Population And Sample In Statistics Example | Population vs Sample In Statistics | Simplilearn
TLDRThis video introduces the concepts of population and sample in statistics, emphasizing their importance in research. It explains that a population is the entire set of items under study, while a sample is a smaller, representative subset used to draw conclusions about the whole. The video also discusses the differences between the two and provides real-world examples, such as student performance and job advertisements. It further explores methods of data collection from both population and sample, highlighting the practicality of sampling in large and dispersed populations to avoid bias and ensure accurate results.
Takeaways
- π A population in statistics refers to the entire set of items or individuals that data is drawn from for a study.
- π The population is the group about which you want to draw conclusions, and it can include people, objects, events, etc.
- βΉ Due to practical limitations, it's often impossible to observe an entire statistical population directly.
- π A sample is a smaller, unbiased subset of the population, used to represent and learn about the whole group.
- πΎ For example, all undergraduate students in the Netherlands could be a population, while a sample might be a subset of Belgian data scientists.
- π The process of collecting information from a sample is called sampling, which allows for making inferences or extrapolations about the population.
- π« When conducting a census or complete enumeration is impractical or impossible, sampling becomes a necessary method.
- π― Ideal samples are randomly selected and representative of the population to reduce sampling bias and enhance validity.
- π Examples of populations and samples include all students in a class, all job advertisements in India, and all countries in the world with specific data.
- π Data from a population is collected when the research question requires it and access to every member's data is available.
- π Collecting data from a sample is useful when the population is large, dispersed, or difficult to contact, allowing for manageable hypotheses testing.
Q & A
What is the definition of a population in statistics?
-In statistics, a population is the entire set of items from which data is drawn for a statistical study. It can be a group of individuals, a set of items, or anything else that constitutes the data pool for a study. It represents the entire group about which conclusions are drawn.
What are some examples of populations in real-world scenarios?
-Examples of populations include all undergraduate students in the Netherlands, Belgian data scientists, or all countries in the world. A population can refer to people living in an area at a time, but it can also consist of objects, events, organizations, species, or any other elements that researchers want to study.
Why is it impractical to observe an entire statistical population?
-Observing an entire statistical population is often impractical due to time constraints, geographical accessibility issues, and resource limitations. Researchers may not have the ability to reach every member of the population, making it necessary to use a sample to learn about the population as a whole.
How is a sample defined and what is its purpose?
-A sample is a subset of the population, consisting of observations drawn from it. It is an unbiased and representative group used to represent the data of the whole population. The purpose of a sample is to allow researchers to make inferences or extrapolations from the sample data to the larger population.
What are the typical characteristics of a sample compared to a population?
-A sample is always smaller in size than the total size of the population. It is a manageable subset used for practical reasons, as it is often impractical or impossible to collect data from every member of a large population. The sample is used to participate in the study and provide data for analysis.
How can data be collected from a population?
-Data from a population is collected when the research question requires extensive data and information about every member of the population is available. This approach is typically feasible when the population is small, accessible, and cooperative, allowing for the collection of comprehensive data.
What are some challenges associated with conducting a census or complete enumeration of a population?
-Challenges with conducting a census include the difficulty of contacting, locating, and encouraging participation from every individual, especially historically marginalized and low-income groups. This can result in an incomplete and biased population count, leading to disproportionate funding and resource allocation.
How do researchers ensure that a sample is representative of the population?
-Researchers ensure a sample is representative by using probability sampling methods, such as simple random sampling or stratified sampling. These methods reduce the risk of sampling bias and enhance both internal and external validity, making the sample a more accurate reflection of the population.
What is the process of making estimates or testing hypotheses about population data based on sample data called?
-The process of making estimates or testing hypotheses about population data based on sample data is called statistical inference. It involves using sample data to draw conclusions about the larger population through statistical analysis.
How can a sample be used to study political attitudes in young people?
-A sample can be used to study political attitudes in young people by selecting a representative group of young individuals, for example, 300 undergraduate volunteers from three Dutch universities. This sample would complete an online study, and the data collected could be used to make inferences about the political attitudes of the larger population of young people in the Netherlands.
What is the importance of using a randomly selected sample in research?
-Using a randomly selected sample is important in research because it helps ensure that the sample is unbiased and representative of the population. This random selection process reduces the risk of sampling bias and increases the validity of the study's findings, allowing for more accurate and reliable inferences to be made about the population.
Outlines
π Introduction to Population and Sample Concepts
This paragraph introduces the viewer to the fundamental concepts of population and sample in the context of statistical studies. It outlines the purpose of the video, which is to explain what a population and a sample are, and to provide real-world examples of their application. The paragraph emphasizes the importance of understanding these concepts to accurately draw conclusions from a data set. It also sets the stage for the subsequent discussion on the differences between populations and samples, and how data is collected from them.
π Collecting Data from Populations and Samples
This paragraph delves into the specifics of data collection from both populations and samples. It explains that data from a population is collected when the research question requires information from every member, and access to such data is feasible. This is typically the case when the population is small, accessible, and cooperative. However, for larger or more dispersed populations, it becomes impractical or impossible to collect data from every individual, leading to the use of samples. The paragraph also discusses the challenges associated with collecting data from the entire population, such as the U.S. Census, and how sampling can help mitigate these issues by providing more precise estimates about the population.
Mindmap
Keywords
π‘Population
π‘Sample
π‘Statistical Study
π‘Data Collection
π‘Sampling
π‘Census
π‘Inferences
π‘Validity
π‘Bias
π‘Representative
Highlights
The video introduces the concepts of population and sample in the context of statistical studies.
Population is defined as the entire set of items from which data is drawn for a statistical study.
A population can be a group of individuals, items, events, organizations, countries, species, etc.
A sample is a smaller, unbiased, and representative group drawn from the larger population.
The size of the sample is always less than the total size of the population.
A census or complete enumeration of all values in the population is often impractical or impossible.
Sampling is the process of collecting information from a sample to make inferences or extrapolations about the population.
The video provides real-world examples to illustrate the differences between population and sample.
Data from a population is collected when the research question requires information about every member.
Sampling is used when the population is too large, dispersed, or difficult to contact.
The U.S. Census is an example of an attempt to collect data from an entire population, facing challenges with marginalized and low-income groups.
Sampling can help make more precise inferences about the population when a census is incomplete or biased.
Ideally, a sample should be randomly selected and representative to reduce sampling bias and enhance validity.
Probability sampling methods like simple random sampling or stratified sampling are recommended for selecting a sample.
The video concludes by emphasizing the importance of understanding population and sample concepts in statistical research.
Transcripts
Browse More Related Video
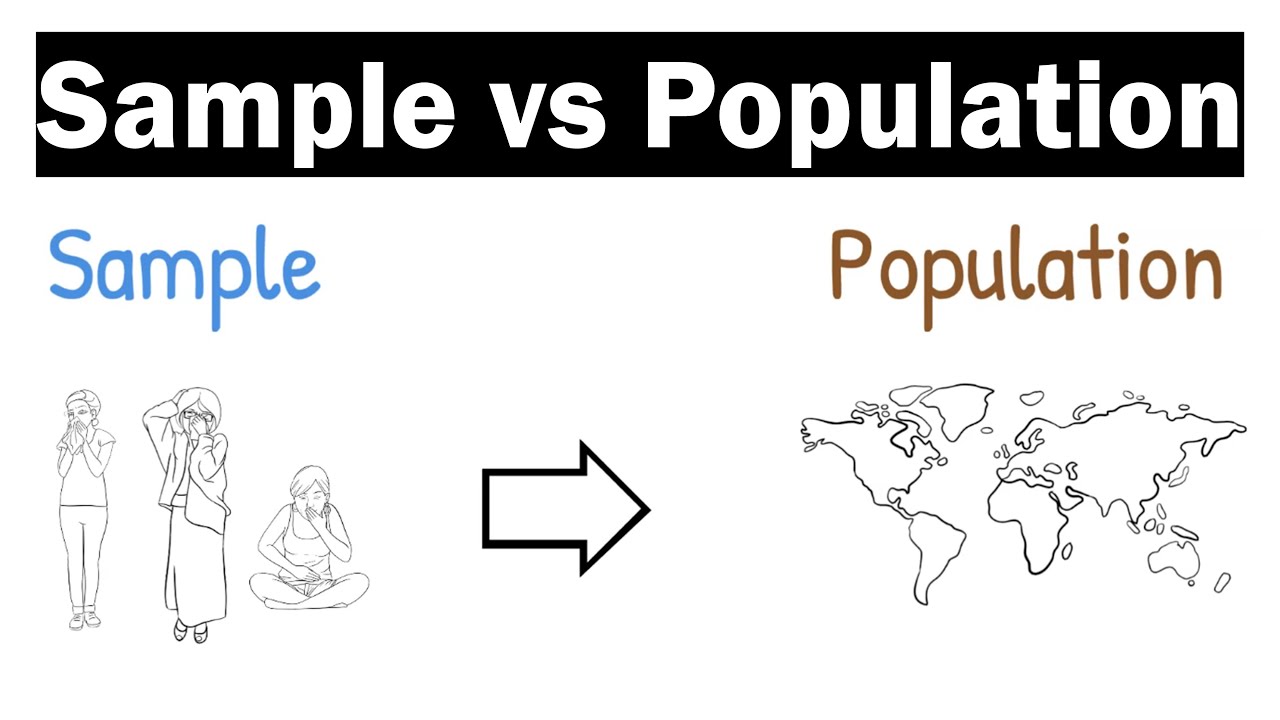
Sample vs Population - Clearly Explained
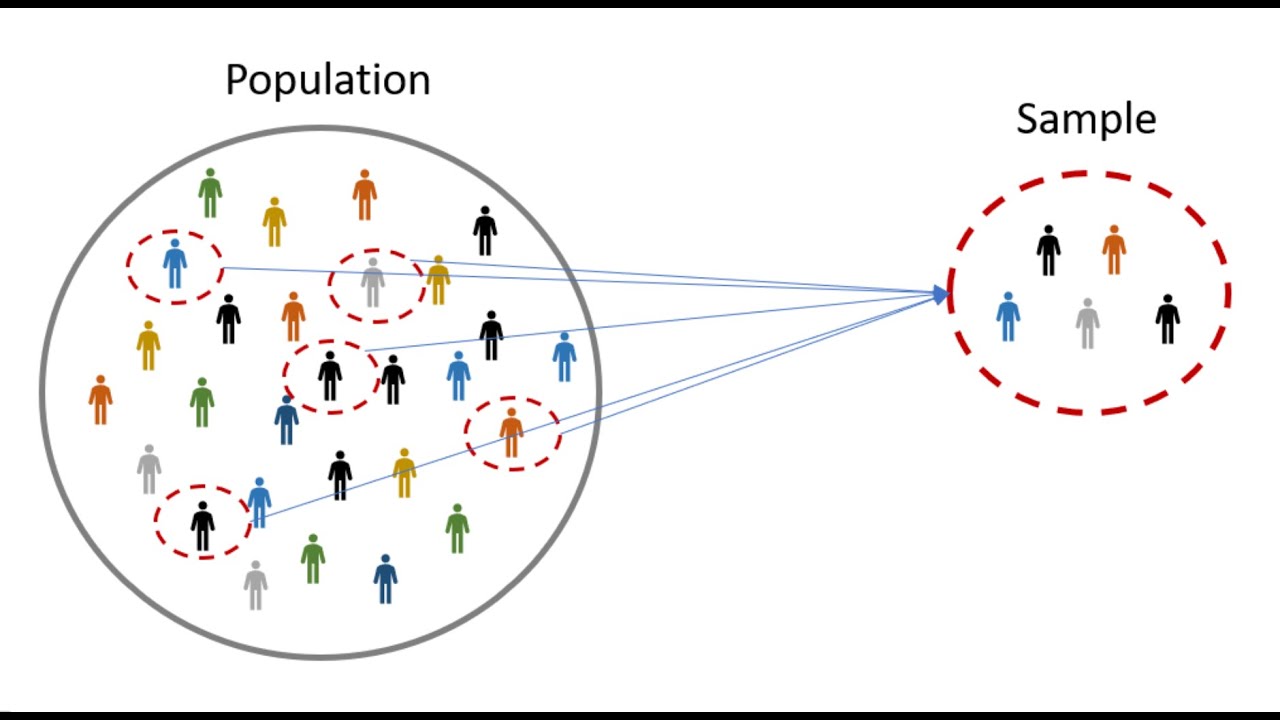
Statistics: Populations & Samples and Parameters vs Statistics
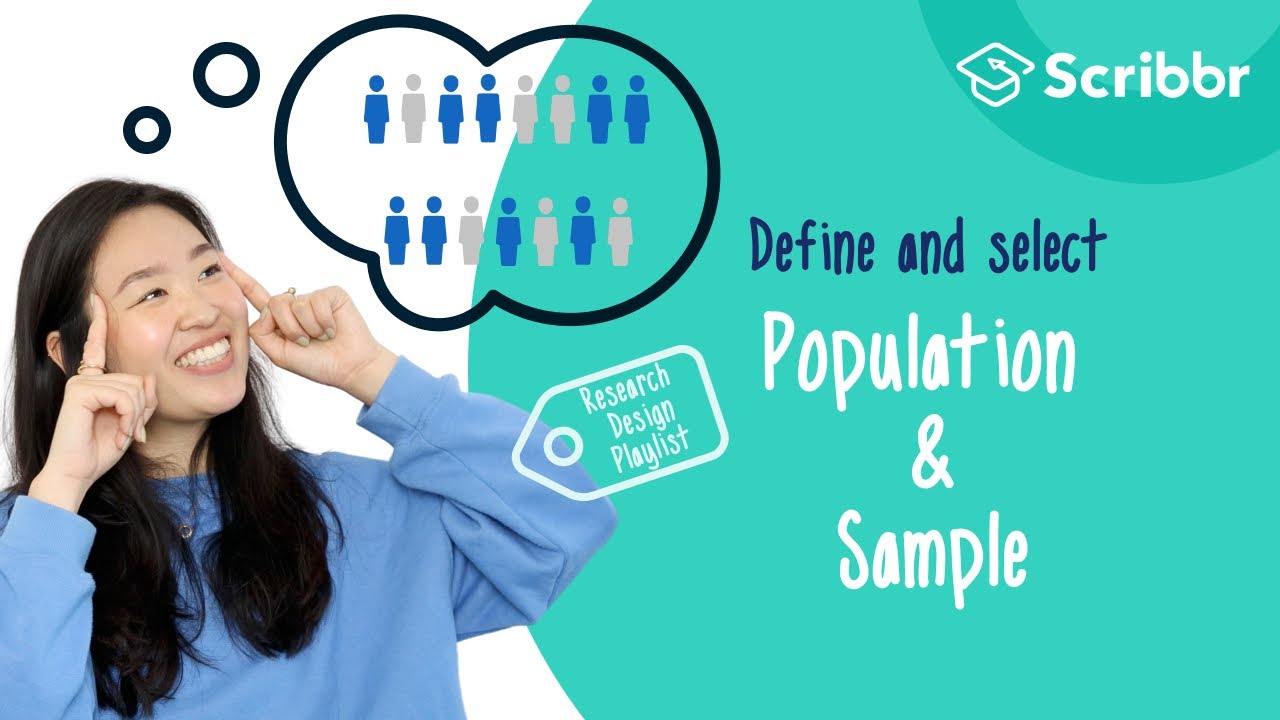
Research Design: Defining your Population and Sampling Strategy | Scribbr π
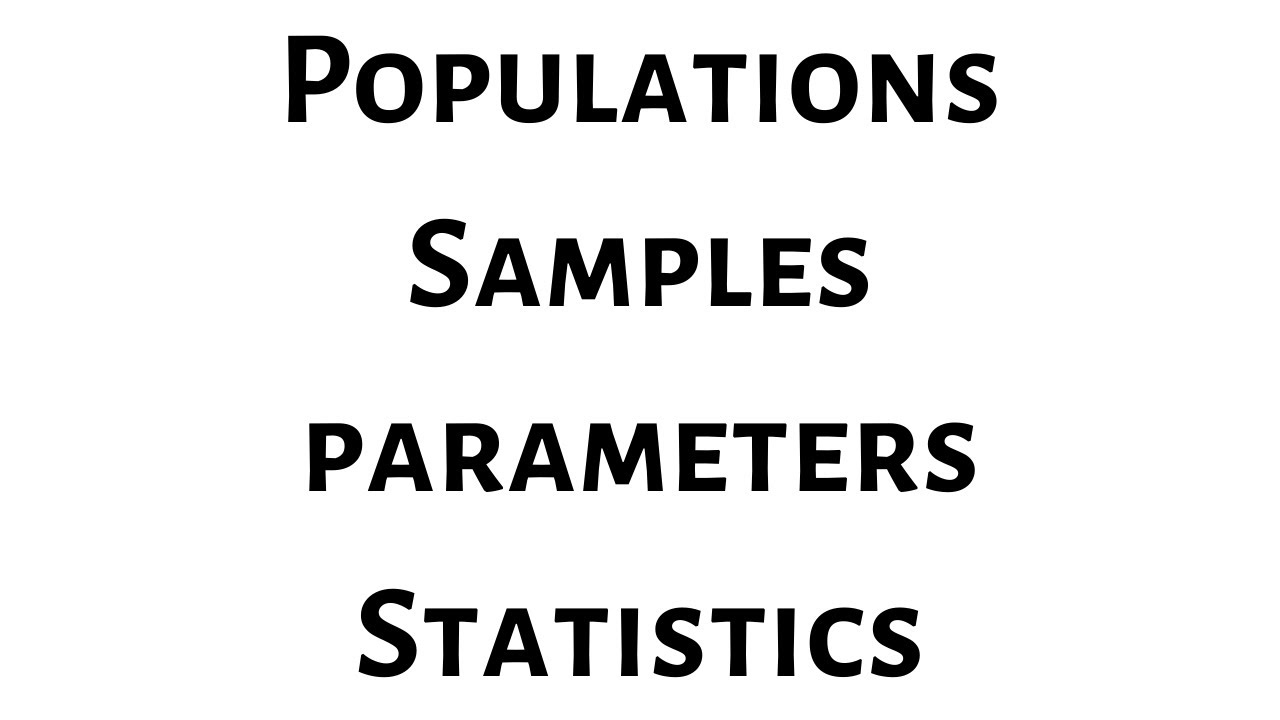
Populations, Samples, Parameters, and Statistics
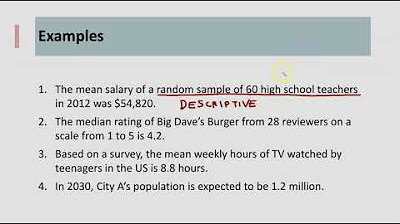
Descriptive vs Inferential Statistics
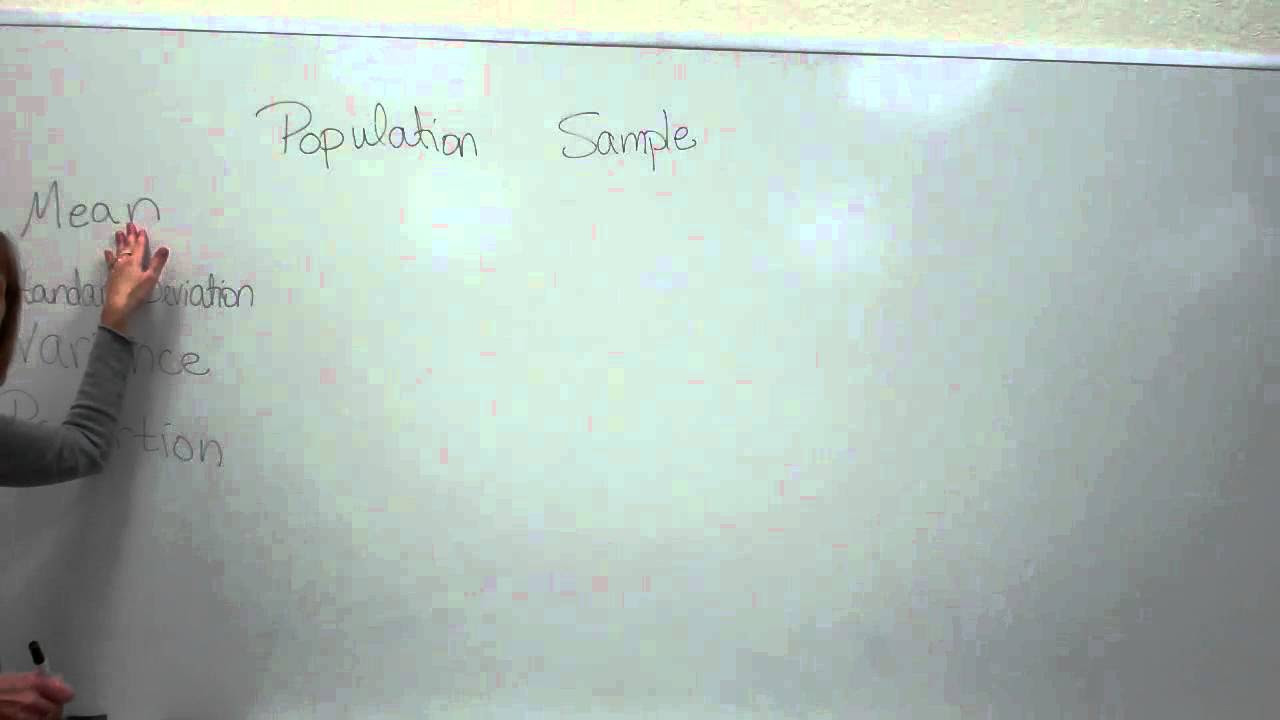
Symbols commonly used in statistics
5.0 / 5 (0 votes)
Thanks for rating: