Errors and Power in Hypothesis Testing | Statistics Tutorial #16 | MarinStatsLectures
TLDRThis video script delves into the concept of Type 1 and Type 2 errors in hypothesis testing. It explains that a Type 1 error, or false positive, occurs when the null hypothesis is incorrectly rejected, while a Type 2 error, or false negative, happens when the null hypothesis is not rejected despite the alternative being true. The video uses examples such as court cases and drug testing to illustrate these errors. It also discusses the probability of these errors, denoted by alpha for Type 1 and beta for Type 2, and highlights the trade-off between them. The script sets the stage for a future video that will explore the calculation of these error probabilities and the concept of test power in more detail.
Takeaways
- 🔍 When testing a hypothesis, there are two types of errors that can be made: Type 1 (false positive) and Type 2 (false negative).
- ❌ Type 1 error occurs when the null hypothesis is incorrectly rejected when it is actually true.
- ✅ Type 2 error happens when the null hypothesis is not rejected when it should be, given that the alternative hypothesis is true.
- 🎯 The probability of making a Type 1 error is denoted by alpha (α), which is chosen by the researcher and often set at 5%.
- 🎭 In a court case analogy, a Type 1 error equates to convicting an innocent person, while a Type 2 error results in setting a guilty person free.
- 💊 In drug testing, a Type 1 error leads to approving an ineffective drug, and a Type 2 error causes the rejection of an effective drug.
- 🔄 There is a trade-off between Type 1 and Type 2 errors; reducing one type increases the likelihood of the other.
- 🔢 The probability of making a Type 2 error is denoted by beta (β), and the power of a test (1 - β) is the probability of correctly rejecting the null hypothesis when the alternative is true.
- 📈 Increasing the sample size reduces the likelihood of making a Type 2 error.
- 🔍 The 'difference we wish to detect' in a study affects the probability of making a Type 2 error; the larger the difference, the lower the likelihood of a Type 2 error.
- 📊 Further videos will delve into calculating the power of a test and understanding the impact of sample size and the desired detectable difference.
Q & A
What are the two types of errors discussed in the video?
-The two types of errors discussed are Type 1 error, also known as a false positive, where the null hypothesis is rejected when it is actually true, and Type 2 error, also known as a false negative, where the null hypothesis is not rejected when it is false.
What is the probability associated with a Type 1 error?
-The probability associated with a Type 1 error is denoted by alpha (α). It represents the probability of rejecting the null hypothesis when it is true.
What is the probability associated with a Type 2 error?
-The probability associated with a Type 2 error is denoted by beta (β). It represents the probability of failing to reject the null hypothesis when the alternative hypothesis is true.
In the context of a court case, what would be an example of a Type 1 error?
-In a court case, a Type 1 error would occur if an innocent person is found guilty, meaning the null hypothesis (not guilty) is rejected when it should not be.
In the context of a court case, what would be an example of a Type 2 error?
-In a court case, a Type 2 error would occur if a guilty person is found not guilty, meaning the null hypothesis (not guilty) is not rejected when it should be.
What is the significance of the term 'power' in hypothesis testing?
-In hypothesis testing, 'power' refers to the probability of correctly rejecting the null hypothesis when the alternative hypothesis is true. It is calculated as 1 minus the Type 2 error rate (1 - β) and represents the test's ability to detect an effect when there is one.
How does the choice of alpha affect the likelihood of making a Type 2 error?
-As the alpha (Type 1 error rate) increases, the likelihood of making a Type 2 error decreases. This is because a higher alpha means the threshold for rejecting the null hypothesis is lower, making it easier to detect an effect if there is one.
What is the trade-off between Type 1 and Type 2 errors?
-There is a trade-off between Type 1 and Type 2 errors where increasing the likelihood of one decreases the likelihood of the other. A higher alpha (more Type 1 errors) leads to fewer Type 2 errors, and vice versa.
How does sample size impact the probability of making a Type 2 error?
-As the sample size increases, the probability of making a Type 2 error decreases. This is because a larger sample provides more data, which can more accurately reflect the true state of the population and reduce the chance of failing to detect an effect.
What is the historical context behind the commonly accepted alpha level of 5%?
-The commonly accepted alpha level of 5% originated from a suggestion by Ronald Fisher, who proposed that making a false positive (Type 1 error) in one out of 20 times was an acceptable rate. This number is arbitrary but has become a standard in statistical testing.
What is the relationship between the 'difference we wish to detect' and the probability of making a Type 2 error?
-As the 'difference we wish to detect' increases, the probability of making a Type 2 error decreases. This is because a larger effect size is easier to detect, thus reducing the likelihood of failing to identify it with the test.
What are the implications of making a Type 1 error in the context of drug testing?
-In the context of drug testing, making a Type 1 error would result in the approval of a drug that does not actually work, leading to the promotion of an ineffective treatment.
What are the implications of making a Type 2 error in the context of drug testing?
-In the context of drug testing, making a Type 2 error would result in the failure to approve a drug that actually works, missing out on a potentially beneficial treatment for patients.
Outlines
🔍 Understanding Type 1 and Type 2 Errors
This paragraph introduces the concept of Type 1 and Type 2 errors in hypothesis testing. It explains that when testing a hypothesis, there are two possible errors: rejecting the null hypothesis when it is true (Type 1 error or false positive) and failing to reject the null hypothesis when it is false (Type 2 error or false negative). The paragraph also introduces the terms 'alpha' for the probability of a Type 1 error and 'beta' for the probability of a Type 2 error, highlighting that these errors are fundamental to understanding the risks in decision-making processes.
📚 Examples of Type 1 and Type 2 Errors
The second paragraph provides real-world examples to illustrate Type 1 and Type 2 errors. It uses the analogy of a court case, where a Type 1 error would be convicting an innocent person, and a Type 2 error would be acquitting a guilty person. Another example is drug testing, where a Type 1 error would be approving an ineffective drug, and a Type 2 error would be not approving an effective drug. The paragraph emphasizes that the severity of these errors depends on the context and societal values.
📈 The Relationship Between Type 1 and Type 2 Errors
This paragraph delves into the relationship and trade-off between Type 1 and Type 2 errors. It explains that as the likelihood of a Type 1 error (alpha) increases, the likelihood of a Type 2 error (beta) decreases, and vice versa. The paragraph also mentions that the sample size and the desired level of significance (the difference we wish to detect) play a role in the error rates. It concludes by noting that these concepts will be further explored in a future video, hinting at a deeper numerical analysis to come.
Mindmap
Keywords
💡Hypothesis Testing
💡Type 1 Error
💡Type 2 Error
💡Null Hypothesis
💡Alternative Hypothesis
💡Probability
💡Error
💡Alpha
💡Beta
💡Power of a Test
💡Sample Size
Highlights
The video discusses the concept of Type 1 and Type 2 errors in hypothesis testing.
Type 1 error, also known as a false positive, occurs when the null hypothesis is rejected when it is actually true.
Type 2 error, or false negative, happens when the null hypothesis is not rejected even though it is false.
The probability of making a Type 1 error is denoted by alpha and can be controlled by the researcher.
The probability of making a Type 2 error is denoted by beta.
In a court case example, a Type 1 error would be convicting an innocent person, while a Type 2 error would be acquitting a guilty person.
In drug testing, a Type 1 error could lead to approving an ineffective drug, and a Type 2 error could result in not approving an effective drug.
The decision-making process in hypothesis testing involves either rejecting or failing to reject the null hypothesis.
The true positive rate, or power of a test, is the probability of correctly rejecting the null hypothesis when the alternative is true.
There is a trade-off between Type 1 and Type 2 errors; as one decreases, the other tends to increase.
The sample size can impact the probability of making a Type 2 error, with larger samples reducing the likelihood.
The desired level of detection difference also affects the Type 2 error rate, with a larger difference reducing the error rate.
The video provides a table to help remember the types of errors and their outcomes.
The concept of specificity is introduced as a correct decision to not reject the null hypothesis when it is true.
The video emphasizes the importance of understanding the balance between Type 1 and Type 2 errors in decision-making.
The video is part of a series that will include a numerical focus on calculating the probabilities of Type 1 and Type 2 errors.
The impact of Type 1 and Type 2 errors varies by context, with some situations prioritizing one type of error over the other.
The video concludes with a call to action for viewers to subscribe for more content on this topic.
Transcripts
Browse More Related Video

How To Identify Type I and Type II Errors In Statistics

Type 1 (Alpha) vs. Type 2 (Beta) Error
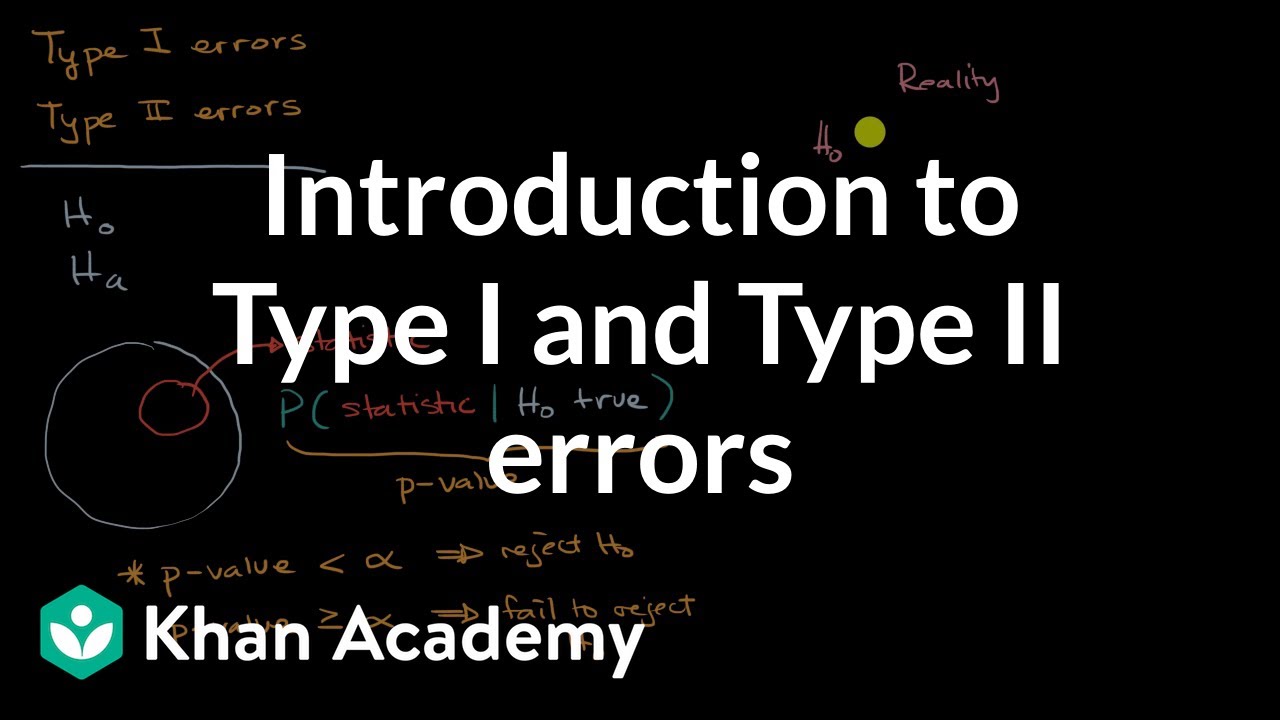
Introduction to Type I and Type II errors | AP Statistics | Khan Academy
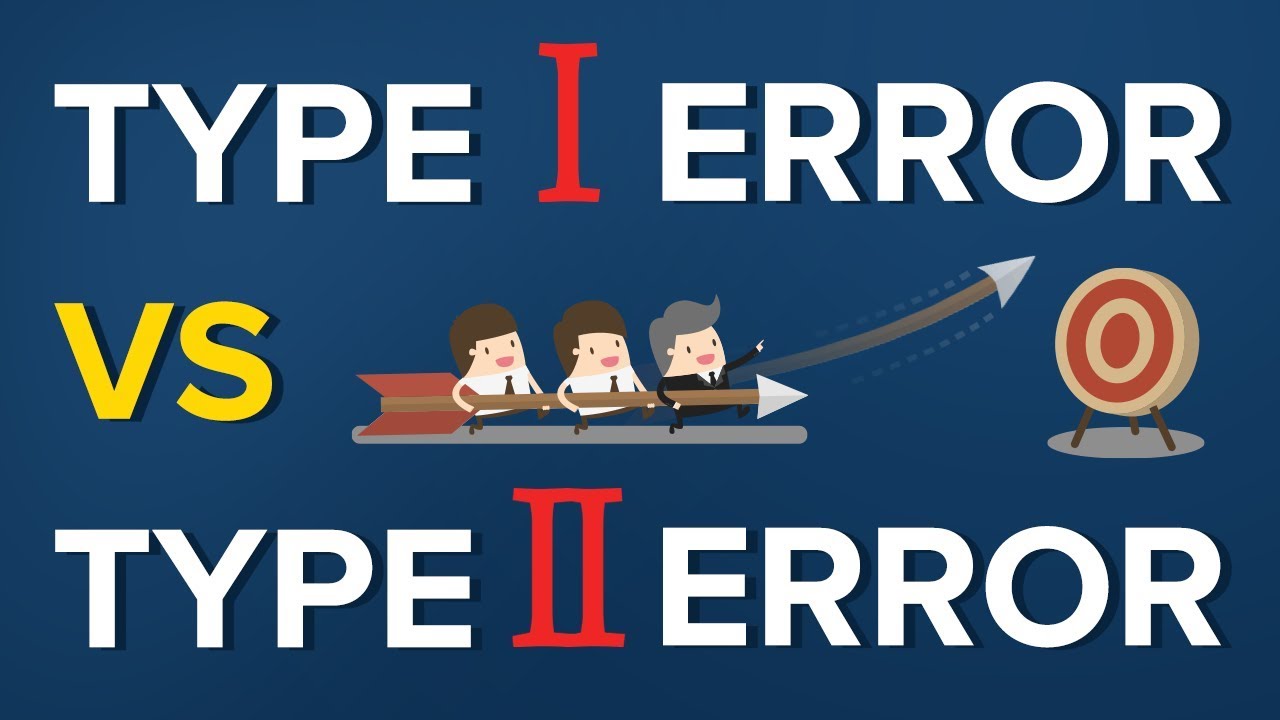
Type I error vs Type II error
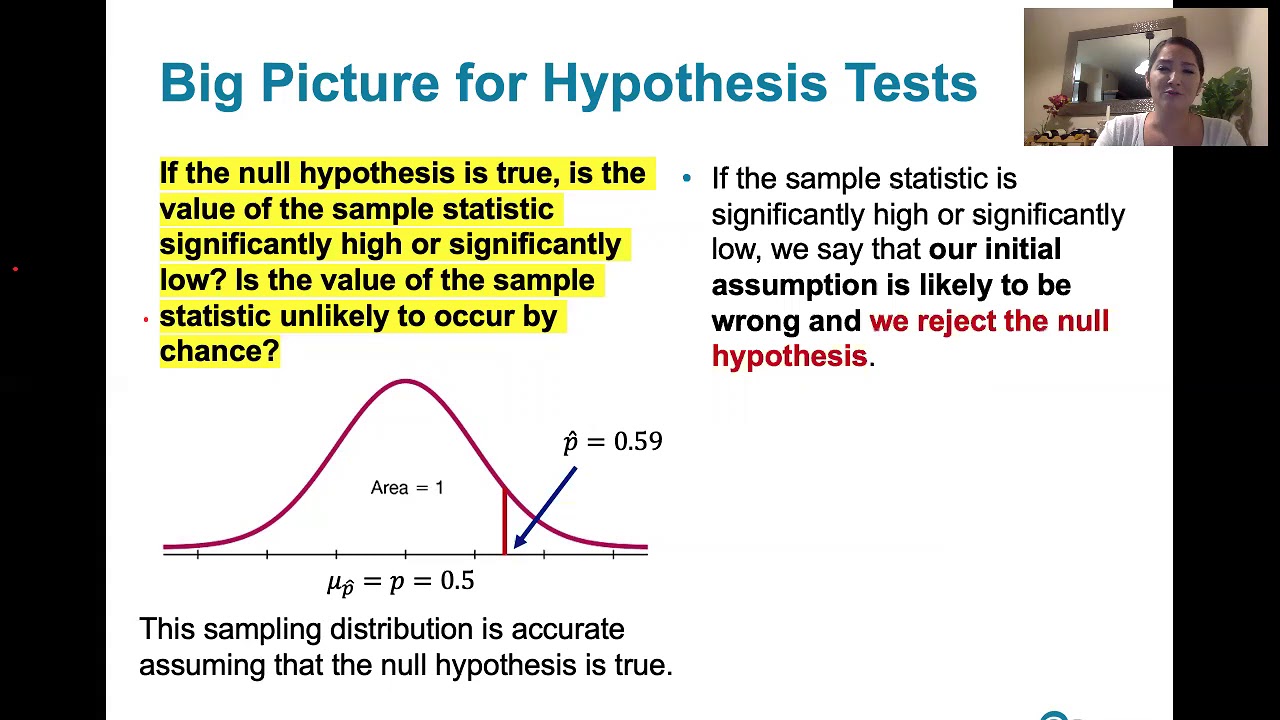
8.1.2 Basics of Hypothesis Testing - Significance Level, Type I and Type II Error
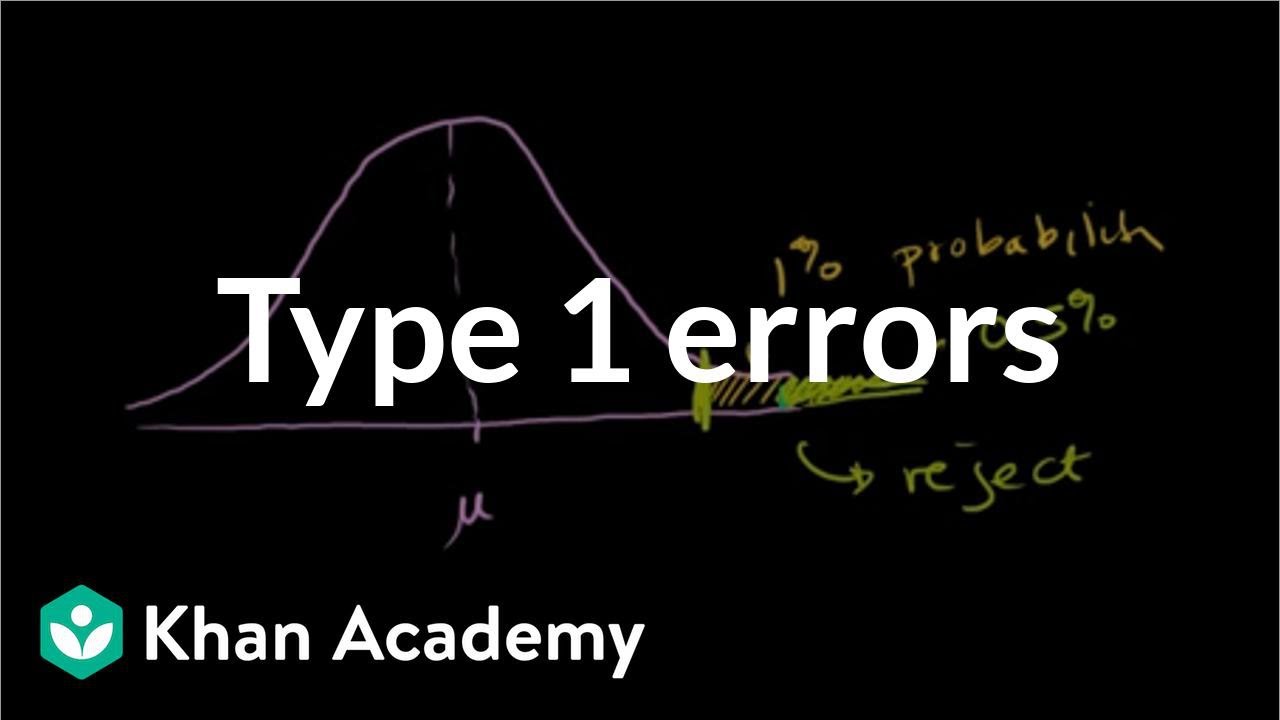
Type 1 errors | Inferential statistics | Probability and Statistics | Khan Academy
5.0 / 5 (0 votes)
Thanks for rating: