The Problem of Multiple Comparisons | NEJM Evidence
TLDRA study of 10 million adults in Ontario, Canada, explored the correlation between astrological signs and health outcomes, revealing that Sagittarians had a higher risk of humerus fractures and Leos were more likely to suffer from gastrointestinal bleeds. However, the study's true purpose was to highlight the issue of multiple hypothesis testing, which can lead to false positive results by chance. The concept of a Type 1 Error, or false positive, is introduced, where a statistical test incorrectly asserts an association when none exists. The problem of multiple comparisons, or Ξ± inflation, occurs when conducting numerous tests increases the likelihood of false positives. The family-wise error rate, calculated at 5% for a single test, can rise significantly with multiple tests, emphasizing the need for statistical methods to control the alpha level and correct for multiple comparisons. The video script cautions viewers to critically assess trials with multiple statistical tests and question the validity of highlighted positive outcomes.
Takeaways
- π A study in Ontario, Canada, examined the link between astrological signs and health outcomes, specifically finding increased risks for Sagittarians and Leos.
- π€ The study aimed to demonstrate the pitfalls of multiple hypothesis testing, which can lead to chance associations being mistaken for significant findings.
- β οΈ Multiple comparisons can result in Type 1 Errors, or false positives, where an association is wrongly claimed to exist.
- π The concept of alpha (Ξ±) is introduced as the predetermined error rate in a statistical test, commonly set at 0.05, or a 5% chance of a false positive.
- π The risk of false positives increases with the number of tests conducted, a phenomenon known as Ξ± inflation.
- π§© The family-wise error rate is the probability of getting at least one false positive in multiple tests and can be calculated using a specific formula.
- π Conducting multiple tests at Ξ± = 0.05 increases the chance of observing false positives, potentially exceeding 20% with just five tests.
- π οΈ To counteract the problem of multiple comparisons, it's necessary to control the alpha level through statistical methods that correct for multiple tests.
- π¬ Several statistical methods exist to correct for multiple comparisons, each with its considerations and applications.
- π΅οΈββοΈ Readers are encouraged to be critical of trials with multiple statistical tests and to consider whether corrections for multiple comparisons were applied.
- π A light-hearted note for Sagittarians to be cautious, playing on the study's findings, despite the emphasis on the limitations of multiple comparisons.
Q & A
What was the purpose of the study conducted by the team of researchers?
-The study aimed to illustrate the issue of multiple hypothesis testing, also known as multiple comparisons, and how it can produce associations simply by chance.
What did the researchers find regarding the relationship between astrological birth signs and health outcomes in the study?
-The researchers found that Sagittarians had an increased risk of humerus fractures and Leos had a higher probability of gastrointestinal bleed, but these findings were used to highlight the problem with multiple comparisons rather than to suggest a real association.
What is a Type 1 Error in the context of statistical hypothesis testing?
-A Type 1 Error, or false positive, occurs when we reject the null hypothesis when it is actually true, asserting an association between variables that does not exist.
What is alpha in statistical hypothesis testing, and why is it set at 0.05 in many scientific studies?
-Alpha is the predetermined level of error that researchers are willing to accept when conducting a statistical hypothesis test. It is often set at 0.05, meaning there is a 5% chance of rejecting the null hypothesis when it is true.
What is Ξ± inflation, and how does it affect the results of multiple statistical tests?
-Ξ± inflation occurs when the more tests that are performed, the more likely it becomes that we will get a false positive result. It inflates the alpha, increasing the probability of a Type 1 Error.
What is the family-wise error rate, and how is it calculated?
-The family-wise error rate is the probability of obtaining at least one false positive in a family of hypothesis tests. It can be calculated using a formula that takes into account the alpha level and the number of tests conducted.
How does the chance of observing one or more false positive results change as the number of tests increases?
-As the number of tests increases, the chance of observing one or more false positive results also increases. For example, conducting 5 tests at an Ξ± of 0.05 results in a greater than 20% chance of observing a false positive.
What are some ways to address the problem of multiple comparisons in statistical analysis?
-To address the problem of multiple comparisons, researchers can use various statistical methods to control the alpha level, ensuring that the false positive rate does not inflate due to the number of tests conducted.
Why is it important to correct for multiple comparisons in a scientific study?
-Correcting for multiple comparisons is important to maintain the integrity of the study's findings and to avoid inflating the false positive rate, which can lead to incorrect conclusions.
How should one interpret the 'positive' secondary outcome of a study that conducted multiple statistical tests?
-One should be cautious and consider whether the researchers corrected for multiple comparisons. The 'positive' outcome might be a false positive if the study did not account for the increased chance of such errors with multiple tests.
What is the humorous advice given to Sagittarians at the end of the script, and what does it imply about the study's findings?
-The humorous advice to Sagittarians to tread more carefully implies that the study's findings should not be taken at face value, as the increased risk of humerus fractures for Sagittarians was used as an example of a potential false positive result.
Outlines
π Astrological Signs and Health Outcomes Study
This paragraph discusses a study conducted on 10 million adults in Ontario, Canada, to examine the relationship between astrological birth signs and health outcomes. The study found that Sagittarians were more prone to humerus fractures and Leos to gastrointestinal bleeds. However, the findings are presented as a cautionary tale about the pitfalls of multiple hypothesis testing, also known as multiple comparisons, which can lead to associations by chance. The paragraph explains the concept of Type 1 Error, or false positives, and introduces the idea of alpha, the acceptable level of error in statistical testing, often set at 0.05. It further explores the problem of Ξ± inflation, where the likelihood of false positives increases with the number of tests conducted. The paragraph concludes with a mathematical explanation of the family-wise error rate and the importance of controlling alpha to prevent false positives in multiple statistical tests.
Mindmap
Keywords
π‘Astrological Birth Sign
π‘Health Outcomes
π‘Multiple Hypothesis Testing
π‘Type 1 Error
π‘Null Hypothesis
π‘Alpha (Ξ±)
π‘Ξ± Inflation
π‘Family-Wise Error Rate (FWER)
π‘Statistical Correction Methods
π‘Sagittarians and Humerus Fractures
π‘Leos and Gastrointestinal Bleed
Highlights
A study of 10 million adults in Ontario, Canada, investigated the link between astrological signs and health outcomes.
Sagittarians were found to have an increased risk of humerus fractures.
Leos had a higher probability of gastrointestinal bleed compared to other signs.
The study aimed to demonstrate the pitfalls of multiple hypothesis testing.
Multiple comparisons can lead to chance associations, like the ones found.
Statistical hypothesis testing carries a risk of Type 1 Error, or false positives.
Alpha level is set to determine acceptable error rate, commonly at 0.05 or 5%.
Multiple tests increase the likelihood of false positives due to alpha inflation.
Alpha inflation occurs even in well-controlled randomized studies.
The family-wise error rate can be calculated to understand the risk of false positives.
Conducting 5 tests at Ξ±=0.05 increases the chance of false positives to over 20%.
To combat multiple comparisons, alpha must be controlled to maintain a 0.05 rate.
There are various statistical methods to correct for multiple comparisons.
It's important to question whether a study corrected for multiple comparisons.
A single positive outcome in a study may not be significant if many tests were conducted.
The study humorously suggests that Sagittarians should be cautious, despite the findings being illustrative.
Transcripts
Browse More Related Video
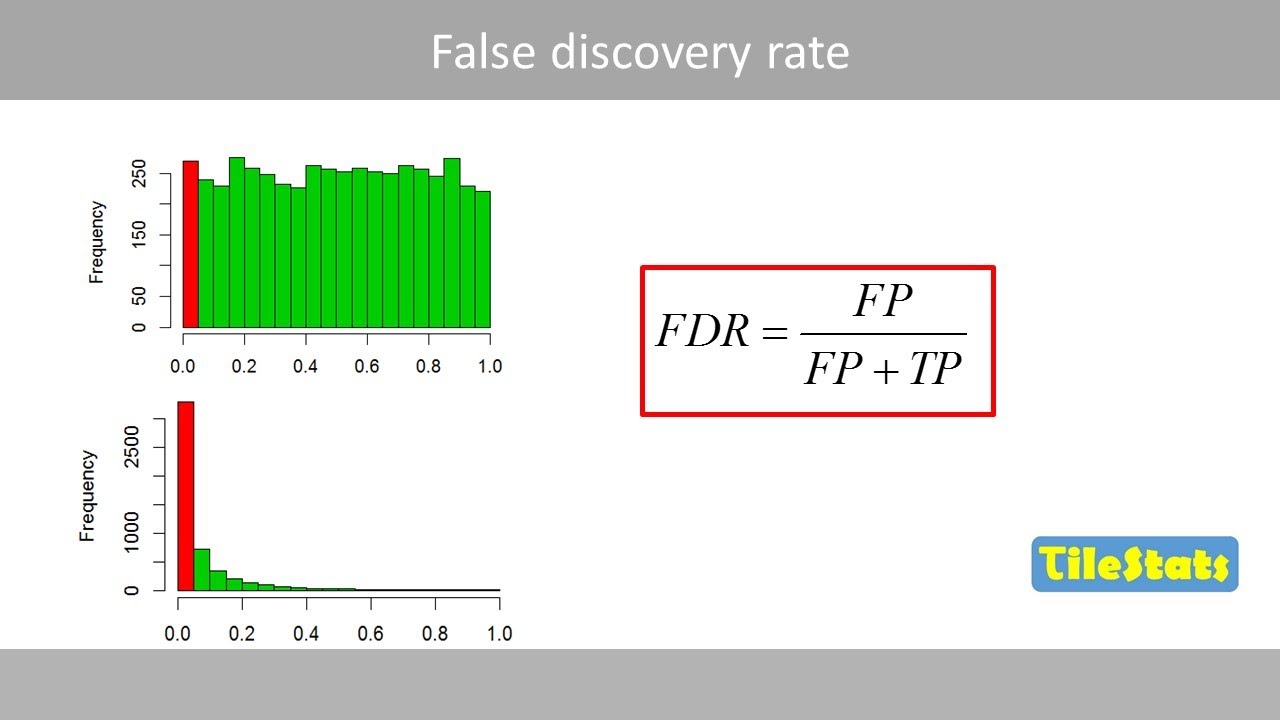
False discovery rate (FDR) - explained | vs FWER

P-Hacking: Crash Course Statistics #30
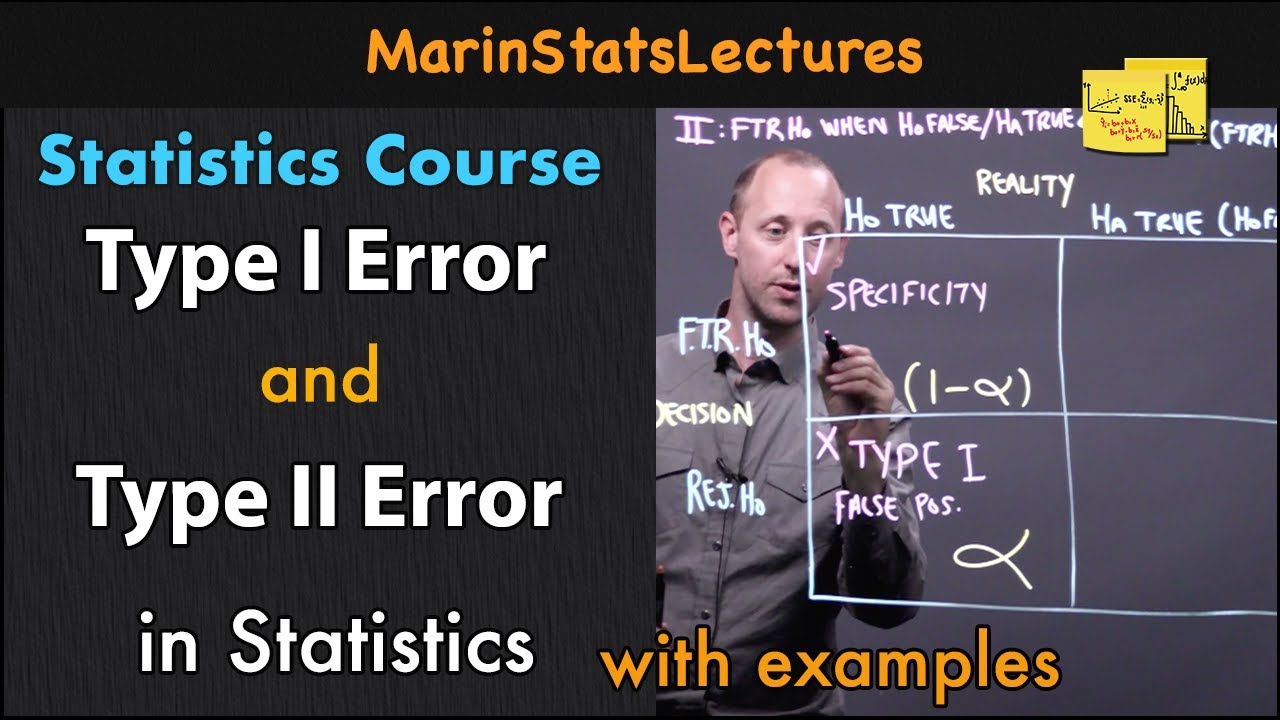
Errors and Power in Hypothesis Testing | Statistics Tutorial #16 | MarinStatsLectures
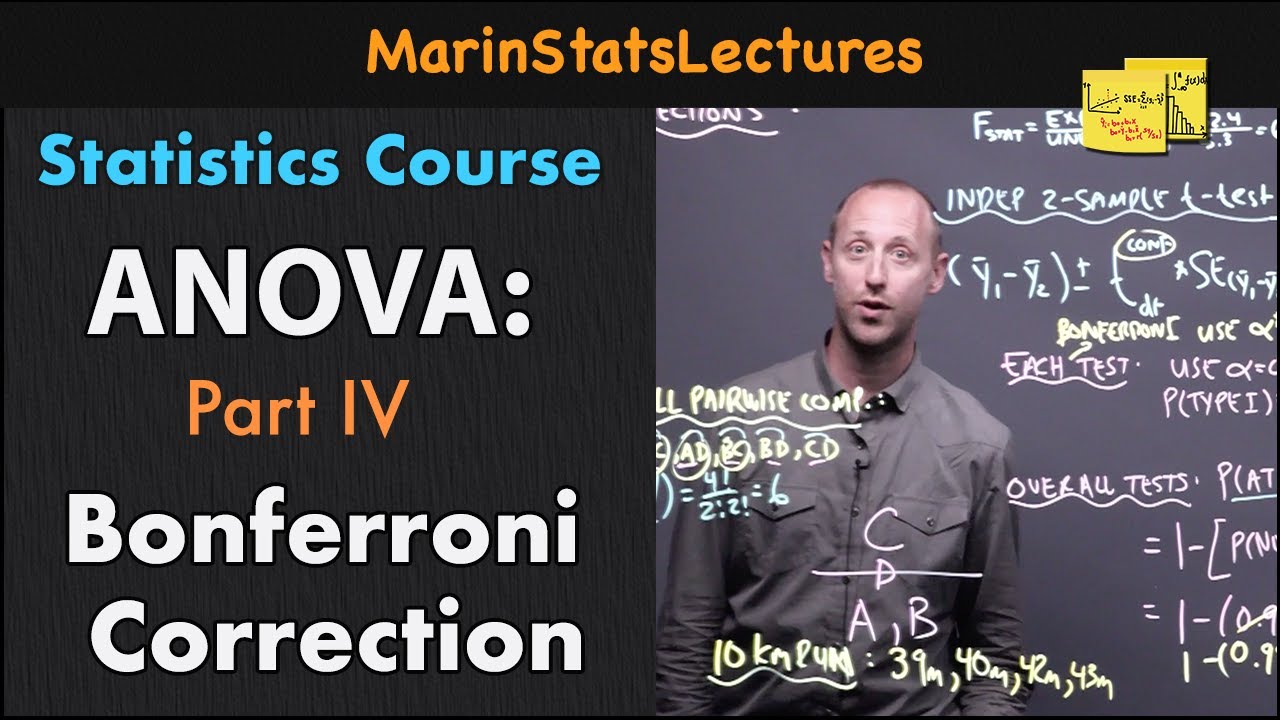
ANOVA Part IV: Bonferroni Correction | Statistics Tutorial #28 | MarinStatsLectures
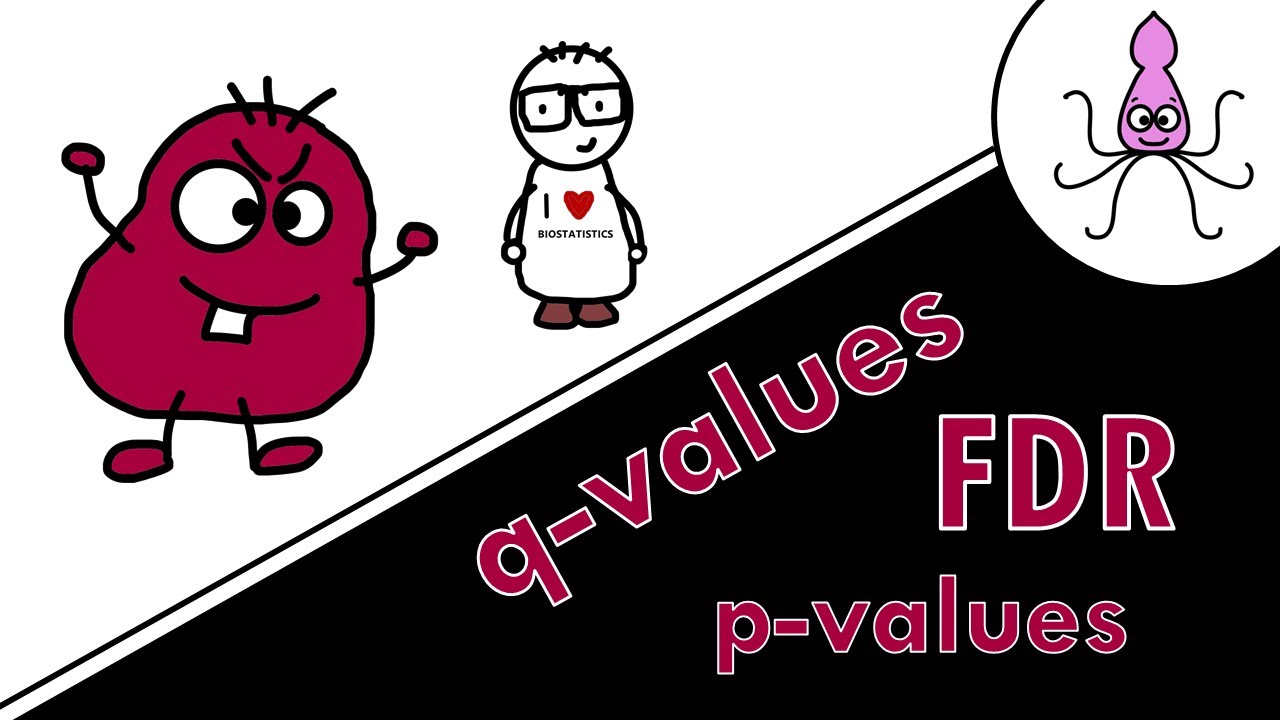
FDR, q-values vs p-values: multiple testing simply explained!

How To Identify Type I and Type II Errors In Statistics
5.0 / 5 (0 votes)
Thanks for rating: