1. Introduction to Statistics
TLDRIn the introduction to MIT's 18.650 course, Professor Rigollet emphasizes the importance of understanding statistics for various real-life applications, including machine learning and decision-making. The course focuses on the mathematical foundations of statistical methods, aiming to equip students with the knowledge to apply statistical principles effectively. The professor discusses the difference between probability and statistics, the role of modeling, and the significance of assumptions in statistical analysis. The lecture also touches on the concept of estimators, the interpretation of data, and the critical thinking required to discern the validity of statistical findings.
Takeaways
- ๐ The course 18.650, Fundamentals of Statistics, aims to introduce students to statistical methods and theory without prior knowledge of statistics, but with an expectation of understanding probability.
- ๐ฏ The course focuses on theoretical guarantees and estimators, aiming to prepare students for more advanced applications and potentially machine learning classes.
- ๐๏ธ The course structure includes weekly homework assignments, two midterms, and a final exam, with a strong emphasis on problem-solving.
- ๐ The importance of statistics in real-life situations is highlighted, including its role in machine learning, scientific studies, and decision-making processes.
- ๐ซ The course does not cover statistical software implementation, but emphasizes the mathematical foundation behind statistical methods.
- ๐ Statistics is positioned as a tool for understanding and making sense of data, rather than just a collection of tests and formulas.
- ๐ค The course encourages critical thinking about statistical findings, questioning the validity and applicability of study results.
- ๐ง The concept of randomness and its quantification through probability is fundamental to understanding statistical models.
- ๐ The role of modeling in statistics is emphasized, with the acknowledgment that models simplify complex reality to make it manageable and understandable.
- ๐ The iterative process of hypothesis formulation, data collection, and model validation is discussed as a key part of the scientific method.
- ๐ The impact and prevalence of statistics in various fields such as insurance, clinical trials, and genetic studies are used to illustrate its broad applications.
Q & A
What is the main focus of the course 18.650: Fundamentals of Statistics?
-The main focus of the course is to provide an introduction to statistical methods, emphasizing the theoretical aspect and mathematical foundations rather than practical data analysis or software implementation.
What is the significance of the course title change from 'Statistics for Applications' to 'Fundamentals of Statistics'?
-The title change reflects a more accurate representation of the course content, which is centered on the fundamental principles of statistics rather than their application in specific fields.
What is the disclaimer the professor makes about his speaking speed?
-The professor acknowledges that he tends to speak too fast and is aware that it may be difficult for some students to follow. However, he intends to repeat himself often, ensuring that the key messages are communicated effectively.
What are the two main goals of the course?
-The two main goals are to introduce students to statistical methods and to enable them to formulate statistical problems in mathematical terms, preparing them for more advanced studies such as machine learning.
How does the professor plan to handle the use of real data in the course?
-The professor plans to focus more on theoretical guarantees and mathematical equations rather than on real data and statistical thinking, using standard applications to illustrate the principles of statistics.
What is the professor's expectation regarding the students' understanding of probability?
-The professor expects that the students would have seen some statistics in their probability courses and thus have some foundational ideas, but he will not assume any prior knowledge of statistics.
What is the policy for homework submissions in the course?
-Homework is due weekly, and students are allowed two late homeworks, submitted 24 hours late without any questions asked. Beyond that, a valid explanation is required for additional extensions.
How will the two midterm exams be handled in terms of grading?
-The best score of the two midterms will be kept, and this grade will count for 30% of the final grade. The midterms are closed-book and closed-notes.
What is the significance of the book 'All of Statistics' by Wasserman recommended in the course syllabus?
-The book is recommended for its broad coverage of statistical topics at an introductory-graduate level. It provides a good overview of the subject, even though it does not go into great depth on any single topic.
Why does the professor emphasize the importance of understanding the randomness in data?
-Understanding randomness is crucial because it allows for the development of statistical models that can make sense of data, predict outcomes, and inform decision-making processes despite the inherent uncertainties in the data.
Outlines
๐ Introduction to 18.650: Fundamentals of Statistics
The paragraph introduces the course 18.650, Fundamentals of Statistics, and its previous title, Statistics for Applications. The speaker, Professor Rigollet, explains the course's focus on theoretical guarantees and mathematical expectations in statistics, rather than real data and statistical thinking. He emphasizes the importance of understanding the principles of statistics to apply them to real-life situations, particularly at MIT. The course aims to prepare students for more advanced statistical and machine learning classes, with a strong emphasis on the limitations and potential errors of statistical methods.
๐ Course Logistics and Assessment Details
This paragraph outlines the course logistics, including the schedule for lectures and recitations, homework assignments, and exam dates. The professor discusses the expectations for attendance at recitations, the format for submitting homework, and the grading policy, which includes two midterms and a final exam. The paragraph also addresses the use of cheat sheets during midterms and the availability of past lectures on OCW and Stellar for review purposes.
๐ Prerequisites and Recommended Resources
The speaker emphasizes the prerequisite knowledge of probability and basic concepts of calculus and linear algebra for the course. He mentions that while there is no required textbook, problems and slides will be provided to aid learning. A recommended book for further reading is 'All of Statistics' by Wasserman. The paragraph also highlights the importance of understanding the scientific process and the role of statistics in various fields, using news headlines as examples of how statistics are applied in the real world.
๐ง Critical Evaluation of Statistical Findings
This section discusses the need for skepticism and critical evaluation of statistical findings, especially in light of the media's portrayal of studies. The speaker points out that individual results may not apply to everyone and that statistical significance does not always equate to practical significance. He uses examples of scientific studies and the potential for statistical errors, such as p-hacking and randomness, to illustrate the importance of understanding the assumptions and limitations behind statistical data.
๐งฌ Applications of Statistics in Various Fields
The speaker elaborates on the practical applications of statistics across different fields, including insurance, clinical trials, and genetics. He explains how statistical modeling is used to make predictions and decisions based on data, such as determining the height of dikes for flood protection or the effectiveness of a drug. The paragraph also touches on the challenges of handling data in adaptive data analysis and the potential for misuse of statistical methods, as exemplified by the 'salmon experiment' and John Oliver's discussion on p-hacking.
๐ฒ Understanding Randomness and Probability
This paragraph delves into the concepts of randomness and probability, explaining how they are fundamental to understanding and applying statistics. The speaker uses examples of dice rolls and coin flips to illustrate basic probability concepts, then extends these ideas to more complex scenarios, such as choosing a number in a dice game. The paragraph emphasizes the importance of simplifying assumptions to model complex processes and the role of the statistician in interpreting and making sense of data.
๐ The Cycle of Probability and Statistics
The speaker discusses the cyclical relationship between probability and statistics, where probability is used to predict outcomes based on known parameters, while statistics is used to infer the parameters from observed data. The paragraph contrasts the two fields by providing examples of questions that would be asked in each, highlighting the importance of understanding the underlying distributions and the need for accurate modeling to make reliable predictions and inferences.
๐จ The Art of Statistical Modeling
This section emphasizes the art of statistical modeling, which involves simplifying complex processes into simpler, manageable models. The speaker explains that a good model should be simple yet capture the essential aspects of the process being studied. He also discusses the importance of domain knowledge in building plausible models and the role of the statistician as a translator between the complexity of real-world problems and the simplicity of statistical models.
๐ Estimating Parameters and Understanding Data
The speaker introduces the concept of estimating parameters from data, using the example of observing kissing couples to estimate the proportion of couples who turn their heads to the right. He explains the process of defining a statistical experiment, collecting data, and using this data to estimate unknown parameters. The paragraph also touches on the importance of understanding the population that the data represents and the need to consider the sample size when making conclusions.
๐ Indicator Variables and Estimators
This paragraph discusses the use of indicator variables and the concept of an estimator in statistics. The speaker defines Ri as an indicator variable that takes the value 1 if the i-th couple turns their head to the right and 0 otherwise. He then explains how to use these indicators to estimate the parameter p, which is the proportion of couples kissing to the right. The speaker introduces the estimator p-hat as the sample proportion and discusses the difference between an estimator and an estimate.
๐งฎ Modeling Assumptions and Statistical Exercises
The speaker continues the discussion on modeling assumptions, focusing on the importance of assuming that observations are independent and identically distributed (IID). He uses the example of grading a test with 15 students to illustrate how to estimate the mean and variance of a larger population. The paragraph also includes an exercise to help students practice statistical concepts, emphasizing the need to replace expectations with averages in statistical calculations.
๐ฅ Supplementary Materials and Problem Set Guidance
In the final paragraph, the speaker mentions his intention to post instructional videos on statistical tables and other topics for students who may need a refresher. He also provides guidance on the first problem set, encouraging students to complete at least 15 out of 30 exercises and to review probability concepts from previous classes if needed. The speaker emphasizes the importance of understanding basic statistical principles and practices to succeed in the course.
Mindmap
Keywords
๐กStatistics
๐กProbability
๐กModeling
๐กRandomness
๐กEstimator
๐กIndependence
๐กBernoulli Distribution
๐กIID (Independent and Identically Distributed)
๐กStandard Deviation
๐กConfidence Interval
Highlights
The course 18.650, Fundamentals of Statistics, aims to introduce students to statistical methods and theory without prior knowledge of statistics, but with an expectation of understanding probability.
The instructor, Philippe Rigollet, emphasizes the importance of understanding the theoretical underpinnings of statistical methods and how they can be applied to real-world problems.
The course will focus on theoretical guarantees and the mathematical aspects of statistics, rather than practical data analysis or software implementation.
Students are expected to have a background in probability and some knowledge of calculus and linear algebra to fully engage with the course material.
The course syllabus includes a variety of topics such as error bars, statistical estimators, and the relationship between statistics and machine learning.
Rigollet discusses the evolution of statistics into machine learning and the goal of preparing students for more advanced statistical machine learning classes.
The grading system for the course includes weekly problem sets, two midterms, and a final exam, with the opportunity for students to have two late homeworks without penalty.
The course does not have a required textbook, but Rigollet recommends 'All of Statistics' by Wasserman for its broad overview and accessibility.
Rigollet highlights the relevance of statistics in various fields, including news reporting, scientific studies, and everyday decision-making, emphasizing its importance in modern life.
The course aims to equip students with the ability to formulate statistical problems in mathematical terms and to understand the limitations of statistical methods.
Rigollet discusses the concept of 'p-hacking' and the issues with scientific research incentives, which can lead to incorrect conclusions based on statistical analysis.
The importance of understanding randomness and the role of probability in statistical modeling is emphasized, as it is key to making sense of data and drawing accurate conclusions.
The course will cover the mathematical tools necessary for statistical analysis, including concepts like independence, identical distribution, and the central limit theorem.
Rigollet uses the example of a study on couples kissing to illustrate the importance of proper sampling and the potential biases in statistical data collection.
The course will not focus on t-tests or similar specific statistical tests, but rather on the foundational understanding of statistics and its principles.
Rigollet encourages students to think critically about the models and assumptions used in statistical analysis, and to be aware of the potential for model errors and biases.
Transcripts
Browse More Related Video

How Hard Is Statistics? (My Statistics Degree)

Chapter 1 - An Intro to Business Statistics
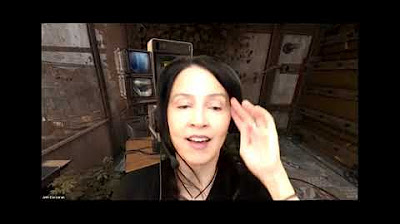
Mathematical Statistics, Lecture 1
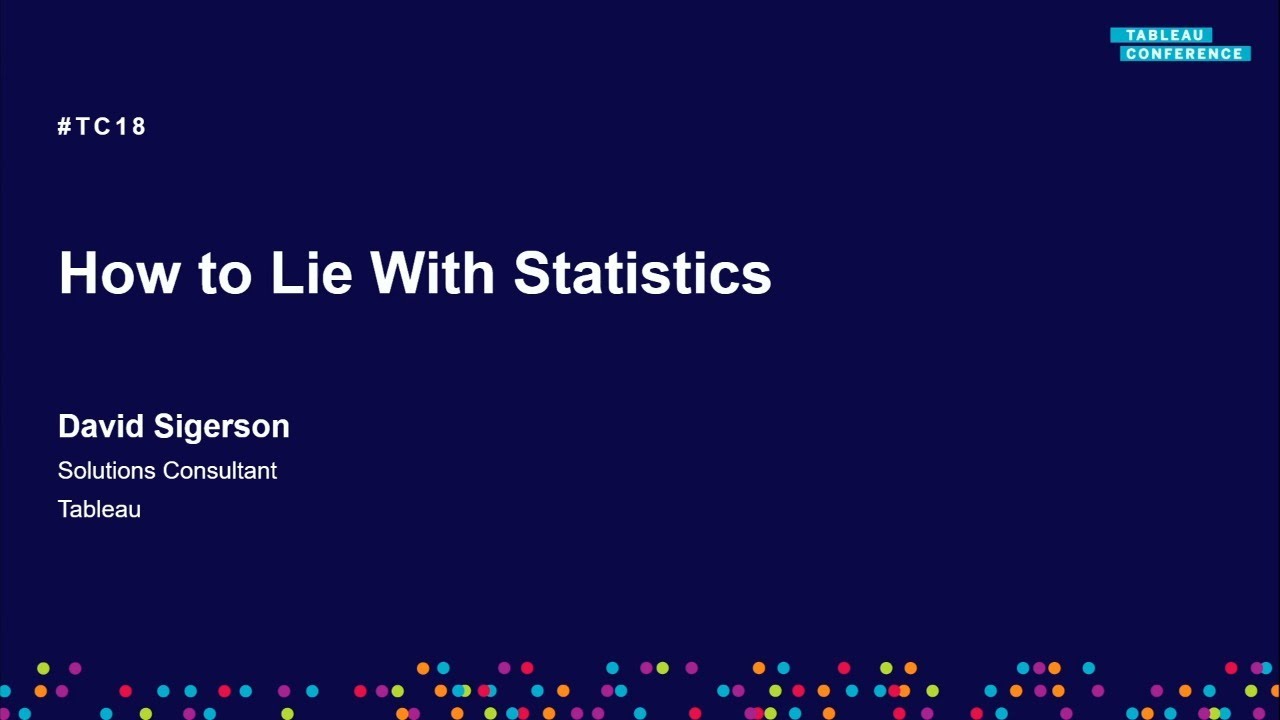
How to lie with statistics

Descriptive Statistics | What is Descriptive Statistics ? | Mean, Median & Mode | Great Learning
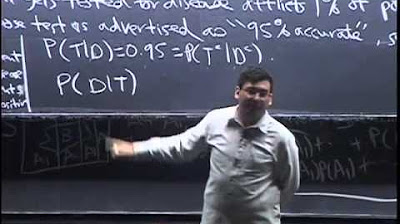
Lecture 5: Conditioning Continued, Law of Total Probability | Statistics 110
5.0 / 5 (0 votes)
Thanks for rating: