Sensitivity, Specificity, PPV, and NPV
TLDRThe video script introduces key concepts in medical testing, focusing on sensitivity, specificity, positive predictive value (PPV), and negative predictive value (NPV). It explains sensitivity as the ability of a test to correctly identify those with a disease, while specificity is the test's ability to correctly identify those without the disease. PPV indicates the likelihood of having the disease when the test is positive, and NPV indicates the likelihood of not having it when the test is negative. The script emphasizes the importance of understanding these values in clinical practice and the necessity of using multiple tests to accurately diagnose conditions.
Takeaways
- π Sensitivity is the ability of a test to correctly identify those with the disease (true positives).
- π Specificity is the ability of a test to correctly identify those without the disease (true negatives).
- π A highly sensitive test is useful for ruling out a disease when the result is negative, providing assurance that the disease is unlikely.
- π₯ Screening tests are typically sensitive to indicate the presence of a problem but may lack specificity to pinpoint the exact issue.
- π Specificity is important for ruling in a disease; a specific test that returns a positive result strongly suggests the presence of the disease.
- π€ Sensitivity and specificity are independent of the disease's prevalence in the population.
- π The 2x2 table is used to calculate sensitivity, specificity, positive predictive value (PPV), and negative predictive value (NPV).
- π€ PPV indicates the probability of having the disease when the test is positive, while NPV indicates the probability of not having the disease when the test is negative.
- π½ As disease prevalence increases in the population, PPV increases and NPV decreases.
- 𧬠In clinical practice, multiple tests are used to increase the likelihood of accurately ruling in or ruling out a condition.
- π Understanding the relationship between sensitivity, specificity, and predictive values is crucial for interpreting test results and making informed clinical decisions.
Q & A
What is sensitivity in the context of medical tests?
-Sensitivity refers to the ability of a test to correctly identify patients who have the disease. It is calculated as the number of true positives divided by the sum of true positives and false negatives.
What are true positives and false negatives in medical testing?
-True positives are the cases where the test correctly identifies patients with the disease as positive. False negatives occur when the test fails to detect the disease in patients who actually have it, resulting in a negative test result.
Why is a highly sensitive test useful for ruling out a disease?
-A highly sensitive test is useful for ruling out a disease because if the test result is negative, it indicates with a high degree of certainty that the disease is not present. This makes it effective as a screening tool.
How is specificity defined in medical testing?
-Specificity is the ability of a test to correctly identify patients who do not have the disease. It is calculated as the number of true negatives divided by the sum of true negatives and false positives.
What are true negatives and false positives in medical testing?
-True negatives are the cases where the test correctly identifies patients without the disease as negative. False positives occur when the test incorrectly identifies healthy patients as having the disease, resulting in a positive test result.
How is positive predictive value (PPV) calculated and what does it measure?
-Positive predictive value is calculated by dividing the number of true positives by the sum of true positives and false positives. It measures the probability that a patient actually has the disease when the test result is positive.
How is negative predictive value (NPV) calculated and what does it measure?
-Negative predictive value is calculated by dividing the number of true negatives by the sum of true negatives and false negatives. It measures the probability that a patient does not have the disease when the test result is negative.
What is the relationship between positive and negative predictive values and the prevalence of a disease?
-Positive predictive value is directly related to the prevalence of a disease in the population. As prevalence increases, PPV also increases. Conversely, negative predictive value is inversely related to prevalence; as prevalence increases, NPV decreases.
Why is it important to use multiple tests in clinical practice?
-Using multiple tests in clinical practice increases the likelihood of accurately ruling in or ruling out a disease. Since no single test is 100% accurate, combining tests with different sensitivities and specificities helps to refine the diagnosis.
What should be considered when applying positive and negative predictive values to a patient's situation?
-It's important to consider that positive and negative predictive values are highly dependent on the prevalence of the disease in the population from which the statistics were derived. If a patient comes from a different population, the predictive values may not be applicable or useful.
How can one improve the accuracy of diagnosing a musculoskeletal disorder?
-In the case of musculoskeletal disorders, using multiple tests that vary in sensitivity and specificity can help to narrow down the diagnosis. This approach increases the likelihood of correctly identifying the problem by considering the strengths and limitations of each test.
Outlines
π Introduction to Medical Test Metrics
This paragraph introduces the concepts of sensitivity, specificity, positive predictive value, and negative predictive value in the context of medical testing. It explains sensitivity as the ability of a test to correctly identify individuals with a disease, using the example of a rotator cuff tear in physical therapy. The paragraph also discusses how a highly sensitive test is useful for ruling out diseases when the result is negative. Specificity is then introduced as the ability to correctly identify individuals without the disease. The importance of understanding these metrics in clinical settings is emphasized, and the relationship between these metrics and the prevalence of disease is clarified.
π Calculation and Interpretation of Test Metrics
This paragraph delves into the calculation and interpretation of positive and negative predictive values. It uses a two-by-two table to illustrate how these values are derived from the number of true positives, false positives, true negatives, and false negatives. The paragraph explains that positive predictive value indicates the probability of having the disease when the test is positive, while negative predictive value indicates the probability of not having the disease when the test is negative. It also discusses how these values are related to the prevalence of the disease in the population. The paragraph concludes by emphasizing the practical use of these metrics in clinical settings, the importance of using multiple tests to increase diagnostic accuracy, and the caution required when applying test results to patients from different populations.
Mindmap
Keywords
π‘Sensitivity
π‘Specificity
π‘True Positives
π‘False Negatives
π‘True Negatives
π‘False Positives
π‘Positive Predictive Value (PPV)
π‘Negative Predictive Value (NPV)
π‘Prevalence
π‘Screening Tests
π‘Diagnostic Tests
Highlights
Introduction to medical test evaluation metrics.
Sensitivity defined as a test's ability to identify patients with the disease.
Example given of a rotator cuff tear and its identification through sensitivity.
Explanation of true positives and false negatives in the context of sensitivity.
High sensitivity tests are useful for ruling out diseases when the test is negative.
Sensitivity is independent of the disease's prevalence in the population.
Specificity defined as a test's ability to identify those without the disease.
Example of true negatives and false positives in the context of specificity.
Specific tests are good for ruling in diseases when the test is positive.
Mnemonic 'spin' for specific tests and 'snout' for sensitive tests for ruling in and out diseases.
Formula for calculating sensitivity given with an example.
Formula for calculating specificity given with an example.
Explanation of positive predictive value (PPV) and its calculation.
Explanation of negative predictive value (NPV) and its calculation.
PPV and NPV are related to the prevalence of the disease in the population.
Use of multiple tests in clinical practice to increase the likelihood of accurate diagnosis.
Recommendation to watch a video by Mark Campo PT PhD on YouTube for a better understanding of these concepts.
Final words on the importance of understanding the relationship between sensitivity, specificity, and predictive values in medical testing.
Transcripts
Browse More Related Video
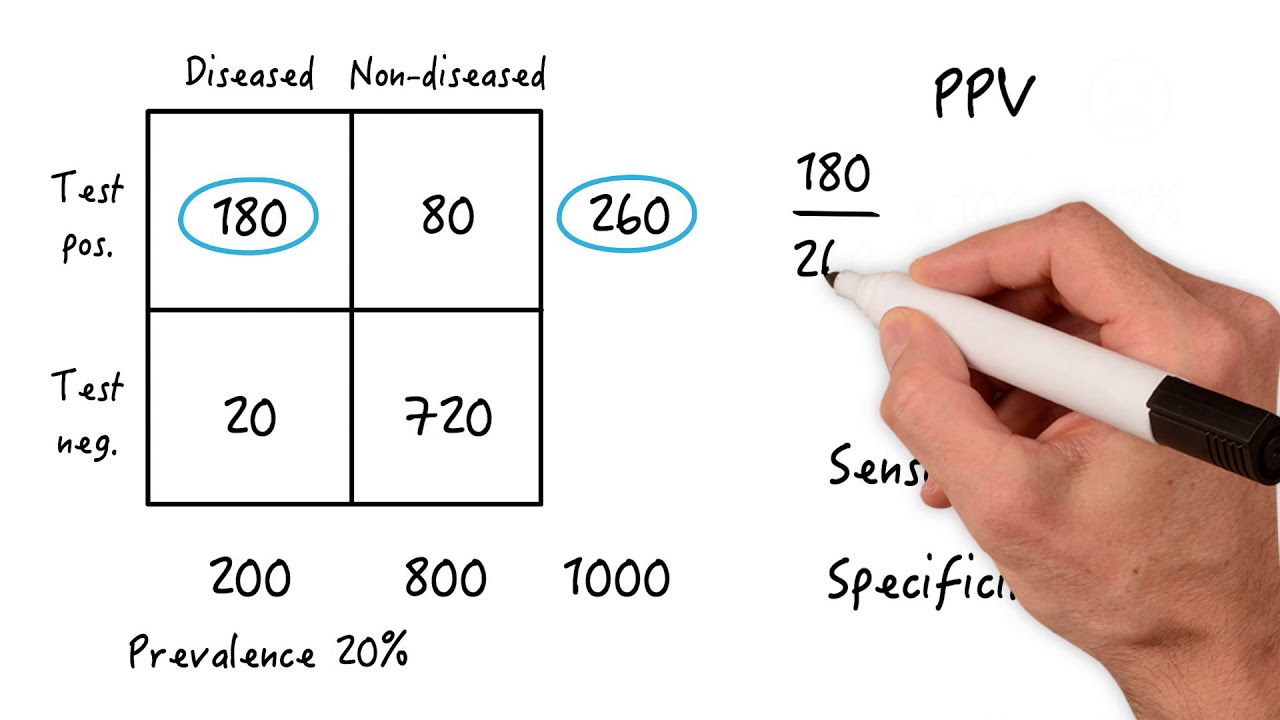
Positive Predictive Value & Disease Prevalence
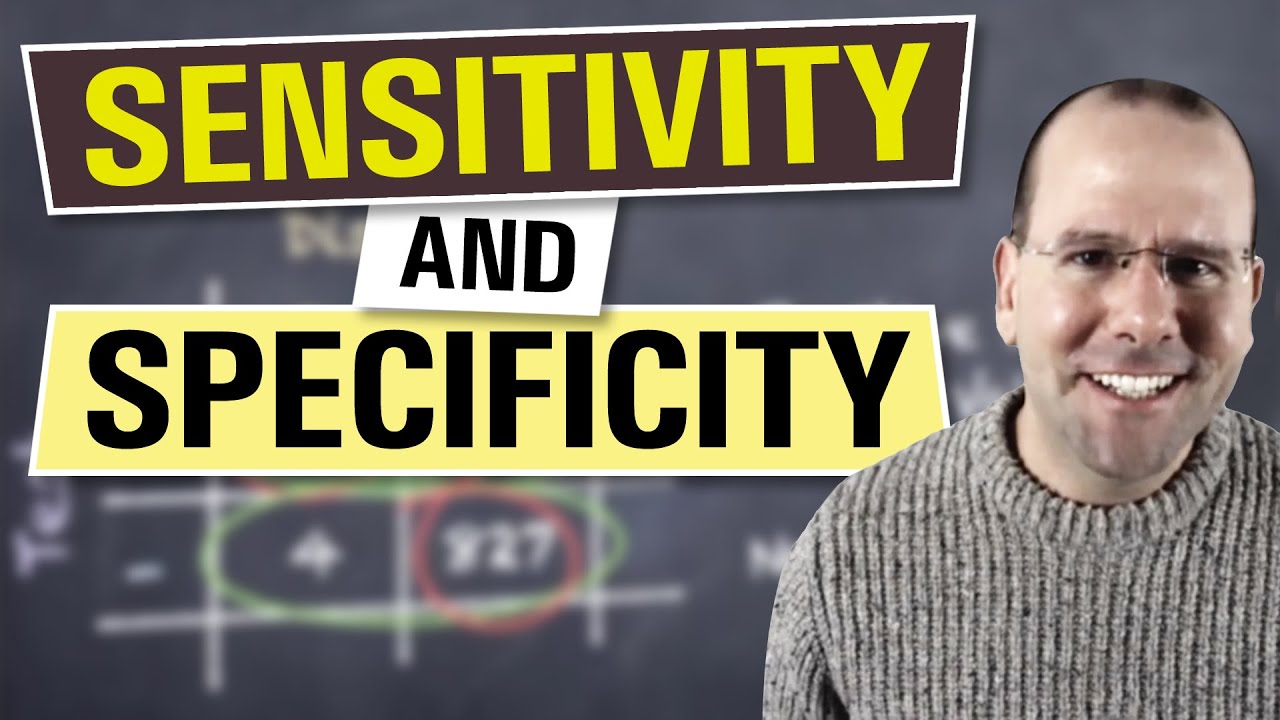
Sensitivity and specificity - explained in 3 minutes
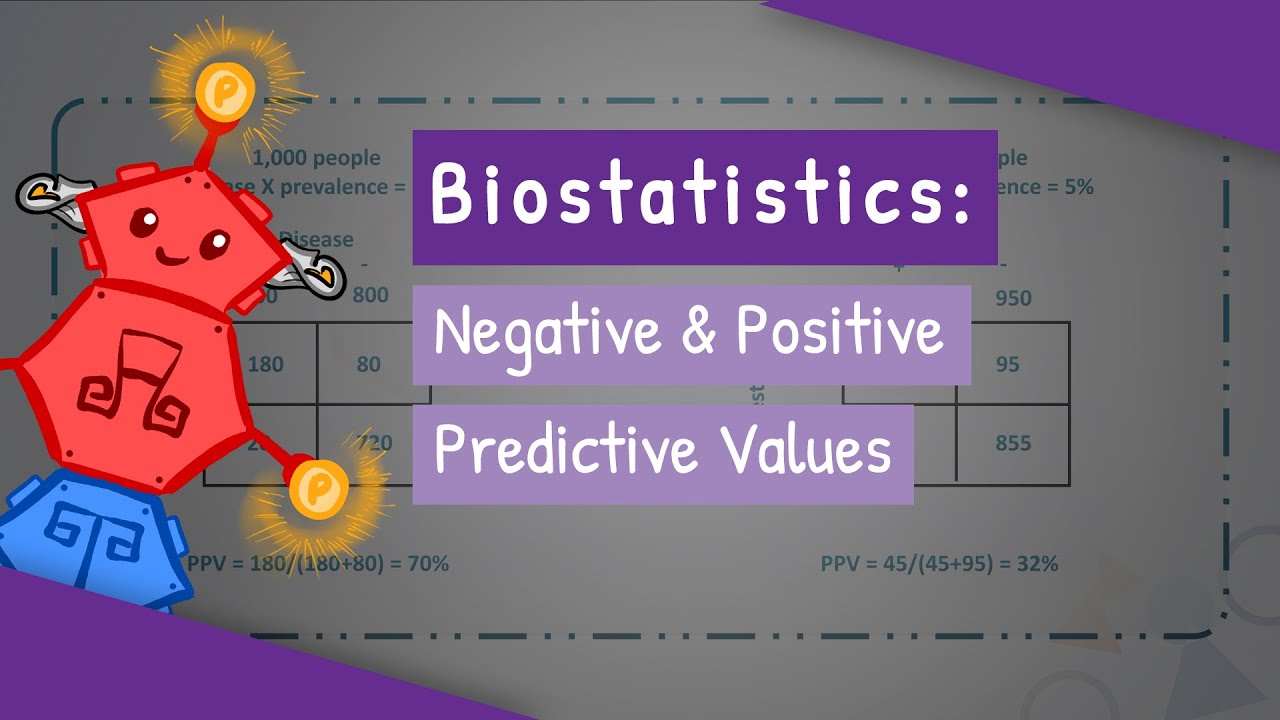
Biostatistics - Negative and Positive Predictive Values
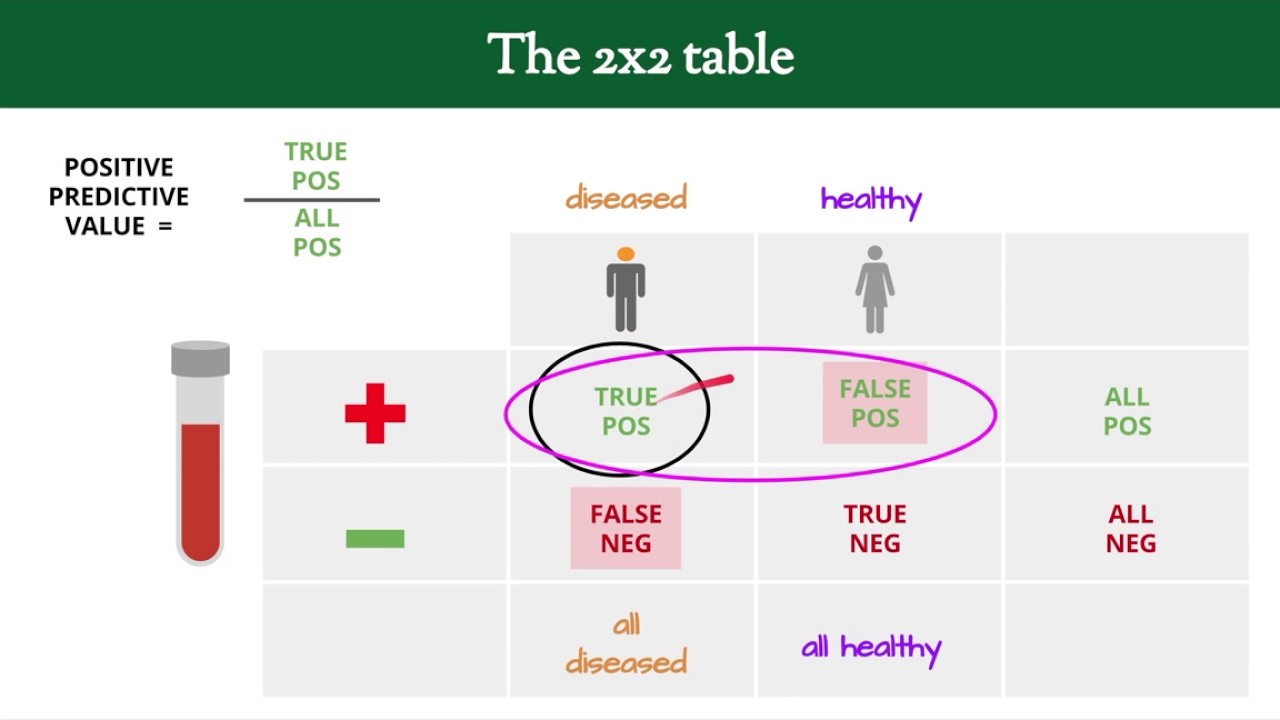
Clinical Reasoning 6: Sensitivity, Specificity & Predictive Values

Sensitivity, Specificity, Positive & Negative Predictive Value | Validity and Reliability of a Test
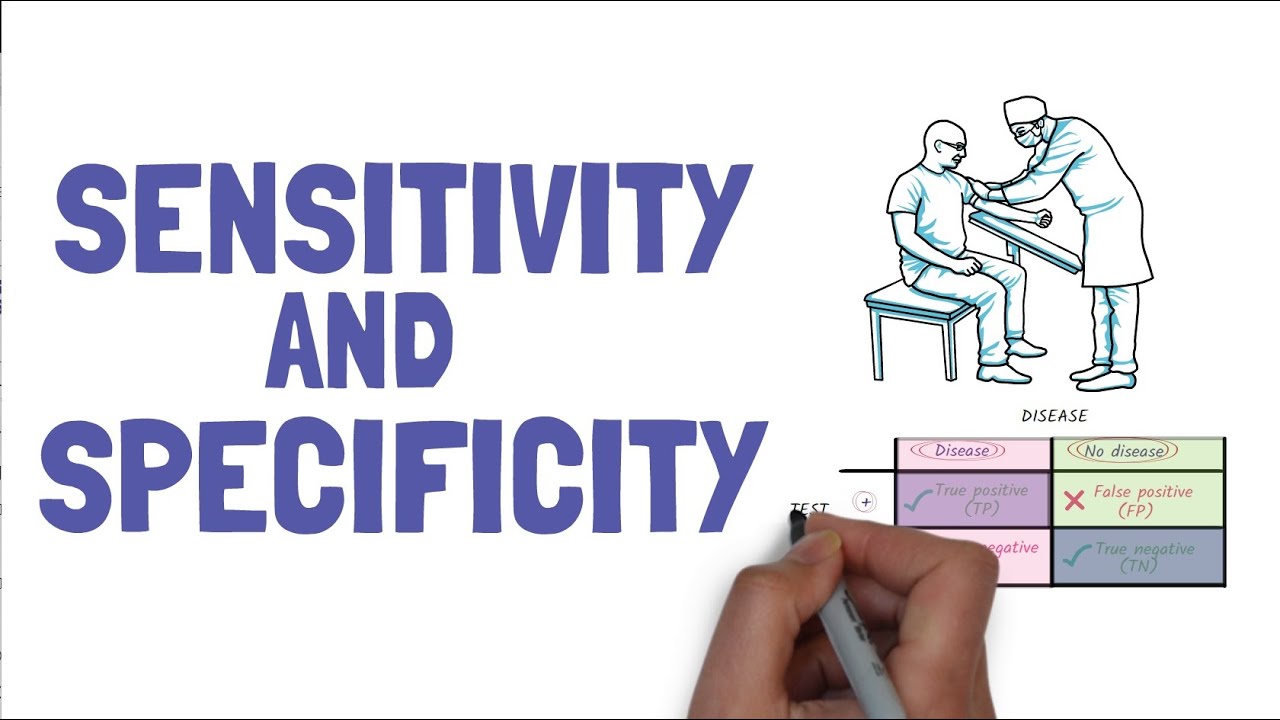
Sensitivity and Specificity simplified
5.0 / 5 (0 votes)
Thanks for rating: