Probability and Non-Probability Sampling in Research Methods
TLDRThis transcript introduces various sampling methods, emphasizing the importance of representative sampling for research accuracy. It distinguishes between probability and non-probability sampling, detailing advantages and disadvantages of each approach. The process of sampling is broken down into steps, from defining the population to reviewing the sampling process. The transcript also discusses specific sampling techniques such as simple random sampling, systematic sampling, stratified sampling, cluster sampling, and multistage sampling, highlighting their applications and potential biases. Additionally, it touches on nonprobability sampling methods like convenience, judgment, and quota sampling, and panel sampling for longitudinal studies.
Takeaways
- π Understanding the purpose of sampling is crucial; it helps us make informed decisions when resources like time, money, and workforce are limited.
- π’ Sampling allows for results with known accuracy that can be calculated through statistical means, providing a feasible approach to research within constraints.
- π A sampling frame, which is the list from which potential respondents are drawn, is essential and must be representative of the population of interest.
- π The representative nature of a sample is influenced by three factors: the sampling procedure, sample size, and participation or response rate.
- π When sampling, it's important to consider the target population, the actual study population, and the sampling frame to ensure a representative sample.
- π― Probability sampling includes methods like simple random, systematic, stratified, multistage, and cluster sampling, each with its own advantages and disadvantages.
- π² Nonprobability sampling, such as convenience, purposive, and quota sampling, is based on certain assumptions about the population and does not guarantee equal selection chances.
- π In simple random sampling, every member of the population has an equal chance of being selected, making it an equal probability of selection (EPS) design.
- π Systematic sampling involves selecting elements at regular intervals from an ordered list, but the starting point is randomly chosen to ensure equal probability of selection.
- π½ Stratified sampling divides the population into distinct categories or strata, allowing for more precise estimates when certain variables are of interest, but it can be complex and sometimes requires larger samples.
- π Cluster sampling and multistage sampling are methods that can reduce costs and administrative efforts, especially when dealing with large populations or geographically dispersed samples.
Q & A
What are the main objectives of the unit on sampling methods?
-The main objectives of the unit are to learn the reasons for sampling, develop an understanding of different sampling methods, distinguish between probability and non-probability sampling, and understand the relative advantages and disadvantages of each approach.
Why do we use sampling instead of surveying the entire population?
-Sampling is used due to limitations in resources such as time, money, and workload, as well as practicality. It provides results with known accuracy that can be calculated through statistical means, and is more feasible when dealing with large populations.
What is a sampling frame and why is it important?
-A sampling frame is the list from which potential respondents are drawn. It is important because it must be representative of the overall population to ensure that all elements of the population of interest are included in the sample.
What are the three factors that influence a sample's representative nature?
-The three factors that influence a sample's representative nature are the sampling procedure, the sample size, and the participation or response rate.
In what situations would you sample the entire population?
-You would sample the entire population when the population is small, when you have ample resources and money to conduct research, and when a high response rate is expected or not a major concern.
What is the difference between probability and non-probability sampling?
-Probability sampling involves every unit in the population having a chance of being selected, with the possibility of equal probability of selection (EPS). Non-probability sampling includes elements that have no chance of being selected and is based on certain assumptions regarding the population of interest.
What are some advantages and disadvantages of simple random sampling?
-Advantages of simple random sampling include ease of calculation for estimates and it being an EPS design. Disadvantages may include impracticality if the sampling frame is large, potential absence of minority subgroups in the study, and possible need for replacement of selected units.
How does systematic sampling differ from simple random sampling?
-Systematic sampling involves arranging the target population in an order and selecting elements at regular intervals. Unlike simple random sampling, different subsets of the same size in systematic sampling do not have the same selection probabilities, which can introduce bias.
What is stratified sampling and what are its potential drawbacks?
-Stratified sampling involves dividing a population into distinct categories or strata and sampling each stratum independently. Drawbacks include the need to prepare a separate sampling frame for each stratum, potential complexity from relating stratifying variables to multiple criteria, and sometimes requiring larger sample sizes than other methods.
How does cluster sampling work and what are its advantages and disadvantages?
-Cluster sampling involves selecting a sample of areas or clusters first, and then selecting a sample of respondents within those clusters. Advantages include reduced costs in preparing a sampling frame and lower travel and administrative expenses. Disadvantages may include higher sampling error compared to a simple random sample of the same size and the requirement to identify clusters beforehand.
What is multistage sampling and when is it typically used?
-Multistage sampling is a complex form of cluster sampling where multiple levels of units are embedded within each other. It is typically used when a complete list of all population members does not exist or is inappropriate, and helps to avoid large costs associated with traditional cluster samples by taking random samples of preceding random samples.
Outlines
π Introduction to Sampling Methods
This paragraph introduces the unit on sampling methods, outlining the learning objectives which include understanding the reasons for sampling, different sampling methods, and their respective advantages and disadvantages. It emphasizes the importance of a sample as a smaller representation of a larger population and discusses factors influencing a sample's representative nature, such as the sampling procedure, sample size, and participation rate. The paragraph also touches on when it might be appropriate to sample the entire population and the process of breaking down a sample, considering the target population, study population, and sampling frame.
π― Types of Probability and Non-Probability Samples
This paragraph delves into the various types of probability samples, including simple random, systematic random, stratified random, multistage, and cluster samples. It explains that probability sampling involves every unit in the population having a chance of being selected. The paragraph then contrasts these with non-probability samples, which may be based on assumptions or convenience and include accidental, quota, and purposive sampling. It also discusses non-response effects that can turn a probability design into a non-probability one and the importance of understanding these effects.
π’ Simple Random and Systematic Sampling
The paragraph discusses simple random sampling as a method applicable to small, homogeneous populations that are easily accessible. It describes the process of assigning numbers to each unit and using a random number table or lottery system to select units. The paragraph also covers systematic sampling, which involves arranging the population according to an ordering scheme and selecting elements at regular intervals. Advantages and disadvantages of both methods are discussed, including practicality, potential biases, and the challenges of assessing precision of estimates.
π Stratified and Cluster Sampling
This section explains stratified sampling, where a population is divided into distinct categories or strata, and each stratum is sampled independently. It highlights the need for a sampling frame prepared for each stratum and potential drawbacks, such as complexity in design and potentially larger sample requirements. The paragraph then introduces cluster sampling, which involves selecting a sample of areas or clusters and then sampling respondents within those clusters. It discusses the cost-effectiveness of this method and its advantages over simple random sampling in certain contexts, as well as the differences between strata and clusters.
π Multistage and Matched Random Sampling
The paragraph describes multistage sampling as a complex form of cluster sampling where multiple levels of units are involved. It explains the process of selecting districts, villages, and houses as examples of units at different stages. Matched random sampling is also discussed, where participants are first matched on a characteristic and then assigned to groups randomly. This method is useful in various contexts, such as studying the effects of interventions or training. The paragraph concludes with a mention of quota sampling, which segments the population and uses judgment to select subjects based on specified proportions.
π Conclusion and Final Sampling Methods
In conclusion, the paragraph summarizes the discussion on probable and non-probable sampling plans, including the various types of sampling frames and methods. It reiterates the importance of understanding these methods for effective research and data collection. The speaker expresses a hope that the audience has found the presentation informative and looks forward to further discussions.
Mindmap
Keywords
π‘Sampling
π‘Probability Sampling
π‘Non-Probability Sampling
π‘Sampling Frame
π‘Sample Size
π‘Response Rate
π‘Stratified Sampling
π‘Cluster Sampling
π‘Multistage Sampling
π‘Quota Sampling
π‘Panel Sampling
Highlights
Exploring the reasons for sampling, which include resource limitations and the ability to determine truths about a larger population through a smaller sample.
Differentiating between probability and non-probability sampling methods, and discussing their respective advantages and disadvantages.
The importance of a sampling frame in ensuring accurate representation of the population of interest and avoiding sampling frame errors.
Three factors influencing a sample's representative nature: sampling procedure, sample size, and participation or response rate.
When to sample the entire population, such as in cases of small populations, abundant resources, and low expected response rates.
The process of transitioning from a theoretical population to the actual study population and then to the sampling frame.
Types of probability samples including simple random, systematic, stratified, multistage, multi-phase, and cluster samples.
Characteristics of probability sampling where every unit in the population has a chance of being selected, known as equal probability of selection (EPS).
Nonprobability samples involve selection based on assumptions regarding the population of interest, such as convenience, purposive, and quota-based samples.
The impact of non-response effects on probability designs, potentially turning them into non-probability designs if not well understood.
Simple random sampling is applicable for small, homogeneous populations and is an EPS design, but may be impractical for large sampling frames.
Systematic sampling involves selecting elements at regular intervals from an ordered list, with the starting point chosen randomly.
Stratified sampling organizes a population into distinct categories or strata, with each unit in a stratum having an equal chance of selection.
Cluster sampling involves selecting a sample of areas or clusters and then sampling respondents within those clusters, reducing costs and administrative efforts.
Multistage sampling is a complex form involving multiple levels of units and is used when a complete list of population members does not exist.
Matched random sampling assigns participants to groups by matching them on a characteristic and then randomly assigning them to specific groups.
Quota sampling segments the population into mutually exclusive subgroups and uses judgment to select subjects from each based on specified proportions.
Convenient sampling, also known as opportunity sampling, involves selecting a sample based on what is readily available and convenient, but may not be representative.
Panel sampling involves selecting a group of participants through random sampling and then repeatedly surveying them over time to gauge changes in the population.
Transcripts
Browse More Related Video
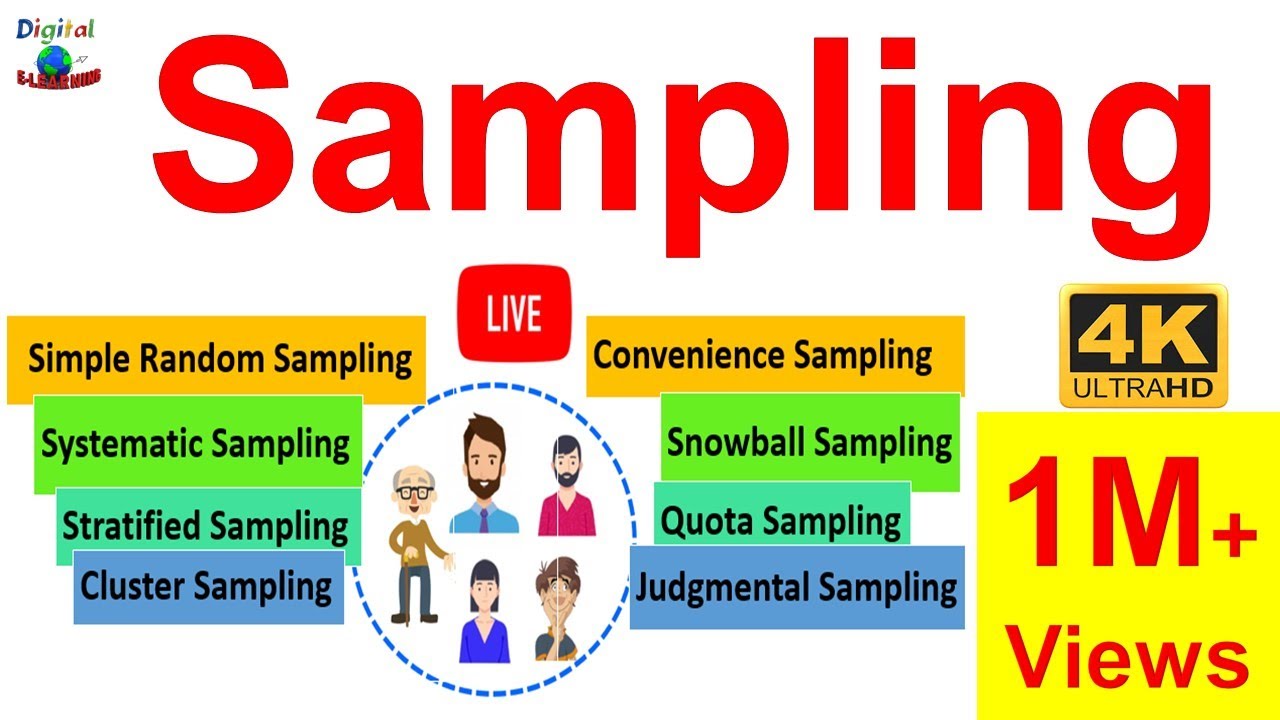
Sampling: Sampling & its Types | Simple Random, Convenience, Systematic, Cluster, Stratified
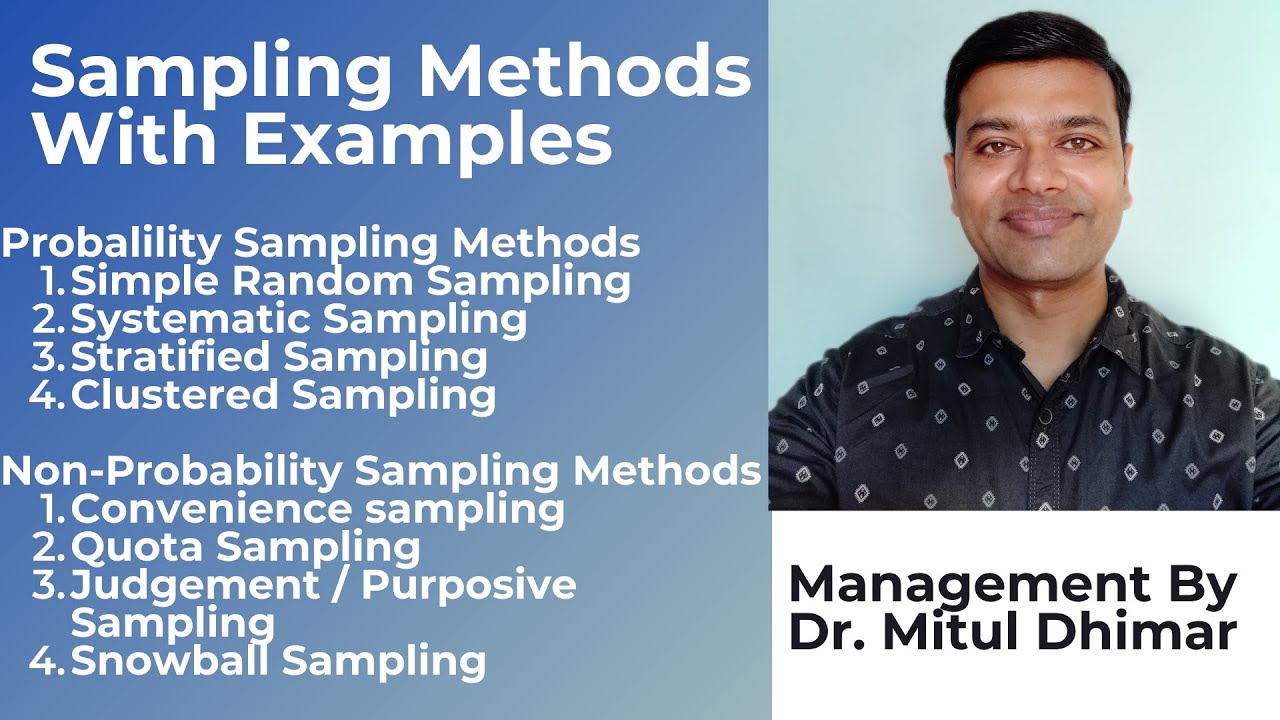
Types of sampling methods with examples / sampling techniques (8)
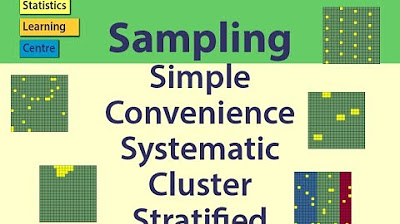
Sampling: Simple Random, Convenience, systematic, cluster, stratified - Statistics Help
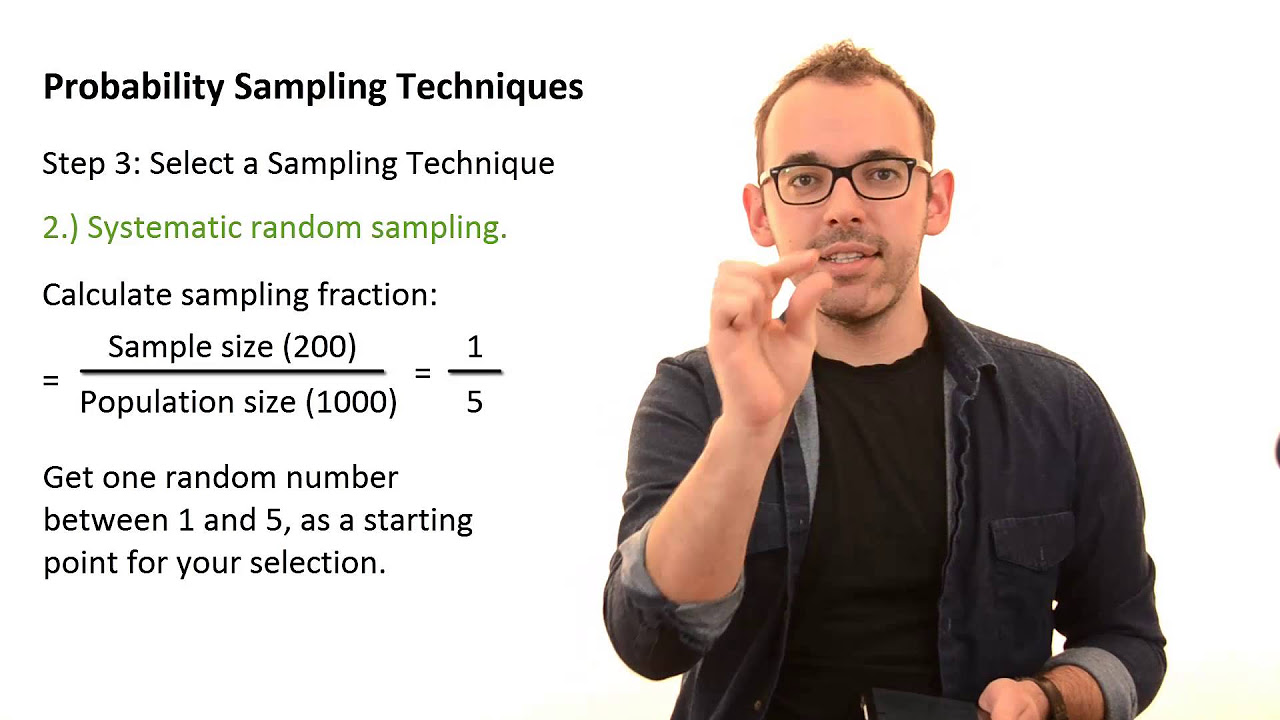
4.2 Probability Sampling Techniques
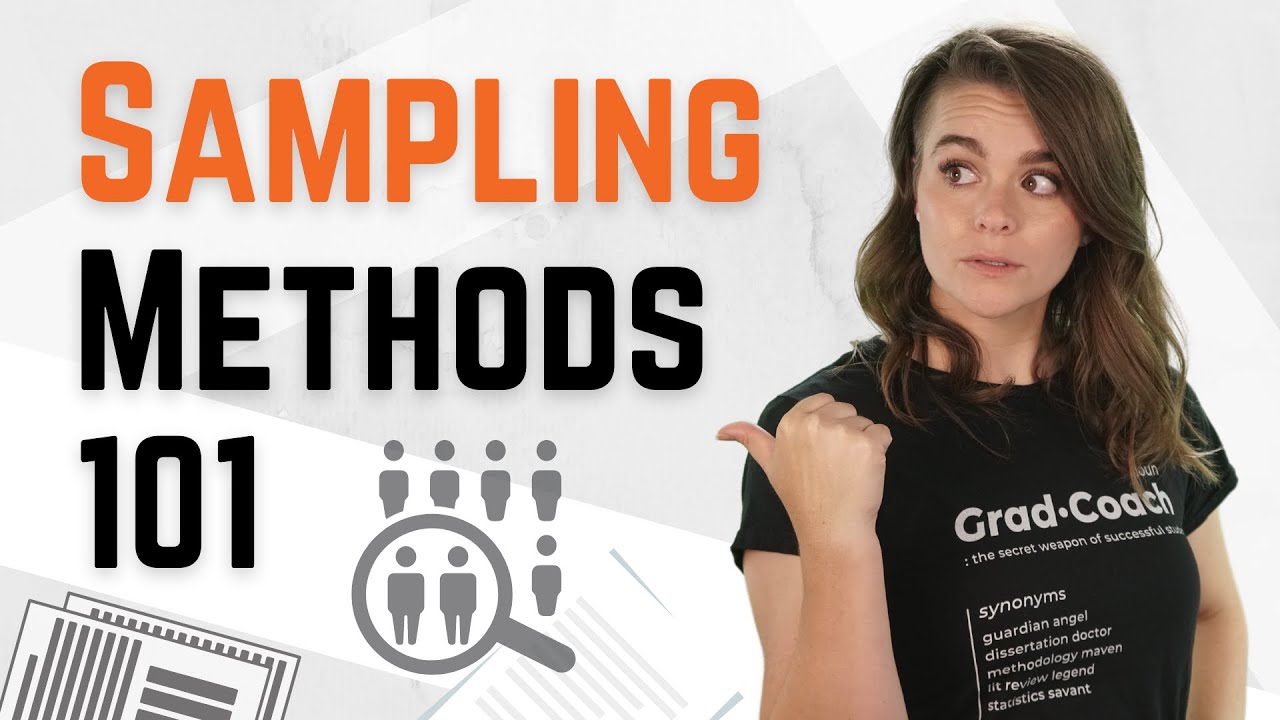
Sampling Methods 101: Probability & Non-Probability Sampling Explained Simply

Statistics Lecture 1.5: Sampling Techniques. How to Develop a Random Sample
5.0 / 5 (0 votes)
Thanks for rating: