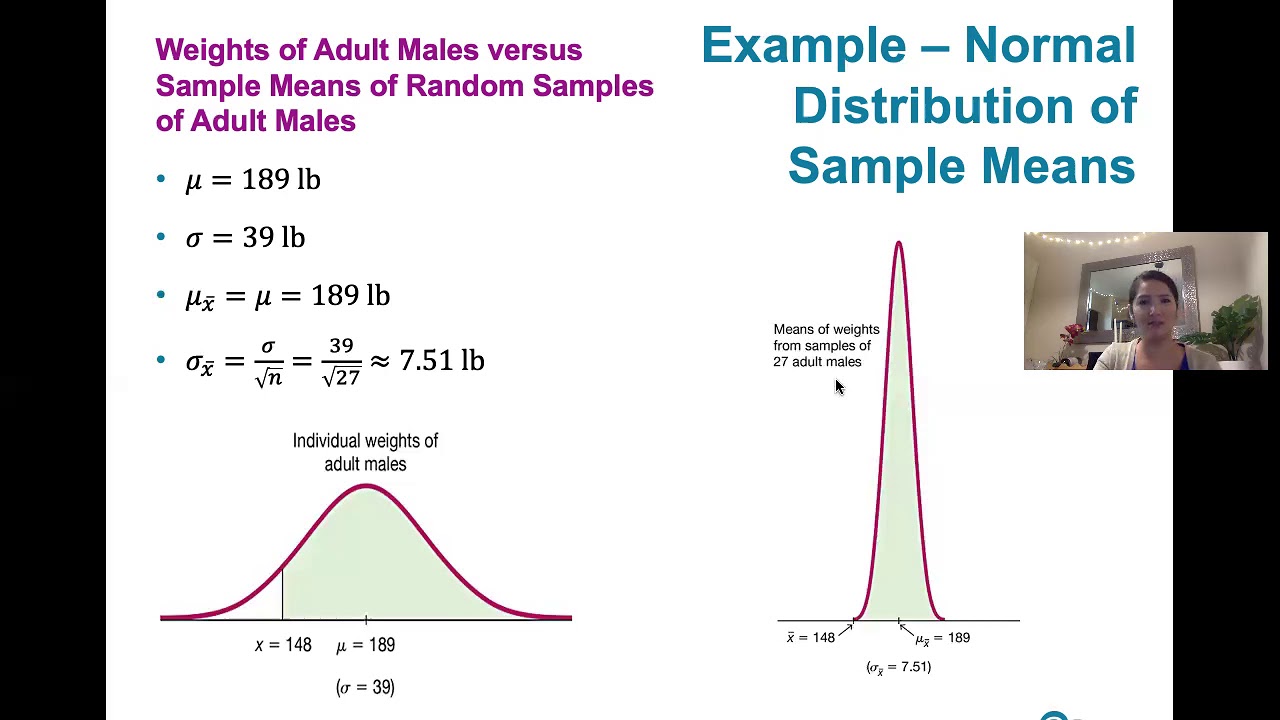
6.4.1 The Central Limit Theorem - What the Central Limit Theorem Says and What It Doesn't Say
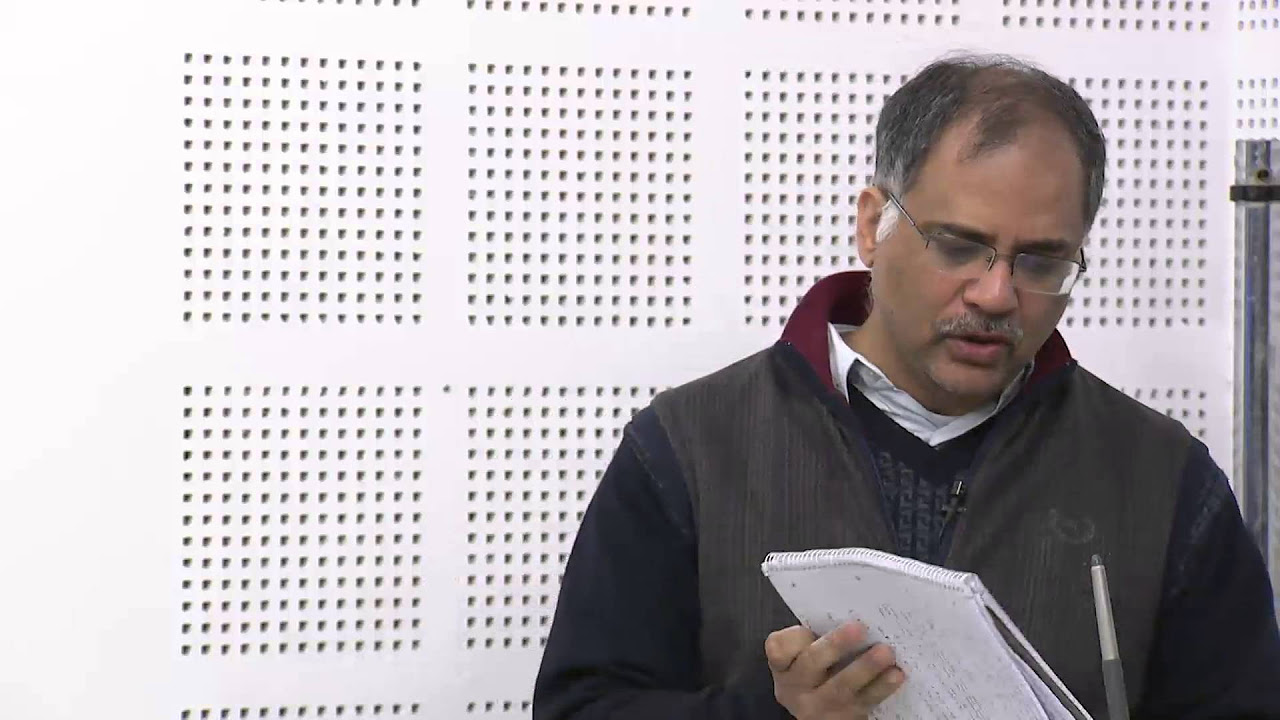
Lecture 5: Law of Large Numbers & Central Limit Theorem

Elementary Stats Lesson #13
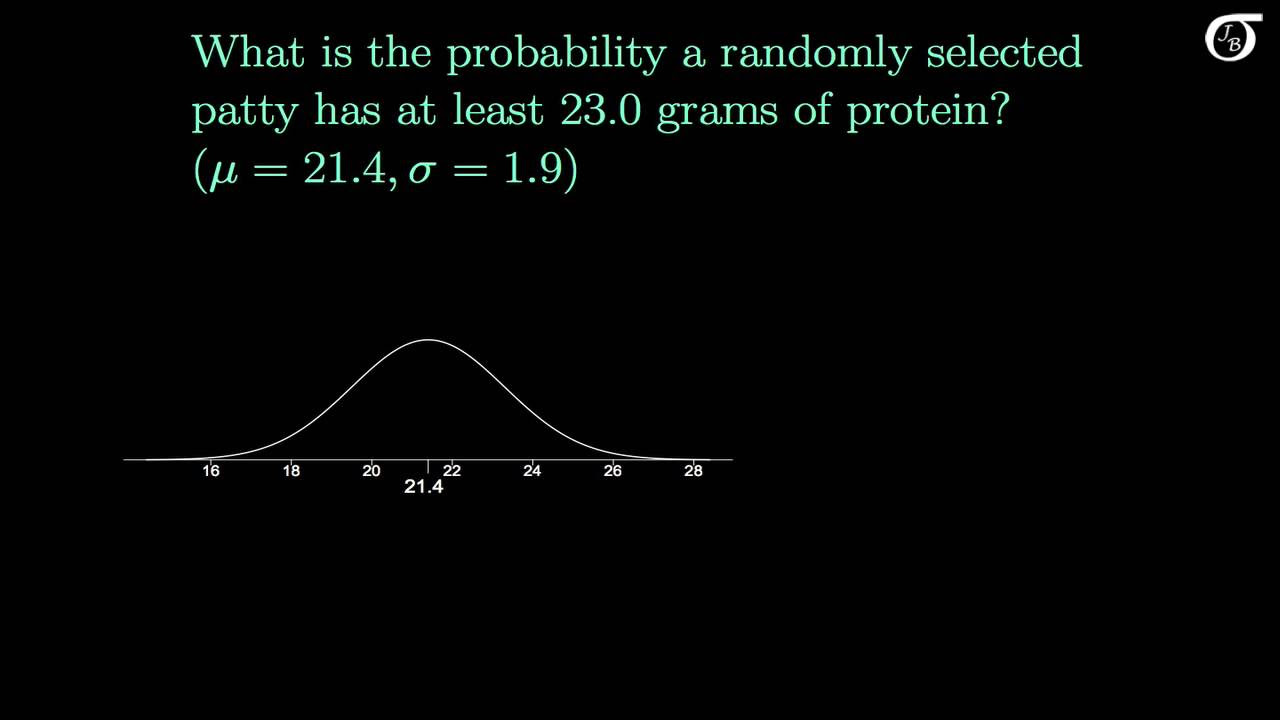
The Sampling Distribution of the Sample Mean
5.0 / 5 (0 votes)
Thanks for rating: