8.1.2 Basics of Hypothesis Testing - Significance Level, Type I and Type II Error
TLDRThis educational video script delves into hypothesis testing, focusing on significance levels and Type 1 and Type 2 errors. It explains the formal process of hypothesis testing, starting with null and alternative hypotheses, through computing sample statistics, and graphing the sampling distribution. The script clarifies the concepts of significance levels (alpha), the probability of making a Type 1 error (rejecting a true null hypothesis), and Type 2 error (failing to reject a false null hypothesis). It uses real-world analogies, like the criminal justice system, to illustrate these errors, emphasizing the importance of minimizing Type 1 errors in decision-making.
Takeaways
- ๐ Hypothesis testing, also known as tests of significance, is a formalized procedure to determine if sample results are significantly high or low compared to a claim.
- ๐ The process begins by formulating a null hypothesis and an alternative hypothesis based on a claim, which are used to test the validity of the claim.
- ๐ A sample statistic and its corresponding sample size are identified or computed, which are crucial for understanding the sampling distribution.
- ๐ The sampling distribution of the sample statistic is graphed assuming the null hypothesis is true, allowing for the visualization of how extreme the sample statistic is.
- ๐ฏ The significance of the sample statistic is determined by comparing it to a chosen significance level (alpha), which is the probability of rejecting a true null hypothesis.
- โ ๏ธ Type 1 error occurs when the null hypothesis is incorrectly rejected when it is actually true, with a probability equal to the significance level (alpha).
- โ ๏ธ Type 2 error happens when the null hypothesis is not rejected when it is actually false, with the probability of this error represented by beta.
- ๐ Critical values and p-values are used in hypothesis testing to make decisions about the null hypothesis, with the test statistic helping to determine if the sample statistic is significantly high or low.
- ๐ The conclusion of a hypothesis test should be stated in non-technical terms, relating back to the original claim and whether the evidence supports or refutes it.
- ๐ฎโโ๏ธ An example given in the script likens hypothesis testing to the legal system, where 'innocent until proven guilty' corresponds to a null hypothesis of innocence that is rejected if evidence of guilt is found.
- ๐ค The balance between Type 1 and Type 2 errors is often a consideration in hypothesis testing, with the aim to minimize one potentially leading to an increase in the other.
Q & A
What is the main focus of the video script?
-The video script focuses on explaining the significance level of hypothesis tests, particularly the concepts of Type 1 and Type 2 errors in the context of hypothesis testing.
What are hypothesis tests also known as?
-Hypothesis tests are also known as tests of significance.
What is the significance of the sample statistic in hypothesis testing?
-The sample statistic is significant in hypothesis testing because it helps determine whether the observed results are significantly high or low compared to what is expected under the null hypothesis.
What is the purpose of converting a sample statistic to a test statistic?
-Converting a sample statistic to a test statistic allows for the comparison of the sample statistic to a standard distribution, making it easier to determine if the sample statistic is significantly high or low.
What are the two methods used to make a decision about the null hypothesis based on the test statistic?
-The two methods are the critical value method, which compares the test statistic to critical values on the horizontal axis, and the p-value method, which deals with the probabilities associated with the test statistic.
What is the significance level (alpha) in hypothesis testing?
-The significance level (alpha) is the probability used as a cutoff for determining when sample evidence is considered significant enough to reject the null hypothesis. It represents the probability of making a Type 1 error.
What is a Type 1 error in the context of hypothesis testing?
-A Type 1 error occurs when the null hypothesis is incorrectly rejected when it is actually true. It is the mistake of concluding that there is an effect or difference when there is none.
What is a Type 2 error in the context of hypothesis testing?
-A Type 2 error occurs when the null hypothesis is not rejected when it is actually false. It is the mistake of failing to detect an effect or difference that actually exists.
How does the choice of significance level (alpha) affect the likelihood of making Type 1 and Type 2 errors?
-A lower significance level (alpha) reduces the likelihood of making a Type 1 error but increases the likelihood of making a Type 2 error. Conversely, a higher significance level increases the risk of a Type 1 error but reduces the risk of a Type 2 error.
Can you provide an example of how Type 1 and Type 2 errors relate to the legal system's 'innocent until proven guilty' principle?
-In the legal system, a Type 1 error would be convicting an innocent person (rejecting the true null hypothesis of innocence), while a Type 2 error would be acquitting a guilty person (failing to reject the false null hypothesis of innocence).
Why is it important to state conclusions in non-technical terms when reporting the results of hypothesis testing to a general audience?
-Stating conclusions in non-technical terms makes the results accessible and understandable to a broader audience, allowing them to grasp the implications of the findings without needing specialized knowledge of statistical terms or concepts.
Outlines
๐ Hypothesis Testing and Significance Level Overview
This paragraph introduces the concept of hypothesis testing, focusing on the significance level and the concept of Type 1 and Type 2 errors. It explains the goal of the video is to help viewers understand and identify these errors by the end. The speaker contextualizes hypothesis testing as a formalized procedure used across various fields and reviews the basic procedure, which includes stating a null and alternative hypothesis, computing a sample statistic, and determining its significance. The paragraph emphasizes the importance of graphing the sampling distribution and using it to assess whether the sample statistic is significantly high or low, which would lead to the rejection of the null hypothesis.
๐ Understanding Test Statistics and Hypothesis Testing Methods
The second paragraph delves deeper into the process of hypothesis testing, explaining the conversion of a sample statistic into a test statistic, which can be a z-score, t-score, or chi-squared value depending on the context. It discusses two methods for determining if the sample statistic is significantly high or low: the critical value method and the p-value method. The paragraph also touches on the concept of the critical region and how extreme values of test statistics correspond to small probabilities, which could lead to the rejection of the null hypothesis. Finally, it stresses the importance of stating conclusions in non-technical terms, relating back to the original claim.
๐ค The Concept of Significance and Errors in Hypothesis Testing
This paragraph explores the meaning of 'significantly high' or 'significantly low' in the context of hypothesis testing, quantifying these terms using probabilities and introducing the significance level (alpha). It explains that the significance level is the probability of making a Type 1 error, which is the mistake of rejecting a true null hypothesis. The speaker uses the example of a sample proportion to illustrate how one might decide to reject the null hypothesis based on an unlikely but still possible event occurring. The paragraph also introduces the concept of a Type 2 error, which is the mistake of failing to reject a false null hypothesis, represented by the probability beta.
๐ Visualizing Hypothesis Testing Outcomes and Errors
The fourth paragraph uses a visual approach to understand hypothesis testing outcomes and the potential for Type 1 and Type 2 errors. It presents a table that organizes the possible states of reality (null hypothesis being true or false) against the evidence's suggestions (suggesting the null is false or not). The paragraph explains the correct decisions of rejecting a false null or not rejecting a true null and contrasts them with the incorrect decisions that lead to Type 1 and Type 2 errors. It also provides hints to help keep track of these errors and emphasizes stating conclusions in terms of the original claim rather than the null hypothesis.
๐จโโ๏ธ Real-World Analogy: Hypothesis Testing and Legal System
In this paragraph, the concept of hypothesis testing is analogized to the legal system's 'innocent until proven guilty' principle. It explains how Type 1 and Type 2 errors relate to finding an innocent person guilty or failing to find a guilty person not guilty, respectively. The speaker emphasizes minimizing Type 1 errors in the legal system and discusses the implications of prioritizing one type of error over the other. The paragraph also provides an example of a medical procedure's effectiveness to illustrate how Type 1 and Type 2 errors might be described in a practical scenario.
๐ Describing Type 1 and Type 2 Errors in a Medical Context
The final paragraph provides a detailed example of Type 1 and Type 2 errors in the context of a medical procedure claimed to increase the likelihood of having a baby girl. It outlines the null and alternative hypotheses and describes the errors in terms of the original claim: a Type 1 error would be concluding the procedure works when it does not, while a Type 2 error would be concluding there is no effect when the procedure actually increases the likelihood. The paragraph reinforces the significance level's role in determining these errors and the importance of evidence in making such conclusions.
Mindmap
Keywords
๐กHypothesis Testing
๐กSignificance Level (alpha)
๐กType I Error
๐กType II Error
๐กNull Hypothesis
๐กAlternative Hypothesis
๐กSample Statistic
๐กSampling Distribution
๐กTest Statistic
๐กP-Value
๐กCritical Value
๐กNon-Technical Conclusion
Highlights
The video discusses learning outcome number two of lesson 8.1, focusing on the significance level of hypothesis tests and type 1 and type 2 errors.
Hypothesis testing is formalized in chapter eight as a unified procedure used in various fields including social science.
The basic procedure for hypothesis testing involves writing claims symbolically to infer null and alternative hypotheses.
Identifying or computing a sample statistic and its corresponding sample size is crucial for hypothesis testing.
Graphing the sampling distribution of the sample statistic under the assumption of the null hypothesis is key to hypothesis testing.
The decision to reject or not reject the null hypothesis is based on whether the sample statistic is significantly high or low.
The significance level (alpha) is the probability of making a type 1 error, which is the error of rejecting a true null hypothesis.
Type 2 error is the mistake of failing to reject a false null hypothesis, represented by the probability beta.
The video explains the concept of critical values and p-values as methods for determining the null hypothesis.
The critical region and extreme values of test statistics correspond to small probabilities, indicating potential type 1 errors.
The video emphasizes the importance of stating conclusions in non-technical terms, relating back to the original claim.
The significance level alpha is quantified using probabilities to determine what constitutes significantly high or low results.
The video provides a clear explanation of type 1 and type 2 errors using the analogy of the criminal justice system.
The balance between minimizing type 1 and type 2 errors is discussed, with a focus on the importance of not convicting innocent individuals.
The video concludes with an example of a medical procedure's effectiveness, illustrating how to frame type 1 and type 2 errors.
The significance level is tied to the probability of rejecting a true null hypothesis, which is a key concept in hypothesis testing.
The video prepares learners for the next topic of test statistics, which is essential for performing hypothesis tests.
Transcripts
Browse More Related Video
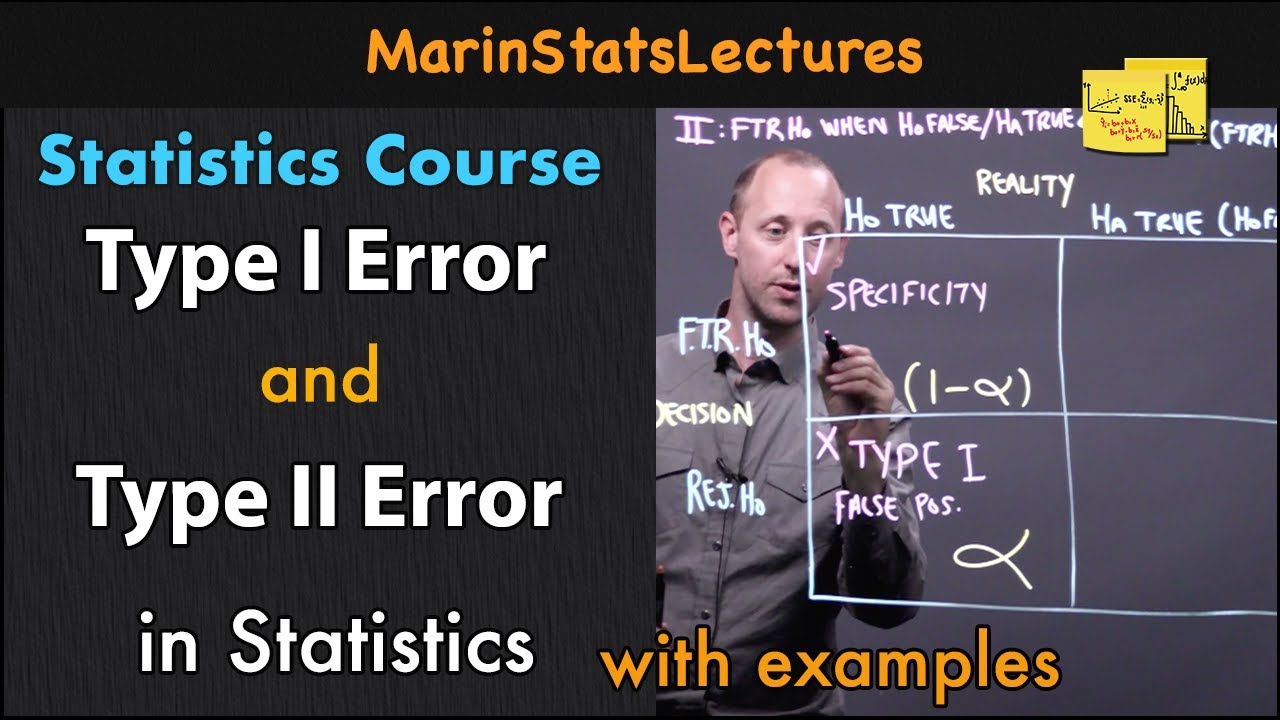
Errors and Power in Hypothesis Testing | Statistics Tutorial #16 | MarinStatsLectures

Type 1 (Alpha) vs. Type 2 (Beta) Error

How To Identify Type I and Type II Errors In Statistics
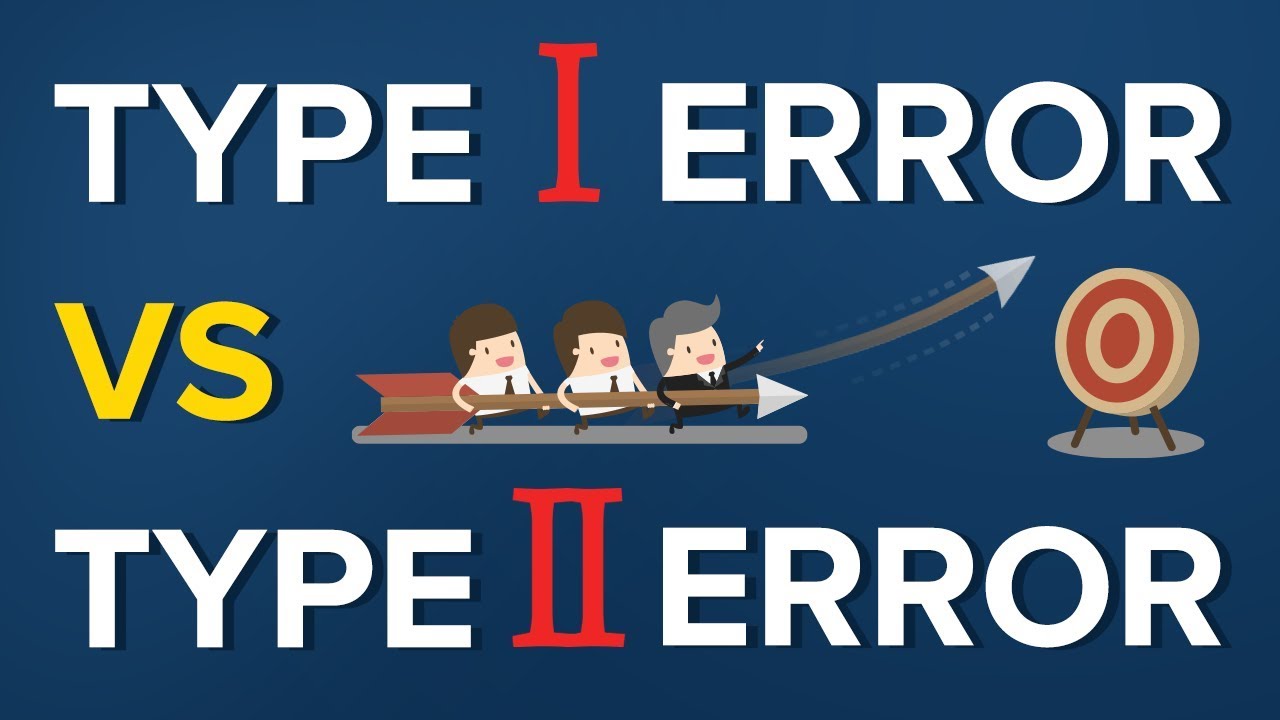
Type I error vs Type II error
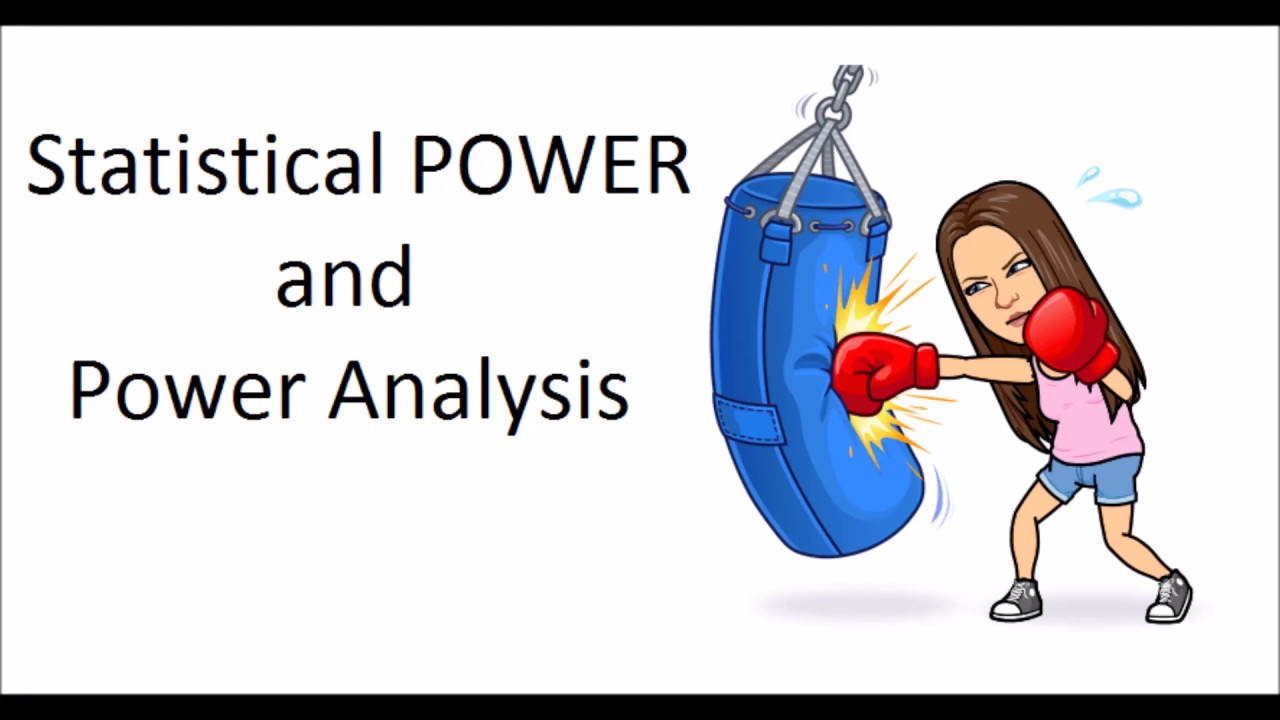
Statistical POWER and Power Analysis
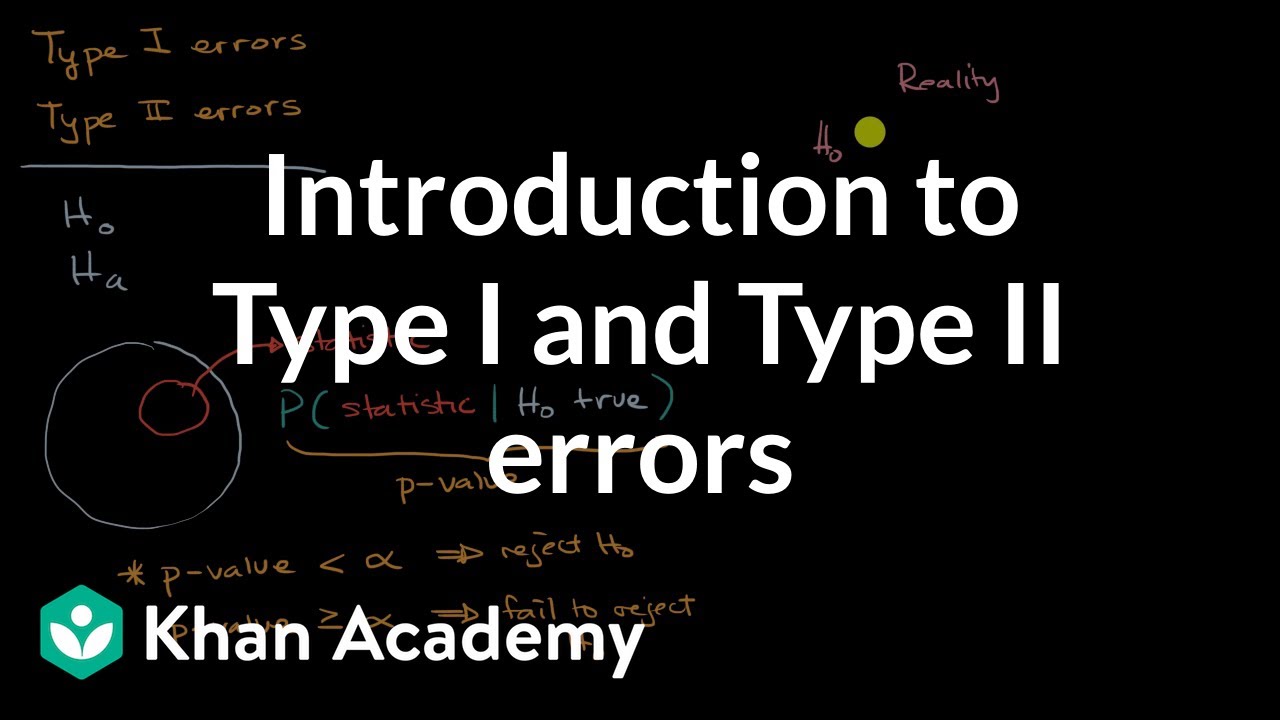
Introduction to Type I and Type II errors | AP Statistics | Khan Academy
5.0 / 5 (0 votes)
Thanks for rating: