Type I error vs Type II error
TLDRThe video script provides an engaging explanation of errors in hypothesis testing, focusing on Type I and Type II errors. Type I error, also known as a false positive, occurs when a true null hypothesis is wrongly rejected, and the responsibility for this serious error lies with the researcher, as they choose the level of significance (alpha). Type II error happens when a false null hypothesis is accepted, with its probability (beta) being influenced by sample size and population variance. This error is considered less serious and often attributed to the difficulty of the subject matter. The video uses a humorous analogy of a romantic scenario to illustrate these errors, emphasizing the importance of understanding and managing them in statistical analysis. It concludes by highlighting the imperfect yet valuable role of statistics in decision-making.
Takeaways
- π **Type I and Type II Errors**: Hypothesis testing can lead to two types of errors, Type I (rejecting a true null hypothesis) and Type II (accepting a false null hypothesis).
- π« **Type I Error**: Also known as a false positive, it is considered more serious and occurs when the null hypothesis is true but is wrongly rejected.
- π― **Probability of Type I Error**: This error's probability is denoted by alpha, which is chosen by the researcher, making them responsible for this error.
- π€ **Type II Error**: Occurs when a false null hypothesis is accepted, and its probability is represented by beta.
- π’ **Factors Influencing Beta**: Beta depends on the sample size and the population variance, which means difficult-to-test topics or high variability can increase the likelihood of a Type II error.
- π‘ **Power of the Test**: The probability of correctly rejecting a false null hypothesis is 1 minus beta, which is the researcher's goal and is called the test's power.
- π **Increasing Test Power**: Researchers can increase the test's power by increasing the sample size.
- π **Statistical Table**: A common table is used by statisticians to summarize the types of errors made in hypothesis testing.
- π **Love Scenario Example**: An analogy is used to explain Type I and Type II errors, with the null hypothesis being that a girl likes you back.
- π **Type I Error in Love**: If she likes you but you don't act, you've made a Type I error, which is serious as you missed a potential mutual connection.
- π **Type II Error in Love**: If she doesn't like you but you ask her out anyway, you've made a Type II error, which is less serious as you can move on from the rejection.
- π§ **Imperfection of Statistics**: Statistics is a useful tool but not perfect, and errors can occur in hypothesis testing.
Q & A
What are the two types of errors that can be made in hypothesis testing?
-The two types of errors in hypothesis testing are Type I error and Type II error.
What is a Type I error in the context of hypothesis testing?
-A Type I error occurs when you reject a true null hypothesis. It is also known as a false positive.
What is the probability of making a Type I error known as?
-The probability of making a Type I error is known as alpha, which is the level of significance chosen by the researcher.
What is a Type II error and what does it involve?
-A Type II error occurs when you accept a false null hypothesis. It is less serious than a Type I error and is more likely to happen with difficult-to-test topics or those with high variability.
What is denoted by beta in the context of hypothesis testing?
-Beta denotes the probability of making a Type II error, which depends mainly on the sample size and population variance.
What is the power of a test and how is it related to the probability of rejecting a false null hypothesis?
-The power of a test is the probability of rejecting a false null hypothesis, which is equal to 1 minus beta. It represents the researcher's goal in hypothesis testing.
How can researchers generally increase the power of a test?
-Researchers can generally increase the power of a test by increasing the sample size.
In the given example about a love interest, what is considered a Type I error?
-In the example, a Type I error is made if the girl likes you back but you do not invite her out, wrongly thinking that she does not like you.
What is considered a Type II error in the love interest example?
-A Type II error is made if you invite the girl out, believing she likes you back (a false null hypothesis), but she actually does not and turns you down.
Why is a Type II error considered less serious than a Type I error?
-A Type II error is considered less serious because it does not lead to a missed opportunity or a significant loss, unlike a Type I error which could result in a missed chance at a potentially successful relationship.
What is the main takeaway from the love/life/statistics lesson?
-The main takeaway is that while you aim to avoid both types of errors in hypothesis testing, it's important to understand that statistics is a useful but not perfect tool, and errors can occur.
How does the speaker aim to make the lecture on hypothesis testing errors engaging?
-The speaker aims to make the lecture engaging by first defining the problems, then providing interesting real-life examples, and maintaining a light-hearted tone throughout the explanation.
Outlines
π Understanding Hypothesis Testing Errors
This paragraph introduces the topic of errors in hypothesis testing, explaining the two main types: type I and type II errors. It emphasizes that while these errors might seem dull, the lecture promises to be engaging. Type I error, also known as a false positive, occurs when a true null hypothesis is rejected and is considered more serious. The probability of this error is denoted by alpha, which is chosen by the researcher. Type II error happens when a false null hypothesis is accepted; its probability is represented by beta and is influenced by sample size and population variance. The text also introduces the concept of the power of a test, which is the probability of correctly rejecting a false null hypothesis, equal to 1 minus beta. Researchers can increase this power by increasing the sample size.
Mindmap
Keywords
π‘Hypothesis Testing
π‘Type I Error
π‘Type II Error
π‘Alpha (Level of Significance)
π‘Beta (Ξ²)
π‘Sample Size
π‘Population Variance
π‘Power of the Test
π‘Statistical Significance
π‘Null Hypothesis
π‘Alternative Hypothesis
Highlights
Hypothesis testing involves two types of errors: Type I and Type II.
Type I error, or false positive, is when a true null hypothesis is rejected.
The probability of a Type I error is denoted by alpha, chosen by the researcher.
Type II error occurs when a false null hypothesis is accepted.
Beta represents the probability of a Type II error and is influenced by sample size and population variance.
Type II errors are considered less serious, often due to the difficulty in testing the topic.
The power of the test, or the probability of rejecting a false null hypothesis, is 1 minus beta.
Increasing the sample size is a common method to increase the power of a test.
Researchers aim to maximize the power of their tests to minimize errors.
An example of Type I error involves missing an opportunity due to incorrectly rejecting a true null hypothesis.
In the given example, not inviting a girl out when she likes you back is a Type I error.
A Type II error is illustrated by wrongly accepting a false null hypothesis and facing an awkward outcome.
Inviting a girl out when she doesn't like you back, despite the false null hypothesis, is a Type II error.
Statistics is a useful but not perfect tool, and errors in hypothesis testing are sometimes inevitable.
Understanding the implications of Type I and Type II errors is crucial for researchers.
The lecture uses a relatable scenario of love and relationships to explain statistical concepts.
The importance of hypothesis testing in decision-making is emphasized through the analogy.
The lecture concludes by reminding viewers of the practical applications of statistical analysis in everyday life.
Transcripts
Browse More Related Video
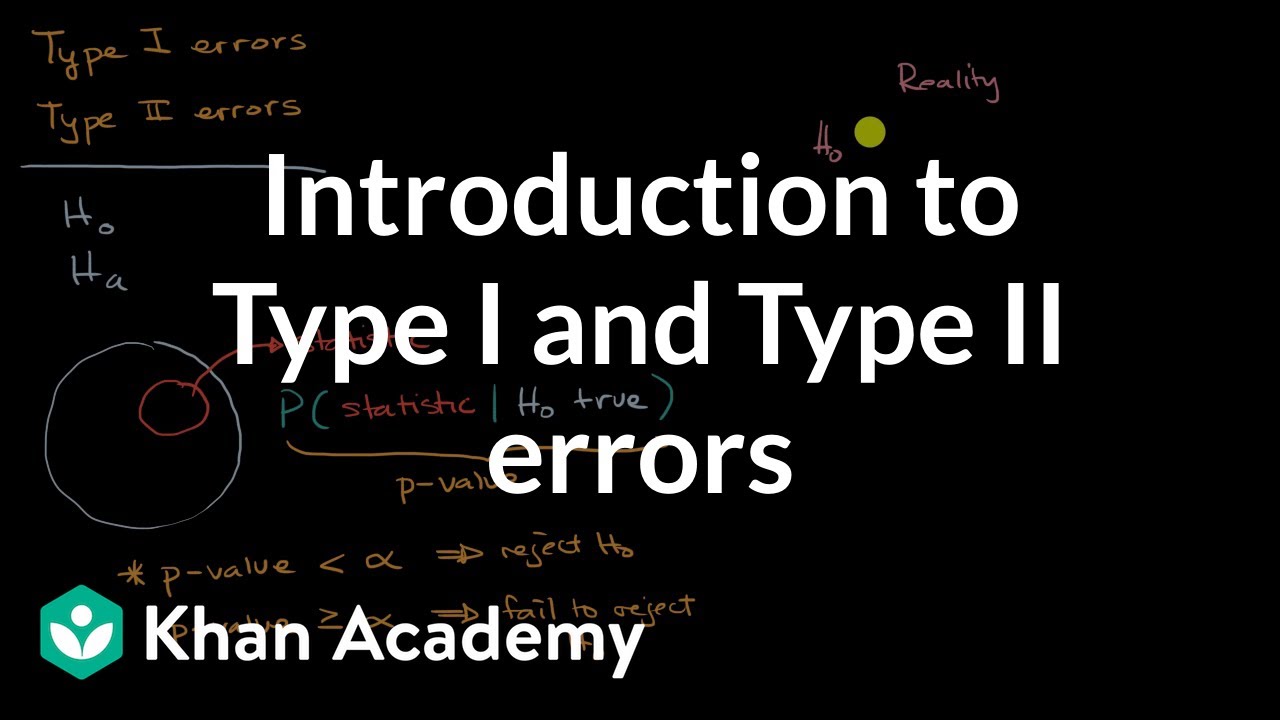
Introduction to Type I and Type II errors | AP Statistics | Khan Academy
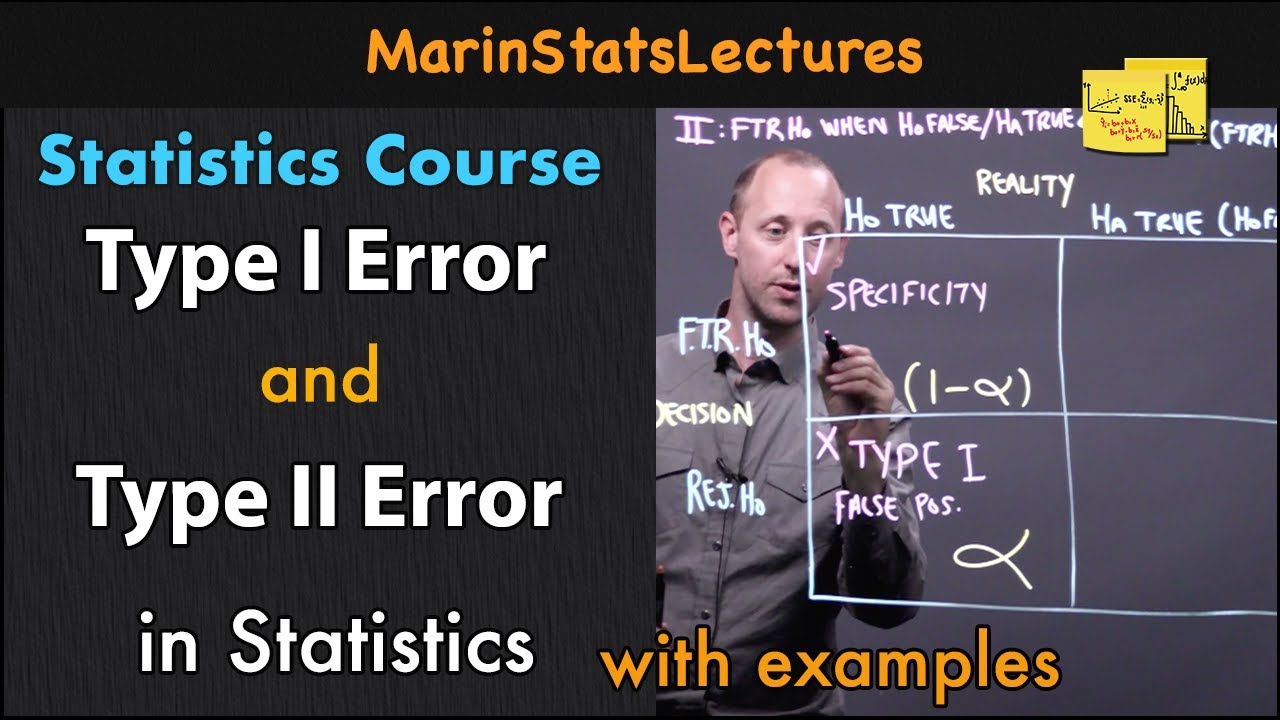
Errors and Power in Hypothesis Testing | Statistics Tutorial #16 | MarinStatsLectures

How To Identify Type I and Type II Errors In Statistics
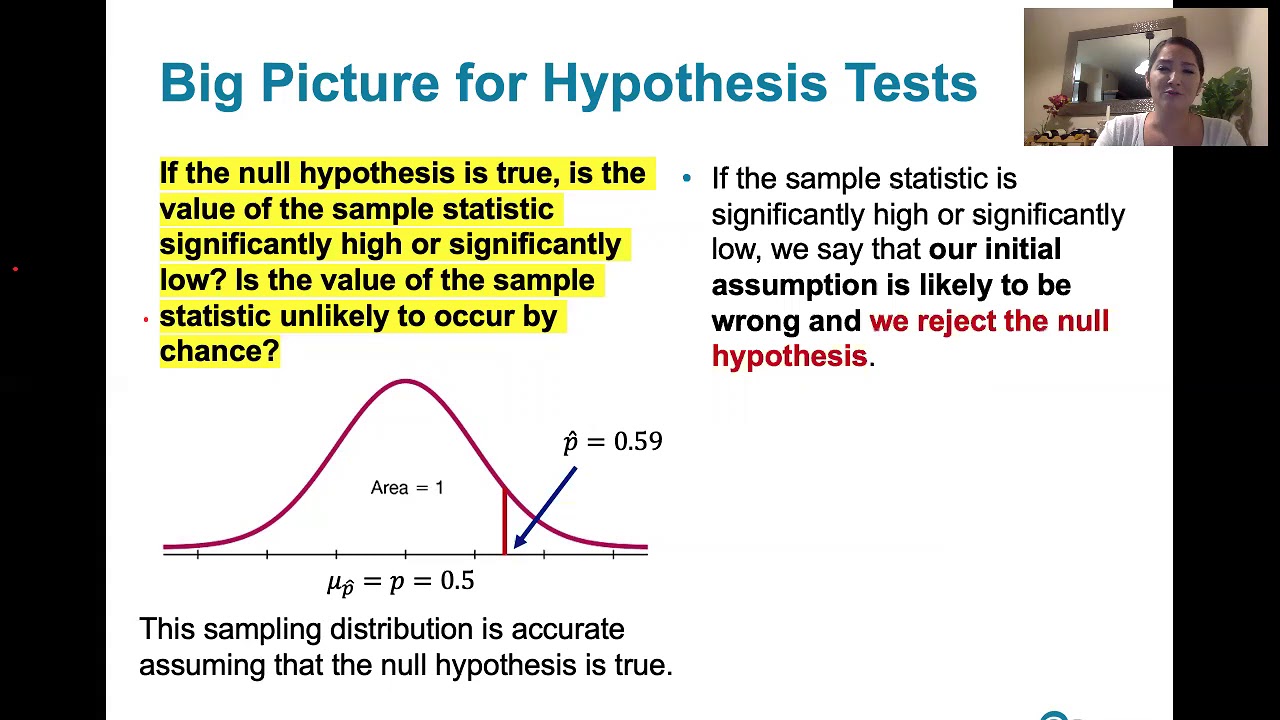
8.1.2 Basics of Hypothesis Testing - Significance Level, Type I and Type II Error
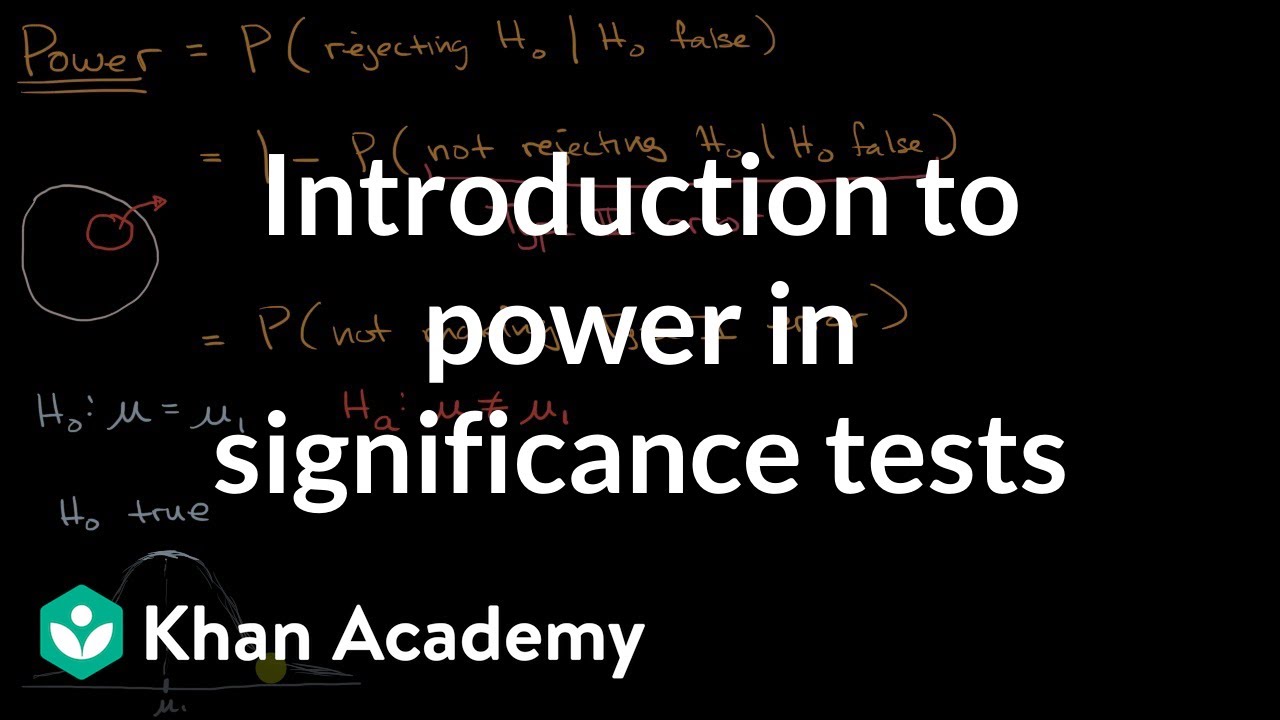
Introduction to power in significance tests | AP Statistics | Khan Academy

Type 1 (Alpha) vs. Type 2 (Beta) Error
5.0 / 5 (0 votes)
Thanks for rating: