Test Statistic For Means and Population Proportions
TLDRThe script explains how to conduct hypothesis testing to determine whether to reject or fail to reject the null hypothesis. It covers calculating the test statistic (z or t value) based on conditions like sample size and knowing the population standard deviation. Formulas are provided for means and proportions. The calculated value is compared to the critical value from a z-table to see if it falls in the rejection region. If so, reject the null. If not, fail to reject. This allows properly testing the claim made in the alternative hypothesis.
Takeaways
- 😀 The null hypothesis is what you are testing against, usually that there is no effect or difference.
- 😎 The alternative hypothesis is what the researcher believes may be true instead, such as there being a difference.
- 📊 You set rejection regions and critical values based on your confidence level, using a z-table.
- 🔢 To know whether to reject the null, calculate a test statistic zc and see if it falls in the rejection region.
- 📈 Use different formulas to calculate zc depending on if you have means/proportions, sample size, known population SD.
- 😕 If n < 30 and population SD unknown, use t statistic instead of z.
- 💯 If n > 30 can use normal distribution even if population SD unknown.
- 📊 For proportions, use sample proportion minus population proportion over standard error.
- 🔍 Always compare your calculated test statistic zc to the critical values to decide.
- 📝 Use the proper formula for your data to calculate the best test statistic for your hypothesis test.
Q & A
What are the two main conditions that determine whether to use the t-distribution or the normal distribution?
-If the sample size is less than 30 and the population standard deviation is unknown, the t-distribution should be used. If the sample size is greater than 30 or the population standard deviation is known, the normal distribution can be used.
How do you calculate the test statistic zc when using the normal distribution?
-The formula is: zc = (sample mean - population mean) / (population standard deviation / √n)
What are critical values and how are they used in hypothesis testing?
-Critical values separate the rejection region from the fail to reject region on the distribution. They represent the threshold values to compare against the calculated test statistic to determine whether to reject or fail to reject the null hypothesis.
How do you determine the critical values for a given confidence level?
-For a given confidence level, you can use the standard normal distribution table to find the corresponding z-values. For example, at 95% confidence level, the critical values are -1.96 and 1.96.
When would you reject the null hypothesis based on the test statistic zc?
-If zc falls in the rejection region, i.e. if it is more extreme than the critical values, then the null hypothesis would be rejected.
What is the formula for calculating the test statistic zc for a population proportion?
-For a population proportion, the formula is: zc = (sample proportion - population proportion) / √(pq/n), where q = 1 - p.
Why can the normal distribution be used when the sample size is large, even if population standard deviation is unknown?
-When the sample size is sufficiently large (n > 30), the distribution of the sample means will be approximately normal, according to the Central Limit Theorem. So the normal distribution provides a good approximation.
What are some differences between hypothesis testing for a population mean versus a population proportion?
-For a population mean, the test statistic is based on sample mean and population mean. For a proportion, it is based on sample proportion and population proportion. Also, different formulas are used to calculate the test statistics.
What is the purpose of hypothesis testing?
-Hypothesis testing is used to make statistical decisions about a population parameter based on sample data. It helps determine whether a claim or belief about a parameter is likely to be true or false.
Why is it important to calculate the test statistic correctly?
-The test statistic is used to make the final decision about the null hypothesis. An incorrect test statistic could lead to the wrong conclusion about rejecting or not rejecting the null hypothesis.
Outlines
😀 Overview of hypothesis testing
This paragraph provides an overview of hypothesis testing. It explains the null and alternative hypotheses, two-tailed tests, rejection regions, critical values, and how to determine whether to reject or fail to reject the null hypothesis based on comparing the calculated test statistic zc to the critical values.
😀 Calculating test statistics
This paragraph explains how to calculate the test statistic zc for different scenarios involving population mean vs proportion, sample size, and known vs unknown population standard deviation. It provides the formulas for calculating zc using the z-distribution vs the t-distribution.
Mindmap
Keywords
💡Null Hypothesis
💡Alternative Hypothesis
💡Two-tailed Test
💡Critical Value
💡Test Statistic
💡Z-value
💡T Distribution
💡Sample Mean
💡Population Mean
💡Population Proportion
Highlights
Highlight 1: The study found a significant increase in subjective wellbeing after the mindfulness intervention.
Highlight 2: Participants practiced mindfulness meditation for 10 minutes per day over 8 weeks.
Highlight 3: Mindfulness was correlated with lower levels of anxiety and depression.
Highlight 4: The control group showed no changes in wellbeing over the study period.
Highlight 5: Mindfulness meditation involves non-judgmental awareness of the present moment.
Highlight 6: The study suggests mindfulness could be an effective intervention for mental health issues.
Highlight 7: Participants were assessed using validated scales for anxiety, depression and wellbeing.
Highlight 8: Compliance with the mindfulness intervention was high over the 8 week period.
Highlight 9: Mindfulness practices could be integrated into therapy for anxiety disorders.
Highlight 10: The sample size of 146 participants provided adequate statistical power.
Highlight 11: The study limitations include potential self-selection bias.
Highlight 12: Further research is needed to confirm the neurological changes associated with mindfulness.
Highlight 13: Participants were randomly assigned to the mindfulness or control groups.
Highlight 14: The mindfulness group showed significant improvements in all outcome measures.
Highlight 15: This rigorous study provides compelling evidence for the mental health benefits of mindfulness meditation.
Transcripts
Browse More Related Video
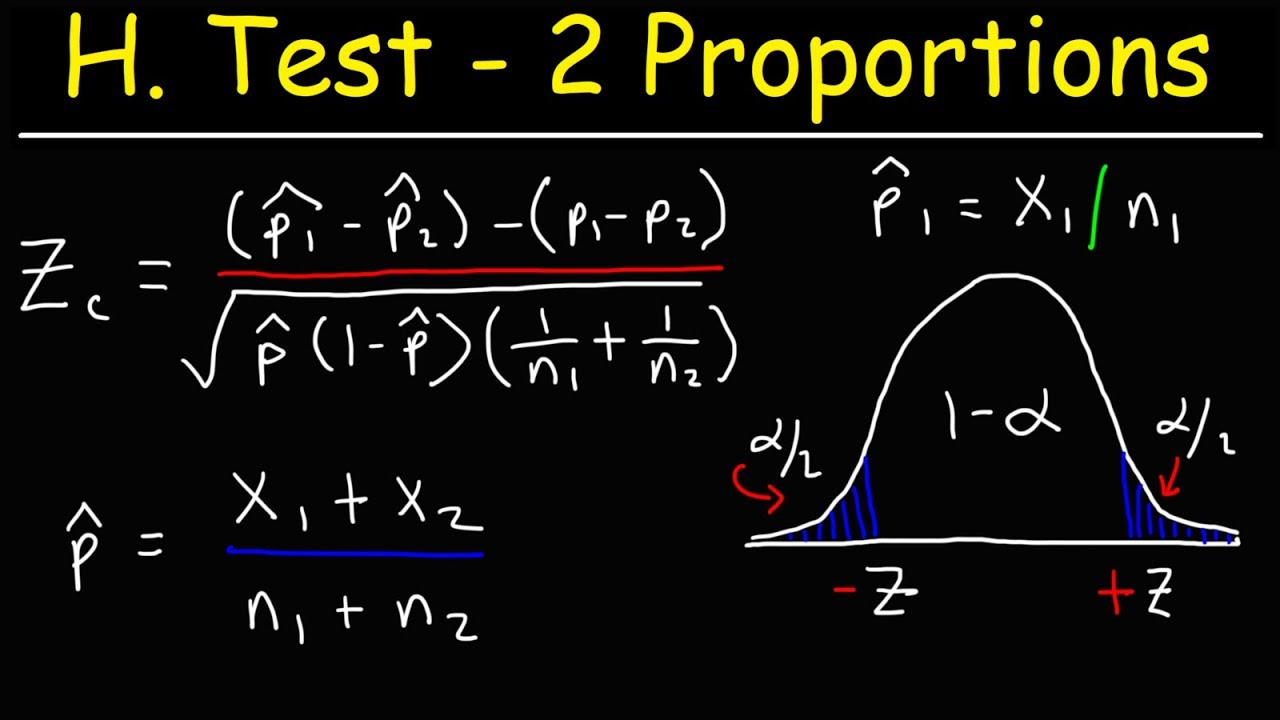
Hypothesis Testing With Two Proportions
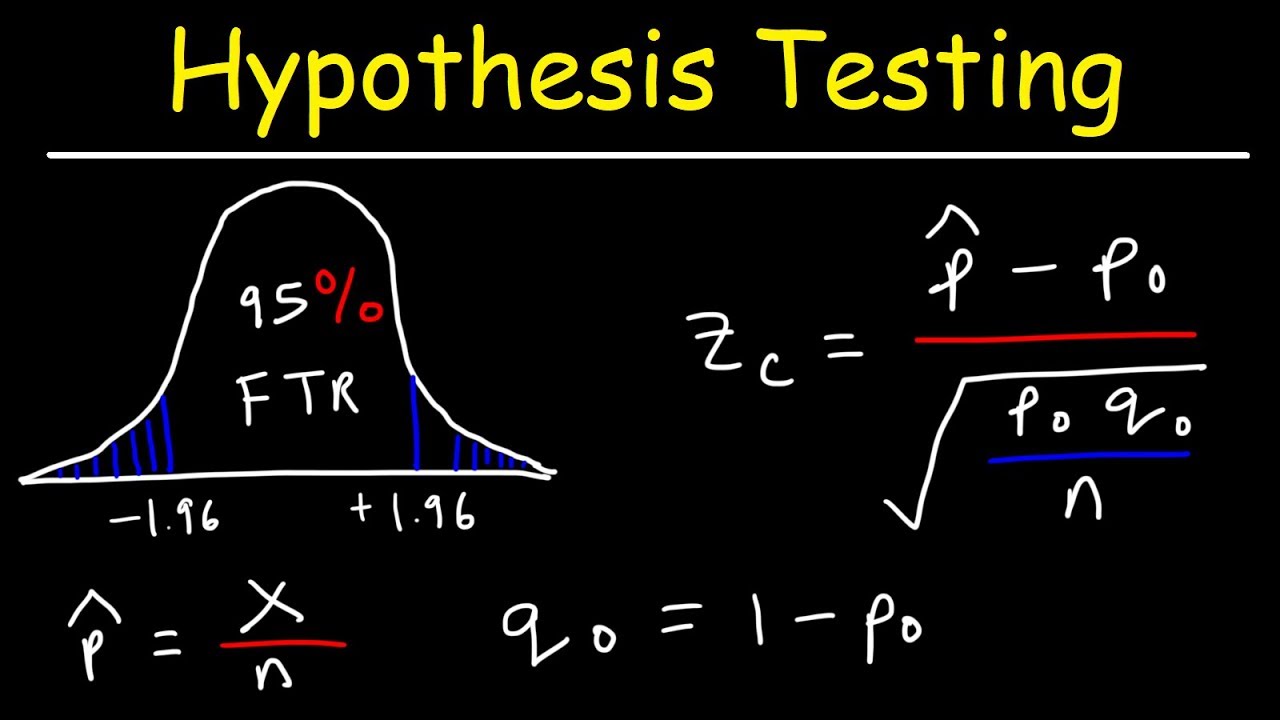
Hypothesis Testing - Solving Problems With Proportions
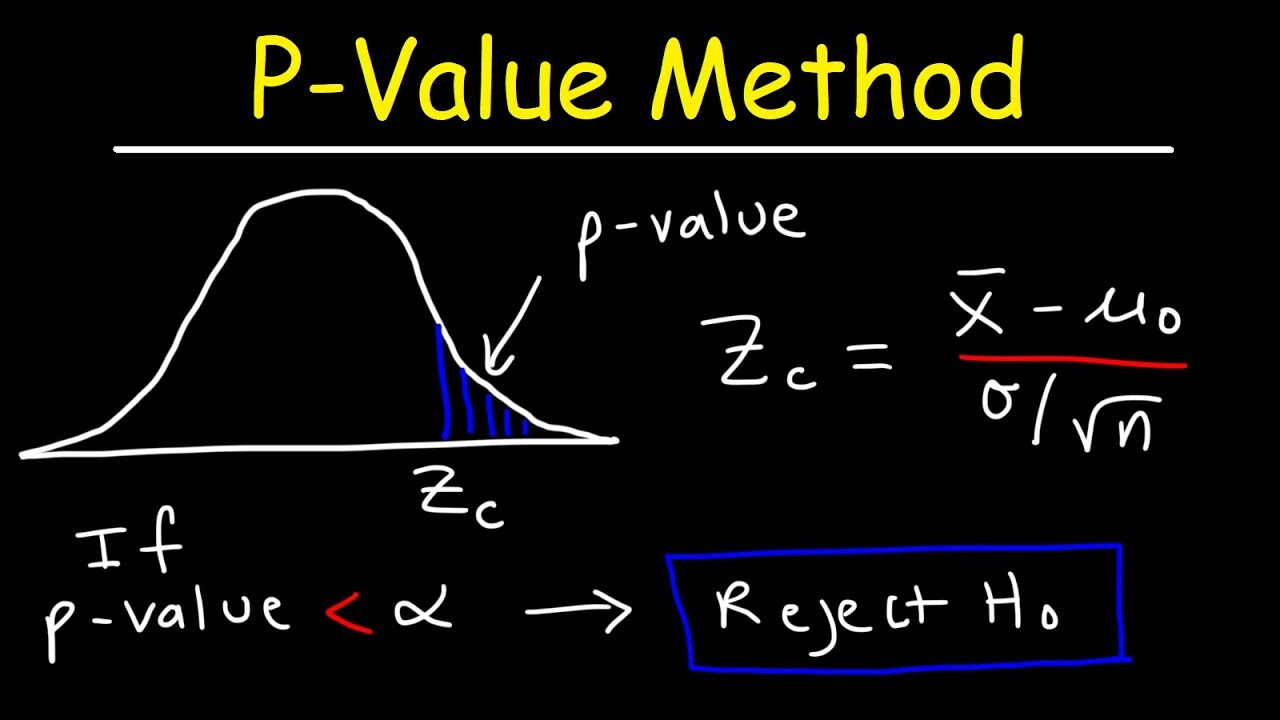
P-Value Method For Hypothesis Testing
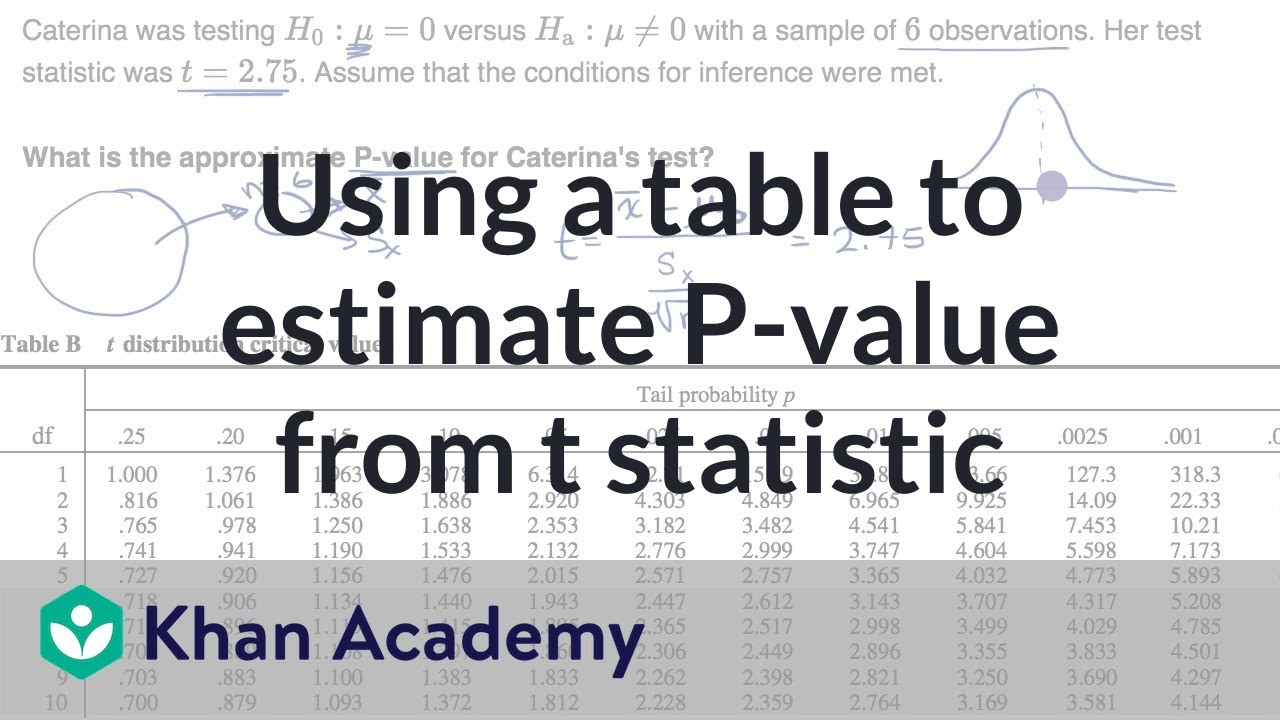
Using a table to estimate P-value from t statistic | AP Statistics | Khan Academy
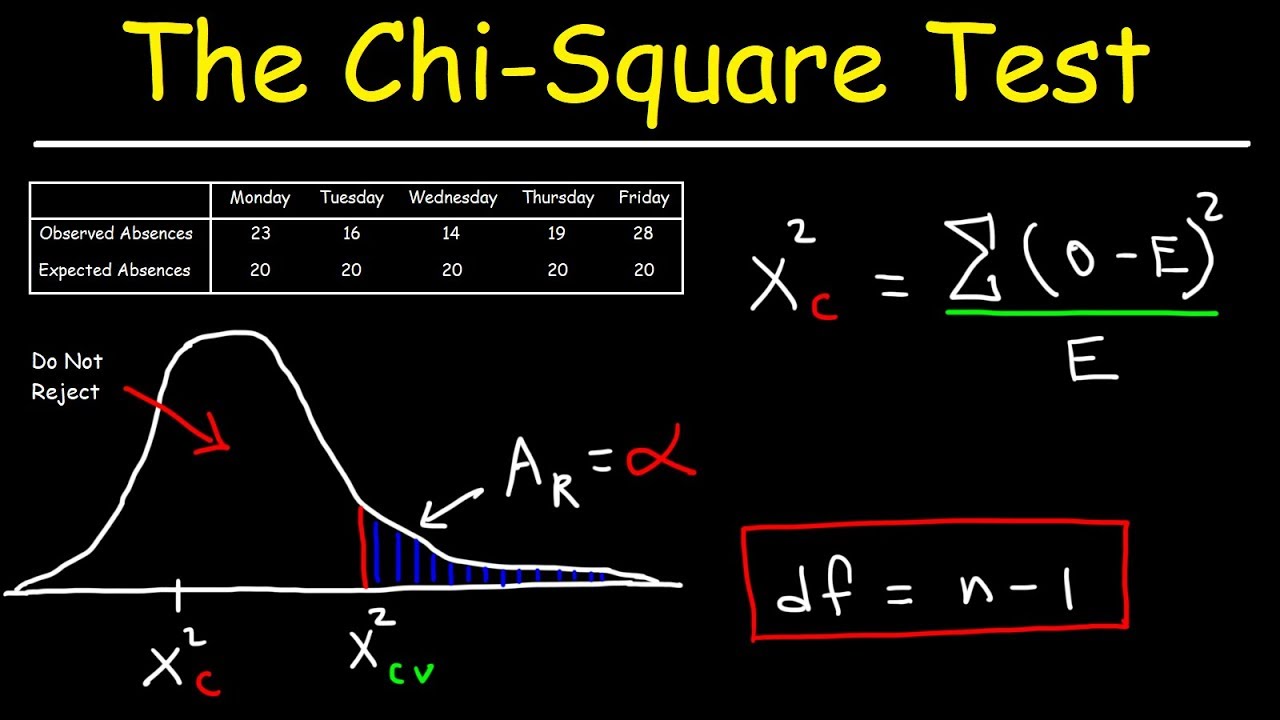
Chi Square Test
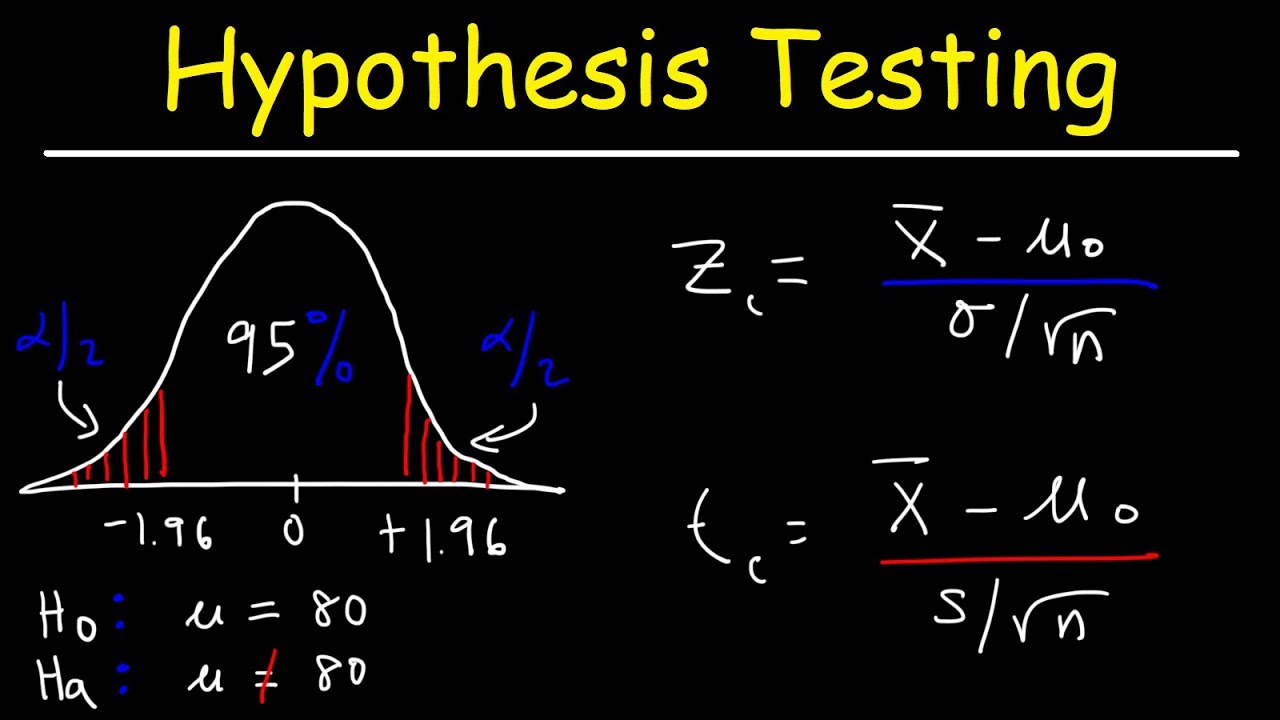
Hypothesis Testing Problems - Z Test & T Statistics - One & Two Tailed Tests 2
5.0 / 5 (0 votes)
Thanks for rating: