02 - What is the Central Limit Theorem in Statistics? - Part 1
TLDRThe provided transcript is a detailed explanation of the Central Limit Theorem (CLT) from a statistics lesson. The speaker emphasizes that while students often find the concept challenging, it's not inherently difficult but rather requires a clear understanding and visualization of the process. The CLT is introduced as a powerful tool for estimating population characteristics by sampling, regardless of the population's distribution shape. The lesson outlines that the mean of all sample means will equal the population mean and that the standard deviation of these sample means can be related to the population standard deviation. The speaker also highlights that if the population is normal, the distribution of sample means will also be normal, and even if the population is not normal, the sampling distribution of sample means will approximate a normal distribution if the sample size is greater than 30. This theorem is fundamental for statistical inference and the application of normal distribution methods to various populations, which is further explored through practical problem-solving in subsequent sections of the lesson.
Takeaways
- ๐ The Central Limit Theorem (CLT) is a fundamental statistical concept that helps in understanding the distribution of sample means regardless of the population's distribution shape.
- ๐ค The CLT can be challenging for students because it requires a good mental visualization of the process and its implications, not just memorization of formulas.
- ๐ The mean of all sample means taken from a population is equal to the population mean, which is a powerful result of the CLT and holds true regardless of the sample size or the population's distribution.
- ๐ The standard deviation of the sample means can be calculated and is related to the population standard deviation, with the relationship being dependent on the sample size (n).
- ๐งฎ If the population is normally distributed, the distribution of sample means will also be normal, regardless of the sample size.
- ๐ For non-normal populations, if the sample size (n) is greater than 30, the sampling distribution of sample means approximates a normal distribution, which is a significant practical application of the CLT.
- ๐ The CLT is useful because it allows statisticians to make inferences about a population based on sample data, even when the population distribution is unknown or not normal.
- ๐ The theorem is applicable to any population distribution shape, which makes it a versatile tool in statistical analysis.
- ๐ข The concept of sample size is critical in the CLT; a larger sample size increases the likelihood of the sampling distribution of sample means approximating a normal distribution.
- ๐ In practice, while it's not feasible to sample the entire population, taking a sufficient number of samples can provide a close estimate of the population mean.
- โ The CLT is not just a theoretical concept; it's a practical tool that can be used to solve a wide range of statistical problems involving sample data.
Q & A
What is the central limit theorem?
-The central limit theorem (CLT) is a statistical theory that states that given a population with a mean ฮผ and standard deviation ฯ, the sampling distribution of the sample means will be approximately normally distributed if the sample size is large enough, regardless of the shape of the population distribution.
Why is the central limit theorem important?
-The central limit theorem is important because it allows statisticians to make inferences about a population based on sample data. It is particularly useful because it doesn't require the population distribution to be normal, and it provides a basis for constructing confidence intervals and conducting hypothesis tests.
What are the two key properties that the central limit theorem is based on?
-The two key properties that the central limit theorem is based on are the mean (ฮผ) and the standard deviation (ฯ) of the population.
What does the central limit theorem state about the mean of the sample means?
-The central limit theorem states that the mean of the sample means is equal to the mean of the population (ฮผ), regardless of the sample size or the shape of the population distribution.
How is the standard deviation of the sample means related to the population standard deviation?
-The standard deviation of the sample means is equal to the population standard deviation (ฯ) divided by the square root of the sample size (n).
What happens if the population distribution is normal?
-If the population distribution is normal, then the distribution of the sample means will also be normal, regardless of the sample size.
What is the significance of a sample size greater than 30 in the context of the central limit theorem?
-If the sample size (n) is greater than 30, even if the population distribution is not normal, the sampling distribution of the sample means will approximate a normal distribution.
Why is it not practical to sample the entire population in real life?
-It is not practical to sample the entire population in real life because it would require collecting data from every individual in the population, which is often impossible or impractical due to time, cost, and logistical constraints.
What is the role of visualization in understanding the central limit theorem?
-Visualization is crucial in understanding the central limit theorem as it helps to create a mental picture of how sample means are derived from a population and how they distribute. This aids in comprehending how the theorem allows for the approximation of a normal distribution under certain conditions.
How does the central limit theorem apply to skewed distributions?
-The central limit theorem applies to skewed distributions by stating that if the sample size is large enough (greater than 30), the sampling distribution of the sample means will approximate a normal distribution, even if the original population distribution is skewed.
What is the 'magic number' often referenced in discussions about the central limit theorem?
-The 'magic number' often referenced is 30, which is the sample size at which the sampling distribution of sample means begins to approximate a normal distribution, regardless of the shape of the original population distribution.
Why is the central limit theorem useful for solving statistical problems?
-The central limit theorem is useful for solving statistical problems because it allows us to assume that the sampling distribution of sample means is normal. This is beneficial because many statistical tests and confidence interval calculations rely on the normal distribution for their procedures.
Outlines
๐ Introduction to the Central Limit Theorem
The first paragraph introduces the central limit theorem (CLT), emphasizing that while it can be challenging for students, the difficulty arises from the need to visualize the process rather than the complexity of the concept itself. The speaker sets expectations that understanding the CLT requires getting through the explanation and working through a few problems. The CLT is described as highly useful, especially when applied to problem-solving. The given population's mean (ฮผ) and standard deviation (ฯ) are foundational to the discussion, and the concept of sampling from this population is introduced.
๐ The Power and Application of the Central Limit Theorem
The second paragraph delves into the versatility of the CLT, highlighting its application regardless of the population's distribution shape. The process of sampling from a population of any distribution, calculating sample means, and the resulting distribution of these means is explained. The paragraph also touches on the theoretical aspect of sampling where every possible sample of a given size (n) is taken from the population. The practicality of the CLT is emphasized, noting its utility even when not every individual in the population is sampled.
๐ฏ Central Limit Theorem's Key Conclusions
The third paragraph presents the core conclusions of the CLT. It states that the mean of all sample means will equal the population mean, irrespective of the sample size or the population's distribution. This is a powerful concept as it implies that by averaging enough sample means, one can approximate the population mean. The standard deviation of the sample means is also discussed, showing how it relates to the population standard deviation by a factor of the sample size's square root.
๐งฎ Estimating Population Standard Deviation Through Sampling
The fourth paragraph focuses on how to estimate the population's standard deviation using sample means and their standard deviation. It explains that by collecting multiple sample means and calculating their standard deviation, one can infer the population's standard deviation. The caveat of needing to sample the entire population to achieve this is acknowledged, but the paragraph clarifies that even without exhaustive sampling, a close approximation can be obtained through a large number of samples.
๐ Normal Distribution of Sample Means
The fifth paragraph discusses the implications of the CLT when the population is normally distributed. It explains that if the population is normal, the distribution of sample means will also be normal, regardless of the sample size. The importance of this is underscored by the familiarity and ease with which statisticians can work with normal distributions. The concept is further extended to the scenario where the population is not normal but still results in a normal distribution of sample means if the sample size is greater than 30.
๐ง The Impact of Non-Normal Populations on the Central Limit Theorem
The sixth paragraph addresses the scenario where the population distribution is not normal. It clarifies that even with non-normal populations, if the sample size is greater than 30, the sampling distribution of sample means will approximate a normal distribution. This is a significant revelation as it implies that the shape of the original population distribution is not a limiting factor when applying the CLT with sufficiently large samples. The practical upshot is that one can still use normal distribution tables and methods to solve problems, which is particularly useful for statisticians.
๐ Visualizing the Central Limit Theorem with MIT Students' IQs
The seventh and final paragraph provides a hypothetical example using the IQ distribution of MIT students to illustrate the CLT. It visualizes how even if the IQ distribution of MIT students is skewed due to selection bias, taking numerous samples of 30 students each and calculating their means will result in a normal distribution centered around the average IQ of MIT students. This example solidifies the concept that the CLT allows for the application of normal distribution methods to a wide array of distributions when the sample size is sufficiently large.
Mindmap
Keywords
๐กCentral Limit Theorem
๐กSample Size (n)
๐กPopulation Mean (ฮผ)
๐กStandard Deviation (ฯ)
๐กSampling Distribution
๐กNormal Distribution
๐กSample Mean (xฬ)
๐กConfidence Intervals
๐กSkewed Distribution
๐กStatistical Inference
๐กHypothesis Testing
Highlights
The central limit theorem (CLT) is introduced as a fundamental concept in statistics that can be challenging for students to visualize but is not inherently difficult to understand.
The CLT is essential for studying a variety of statistical problems and becomes increasingly useful as problems are worked through.
Two key properties of a population, the mean (ฮผ) and standard deviation (ฯ), are prerequisites for applying the CLT.
Sampling involves selecting a sample of size n from a population and calculating the sample mean (xฬ), which is distinct from the population mean.
The CLT assumes that all possible samples of size n are taken from the population until the entire population is exhausted.
The theorem states that the mean of all sample means (xฬ) is equal to the population mean (ฮผ), regardless of the sample size or the population's distribution shape.
The standard deviation of the sample means can be calculated and is related to the population standard deviation through the formula ฯ_xฬ = ฯ/โn.
If the population is normal, the distribution of sample means will also be normal, regardless of the sample size.
For non-normal populations, if the sample size (n) is greater than 30, the sampling distribution of sample means approximates a normal distribution.
The CLT is powerful because it allows for the estimation of population parameters without knowing the population's distribution shape.
The theorem's utility is demonstrated through practical examples, such as estimating the mean IQ of MIT students despite the population's non-normal distribution.
The CLT enables the use of normal distribution tables and z-scores for a wide range of statistical calculations, even when the population distribution is unknown or non-normal.
The importance of the CLT is reinforced by emphasizing its application in solving real statistical problems through the use of sample means.
The concept of a sampling distribution is central to the CLT, representing the distribution that results from all possible sample means.
The mean of the sampling distribution of sample means is always equal to the population mean, a key takeaway from the CLT.
The CLT provides a foundation for solving problems involving confidence intervals and hypothesis testing by approximating distributions.
The lecture concludes with a promise to engage in problem-solving activities that will solidify the understanding and application of the CLT.
Transcripts
Browse More Related Video
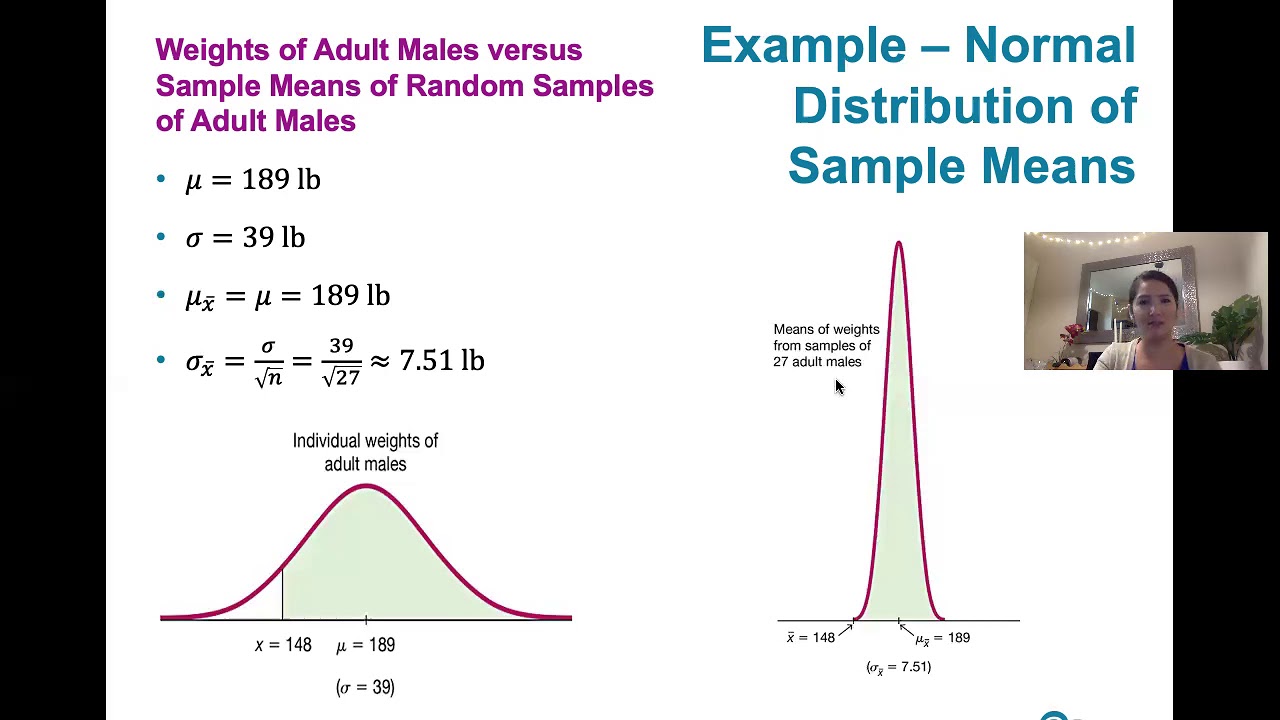
6.4.1 The Central Limit Theorem - What the Central Limit Theorem Says and What It Doesn't Say
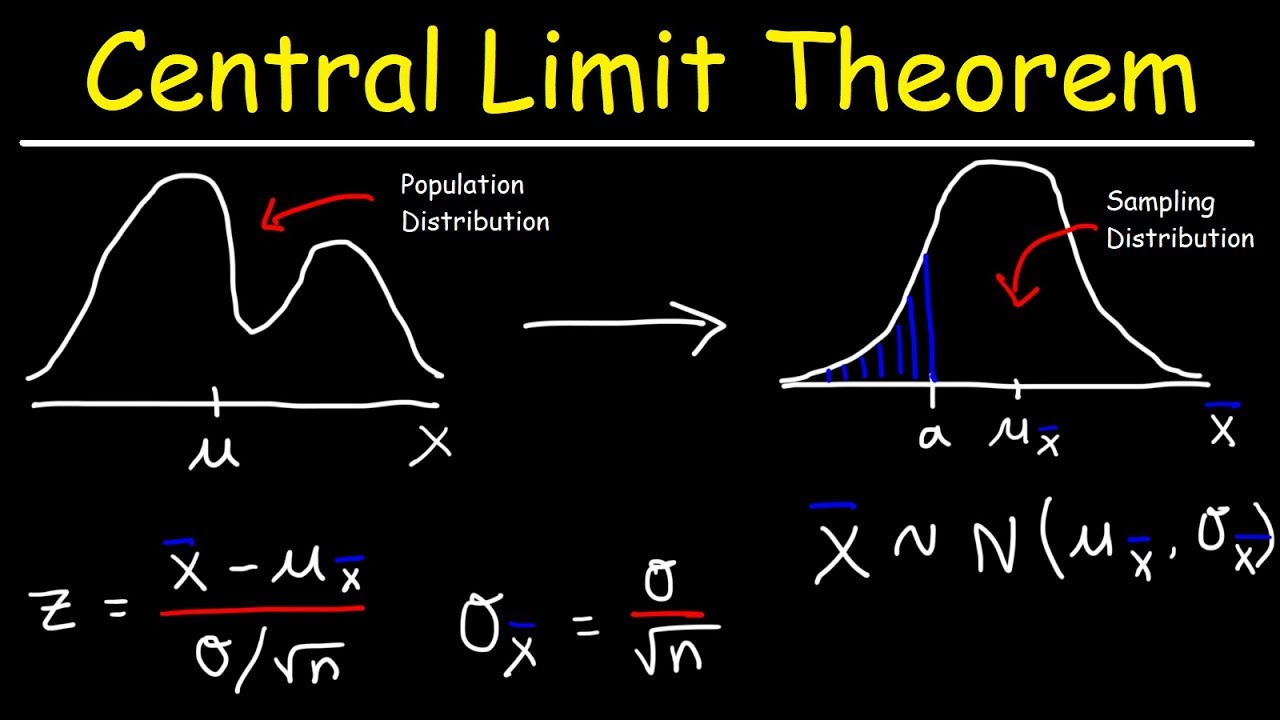
Central Limit Theorem - Sampling Distribution of Sample Means - Stats & Probability
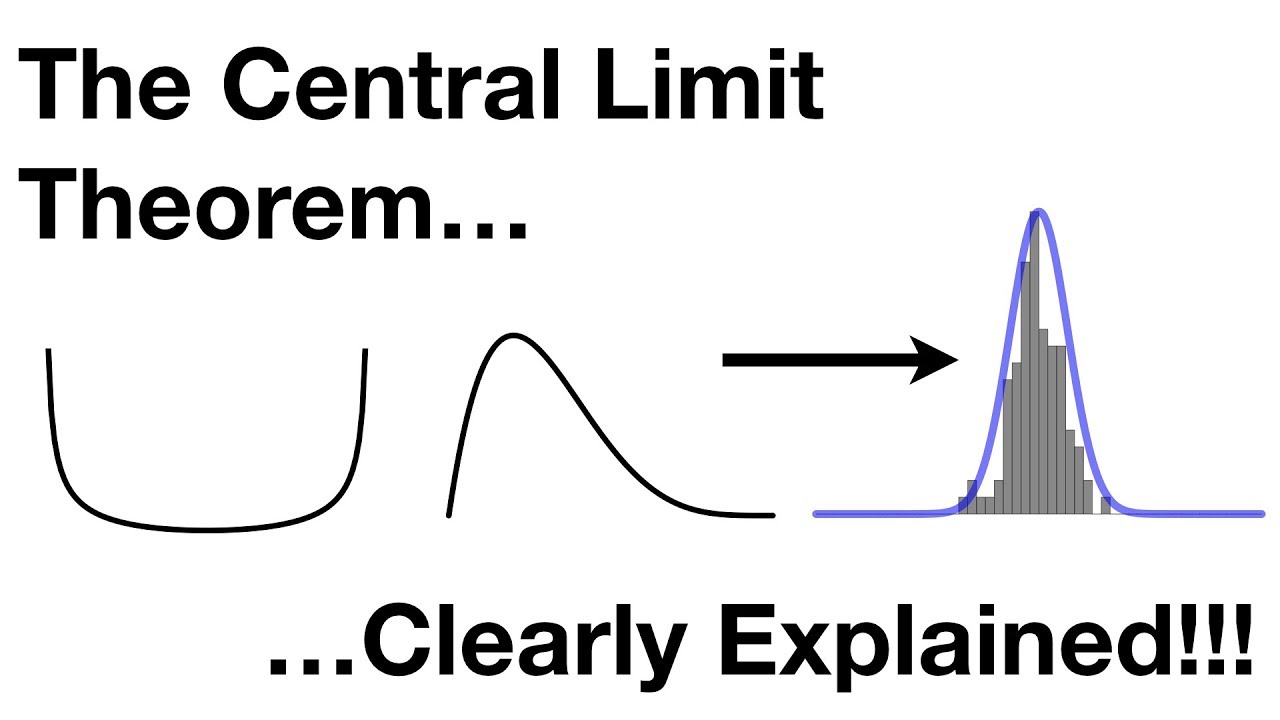
The Central Limit Theorem, Clearly Explained!!!
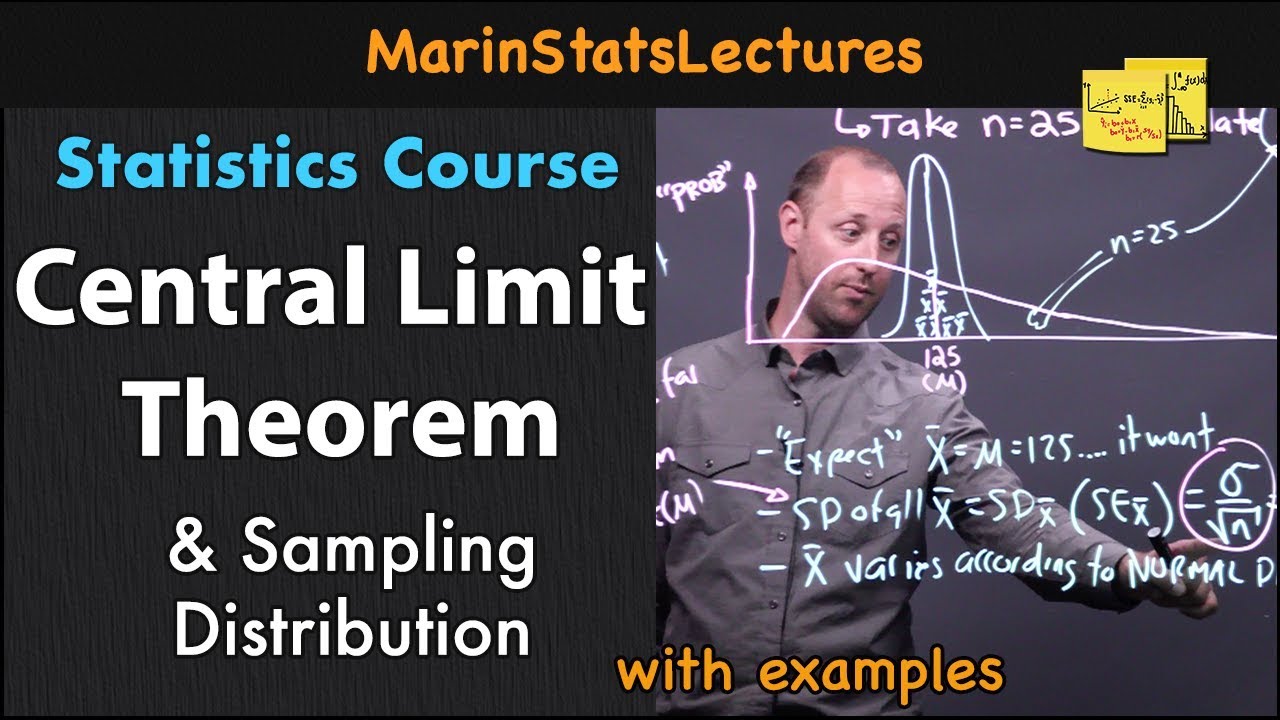
Central Limit Theorem & Sampling Distribution Concepts | Statistics Tutorial | MarinStatsLectures
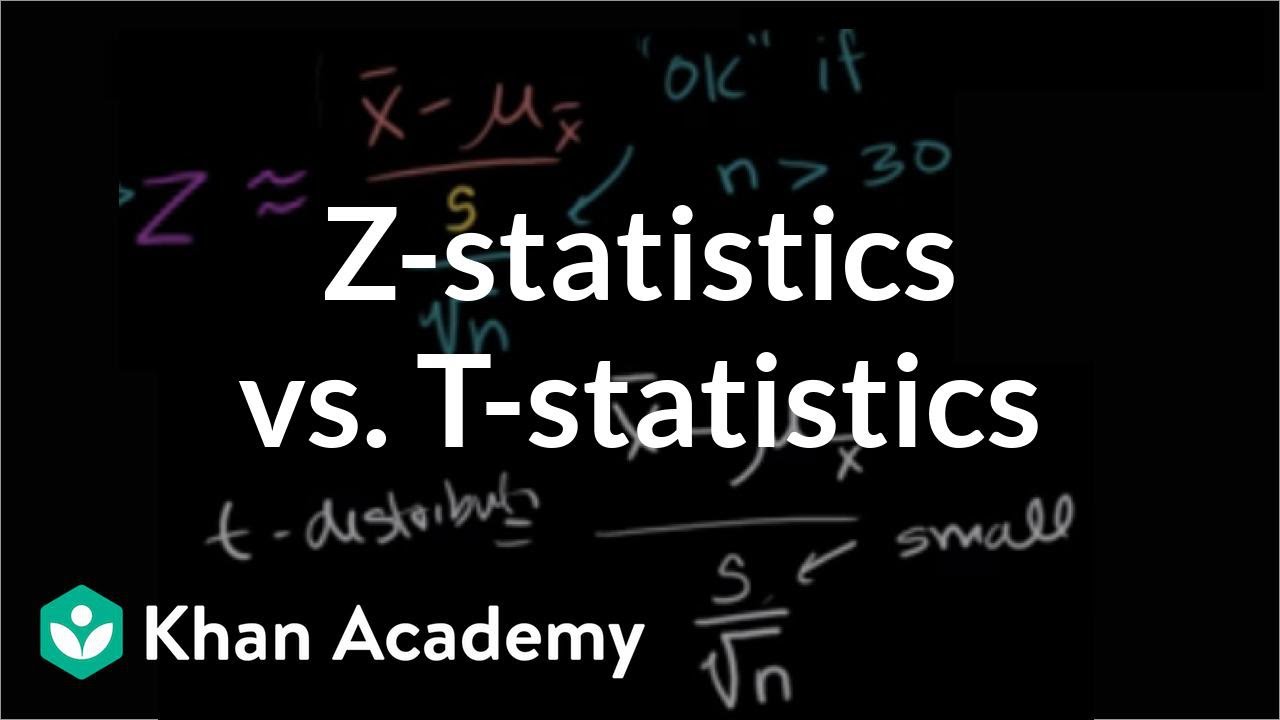
Z-statistics vs. T-statistics | Inferential statistics | Probability and Statistics | Khan Academy
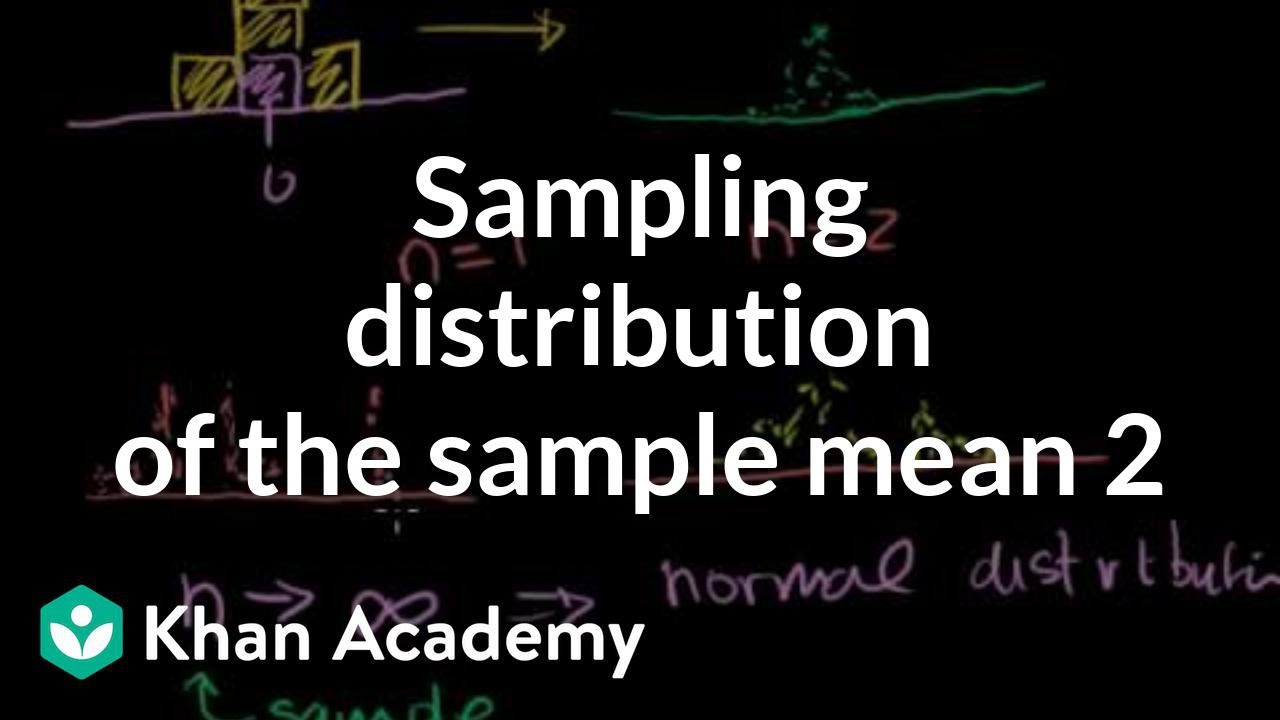
Sampling distribution of the sample mean 2 | Probability and Statistics | Khan Academy
5.0 / 5 (0 votes)
Thanks for rating: