Sampling Methods 101: Probability & Non-Probability Sampling Explained Simply
TLDRThis video script delves into the concept of sampling within research, explaining its importance and the various methods involved. It distinguishes between probability and non-probability sampling, highlighting popular techniques such as simple random sampling, stratified random sampling, cluster sampling, purposive sampling, convenience sampling, and snowball sampling. The script emphasizes the necessity of aligning the chosen sampling method with research aims and available resources, and acknowledges the trade-offs and limitations inherent in sampling strategies.
Takeaways
- π Sampling is the process of selecting a subset of participants from a larger group for research purposes.
- π― There are two main approaches to sampling: probability sampling (random) and non-probability sampling.
- π Probability sampling methods include simple random sampling, stratified random sampling, and cluster sampling, which are suitable for quantitative research aiming for generalizable findings.
- π Non-probability sampling methods such as purposive sampling, convenience sampling, and snowball sampling are more appropriate for qualitative research focusing on in-depth insights.
- π° The population is the entire group of interest, while the sample is a smaller, representative subset.
- π© Strata in stratified sampling are predefined subgroups that share a common trait, allowing for control over the impact of large subgroups.
- π Cluster sampling involves selecting participants from naturally occurring, mutually exclusive clusters within a population, which is practical for large geographic areas.
- π‘ When choosing a sampling method, consider your research aims, objectives, questions, and available resources.
- π Be prepared to make trade-offs in your sampling approach and clearly articulate the limitations of your chosen method.
- π Ensure that your sampling method aligns with your broader research methodology, whether it is quantitative or qualitative.
Q & A
What is the definition of sampling in a research context?
-Sampling in a research context refers to the process of selecting a subset of participants from a larger group, known as the population, to study and draw conclusions about the whole group based on the findings from the smaller group, called the sample.
What are the two main approaches to sampling?
-The two main approaches to sampling are probability sampling (random sampling) and non-probability sampling. Probability sampling involves selecting participants on a statistically random basis, while non-probability sampling involves participant selection based on the researcher's discretion and judgment.
Why is it important for a sample to be representative of the population?
-A representative sample is important because it allows researchers to generalize their findings to the entire population. This means that the sample should reflect every layer of the population in exact proportion, providing accurate and reliable insights that can be applied to the larger group.
What are the advantages and disadvantages of simple random sampling?
-Simple random sampling is easy to implement, typically quite cost-effective, and can yield fairly reliable generalizations due to its completely random selection process. However, it may not account for the impact of large subgroups within the data, potentially leading to underrepresentation of minority subgroups.
How does stratified random sampling differ from simple random sampling?
-Stratified random sampling involves selecting participants randomly but from within certain predefined subgroups (strata) that share a common trait, such as gender, ethnicity, or level of education. This method provides more control over the impact of large subgroups within the population, allowing for the identification of differences between subgroups.
What is cluster sampling and how does it compare to stratified random sampling?
-Cluster sampling involves sampling from naturally occurring, mutually exclusive clusters within a population, such as area codes within a city. Unlike stratified random sampling, which requires data collection across a broader geographic area, cluster sampling focuses on a smaller number of defined clusters, making it more practical and economical, especially for populations spread over large geographic areas.
What are three popular non-probability-based sampling methods?
-Three popular non-probability-based sampling methods are purposive sampling, convenience sampling, and snowball sampling. Purposive sampling relies on the researcher's judgment to select participants based on the study's purpose. Convenience sampling selects participants based on their availability or accessibility. Snowball sampling uses referrals from initial participants to recruit additional participants.
How does snowball sampling work and when is it typically used?
-Snowball sampling relies on referrals from initial participants to recruit additional participants, creating a sample that grows like a rolling snowball. It is often used when it is difficult to identify and access a particular population, such as people with rare medical conditions or members of exclusive groups, and is particularly useful when the research topic is sensitive or taboo.
What factors should be considered when choosing a sampling method for a research project?
-When choosing a sampling method, researchers should consider their research aims and objectives, as well as their resources and practical constraints. The alignment of the sampling method with the research methodology and the need for generalizable findings or rich, deep insights should guide the choice between probability-based and non-probability-based sampling methods.
How can researchers ensure they are on the right track with their sampling choices?
-Researchers can ensure they are on the right track by clearly explaining and justifying their sampling choices and by articulating the limitations of their approach. Acknowledging the limitations and making trade-offs when necessary is part of the research process, and as long as these aspects are transparently discussed, the study's integrity will be maintained.
What are the key takeaways from the video script about sampling?
-The key takeaways include understanding that sampling is selecting a subgroup from a larger population, recognizing the two main approaches to sampling (probability and non-probability), being aware of popular sampling methods under each approach, and knowing how to choose the right sampling method based on research aims, objectives, questions, and practical constraints.
Outlines
π Introduction to Sampling in Research
This paragraph introduces the concept of sampling within a research context, explaining it as the process of selecting a subset of participants from a larger group, known as the population. It emphasizes the importance of having a representative sample to generalize findings to the entire population. The video also mentions the provision of free dissertation templates for research projects and touches on the challenges of achieving a truly representative sample due to practical obstacles.
π― Probability vs. Non-Probability Sampling
This section delves into the two main approaches to sampling: probability sampling and non-probability sampling. Probability sampling, also known as random sampling, involves selecting participants based on a statistically random basis, making it suitable for quantitative research aiming for generalizable findings. On the other hand, non-probability sampling allows for researcher discretion in participant selection and is often used in qualitative research where the depth of data is prioritized over generalizability.
π’ Exploring Probability-Based Sampling Methods
The paragraph discusses three common probability-based sampling methods: simple random sampling, stratified random sampling, and cluster sampling. Simple random sampling is akin to a lottery draw, where each participant has an equal chance of being selected. Stratified random sampling involves selecting participants from predefined subgroups to ensure representation of specific traits. Cluster sampling focuses on sampling from naturally occurring, exclusive groups within the population, which is practical for large or geographically dispersed populations.
π Non-Probability Sampling: Purpose, Convenience, and Snowball
This part examines three non-probability sampling methods: purposive (judgment) sampling, convenience sampling, and snowball sampling. Purposive sampling relies on the researcher's judgment to select participants based on the study's aims. Convenience sampling chooses participants based on their accessibility to the researcher. Snowball sampling uses referrals from initial participants to recruit more participants, which is useful for hard-to-reach populations or sensitive topics.
π Choosing the Right Sampling Method
The final paragraph provides guidance on selecting the appropriate sampling method for a research project. It emphasizes the importance of aligning the sampling method with the research aims and available resources. The paragraph also acknowledges that trade-offs may be necessary and encourages researchers to clearly articulate the limitations of their chosen sampling approach.
Mindmap
Keywords
π‘Sampling
π‘Population
π‘Probability Sampling
π‘Non-Probability Sampling
π‘Simple Random Sampling
π‘Stratified Random Sampling
π‘Cluster Sampling
π‘Purposive Sampling
π‘Convenience Sampling
π‘Snowball Sampling
π‘Research Aims
Highlights
Exploring the jargon-filled world of sampling and sampling methods.
Defining sampling as the process of selecting a subset of participants from a larger group.
Describing the larger group as the population and the engaged subset as the sample.
Discussing the ideal of a sample being perfectly representative of the population for generalization.
Introducing the two high-level approaches to sampling: probability and non-probability sampling.
Explaining probability sampling as random selection based on a predetermined process.
Mentioning that probability sampling is commonly used in quantitative research for generalizable findings.
Describing non-probability sampling where participant selection is based on researcher discretion.
Highlighting that non-probability sampling is used in qualitative research for rich, in-depth data.
Introducing three popular probability-based sampling methods: simple random, stratified random, and cluster sampling.
Explaining simple random sampling as selecting participants with equal chance, like drawing names from a hat.
Discussing stratified random sampling, which involves selecting from predefined subgroups (strata).
Describing cluster sampling, which involves selecting from naturally occurring, mutually exclusive clusters within a population.
Presenting three non-probability-based sampling methods: purposive, convenience, and snowball sampling.
Explaining purposive sampling where participants are selected based on researcher judgment and study aims.
Detailing convenience sampling, where participants are chosen based on their availability and accessibility.
Discussing snowball sampling, which relies on referrals from initial participants to recruit more participants.
Guiding on choosing the right sampling method by considering research aims, objectives, questions, and resources.
Recapping the importance of aligning the sampling approach with research aims and considering practical constraints.
Transcripts
Browse More Related Video
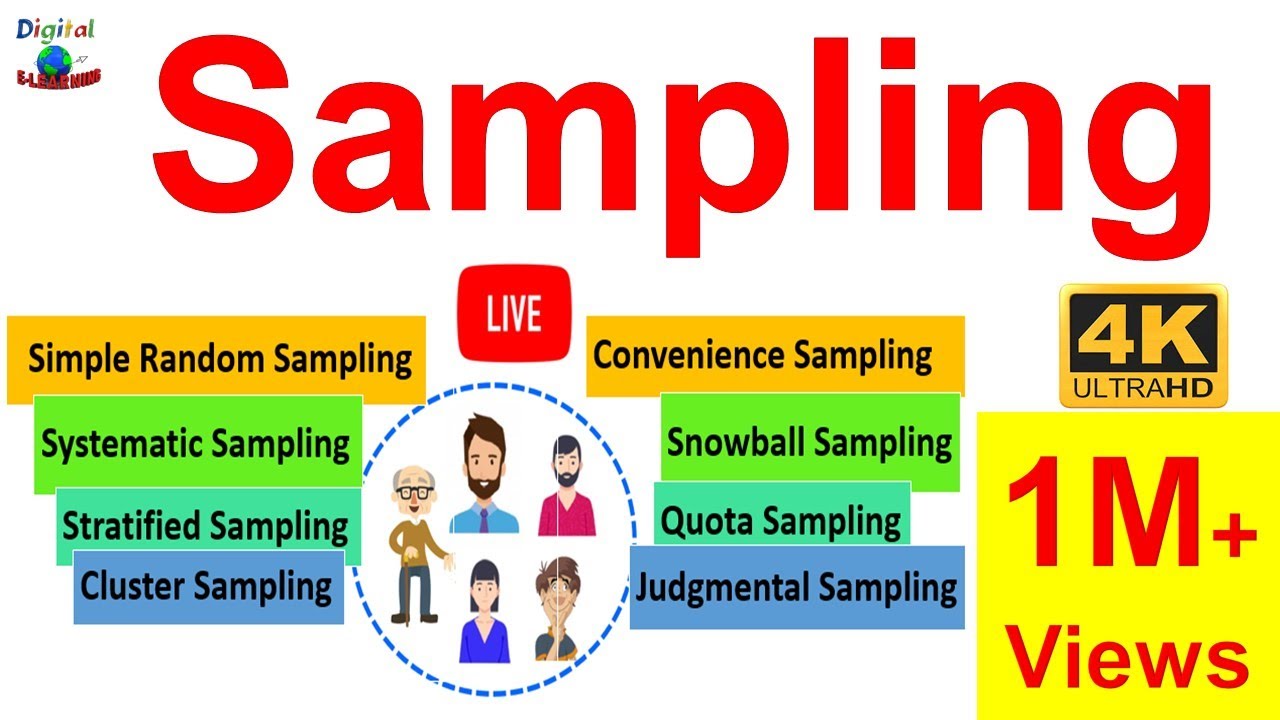
Sampling: Sampling & its Types | Simple Random, Convenience, Systematic, Cluster, Stratified
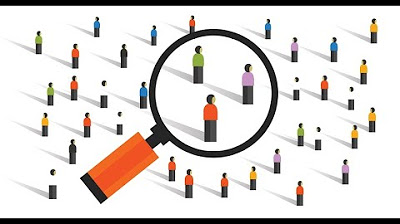
Probability and Non-Probability Sampling in Research Methods
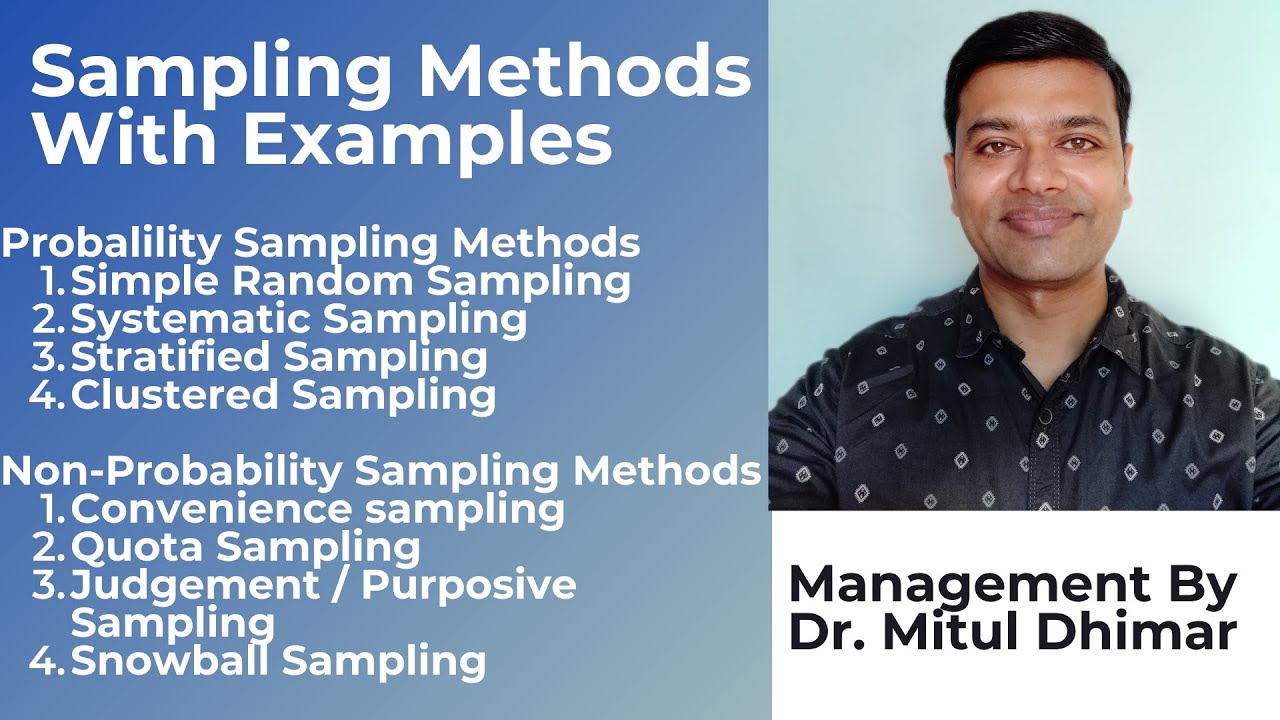
Types of sampling methods with examples / sampling techniques (8)
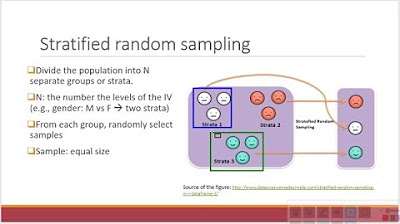
Research Methods 1: Sampling Techniques
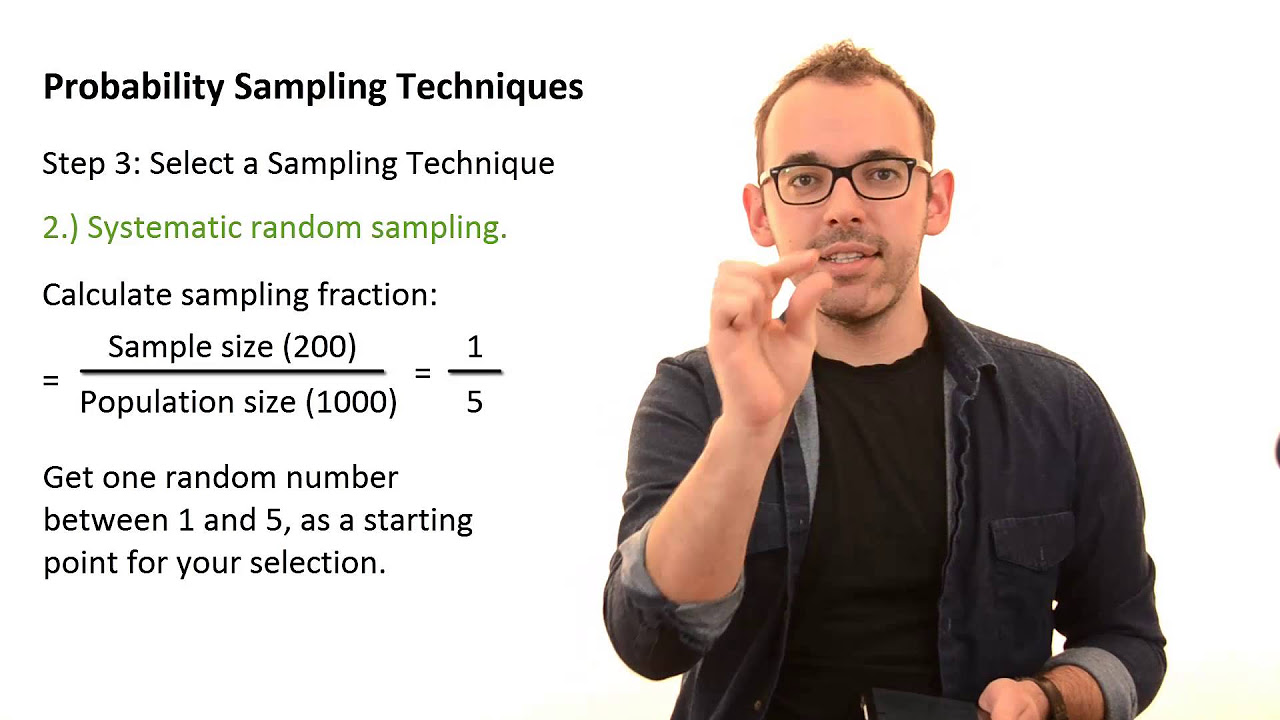
4.2 Probability Sampling Techniques
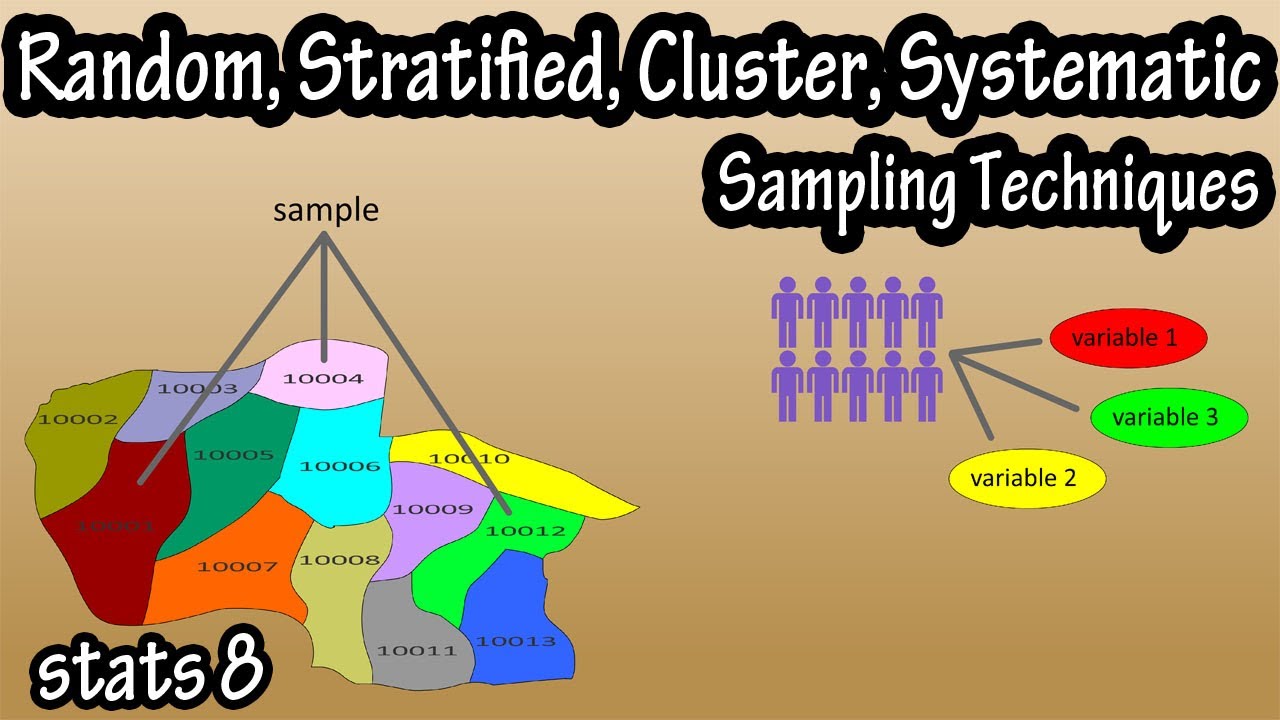
What Are The Types Of Sampling Techniques In Statistics - Random, Stratified, Cluster, Systematic
5.0 / 5 (0 votes)
Thanks for rating: