What Are The Types Of Sampling Techniques In Statistics - Random, Stratified, Cluster, Systematic
TLDRThis informative video script discusses four primary sampling techniques used in research to obtain unbiased data from a population: random sampling, stratified sampling, cluster sampling, and systematic sampling. Each method is explained with practical examples, highlighting their applications and potential limitations. The script also touches on the concept of sampling error and the convenience sample, which may lead to biased results.
Takeaways
- π Samples are used to gather data about a population, focusing on a variable or variables.
- π― There are four main unbiased sampling techniques: random, stratified, cluster, and systematic sampling.
- π‘ In random sampling, every member of the population has an equal chance of being selected.
- π’ For stratified sampling, the population is divided into subgroups, with a random sample taken from each subgroup.
- π’ In cluster sampling, the population is divided into clusters, and a random selection of clusters is used for the sample.
- π Systematic sampling involves assigning a number to the population and selecting members at regular intervals.
- π Cluster sampling can be efficient and cost-effective, but may not always represent the population accurately.
- π The main difference between stratified and cluster sampling is that stratified subgroups have similar characteristics, while clusters in a cluster sample vary.
- π Sampling error can occur even with the best methods, representing the difference between sample results and the population.
- π« Convenience sampling, though easy to conduct, often leads to biased results due to the selection of easily accessible subjects.
Q & A
What is the purpose of using samples in research?
-The purpose of using samples in research is to collect data and information about a variable or variables from a larger population. This allows researchers to make inferences about the entire population without having to study every single member.
What are the four main sampling techniques mentioned in the transcript?
-The four main sampling techniques mentioned are random sampling, stratified sampling, cluster sampling, and systematic sampling.
How is a random sample achieved?
-A random sample is achieved by ensuring that every member of the population has an equal chance of being selected. This can be done by numbering each member of the population and selecting cards at random, using a calculator or computer to generate random numbers, or using a random number table.
What is the difference between stratified sampling and cluster sampling?
-Stratified sampling involves dividing the population into subgroups with similar characteristics and taking a random sample from each subgroup. Cluster sampling, on the other hand, involves dividing the population into sections or clusters and selecting one or more clusters randomly, then using all members of the chosen cluster as the sample. The key difference is that stratified samples ensure homogeneity within subgroups, while cluster samples accept the variability within clusters.
In what situations might a cluster sample be particularly useful?
-A cluster sample might be particularly useful when dealing with a large population or a large geographic area. It can be an efficient and cost-effective method when it is impractical or too costly to survey every single member of the population, such as in the case of surveying small business owners across a very populated city.
How does systematic sampling work?
-Systematic sampling involves assigning a counting number to each member of the population, selecting a random starting number, and then selecting members for the sample at regular intervals from the starting number. For example, if you wanted a sample of 50 from a population of 1,000, you would number the units from one to a thousand, generate a random starting number, and then select every 20th unit after the starting number.
What is a potential drawback of using a cluster sample?
-A potential drawback of using a cluster sample is that the chosen clusters may not be representative of the entire population. This can lead to sampling bias and affect the accuracy of the research findings.
What is a convenience sample, and why can it lead to biased results?
-A convenience sample is a sample developed from members of the population that are easy to get or convenient for the researcher. This method can lead to biased results because it does not ensure that the sample is representative of the larger population, potentially skewing the findings towards those who are more accessible.
What is sampling error and how can it occur even with the best sampling methods?
-Sampling error is the difference between the results obtained from a sample and the true results of the entire population. It can occur even with the best sampling methods because no sample can perfectly represent the entire population. The goal is to minimize this error by using appropriate sampling techniques and ensuring that the sample is as representative as possible of the population.
Why is it important to use unbiased sampling techniques in research?
-Using unbiased sampling techniques is crucial in research to ensure the validity and reliability of the findings. Biased samples can lead to incorrect conclusions and misinterpretations of the data, which can have significant implications for decision-making and policy development based on the research outcomes.
How can researchers ensure that their samples are representative of the population?
-Researchers can ensure that their samples are representative of the population by using appropriate sampling techniques such as random sampling, stratified sampling, or systematic sampling. They should also carefully consider the population and the research question to select the most suitable sampling method that will yield a representative sample.
Outlines
π Introduction to Sampling Techniques
This paragraph introduces the concept of a sample as a part of a larger population and explains the importance of using unbiased sampling techniques to collect data. It outlines four main sampling methods: random sampling, stratified sampling, cluster sampling, and systematic sampling. The paragraph emphasizes the goal of obtaining representative samples to better understand the population of interest.
π― Random Sampling Method
The paragraph discusses the random sampling method, where every member of the population has an equal chance of being selected. It provides examples of how this can be achieved, such as drawing numbered cards from a container or using a calculator, computer, or random number table to generate selections. The goal is to ensure that the sample is unbiased and reflective of the entire population.
π Stratified Sampling Method
This part explains the stratified sampling method, where the population is divided into subgroups based on certain characteristics, and a random sample is taken from each subgroup. The example given is of age-based subgroups to understand savings behavior. The paragraph highlights the importance of having representative segments and the potential for this method to provide more accurate results than random sampling alone.
π Cluster Sampling Method
The cluster sampling method is described, where the population is divided into clusters or sections, and a random selection of these clusters is made, with all members of the chosen clusters included in the sample. The example of surveying small business owners across different zip codes in a large city illustrates how this method can be cost-effective and efficient, especially for large or geographically dispersed populations. However, it also notes the potential for unrepresentative clusters leading to sampling errors.
π Systematic Sampling Method
This paragraph details the systematic sampling method, where the population is numbered and a random starting number is chosen. Members of the sample are then selected at regular intervals from this starting number. The example provided involves surveying residents of a singles apartment complex about their Netflix watching habits. The paragraph notes that this method is straightforward when the population can be easily numbered, but also mentions the possibility of sampling errors.
π Convenience Sampling and Conclusion
The final part of the paragraph discusses convenience sampling, where researchers select participants that are easy to access, potentially leading to biased results. It also mentions that despite using the best sampling methods, sampling errors can still occur. The paragraph concludes with a reminder that there are more videos available for further learning and signs off with a friendly farewell until the next video.
Mindmap
Keywords
π‘sample
π‘population
π‘random sampling
π‘stratified sampling
π‘cluster sampling
π‘systematic sampling
π‘sampling error
π‘convenience sample
π‘bias
π‘representative
Highlights
A sample is part of a population used to collect data and information about variables.
Four main sampling techniques are discussed: random sampling, stratified sampling, cluster sampling, and systematic sampling.
Random sampling ensures every member of the population has an equal chance of being selected.
Stratified sampling involves dividing the population into subgroups and taking a random sample from each.
Cluster sampling selects whole groups or clusters from the population rather than individuals.
Systematic sampling involves selecting members at regular intervals from a randomly chosen starting point.
Sampling error can occur even with the best sampling methods, representing a difference between sample and population results.
Convenience sampling may lead to biased results as it involves selecting easily accessible members of the population.
The researcher may number each member of the population for random sampling purposes.
Using a calculator or computer to generate random numbers is an alternative for random sampling.
Random number tables can be utilized for random sampling.
In stratified sampling, subgroups have similar characteristics within themselves.
Cluster samples can be efficient and cost-effective, but may not always represent the population accurately.
For systematic sampling, the population is numbered and a random starting number is chosen.
An interval number is calculated for systematic sampling by dividing the population size by the desired sample size.
The process of selecting members for the sample in systematic sampling involves adding the interval number to the starting number repeatedly.
Transcripts
Browse More Related Video
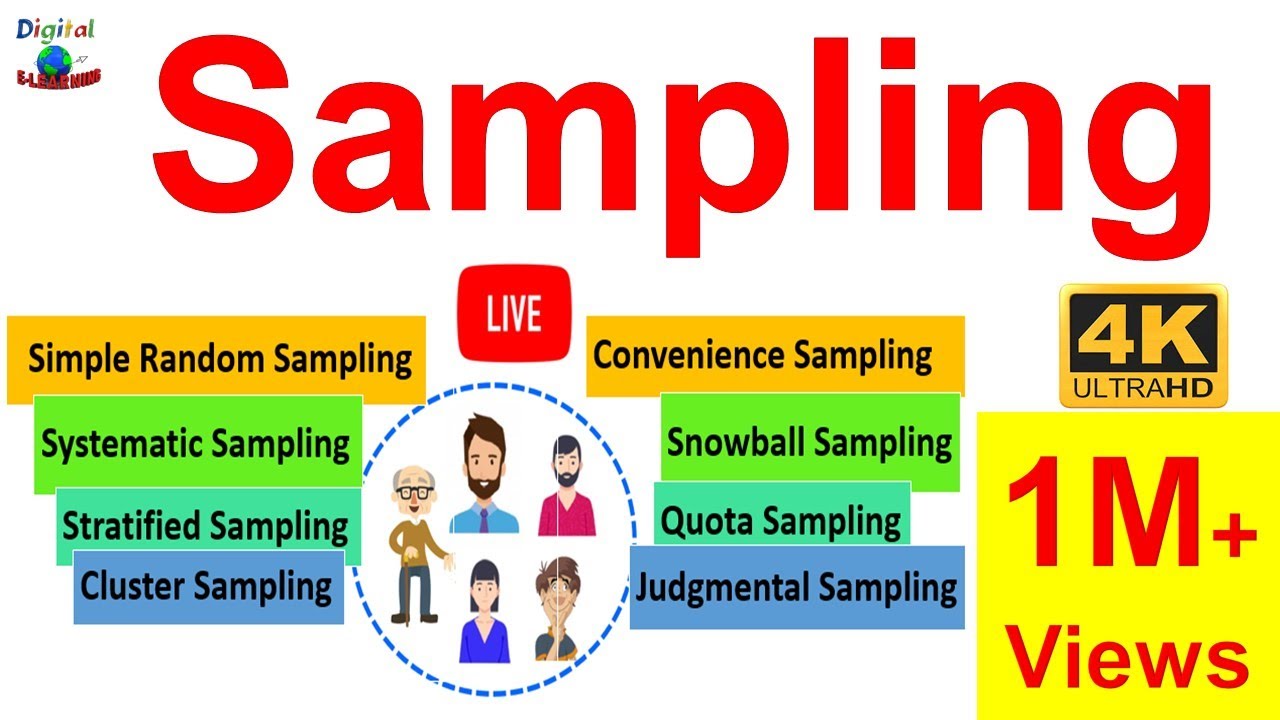
Sampling: Sampling & its Types | Simple Random, Convenience, Systematic, Cluster, Stratified
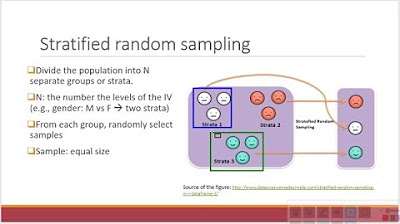
Research Methods 1: Sampling Techniques
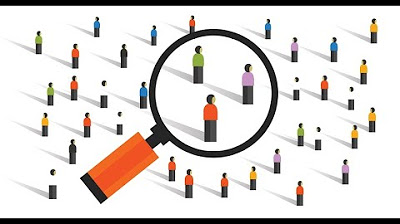
Probability and Non-Probability Sampling in Research Methods
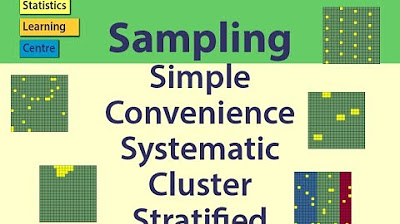
Sampling: Simple Random, Convenience, systematic, cluster, stratified - Statistics Help

Statistics Lecture 1.5: Sampling Techniques. How to Develop a Random Sample
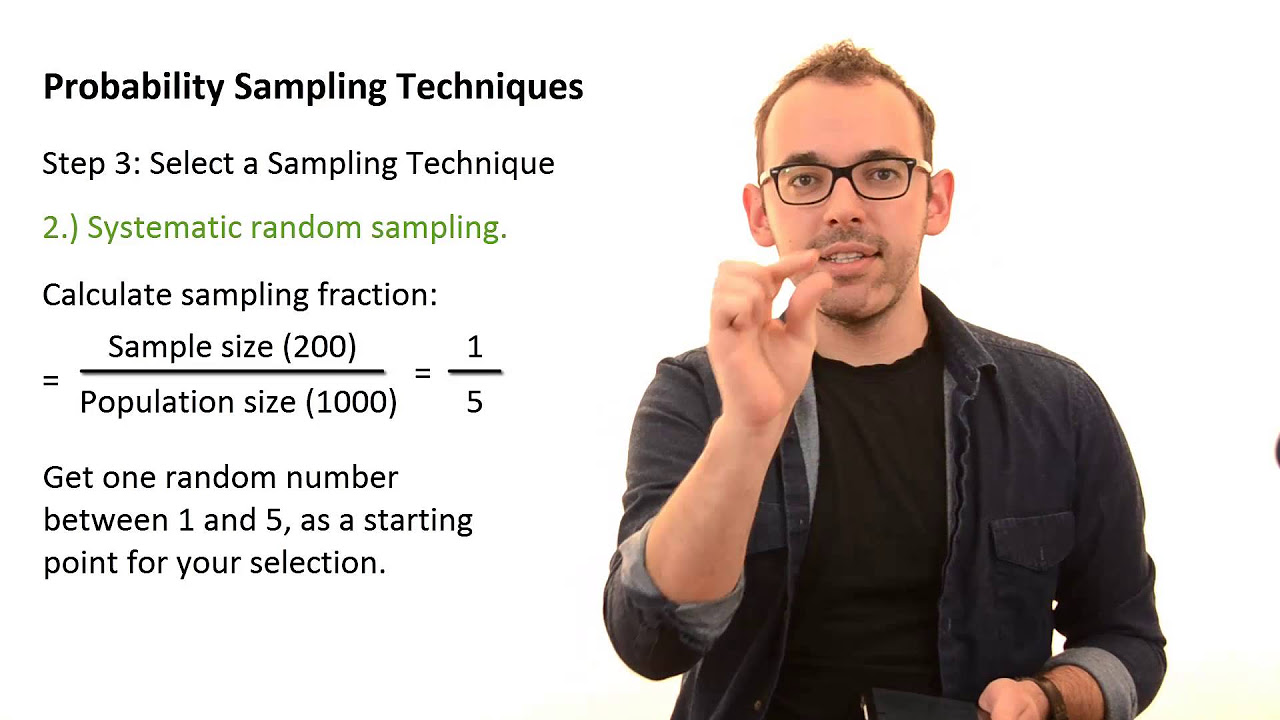
4.2 Probability Sampling Techniques
5.0 / 5 (0 votes)
Thanks for rating: