Aspect Based Sentiment Analysis: A Python Demo
TLDRThis educational video dives into the fascinating world of Aspect-Based Sentiment Analysis (ABSA), a nuanced approach to understanding opinions within text. Starting with an engaging example, it demystifies ABSA by illustrating how it discerns aspects, opinions, and sentiments from a restaurant review. The presentation progresses to explain ABSA's terminology, its advantages over traditional sentiment analysis, and showcases expected outputs using real-world data. A detailed walkthrough of a seminal paper on ABSA introduces viewers to model architecture, data processing, and the extraction of sentiment triplets. The video concludes with a Python demo on Google Colab, demonstrating the model's application to different examples, underscoring its domain-specific effectiveness, and guiding viewers on training models with custom datasets.
Takeaways
- π Aspect-Based Sentiment Analysis (ABSA) is a method to analyze sentiments directed towards specific aspects of a product or service.
- π ABSA provides more detailed insights compared to traditional sentiment analysis by identifying particular aspects people like or dislike and the reasons behind their sentiments.
- π΄ In the given example, 'waiters' and 'pasta' are aspects, while 'friendly' and 'average' represent subjective opinions tied to these aspects with corresponding sentiments.
- π ABSA outputs structured data that can be visualized to show the frequency of mentions and associated sentiments for different aspects.
- π€ The model 'learns' to identify aspects (targets), opinions, and sentiments by breaking sentences into tokens and forming relevant spans.
- π³ The process includes aspect term extraction, opinion term extraction, and classification to reduce computational complexity and focus on valid aspects and opinions.
- π Aspects and opinions are paired and classified to determine the sentiment relationship, which can be positive, negative, or neutral.
- π The paper 'Learning Span Level Interactions for Aspect Sentiment Triplet Extraction' demonstrates a model that significantly improves performance in ABSA tasks.
- ποΈ The model's architecture includes sentence tokenization, span formation, pruning, aspect and opinion classification, and sentiment relation classification.
- π» The authors have made the code for their model publicly available on GitHub, and a Python demo is provided on Google Colab for practical understanding.
- π ABSA models can be trained from scratch using labeled training data and evaluated using metrics like the F1 score.
Q & A
What is Aspect-Based Sentiment Analysis (ABSA)?
-Aspect-Based Sentiment Analysis (ABSA) is a method of analyzing sentiments that focuses on identifying specific aspects or targets within a given product or service and determining the sentiment associated with each aspect. It provides more detailed insights compared to traditional sentiment analysis by extracting what aspects people are discussing and whether they like or dislike each aspect and the reasons behind it.
What are the components of an ABSA output?
-An ABSA output typically consists of structured data that includes aspects (also known as targets), opinions (subjective opinions about the aspects), and sentiments (positive, negative, or neutral feelings expressed towards the aspects).
How does ABSA handle multiple aspects in a single review?
-If a review contains too many aspects, ABSA can employ clustering and dimensionality reduction techniques to group similar aspects together. This helps in managing and understanding the data more effectively.
What is the significance of aspect term extraction and opinion term extraction in ABSA?
-Aspect term extraction and opinion term extraction are crucial steps in the ABSA process. They involve identifying and classifying spans (phrases) as either aspects, opinions, or invalid. This pruning operation helps in filtering out irrelevant information and retaining only the candidate aspects or targets and opinions, which are essential for determining sentiment relationships.
How does the model architecture in the discussed paper handle sentence analysis?
-The model architecture breaks a sentence into tokens, combines these tokens to form spans (phrases), and then classifies each span as invalid, an opinion, or a target (aspect). This process helps in identifying aspect-opinion pairs and determining the sentiment associated with each pair.
What is the role of the sentiment relation classifier in ABSA?
-The sentiment relation classifier in ABSA is responsible for determining whether each aspect-opinion pair conveys a positive, negative, or neutral sentiment. It can also identify if a pair is invalid, such as when the aspect is a fact rather than a subjective opinion, and therefore, no sentiment relationship exists.
How does the model handle computational complexity?
-The model controls computational complexity by performing aspect term extraction and opinion term extraction, which prunes the number of possible spans. This is necessary because the number of potential spans grows exponentially with the length of the sentence.
What are the different training and testing sets used in the paper?
-The paper uses four different training and testing sets, namely Rest 14 (restaurant reviews from 2014) and Lab 14 (laptop reviews from 2014). These datasets were originally released by the Semantic Evaluation Workshop.
How can one evaluate the performance of an ABSA model?
-The performance of an ABSA model can be evaluated using metrics such as F1 score, which measures the model's precision and recall in identifying aspect-opinion pairs and their associated sentiments accurately.
Is it possible to retrain the ABSA model on new data?
-Yes, the ABSA model can be retrained from scratch using your own labeled training data. This allows for the model to be adapted to specific domains or datasets, improving its ability to detect sentiment aspect triplets in the new context.
Where can one find the code and resources for the ABSA model discussed in the paper?
-The authors of the paper have made their code publicly available on GitHub, and there is a Python demo available on Google Colab that demonstrates how to use the model for predicting sentiment aspect triplets.
Outlines
π Introduction to Aspect-Based Sentiment Analysis (ABSA)
This paragraph introduces aspect-based sentiment analysis (ABSA), a method for extracting sentiment from reviews and opinions. It explains how ABSA can identify specific aspects (like 'waiters' and 'pasta' from a restaurant review) and the associated sentiments (positive or negative). The paragraph also outlines the process of ABSA, starting with an example of a restaurant review and discussing the terminology used in the field. It emphasizes the benefits of ABSA over traditional sentiment analysis, such as providing more detailed insights into customer opinions on specific aspects of a product or service.
π Understanding ABSA Outputs and Model Architecture
This paragraph delves into the expected outputs of ABSA and the architecture of a specific model designed for aspect sentiment triplet extraction. It describes how ABSA can process a large number of reviews to identify mentions and sentiments associated with different aspects. The paragraph walks through a hypothetical example of analyzing restaurant reviews and introduces the concept of clustering and dimension reduction for managing numerous aspects. It also explains the model's architecture, including tokenization, span formation, aspect and opinion term extraction, and sentiment relation classification. The performance of the model is discussed, along with its training and testing on various datasets.
Mindmap
Keywords
π‘Aspect-Based Sentiment Analysis (ABSA)
π‘Structured Data
π‘Aspects or Targets
π‘Opinions
π‘Sentiment
π‘Span
π‘Tokenization
π‘Aspect Term Extraction
π‘Opinion Term Extraction
π‘Sentiment Relation Classifier
π‘Model Architecture
π‘Data Sets
Highlights
Introduction to Aspect-Based Sentiment Analysis (ABSA), a method for detailed sentiment analysis.
ABSA provides more detailed insights compared to traditional sentiment analysis by identifying specific aspects people like or dislike.
An example of ABSA output shows structured data that identifies aspects (waiters, pasta), opinions (friendly, average), and sentiments (positive, negative).
Terminology clarification: aspects are also called targets, and opinions are subjective views on these aspects.
Overview of ABSA outputs, which can include counts of mentions and associations with sentiments (e.g., food mentioned 523 times with various sentiments).
Clustering and dimension reduction can be used to group similar aspects together when there are too many aspects.
Walkthrough of a paper titled 'Learning Span Level Interactions for Aspect Sentiment Triplet Extraction', focusing on a model that identifies aspect-sentiment relationships.
The model breaks sentences into tokens and forms spans to identify aspects and opinions within consumer reviews.
The process includes aspect term extraction, opinion term extraction, and pruning to manage computational complexity.
Each aspect is paired with each opinion, and a classifier determines the sentiment relationship between them (positive, negative, or neutral).
The model's performance is significantly improved compared to previous papers, with variations depending on the model used (LSTM or BERT).
Training and testing datasets include restaurant reviews from 2014 (Rest 14) and laptop reviews from 2014 (Lab 14).
Authors have made their code publicly available on GitHub for further exploration and application.
A Python demo is available on Google Colab, showing the process of downloading packages and loading pre-trained models.
Demonstration of how the model works with a training example, identifying the target, opinion, and sentiment in a given sentence.
The model's sensitivity to the training data is highlighted, as it struggles with examples outside its domain of training (e.g., medical domain).
The notebook also shows how to train a model from scratch using your own labeled training data and how to evaluate the model using F1 score.
The video concludes with a call to action for viewers to like and subscribe if they found the content useful.
Transcripts
Browse More Related Video
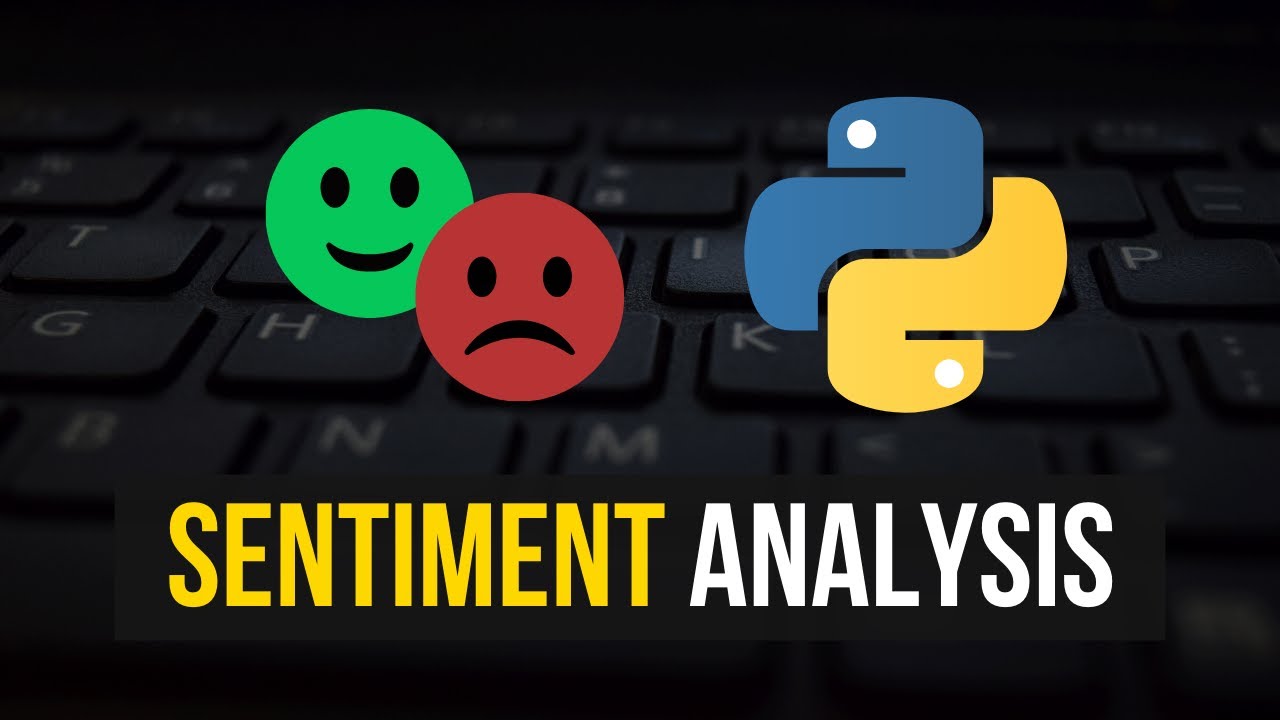
Simple Sentiment Text Analysis in Python
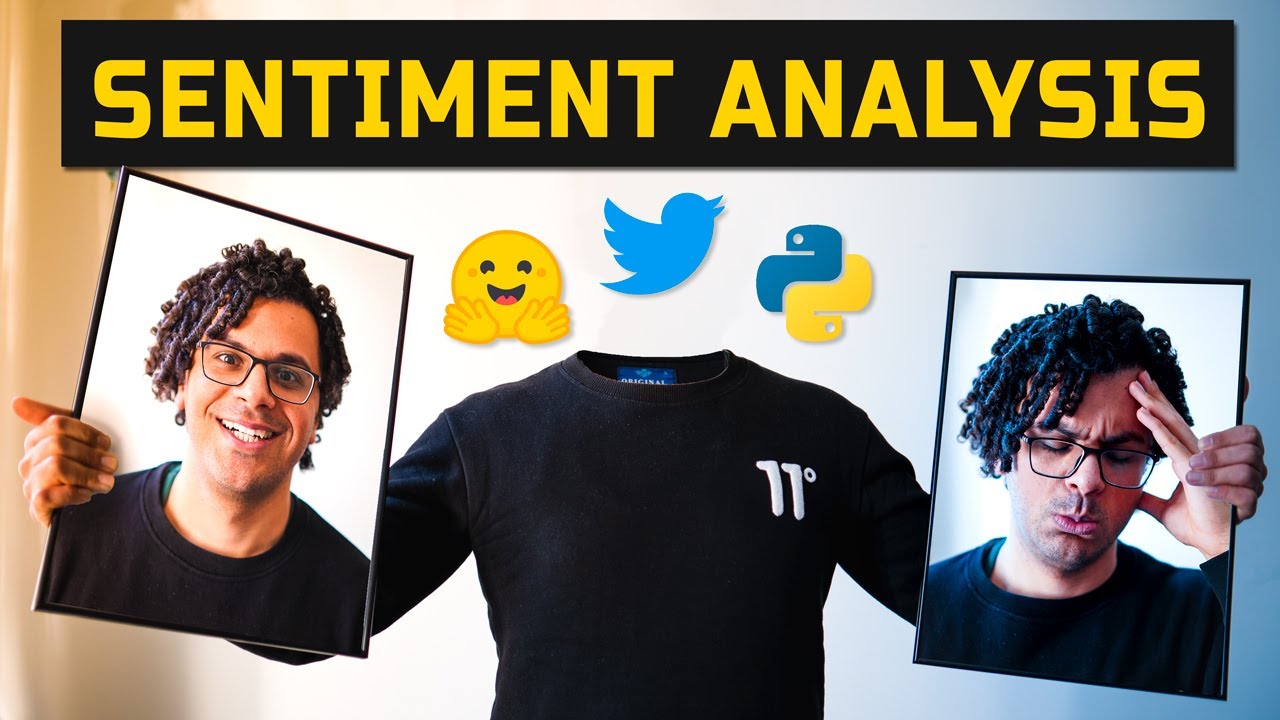
Twitter Sentiment Analysis by Python | best NLP model 2022

Python Sentiment Analysis Project with NLTK and π€ Transformers. Classify Amazon Reviews!!
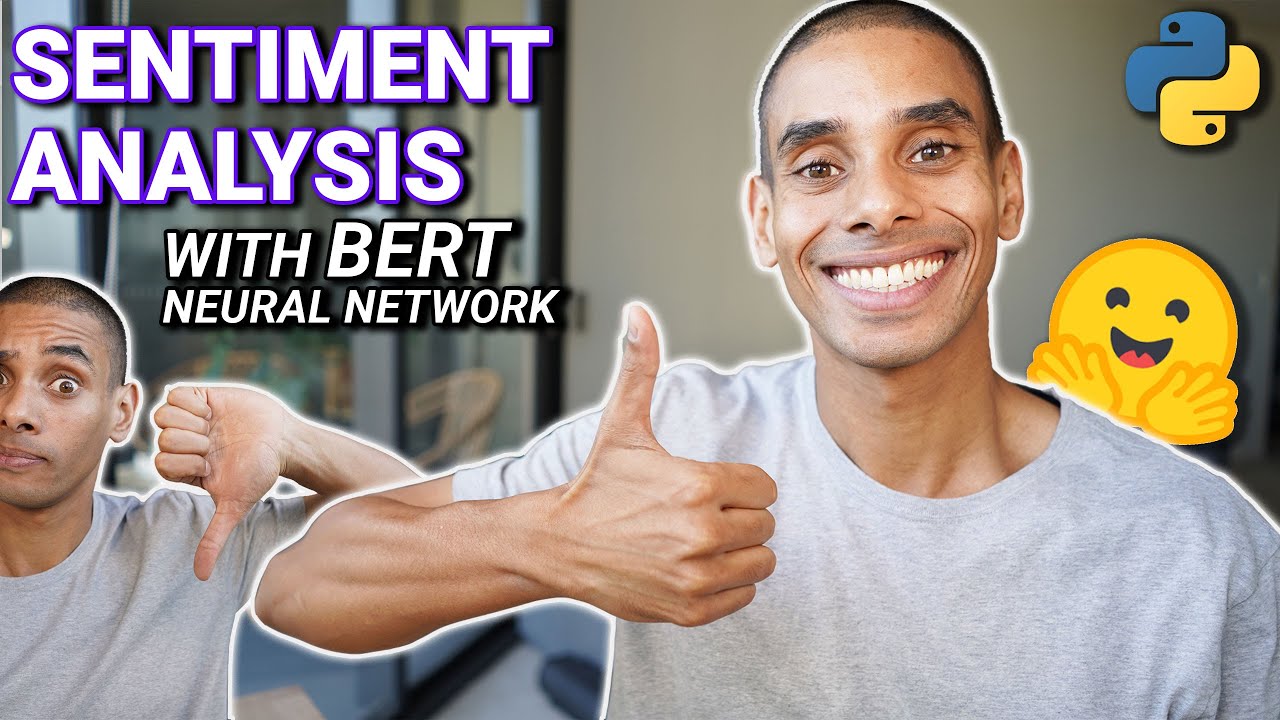
Sentiment Analysis with BERT Neural Network and Python

Stock Market Sentiment Analysis Using Python & Machine Learning
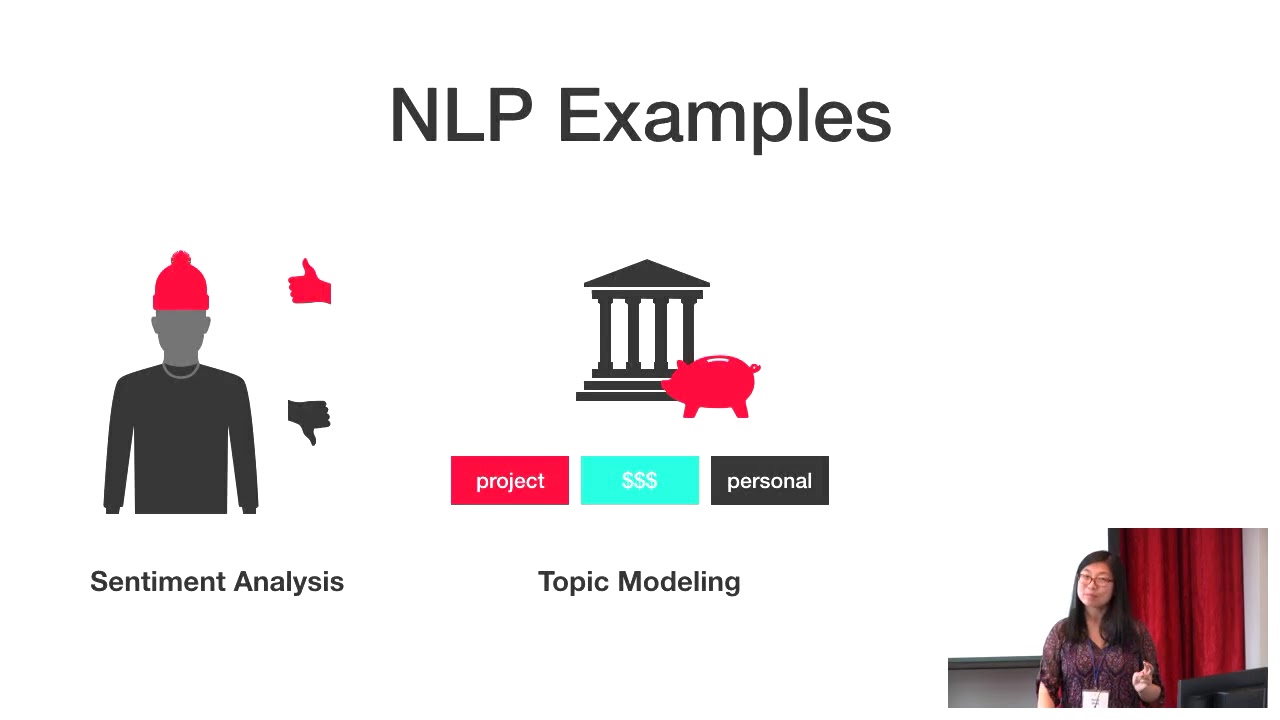
Natural Language Processing in Python
5.0 / 5 (0 votes)
Thanks for rating: