What are degrees of freedom?!? Seriously.
TLDRThe video script by Justin Seltzer from Zen Statistics delves into the concept of degrees of freedom in statistics, emphasizing its importance in understanding statistical foundations. It explains degrees of freedom in the context of descriptive statistics, regression analysis, and chi-squared tests, using the example of sea urchin spike counts to illustrate the concepts. The script clarifies how degrees of freedom relate to estimating population parameters from sample data, highlighting its role in calculating standard deviations, variance, and assessing the relationship between variables.
Takeaways
- π Degrees of freedom (DF) are central to understanding statistics as they relate to estimating population values from sample data.
- π§ The concept of DF is about the number of independent pieces of information available to estimate population parameters.
- π’ In descriptive statistics, like calculating standard deviation, DF is linked to the number of observations minus one (n-1).
- π For a sample size of one, the standard deviation is undefined because there's no variability, hence zero degrees of freedom.
- π With two observations, the mean can be estimated, but the spread (standard deviation) cannot, as there's only one degree of freedom.
- π€ In regression analysis, the number of degrees of freedom is related to the number of observations minus the number of estimated parameters.
- π In a simple linear regression with one predictor variable, the minimum number of observations required for analysis is three to account for degrees of freedom.
- π For chi-squared tests, the degrees of freedom depend on the number of categories and the constraints of the expected frequencies.
- π In chi-squared goodness of fit test, DF is calculated as the number of categories minus one.
- π Adding more predictor variables in regression reduces the degrees of freedom, as each additional variable consumes one degree of freedom.
- π‘ Understanding degrees of freedom is crucial for statistical inference, hypothesis testing, and the reliability of statistical results.
Q & A
What is the main concept discussed in the transcript?
-The main concept discussed in the transcript is the notion of degrees of freedom in statistics, and how it applies to various statistical scenarios such as descriptive statistics, regression, and chi-squared tests.
Why is the concept of degrees of freedom important in statistics?
-Degrees of freedom is important in statistics because it represents the number of independent pieces of information available to estimate population parameters from a sample, which is crucial for understanding the reliability and accuracy of statistical estimates.
What is the intuition behind degrees of freedom?
-The intuition behind degrees of freedom is that it is related to the number of data points we have available to estimate the population values, such as the mean, standard deviation, or other statistical parameters. It reflects the amount of information we can extract from our sample to make inferences about the population.
How does the concept of degrees of freedom apply to descriptive statistics?
-In descriptive statistics, degrees of freedom apply to the calculation of the sample variance and standard deviation. The formula for variance uses 'n - 1' instead of 'n' in the denominator to account for the fact that the sample mean is used as an estimate for the population mean, which reduces the number of independent observations by one.
What is the relationship between degrees of freedom and the sample size in a statistical context?
-The degrees of freedom are directly related to the sample size in that as the sample size increases, the degrees of freedom also increase, providing more information to estimate the population parameters. However, when using sample statistics like the mean, each estimate reduces the degrees of freedom by one because the estimate itself is derived from the data.
How does the addition of more variables in a regression model affect the degrees of freedom?
-Adding more variables to a regression model, known as X variables, reduces the degrees of freedom because each variable adds an additional parameter that needs to be estimated. The degrees of freedom in regression is calculated as 'n - k - 1', where 'n' is the sample size and 'k' is the number of X variables.
What is the significance of the 'n - 1' factor in the calculation of variance and standard deviation?
-The 'n - 1' factor is used in the calculation of variance and standard deviation to correct for the bias that occurs when using the sample mean as an estimate for the population mean. This adjustment provides an unbiased estimate of the population variance and standard deviation, which is essential for making accurate statistical inferences.
How does the concept of degrees of freedom apply to chi-squared tests?
-In chi-squared tests, degrees of freedom are related to the number of independent pieces of information used to calculate the test statistic. For a chi-squared goodness of fit test, the degrees of freedom is equal to the number of categories minus one. For a chi-squared test for independence, the degrees of freedom is calculated as (number of rows - 1) * (number of columns - 1).
What is the role of degrees of freedom in determining the reliability of a statistical estimate?
-The degrees of freedom play a critical role in determining the reliability of a statistical estimate. Higher degrees of freedom generally lead to more reliable estimates as there is more information available to make inferences about the population. Conversely, lower degrees of freedom can result in less reliable estimates due to the smaller amount of data used for estimation.
Can you provide an example of how degrees of freedom are calculated in a real-world scenario as described in the transcript?
-In the transcript, an example is given where a sample of five sea urchins is used to estimate the mean number of spikes. The degrees of freedom for estimating the mean (mu) is equal to the sample size, which is five. However, for estimating the population standard deviation (sigma), the degrees of freedom is 'n - 1', which in this case is 5 - 1 = 4.
What is the significance of the sea urchin example in the transcript?
-The sea urchin example in the transcript serves as a practical illustration of how degrees of freedom operate in various statistical contexts. It helps to demonstrate the concepts of estimation, the calculation of sample statistics, and the application of degrees of freedom in descriptive statistics, regression, and chi-squared tests in a relatable and easy-to-understand manner.
Outlines
π Introduction to Degrees of Freedom
This paragraph introduces the concept of degrees of freedom in the context of statistics, specifically focusing on its relevance in understanding sample statistics such as mean, median, standard deviation, skewness, and kurtosis. It highlights the common confusion students face when first encountering the 'n-1' rule in statistical calculations and sets the stage for a deeper exploration into the topic. The speaker, Justin Seltzer, outlines the structure of the presentation, which includes an explanation of the intuition behind degrees of freedom, its application in descriptive statistics, regression, and chi-squared tests, using the example of sea urchin spike counts to illustrate the concepts.
π§ Estimation and Intuition Behind Degrees of Freedom
In this paragraph, the discussion delves into the intuition behind degrees of freedom by explaining that sample statistics are estimates of population parameters. It emphasizes that statisticians use sample data to infer information about the entire population, and degrees of freedom represent the number of independent pieces of information available for this estimation. The concept is clarified using the example of counting spikes on sea urchins, where the sample mean and standard deviation are used as estimates for the population mean and population standard deviation, respectively. The paragraph also touches on the idea that the number of degrees of freedom is related to the number of observations used to estimate population values.
π Degrees of Freedom in Descriptive Statistics
This paragraph explores the role of degrees of freedom in descriptive statistics, using the examples of sample size and the calculation of mean, range, variance, and standard deviation. It explains how the number of degrees of freedom affects the ability to estimate the spread or dispersion of a dataset. The speaker illustrates this with scenarios of increasing sample size, from one to two observations, and how each additional observation provides more information and thus more degrees of freedom, allowing for better estimation of population parameters. The paragraph also discusses the calculation of variance and standard deviation, highlighting the adjustment made in the denominator from 'n' to 'n-1' to account for the estimation of the population mean from the sample mean.
π Degrees of Freedom in Regression Analysis
The paragraph discusses the application of degrees of freedom in regression analysis, explaining how it relates to the estimation of relationships between variables. It clarifies that regression not only estimates the coefficients (slope and intercept) but also the uncertainty associated with these estimates. The concept is illustrated with the minimum number of observations required to perform a regression analysis and how additional observations provide the necessary degrees of freedom to estimate uncertainty. The speaker also explains how adding more explanatory variables (X variables) to a regression model reduces the degrees of freedom, which in turn affects the estimation of coefficients and their standard errors.
π§© Degrees of Freedom in Chi-Squared Tests
This paragraph focuses on the application of degrees of freedom in chi-squared tests, specifically the chi-squared goodness of fit test and the chi-squared test for independence. It explains how degrees of freedom are calculated in these tests by considering the number of independent pieces of information, which are the deviations between observed and expected frequencies. The speaker uses the example of sea urchin subtypes and their distribution to illustrate how degrees of freedom are determined in a chi-squared test. The paragraph also highlights the importance of understanding the concept of degrees of freedom for correctly interpreting statistical results and making valid inferences from data.
π€ Conclusion and Final Thoughts
In the concluding paragraph, the speaker, Justin Seltzer, wraps up the discussion on degrees of freedom by summarizing the key points covered in the presentation. He reiterates the importance of understanding degrees of freedom in various statistical contexts, including descriptive statistics, regression analysis, and chi-squared tests. The speaker emphasizes that grasping this concept is crucial for a deeper understanding of statistics as a whole. He invites viewers to engage with his content further through his channel, Zen Statistics, and encourages them to subscribe and explore more videos on the topic.
Mindmap
Keywords
π‘Degrees of Freedom
π‘Sample Statistics
π‘Estimation
π‘Population Mean (ΞΌ)
π‘Sample Variance and Standard Deviation
π‘Regression Analysis
π‘Chi-Squared Tests
π‘Sample Size (n)
π‘Estimate of Spread
π‘Independent Observations
Highlights
The concept of degrees of freedom is introduced as a foundational element in statistics, which is often overlooked.
Degrees of freedom are tied to the idea of using sample statistics as estimates for population values.
The number of degrees of freedom is the count of independent pieces of information used to estimate population values.
In descriptive statistics, the degrees of freedom for standard deviation is 'n - 1', where 'n' is the sample size.
With a sample size of one, the standard deviation is undefined because there is no variation to measure.
When estimating the mean, the degrees of freedom is equal to the sample size, as it is the only value available.
In regression analysis, the degrees of freedom are related to the number of observations and the number of independent variables.
For a simple linear regression with one independent variable, the minimum number of observations required is three to estimate the uncertainty.
In chi-squared tests, the degrees of freedom calculation depends on the number of categories and the constraints of the observed and expected frequencies.
Chi-squared tests for goodness of fit assess the deviation of observed frequencies from expected frequencies in categories.
Chi-squared tests for independence evaluate whether there is a relationship between two categorical variables based on observed and expected frequencies.
The presentation uses sea urchin data as a running example to illustrate the application of degrees of freedom in various statistical contexts.
The concept of estimation is central to understanding the role of degrees of freedom in statistical analysis.
The video aims to demystify degrees of freedom and encourage a deeper understanding of its importance in statistical methods.
Justin Seltzer from Zen Statistics provides a comprehensive breakdown of degrees of freedom in different statistical scenarios.
The video content is structured into four sections: intuition behind degrees of freedom, descriptive statistics, regression, and chi-squared tests.
The presentation emphasizes the importance of not dismissing degrees of freedom as it is crucial for understanding the foundations of statistics.
Transcripts
Browse More Related Video
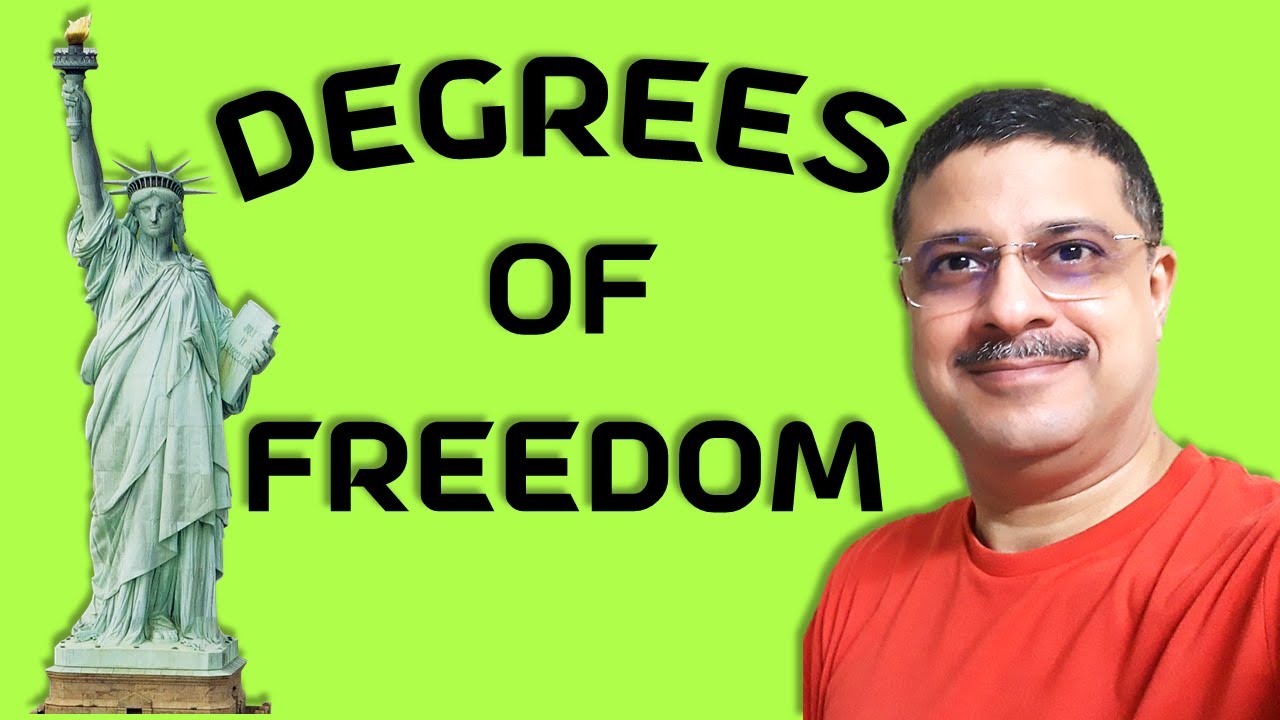
What is Degrees Of Freedom in Statistics? Degrees of freedom in Statistics Explained!
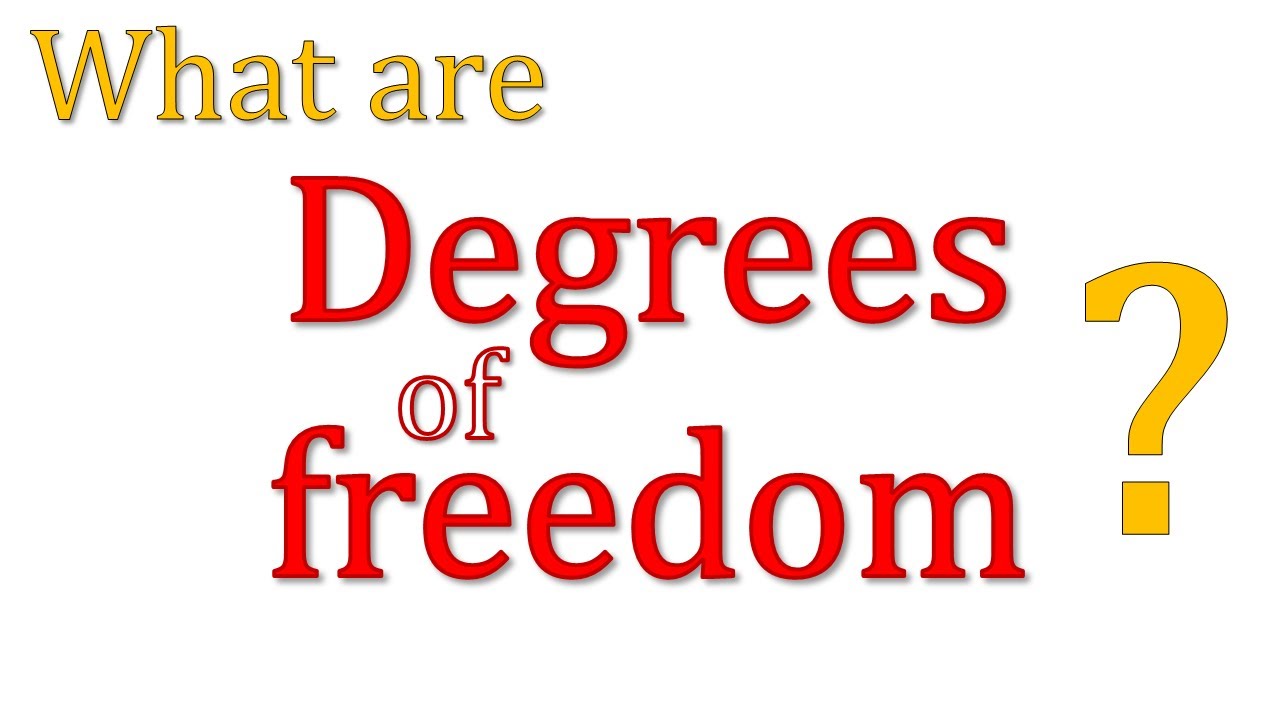
Statistical degrees of freedom - What are they REALLY?
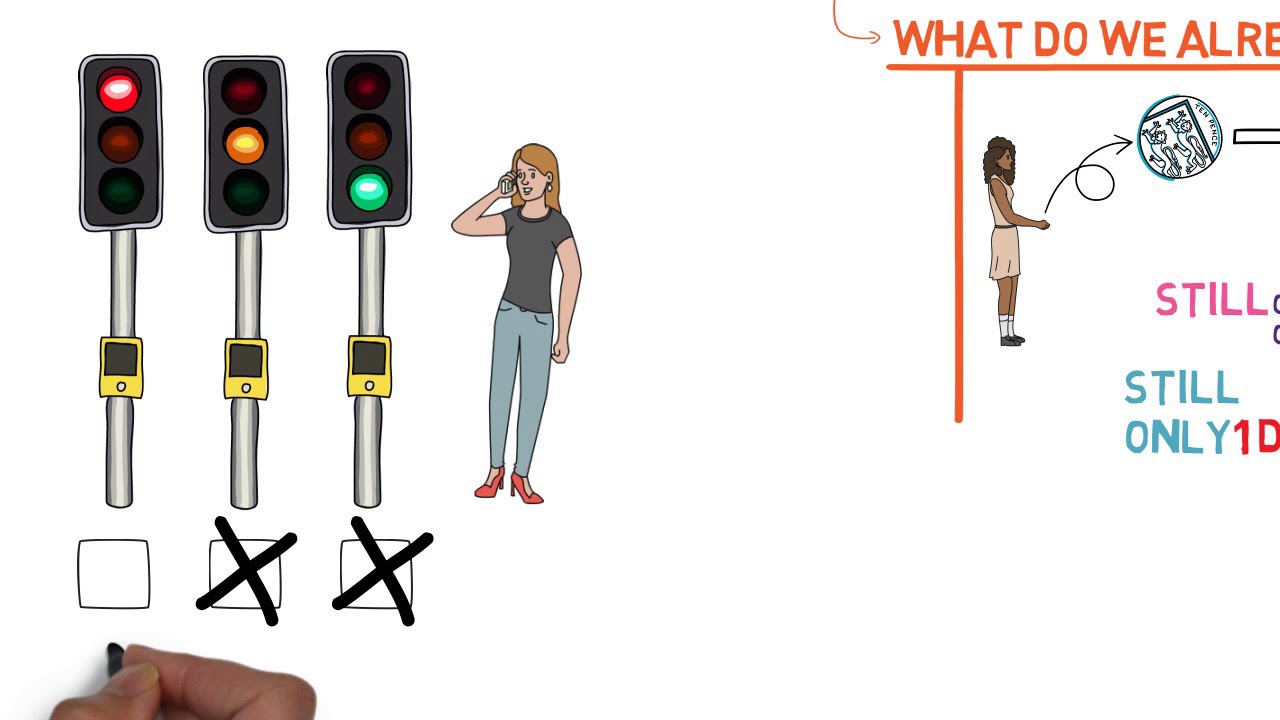
what are degrees of freedom?
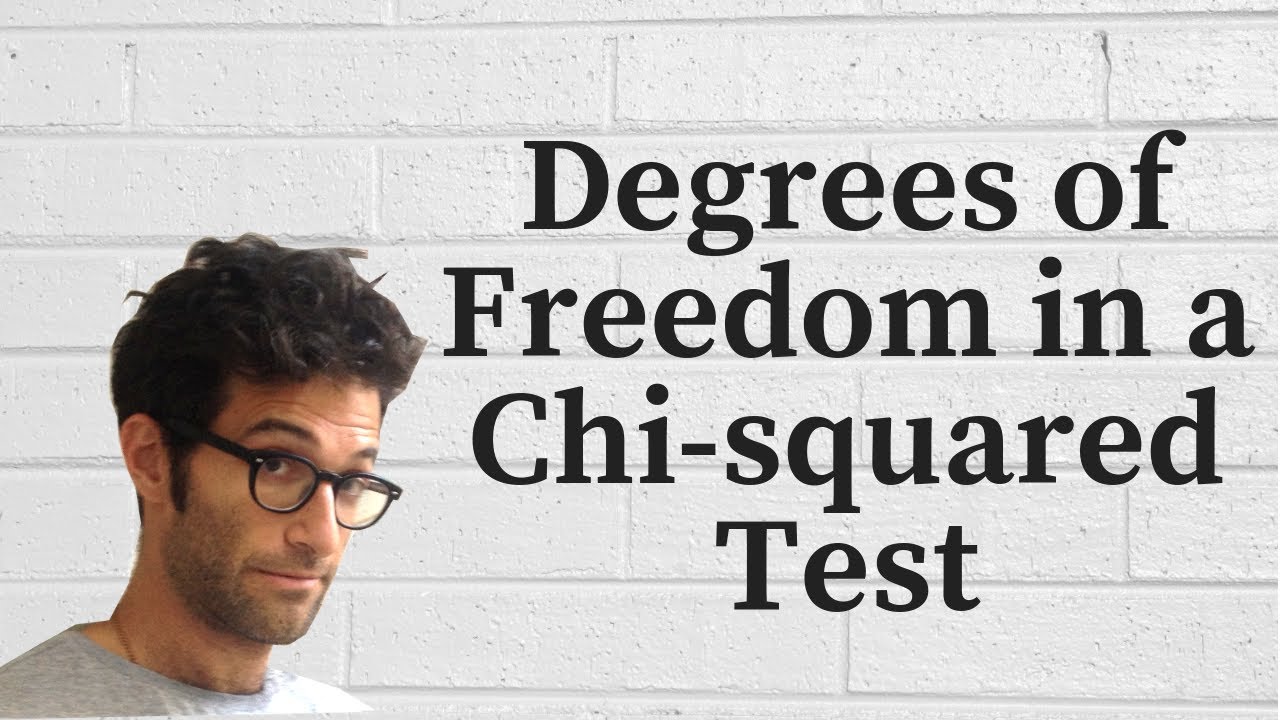
Degrees Of Freedom in a Chi-Squared Test
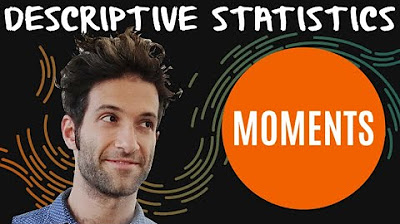
What are "moments" in statistics? An intuitive video!
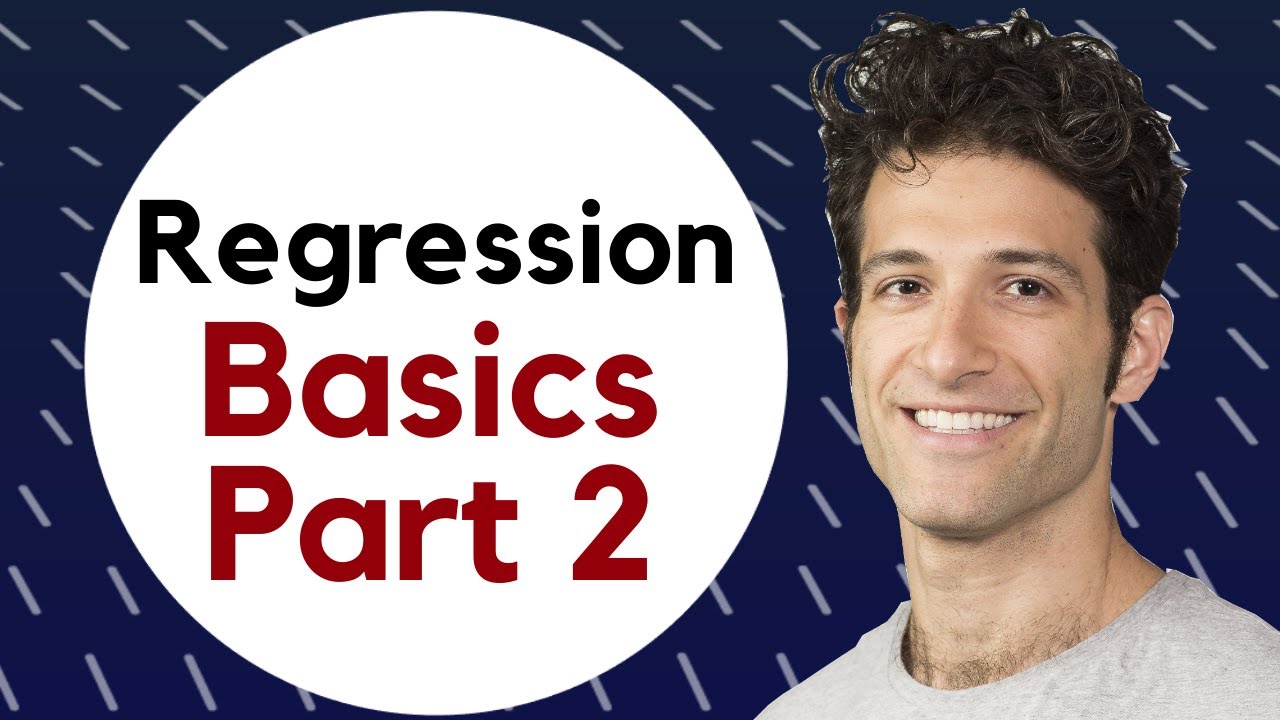
Regression II - Degrees of Freedom EXPLAINED | Adjusted R-Squared
5.0 / 5 (0 votes)
Thanks for rating: