Statistical POWER and Power Analysis
TLDRThis video script delves into the concept of statistical power in inferential statistics, emphasizing the importance of avoiding type 1 and type 2 errors. It explains type 1 errors as incorrect rejections of the null hypothesis and type 2 errors as failing to reject a false null hypothesis. The script highlights the role of p-values in determining significance and the common threshold of 0.05 for alpha. To increase power and reduce the chance of type 2 errors, it suggests strategies like homogeneous experimental units, distinct treatment levels, and larger sample sizes. Power analysis is introduced as a crucial tool for determining sample size and justifying it in grant applications, with the goal of achieving at least 80% power. The script encourages the use of statistical packages for power analysis and suggests literature reviews or pilot studies for informed estimates.
Takeaways
- π§ The video discusses the concept of 'statistical power' in the context of making decisions about populations based on sample data.
- π€ It introduces the idea of making mistakes in inferential statistics, specifically 'Type 1' and 'Type 2' errors.
- π A 'Type 1 error' occurs when one incorrectly rejects the null hypothesis, believing there is a difference when there isn't.
- π Conversely, a 'Type 2 error' happens when one fails to reject the null hypothesis and misses a real difference between populations.
- π The speaker explains that p-values are used to measure the probability of committing a Type 1 error, typically set at a 5% threshold.
- πͺ 'Power' is defined as the probability of correctly rejecting the null hypothesis when there is a true effect (1 minus the probability of a Type 2 error).
- π To increase statistical power and reduce the chance of a Type 2 error, one can improve experimental design, such as using homogeneous units or larger treatment differences.
- π’ Increasing the sample size also boosts statistical power, which is crucial for detecting true effects.
- π Power analysis is essential for determining the required sample size to detect an effect of a certain size with confidence.
- π It's often a requirement in grant applications, especially when the study involves animals or human subjects, to justify the proposed sample size.
- π¬ Conducting a power analysis can also help in deciding whether a study is feasible based on the required sample size to detect the desired effect.
Q & A
What is the main topic discussed in the script?
-The main topic discussed in the script is statistical power and its relation to making decisions in inferential statistics, specifically focusing on type 1 and type 2 errors.
What are the two types of mistakes that can be made in inferential statistics?
-The two types of mistakes that can be made in inferential statistics are type 1 error (incorrectly rejecting the null hypothesis when there is no difference) and type 2 error (incorrectly failing to reject the null hypothesis when there is a difference).
What is a type 1 error?
-A type 1 error occurs when you decide that two populations are different when in reality there is no difference. It is made by rejecting the null hypothesis when it is actually true.
What is a type 2 error?
-A type 2 error occurs when you decide that there is no difference between two populations when in fact there is a difference. It is made by failing to reject the null hypothesis when it is false.
What is the p-value in the context of statistical tests?
-The p-value is a conditional probability that measures the probability of observing the difference between two samples, or a more extreme difference, just by chance alone, given that the populations are actually not different. It helps in determining the likelihood of committing a type 1 error.
What is the commonly accepted threshold for the p-value to reject the null hypothesis?
-The most commonly accepted threshold for the p-value is 0.05, or a 5% chance of committing a type 1 error, also known as alpha.
What is statistical power in the context of hypothesis testing?
-Statistical power is the probability of correctly rejecting the null hypothesis when there is a true difference between populations. It is calculated as 1 minus beta (1 - Ξ²).
How can one increase their statistical power?
-One can increase their statistical power by improving experimental design, such as using homogeneous experimental units, choosing treatment levels that are more different from each other, and increasing the sample size or the number of experimental or observational units.
What is power analysis and why is it important?
-Power analysis is used to determine the sample size required to detect a difference or effect of a given size with a certain amount of confidence. It is important because it helps in justifying sample size in grant applications, especially when using animals or human subjects, and it can inform whether a study is worth pursuing based on the required sample size.
What are the five pieces of information needed to perform a power analysis?
-The five pieces of information needed to perform a power analysis are sample size, magnitude of the difference between populations or effect size, the amount of natural or random variation within the population, the acceptable level of type one error (alpha), and power.
What is a recommended approach for estimating variance when performing power analysis?
-A recommended approach for estimating variance when performing power analysis is to conduct a literature search to find information about what you hope to measure or to conduct a small pilot study to collect preliminary data that can inform your larger study.
Outlines
π Understanding Statistical Power and Errors
This paragraph introduces the concept of statistical power and the potential for making mistakes in inferential statistics. It explains the two types of errors: Type 1 error, which occurs when incorrectly rejecting the null hypothesis and claiming a difference where there is none (significance level, alpha, is commonly set at 0.05); and Type 2 error, which happens when failing to reject the null hypothesis when there actually is a difference (beta represents the probability of this error). The paragraph also discusses the importance of p-values, which measure the probability of observing a difference due to chance if there is no actual difference in populations. Increasing statistical power, which is 1 minus beta, reduces the chance of committing a Type 2 error. Strategies to increase power include experimental design, homogeneous units, different treatment levels, and increasing sample size. A power of 80% or more is generally recommended for experimental design.
π The Importance of Power Analysis in Research
This paragraph delves into the importance and application of power analysis in research. Power analysis is crucial for determining the necessary sample size to detect a given effect size with confidence. It is often a requirement in grant applications, especially when the study involves animals or human subjects, to justify the proposed sample size. Power analysis can also help researchers decide whether a study is feasible based on the required sample size. The process involves understanding the relationship between sample size, effect size, variability within the population, the acceptable type 1 error rate (alpha), and power. Researchers are advised to use statistical software for power analysis and may need to estimate certain parameters like variance, possibly through literature review or pilot studies. The paragraph also encourages viewers to watch an example of power analysis for a two-sample t-test and subscribe for more educational content.
Mindmap
Keywords
π‘Statistical Power
π‘Type 1 Error
π‘Type 2 Error
π‘Null Hypothesis
π‘P-value
π‘Beta
π‘Sample Size
π‘Power Analysis
π‘Effect Size
π‘Alpha
π‘Experimental Design
Highlights
Introduction to the concept of power in statistical analysis
Explaining the inevitability of making mistakes in inferential statistics
Definition and explanation of Type 1 error
Clarification on when Type 1 errors occur
Definition and explanation of Type 2 error
Conditions under which Type 2 errors occur
Importance of avoiding mistakes in research
Introduction to p-values and their significance
Explanation of p-values as conditional probabilities
Commonly accepted Type 1 error rate (alpha)
How researchers use p-values to protect against Type 1 errors
Introduction to the concept of power and beta
Strategies to increase statistical power
Role of experimental design in maximizing power
Importance of sample size in increasing power
Recommendation for a minimum of 80% power in experimental design
Explanation of power analysis and its purpose
Necessity of power analysis in grant applications
Use of power analysis to determine feasibility of a study
Components needed for power analysis and their interrelation
Recommendation to use statistical packages for power analysis
Suggestion to conduct a literature search or pilot study for preliminary data
Invitation to view an example of power analysis for a two-sample t-test
Encouragement to subscribe for more informative videos
Transcripts
Browse More Related Video
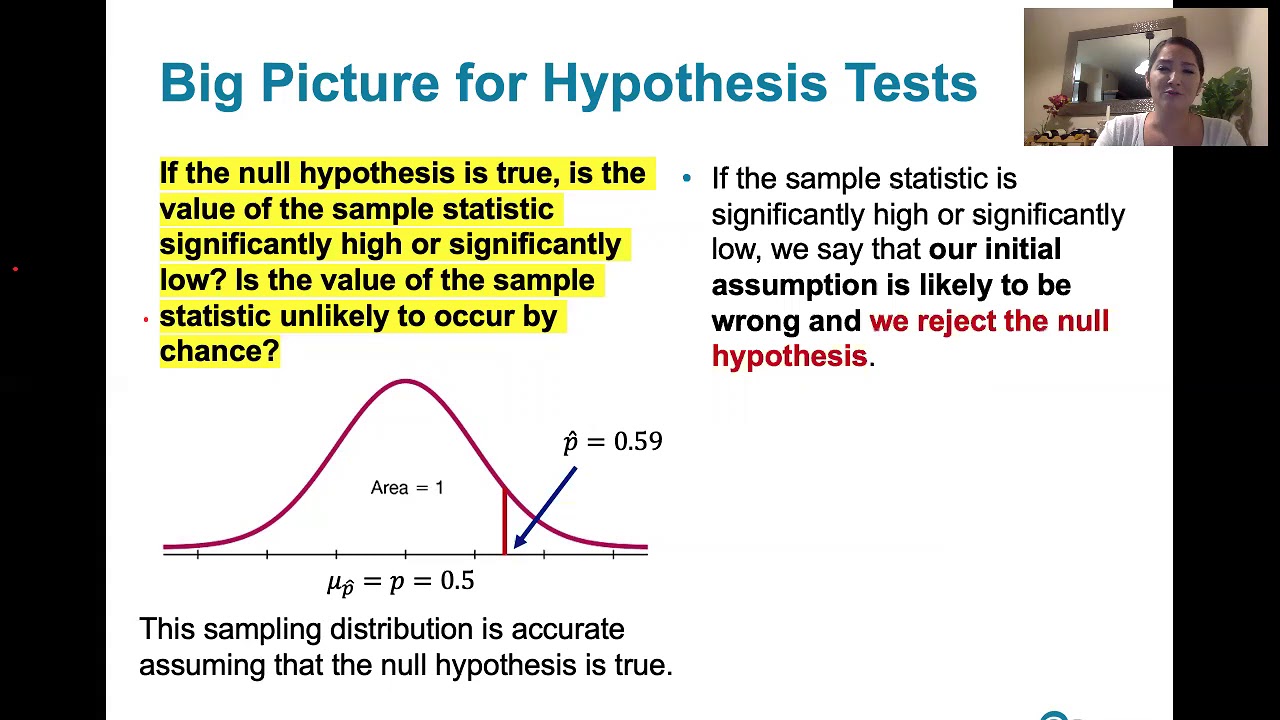
8.1.2 Basics of Hypothesis Testing - Significance Level, Type I and Type II Error
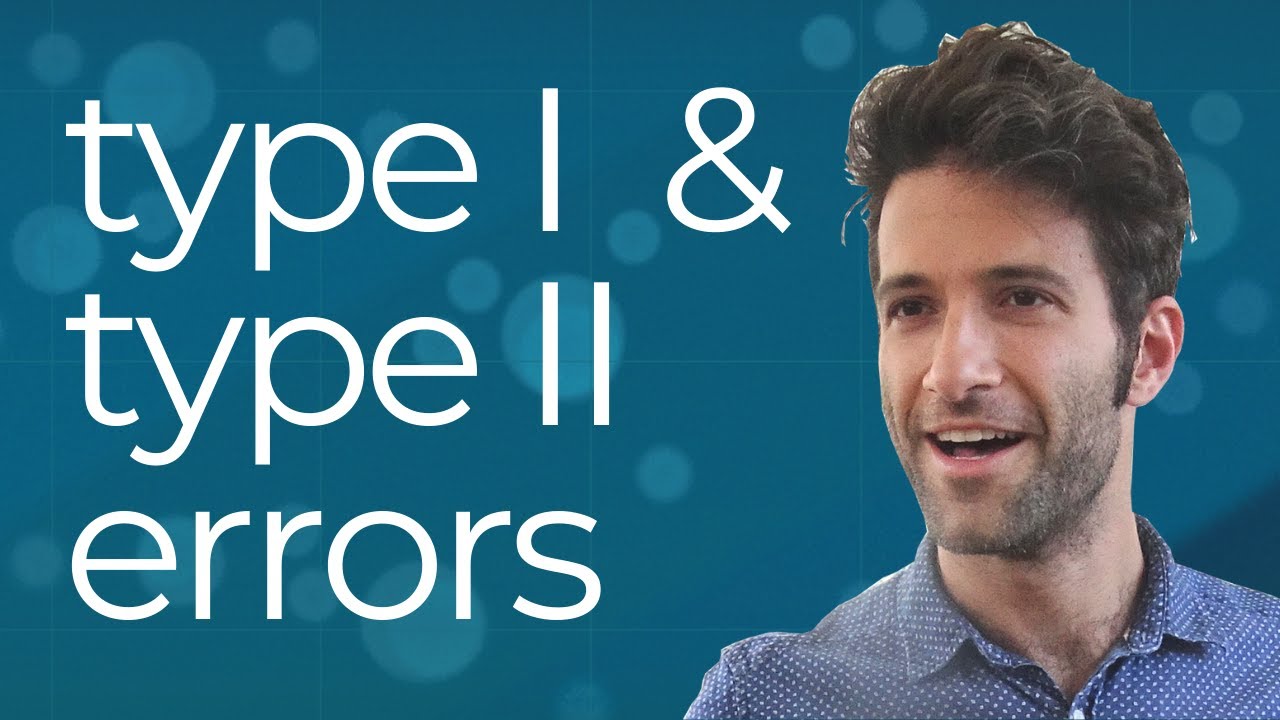
HYPOTHESIS TESTING BASICS: Type 1/Type 2 errors | Statistical power
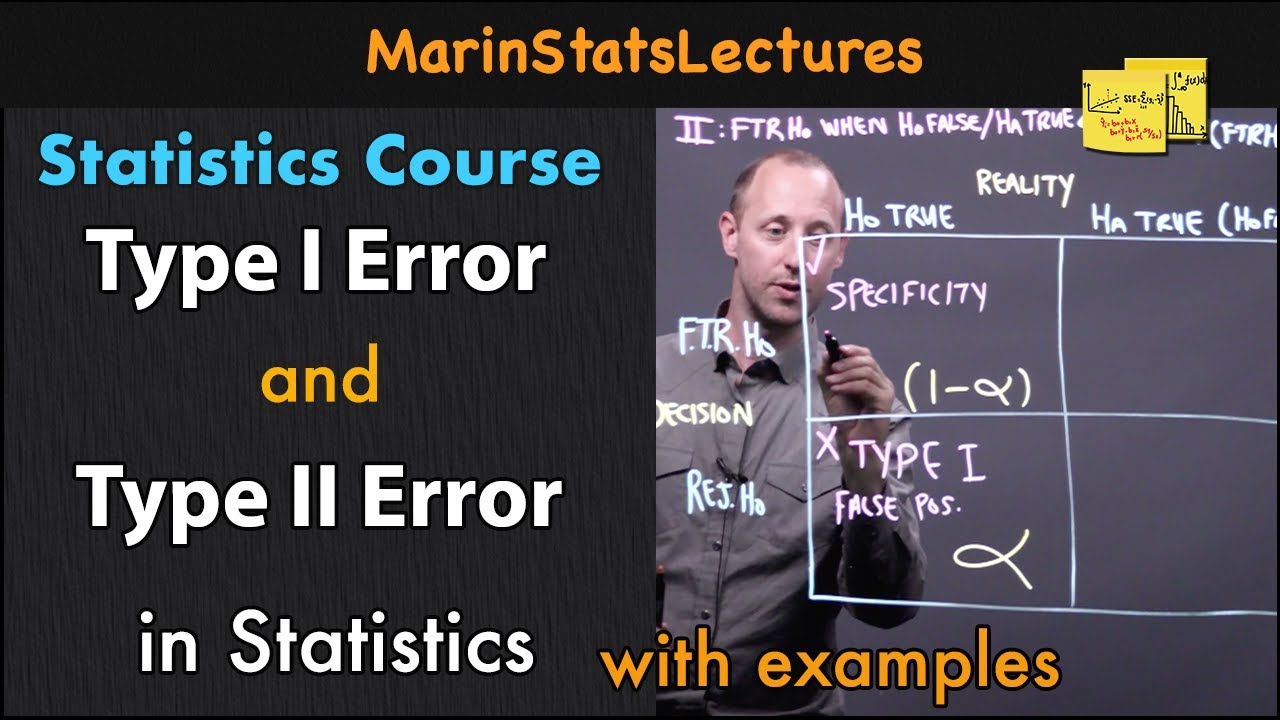
Errors and Power in Hypothesis Testing | Statistics Tutorial #16 | MarinStatsLectures

Power Analysis
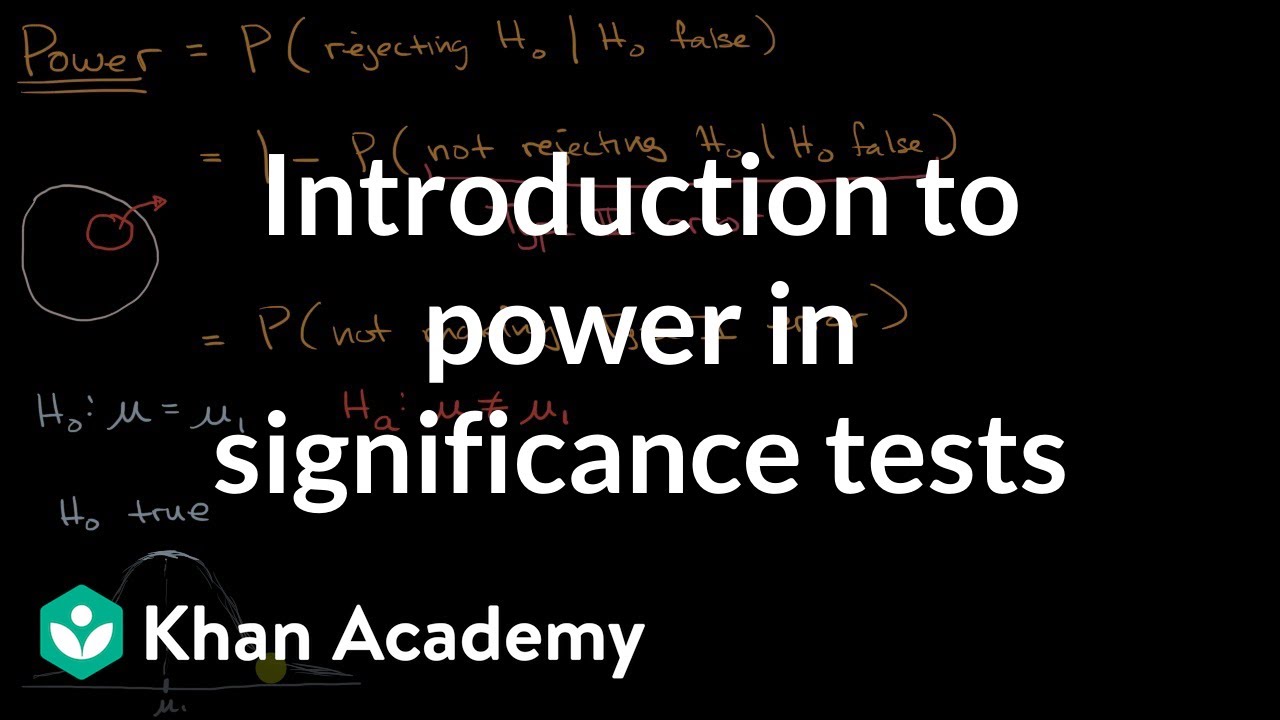
Introduction to power in significance tests | AP Statistics | Khan Academy

How To Identify Type I and Type II Errors In Statistics
5.0 / 5 (0 votes)
Thanks for rating: