P-Hacking: Crash Course Statistics #30
TLDRThe video discusses the problem of p-hacking, where researchers manipulate data or analyses to artificially obtain significant p-values. This can lead to false research being published. The video explains how doing multiple statistical tests increases the chance of getting spuriously significant results. It provides the jelly bean experiment and buffet pricing study as real-world examples. The video stresses the importance of pre-defining analyses, correcting for multiple comparisons, and understanding family-wise error rates to ensure statistically valid, ethical research.
Takeaways
- ๐ P-hacking is manipulating data or analyses to artificially get significant p-values
- ๐ P-hacking can have serious consequences like contributing to incorrect medical studies
- ๐ณ With enough tests, fluke statistically significant results are likely even if there's no effect
- ๐ Ideal analyses are chosen before seeing any data
- ๐ค Statistical significance can be misleading without context of other tests done
- ๐ Bonferroni corrections adjust p-values to account for multiple tests
- ๐ Publishing only statistically significant results biases science
- ๐ก Unethical data practices like p-hacking erode public trust
- ๐ค Spotting questionable science protects people from bad decisions
- ๐ Green jelly beans are the best!
Q & A
What is p-hacking?
-P-hacking is manipulating data or analyses to artificially get significant p-values.
Why might a researcher be motivated to p-hack?
-Researchers are incentivised to find significant results in order to publish their work and advance their careers. Non-significant results are less likely to be published.
How can p-hacking undermine the integrity of scientific research?
-P-hacking can lead to the publication of incorrect or misleading results. This can have consequences ranging from people making poor health choices to serious issues like the anti-vaccination movement.
What was the original hypothesis in the Cornell buffet study example?
-The original hypothesis was that there is an effect of buffet price on the amount that people eat.
Why is running multiple statistical tests on the same data problematic?
-Running multiple tests inflates the chance of getting a significant result by chance even if there is no real effect. Reporting only the significant results is misleading.
How does the jelly bean example illustrate issues with multiple comparisons?
-Testing 20 jelly bean colors substantially increases the chance of a significant result occurring by chance. Significant findings may be false positives.
What is the Family Wise Error rate?
-The inflated Type I error rate that occurs when running multiple related statistical tests is called the Family Wise Error rate.
How can researchers adjust for the Family Wise Error rate?
-Applying a Bonferroni correction adjusts the significance level to account for the number of tests being conducted.
Why should the general public care about issues like p-hacking?
-Questionable research practices can lead to poor policy decisions, health recommendations, and more that impact people's everyday lives.
What might be some non-malicious reasons behind p-hacking?
-P-hacking could come from gaps in statistical knowledge, belief in a theory leading to confirmation bias, or honest mistakes.
Outlines
๐ Understanding p-hacking and its implications
This paragraph introduces p-hacking, which involves manipulating data or analyses to artificially get significant p-values. It states that researchers are incentivized to find significant results, but sometimes things can go wrong through p-hacking. Examples of p-hacking are provided, including choosing analyses based on what makes the p-value significant rather than having a predetermined analysis plan.
๐ The high likelihood of errors when doing multiple statistical tests
This paragraph explains how doing multiple statistical tests, such as testing different colors of jelly beans, greatly increases the chances of getting a significant result just by chance. This is called the Family Wise Error rate. Even if there is no real effect, the more tests done, the more likely spurious significant results occur.
๐ก Recommendations for accountable and ethical statistical analyses
This paragraph suggests ways for researchers to do accountable, ethical statistical analyses, including: determining hypotheses and analyses before looking at data, correcting for inflated Family Wise Error rates, using Bonferroni correction to adjust p-values when doing multiple tests, and understanding that limiting false research results is important.
Mindmap
Keywords
๐กp-value
๐กp-hacking
๐กnull hypothesis
๐กType I error
๐กmultiple comparisons
๐กretraction
๐กfalse positives
๐กBonferroni correction
๐กtransparency
๐กreproducibility
Highlights
P-hacking is manipulating data or analyses to artificially get significant p-values.
Academic journals donโt want to publish results saying there's no evidence that something doesn't work.
Being able to publish results is key for job stability, salary, and prestige in science.
P-hacking is choosing analyses based on what makes the p-value significant, not the best analysis plan.
P-hacked analyses can mislead and contribute to incorrect studies with serious ramifications.
Ideally, choose analyses before seeing data. Accept some false positives due to chance.
With multiple related tests, Family Wise Error rates increase, inflating false positives.
Reporting only significant results of many tests is misleading without full context.
By 14 tests, it's likely at least 1 false positive result even if nothing there.
Bonferroni correction: Divide usual p-value threshold by # tests for new threshold.
Putting out false research matters - can affect laws, food/water regulations, more.
Spotting questionable science means not having to avoid those green jelly beans.
P-hacking is manipulating data or analyses to artificially get significant p-values.
Reporting only significant results of many tests is misleading without full context.
Bonferroni correction: Divide usual p-value threshold by # tests for new threshold.
Transcripts
Browse More Related Video
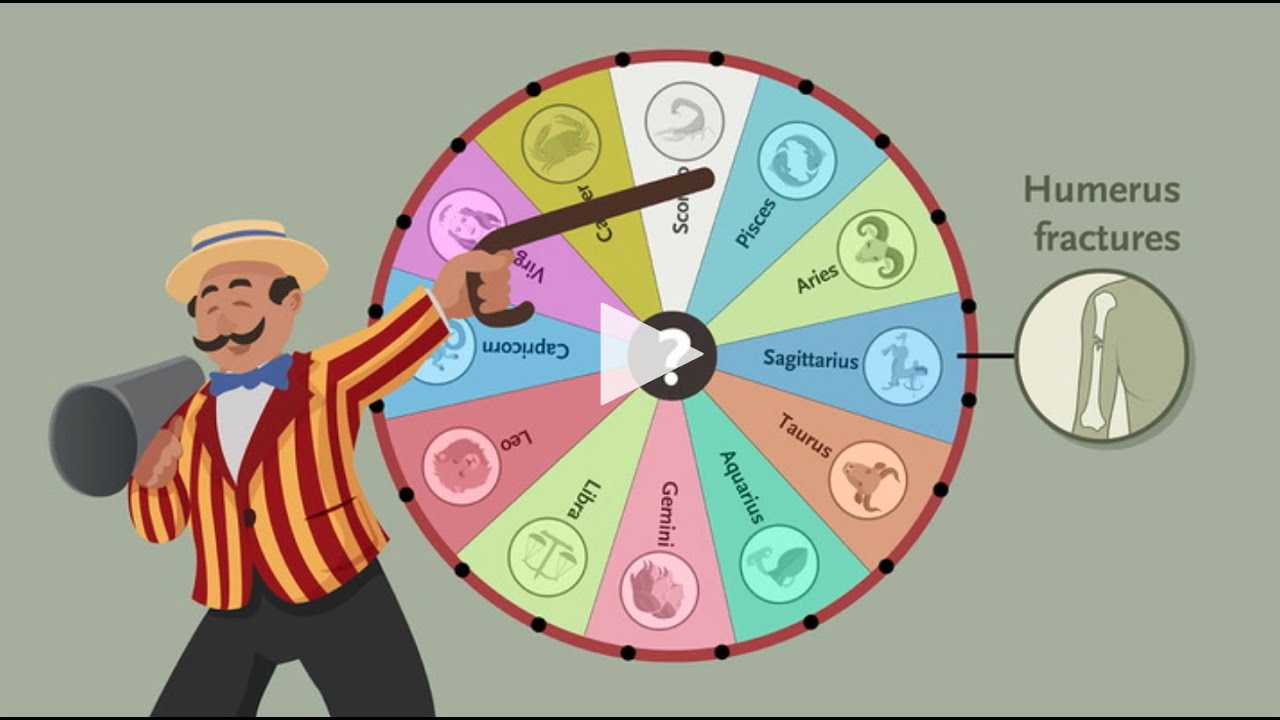
The Problem of Multiple Comparisons | NEJM Evidence
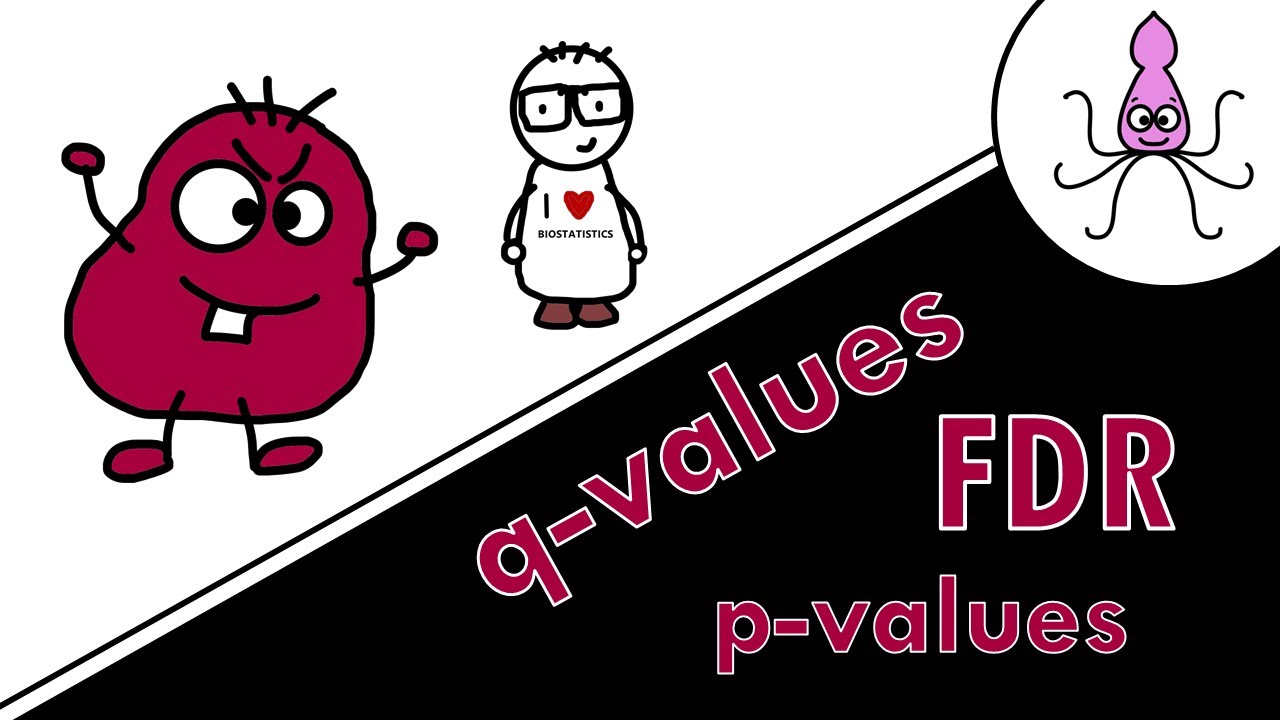
FDR, q-values vs p-values: multiple testing simply explained!
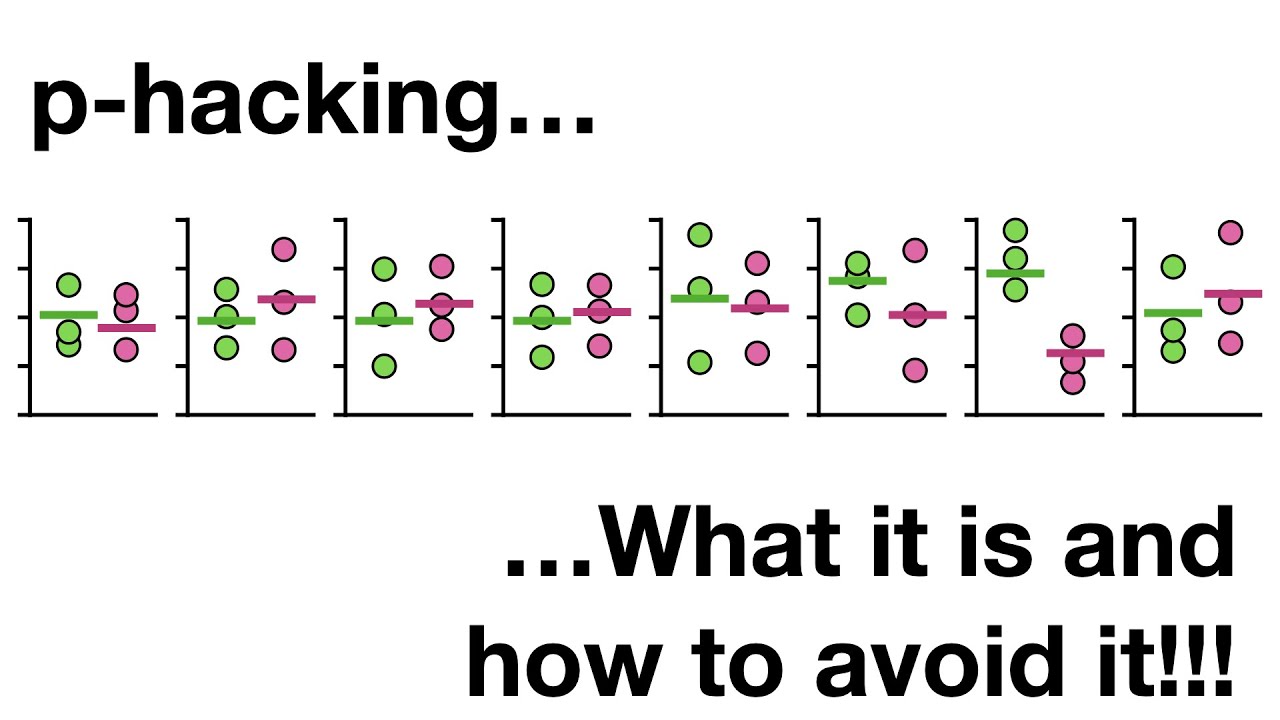
p-hacking: What it is and how to avoid it!
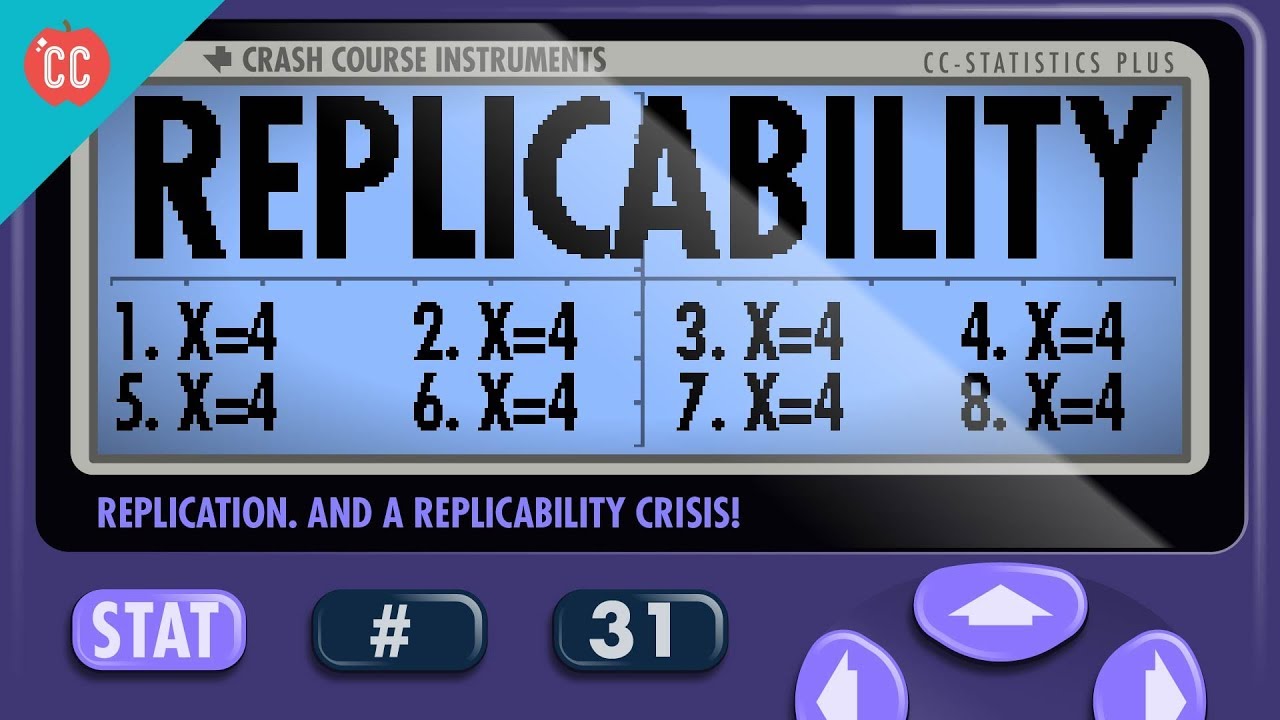
The Replication Crisis: Crash Course Statistics #31
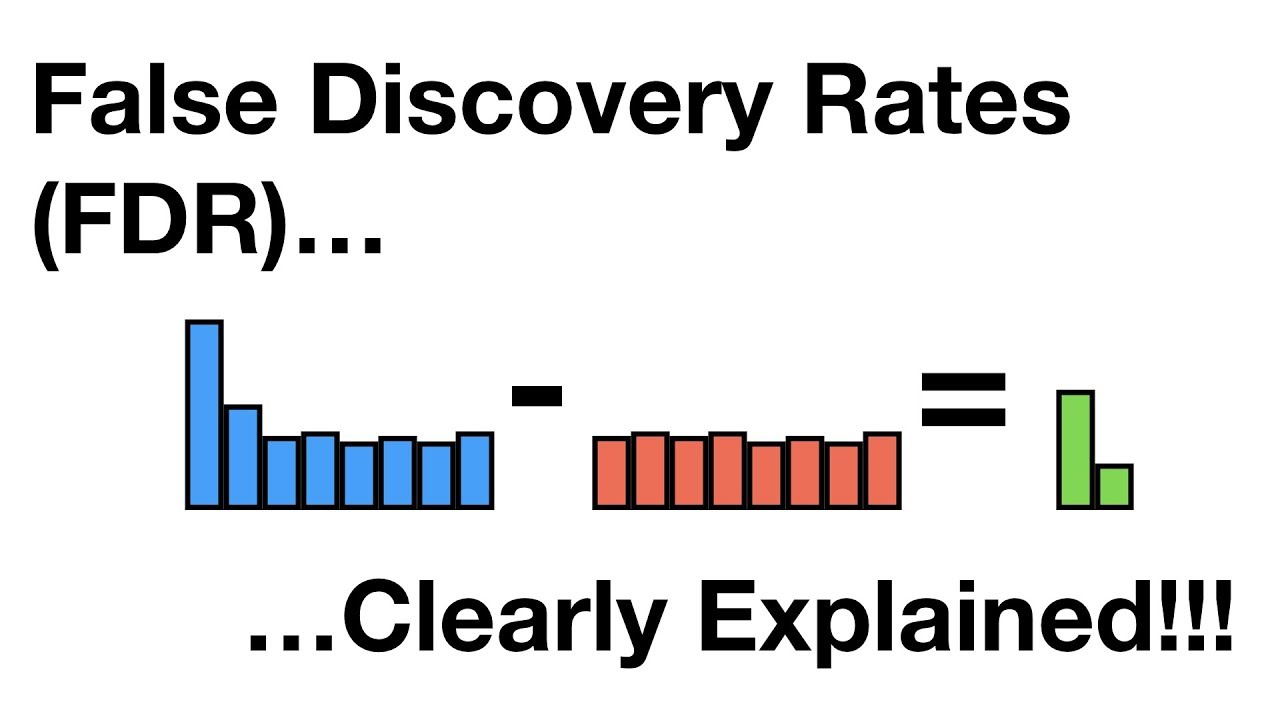
False Discovery Rates, FDR, clearly explained

Types of Variables in Research and Their Uses (Practical Research 2)
5.0 / 5 (0 votes)
Thanks for rating: