Biostatistics - Negative and Positive Predictive Values
TLDRThis video script discusses the evaluation of diagnostic tests, focusing on the concepts of negative and positive predictive values. It explains that negative predictive value is the likelihood that a person with a negative test does not have the disease, while positive predictive value is the probability that a person with a positive test actually has the disease. The script emphasizes the importance of understanding these values in clinical settings, especially when interpreting test results for patients. It also introduces the concept of prevalence and its direct relationship with positive predictive value and inverse relationship with negative predictive value, using examples and logical reasoning to illustrate these points.
Takeaways
- π Negative Predictive Value (NPV) is the probability that a person with a negative test result does not have the disease.
- π NPV is calculated as the number of true negatives divided by the sum of true negatives and false negatives.
- π€ Understanding NPV is crucial for interpreting test results accurately, especially when a patient receives a negative test.
- π Clinical vignettes often test knowledge of NPV, where the physician must explain the meaning of a negative test result to a patient.
- π Positive Predictive Value (PPV) is the probability that a person with a positive test result actually has the disease.
- π PPV is calculated as the number of true positives divided by the sum of true positives and false positives.
- π The relationship between prevalence, PPV, and NPV is significant: prevalence is directly proportional to PPV and inversely proportional to NPV.
- π An increase in prevalence leads to a higher PPV but a lower NPV, which can be demonstrated through numerical examples or logical reasoning.
- π§ In populations with a low prevalence of a disease, a positive test result is less likely to indicate the actual presence of the disease.
- π The concepts of prevalence, sensitivity, specificity, PPV, and NPV are essential for understanding and evaluating diagnostic tests.
- π The video script serves as a comprehensive guide for medical professionals to better understand and communicate diagnostic test results to patients.
Q & A
What is the definition of negative predictive value (NPV)?
-Negative predictive value is the probability that a person with a negative test result actually does not have the disease. It is calculated as the true negatives divided by the sum of true negatives and false negatives.
How can you remember the formula for NPV?
-To remember the NPV formula, focus on the negatives: true negative over true negative plus false negative.
Why is understanding the definition of NPV important in clinical practice?
-Understanding NPV is crucial because it helps doctors interpret test results accurately, informing patients about the likelihood of not having a disease based on a negative test result.
What is positive predictive value (PPV)?
-Positive predictive value is the probability that a person with a positive test result actually has the disease. It is calculated as the true positives divided by the sum of true positives and false positives.
How does prevalence affect PPV and NPV?
-Prevalence is directly proportional to PPV and inversely proportional to NPV. As prevalence increases, PPV increases, and NPV decreases.
What is the relationship between prevalence and pretest probability?
-Pretest probability is the probability of having a disease before testing, and it is the same as prevalence. They both represent the likelihood of disease presence in a given population.
How do you calculate the pretest probability of a disease?
-You can calculate the pretest probability by using the prevalence of the disease in the population. If the prevalence is 10%, the pretest probability is also 10%.
In the example with two populations, why does the PPV differ between them?
-The PPV differs because the prevalence of the disease is different in each population. A higher prevalence leads to a higher proportion of true positives among all positives, thus increasing PPV.
What is the logical explanation for the relationship between prevalence and PPV/NPV?
-For a rare disease with low prevalence, a positive test result is less likely to be true, as there are fewer cases in the population. Conversely, for a common disease with high prevalence, a positive test result is more likely to be accurate, increasing PPV.
How does the specificity and sensitivity of a test affect PPV and NPV?
-In the given examples, specificity and sensitivity were held constant at 90%. Changes in PPV and NPV were due to changes in prevalence, not sensitivity or specificity. However, in different scenarios, varying sensitivity and specificity can also impact PPV and NPV.
What is the significance of the 90% rule used in the examples for sensitivity and specificity?
-The 90% rule is a simplification used in the examples to illustrate how sensitivity and specificity affect the calculation of PPV and NPV. It does not represent an actual clinical scenario but helps in understanding the concepts.
How can healthcare professionals apply the concepts of PPV and NPV in their practice?
-Healthcare professionals can use PPV and NPV to communicate the accuracy of diagnostic tests to patients, helping them understand the likelihood of having a disease based on test results and the prevalence of the disease in the population.
Outlines
π§ͺ Understanding Negative Predictive Value and Its Clinical Significance
This paragraph introduces the concept of negative predictive value (NPV) and its importance in clinical settings. NPV is defined as the probability that a person with a negative test result is truly free of the disease. The explanation emphasizes the need to distinguish between true negatives and false negatives. It also highlights the clinical scenario where a patient with a negative test result seeks clarification on the meaning of 'negative,' leading to the selection of NPV as the relevant statistical measure. The paragraph underscores the significance of prevalence in relation to predictive values, setting the stage for a deeper discussion on the topic in subsequent content.
π Calculating and Interpreting Positive Predictive Value in Different Populations
The second paragraph delves into the calculation and interpretation of positive predictive value (PPV) in different populations with varying prevalence rates. It begins by applying the 90% sensitivity and specificity rules to populations with different prevalence rates for a hypothetical disease X. The calculations demonstrate how PPV changes with prevalence: in a population with a 20% prevalence, the PPV is approximately 70%, whereas in a population with a 5% prevalence, the PPV drops to about 32%. The paragraph concludes with a logical explanation for the inverse relationship between prevalence and PPV, using the example of a rare disease. It also touches on the skepticism that might arise when a positive test result is obtained in a population with low disease prevalence.
Mindmap
Keywords
π‘Negative Predictive Value (NPV)
π‘True Negatives
π‘False Negatives
π‘Positive Predictive Value (PPV)
π‘True Positives
π‘False Positives
π‘Prevalence
π‘Pretest Probability
π‘Sensitivity
π‘Specificity
π‘Clinical Vignette
Highlights
Introduction to the second part of a series on evaluating diagnostic tests.
Explanation of negative predictive value (NPV) and its definition.
How to remember the NPV formula by focusing on 'negatives'.
The importance of understanding NPV in clinical practice when interpreting test results for patients.
The definition and calculation of positive predictive value (PPV).
The relationship between prevalence, PPV, and NPV, and how changes in prevalence affect these values.
The concept of prevalence and its impact on pretest probability.
Deriving pretest probability from the prevalence of a disease in a population.
Illustration of how prevalence affects PPV and NPV through examples of different populations.
Explanation of why PPV increases and NPV decreases with higher prevalence using both numerical and logical reasoning.
The practical implications of disease rarity on the interpretation of PPV.
The logical skepticism towards a positive test result in the context of a rare disease.
Conclusion and call to action for viewers to like and subscribe.
Transcripts
Browse More Related Video
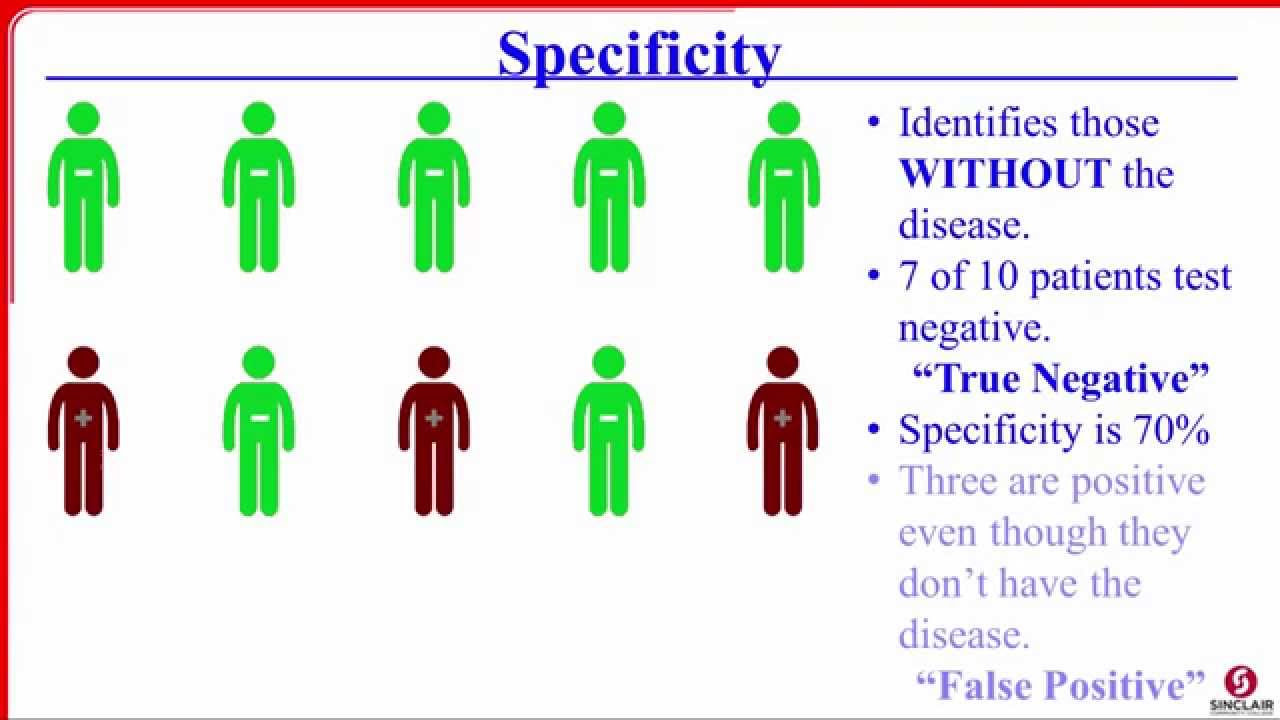
Sensitivity, Specificity, PPV, and NPV
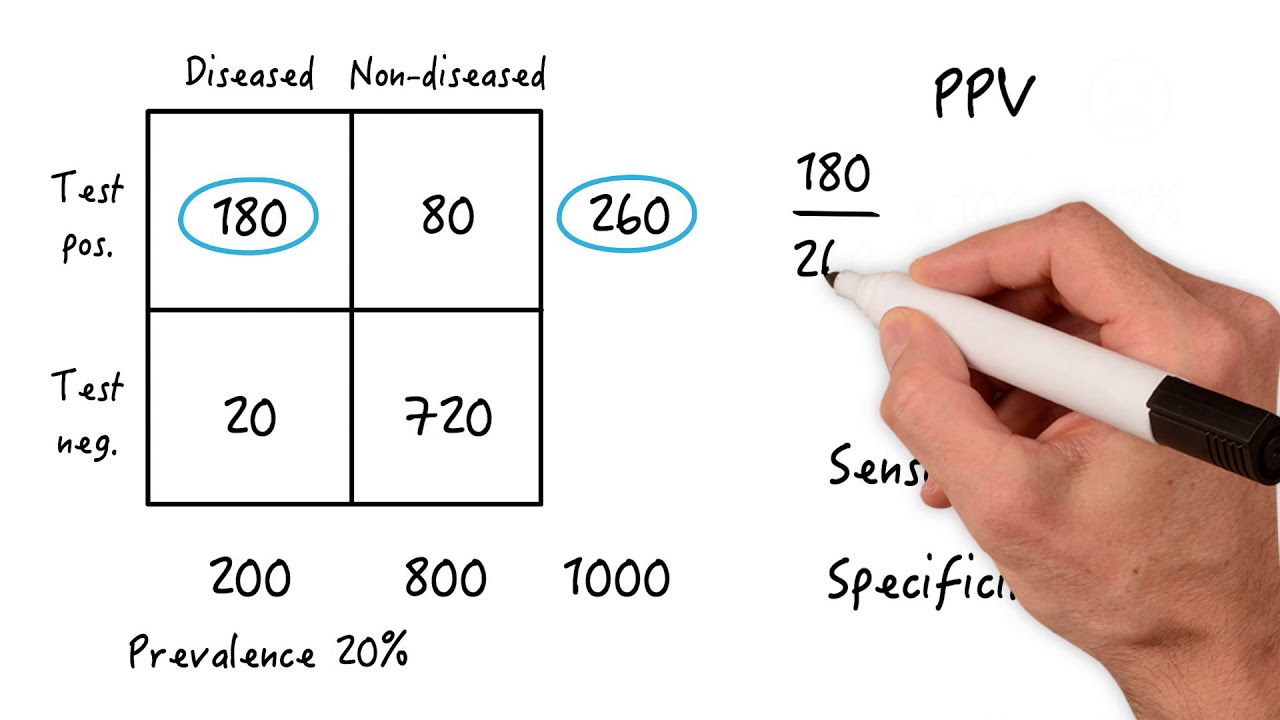
Positive Predictive Value & Disease Prevalence
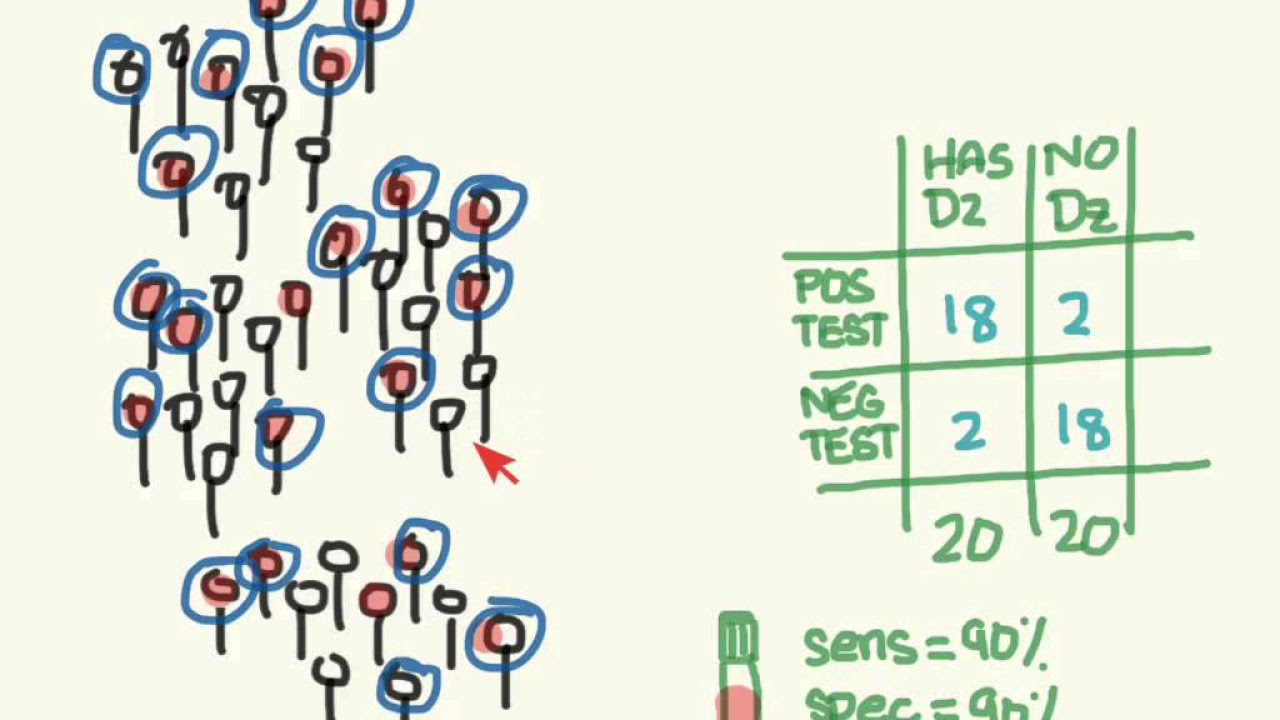
EBM: predictive values
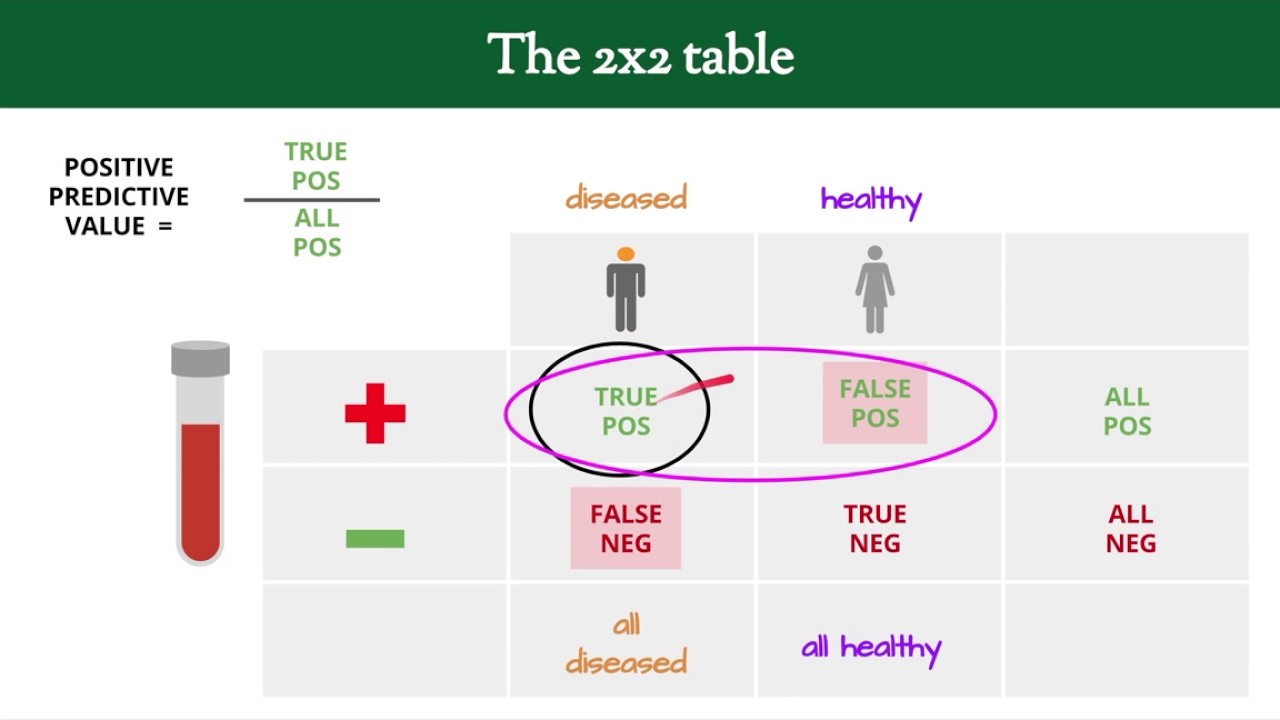
Clinical Reasoning 6: Sensitivity, Specificity & Predictive Values
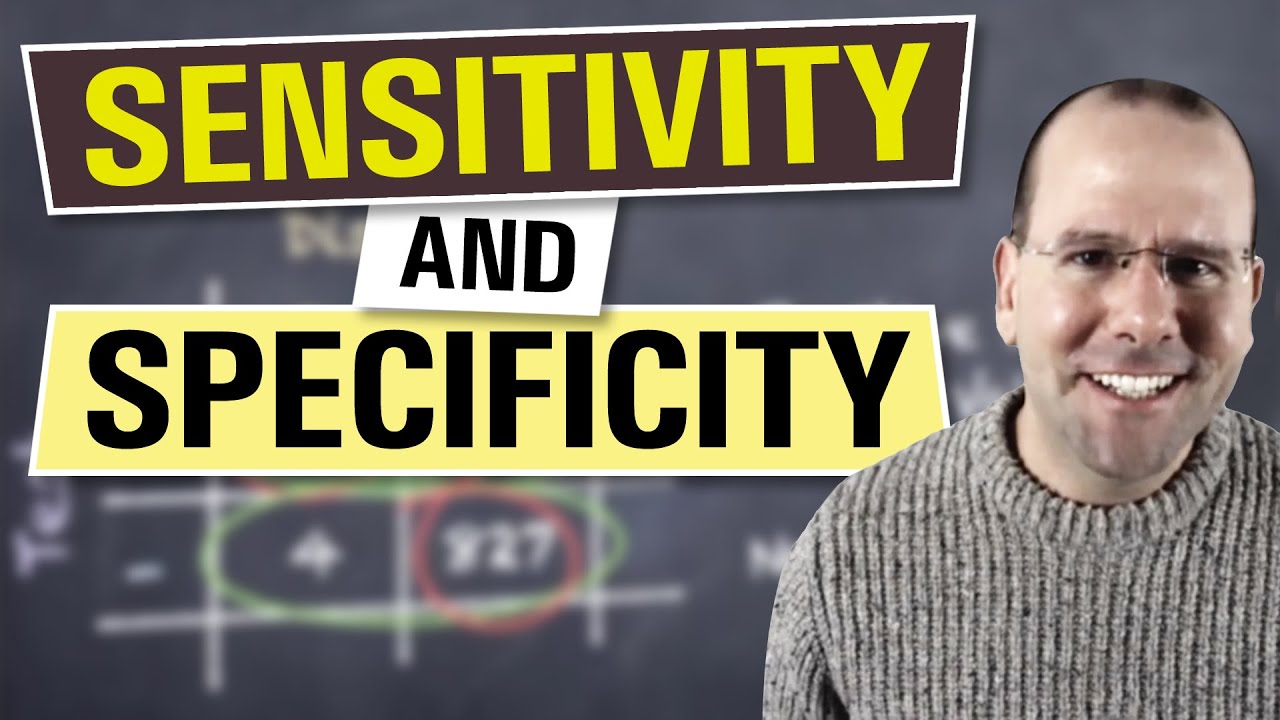
Sensitivity and specificity - explained in 3 minutes
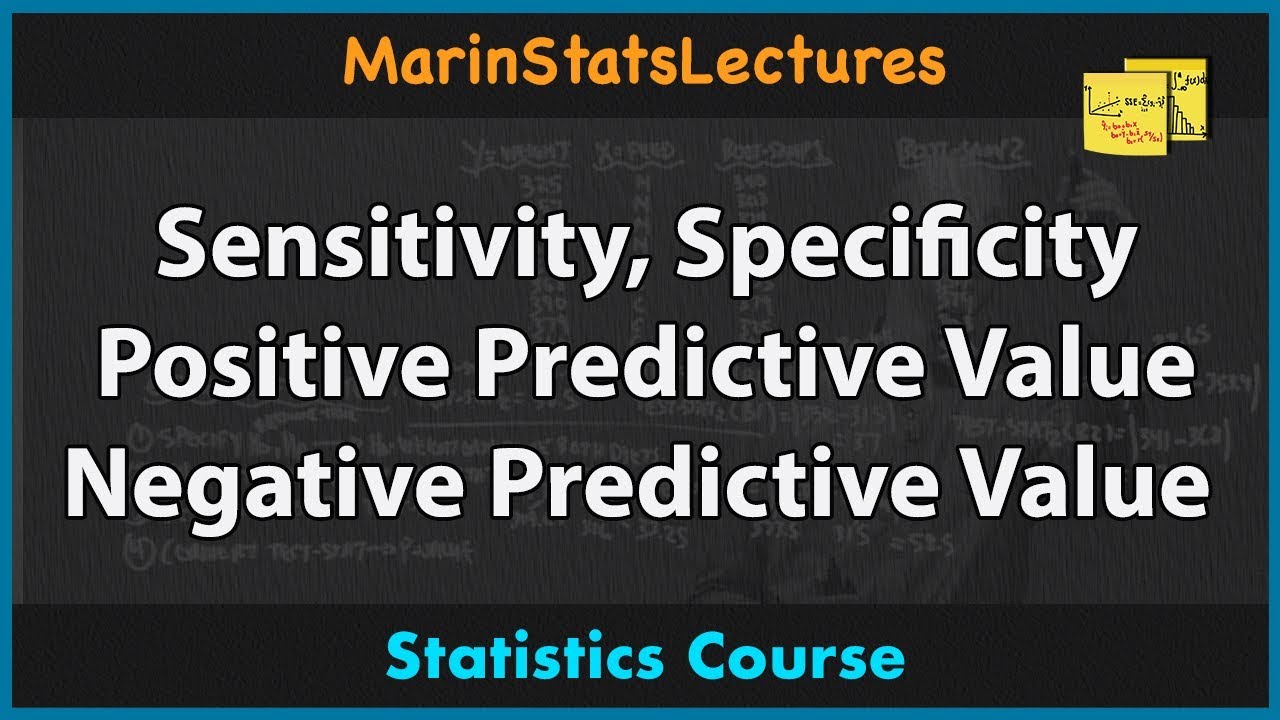
Sensitivity, Specificity, Positive and Negative Predictive Values | MarinStatsLectures
5.0 / 5 (0 votes)
Thanks for rating: