Kruskal-Wallis-Test (Simply explained)
TLDRThe video tutorial introduces the Kruskal-Wallis test, a non-parametric alternative to the analysis of variance (ANOVA) used when data does not meet the assumptions of normal distribution. It explains that unlike ANOVA, which compares means, the Kruskal-Wallis test compares the rank sums of groups. The video outlines the test's assumptions, which include having independent random samples with ordinally scaled characteristics. It demonstrates how to calculate the test by assigning ranks to data points, calculating rank sums and mean rank sums for each group, and then using these values to compute the test statistic 'H'. The tutorial also guides viewers on how to interpret the results using a critical chi-squared value and shows how to perform the test online using DataTab, a tool that calculates the test statistic and provides a p-value for significance testing.
Takeaways
- ๐ The Kruskal-Wallis test is a non-parametric method used to determine if there are statistically significant differences between two or more groups.
- ๐ It is an alternative to the Analysis of Variance (ANOVA) when the data does not meet the assumptions of normal distribution.
- โ๏ธ Unlike ANOVA, which compares means, the Kruskal-Wallis test compares the rank sums of the groups.
- ๐ The test involves assigning a rank to each data point and then calculating the rank sum for each group.
- ๐ฏ The null hypothesis states that there is no difference in the rank sums among the groups, suggesting they come from the same population.
- ๐ The alternative hypothesis is that at least one group differs in rank sums, indicating it comes from a different population.
- ๐ To calculate the test, you need to know the number of cases, the mean rank sums of the groups, the expected value of the ranks, and the variance of the ranks.
- ๐งฎ The test statistic (H) is calculated using the formula provided in the script, which is similar to a chi-square value.
- ๐ The critical chi-square value is used to determine if the null hypothesis should be rejected based on the significance level and degrees of freedom.
- ๐ The online tool DataTab can be used to easily calculate the Kruskal-Wallis test by entering the relevant data.
- ๐ DataTab provides a p-value, which, when compared to the significance level, helps in making a decision about the null hypothesis.
- ๐ The interpretation of the test results is straightforward: if the p-value is greater than the significance level, the null hypothesis is not rejected, indicating no significant difference between the groups.
Q & A
What is the Kruskal-Wallis test used for?
-The Kruskal-Wallis test is used to determine if there are statistically significant differences between the central tendencies of three or more independent groups. It is a non-parametric alternative to the one-way ANOVA and is used when the data do not meet the normality assumption required for ANOVA.
When should you choose the Kruskal-Wallis test over ANOVA?
-You should choose the Kruskal-Wallis test over ANOVA when your data are not normally distributed, or when the assumptions necessary for ANOVA, such as homogeneity of variances, are not met.
What does the Kruskal-Wallis test compare?
-Unlike ANOVA that compares the means of the groups, the Kruskal-Wallis test compares the rank sums of the groups. It assesses whether the distribution of ranks differs significantly across the groups.
How are data ranked in the Kruskal-Wallis test?
-In the Kruskal-Wallis test, all data points across all groups are ranked together from smallest to largest. Each data point is assigned a rank based on its size relative to the others, starting with the smallest value assigned rank one.
What is the null hypothesis for the Kruskal-Wallis test?
-The null hypothesis for the Kruskal-Wallis test is that the central tendency (median) of all groups is equal, meaning that there is no significant difference in the rank sums among the groups.
What are the assumptions of the Kruskal-Wallis test?
-The Kruskal-Wallis test assumes that each group is an independent random sample and the data should be at least ordinal. Unlike parametric tests, it does not require the data to follow a normal distribution.
How is the Kruskal-Wallis test statistic calculated?
-The Kruskal-Wallis test statistic is calculated using the ranks of the data. Each group's mean rank is compared to the overall mean rank, and these comparisons are used to calculate the H statistic, which follows a chi-square distribution under the null hypothesis.
What does a significant Kruskal-Wallis test indicate?
-A significant Kruskal-Wallis test indicates that at least one of the groups significantly differs in its central tendency compared to the others. It suggests that not all groups come from the same distribution.
Can you use the Kruskal-Wallis test for data with more than three groups?
-Yes, the Kruskal-Wallis test can be used for data involving three or more groups. It is flexible in handling any number of groups as long as the other assumptions of the test are met.
How do you interpret the results of the Kruskal-Wallis test?
-To interpret the results of the Kruskal-Wallis test, compare the calculated H statistic to the critical value from the chi-square distribution at the desired level of significance. If the H statistic is greater than the critical value, reject the null hypothesis, indicating a significant difference between the groups.
Outlines
๐ Introduction to the Kruskal-Wallis Test
This paragraph introduces the Kruskal-Wallis test, a non-parametric statistical test used to determine if there are statistically significant differences between two or more independent groups. It explains that the test is an alternative to the one-way ANOVA when the data does not meet the assumptions of normality. The key concept explained is that instead of comparing means, the Kruskal-Wallis test compares the rank sums of the groups. The paragraph outlines the test's assumptions, which include having independent random samples with at least ordinally scaled characteristics and not requiring a specific distribution for the data. It also sets up the scenario for the test's application, mentioning an example of comparing reaction times across three groups.
๐ข Calculating the Kruskal-Wallis Test
This paragraph details the process of calculating the Kruskal-Wallis test. It begins by assigning ranks to each observation, then calculating the rank sum and mean rank sum for each group. The expected value of the rank sums is also discussed, which would be equal if there were no difference between the groups. The paragraph continues with the calculation of the test statistic 'H', which is analogous to the chi-square value, using the formula provided. It explains the components needed for the calculation, such as the number of cases, mean rank sums, expected value of ranks, and variance of ranks. The critical chi-square value is then used to determine whether to reject the null hypothesis, with an example calculation resulting in a non-significant H value leading to the retention of the null hypothesis. The paragraph concludes with a demonstration of how to perform the test online using a tool like datatab.net.
๐ Online Calculation and Interpretation of the Kruskal-Wallis Test
The final paragraph demonstrates how to calculate the Kruskal-Wallis test online using datatab.net. It guides the user through entering data into the platform, selecting the appropriate variables, and obtaining the test's results, including the chi-square value, degrees of freedom, and p-value. The interpretation of the results is also provided, with an example showing no significant difference between categories based on the p-value obtained. The paragraph encourages the viewer to try the process themselves and concludes the tutorial with a farewell note.
Mindmap
Keywords
๐กKruskal-Wallis Test
๐กNon-parametric
๐กRank Sum
๐กNormal Distribution
๐กHypothesis Testing
๐กDatatab.net
๐กDegrees of Freedom
๐กVariance
๐กTest Statistic (H value)
๐กSignificance Level
๐กData Entry
Highlights
The Kruskal-Wallis test is a non-parametric method used to determine if there are statistically significant differences between two or more groups.
It is an alternative to the one-way ANOVA when data do not meet the assumptions of normality.
The test compares the rank sums of the groups rather than their means.
Ranks are assigned to all individuals in the dataset, regardless of the group they belong to.
The Kruskal-Wallis test does not require data to follow a specific distribution.
The null hypothesis states that there is no difference in the rank sums among the groups.
The alternative hypothesis suggests that at least one group differs in rank sums from the others.
The test is applicable when there is a nominal or ordinal variable with more than two values and a metric variable.
The calculation of the Kruskal-Wallis test involves assigning ranks, calculating rank sums, and comparing them to expected values.
The test statistic H is calculated using the formula involving the number of cases, mean rank sums, expected rank value, and variance of ranks.
The H value corresponds to the chi-square value, which can be compared to a critical chi-square value from statistical tables.
Data can be analyzed using an online tool such as DataTab, which automates the calculation of the Kruskal-Wallis test.
DataTab provides a p-value, which helps in determining whether to reject or retain the null hypothesis.
A p-value greater than the significance level (e.g., 0.05) indicates that the null hypothesis is not rejected.
The tutorial demonstrates how to perform the Kruskal-Wallis test with example data on reaction times of three groups.
The tutorial concludes that with the given data, there is no significant difference between the categories based on the p-value obtained.
The online calculation tool simplifies the process and makes it accessible for users without a strong statistical background.
The video encourages viewers to try out the online tool themselves for hands-on experience.
Transcripts
Browse More Related Video
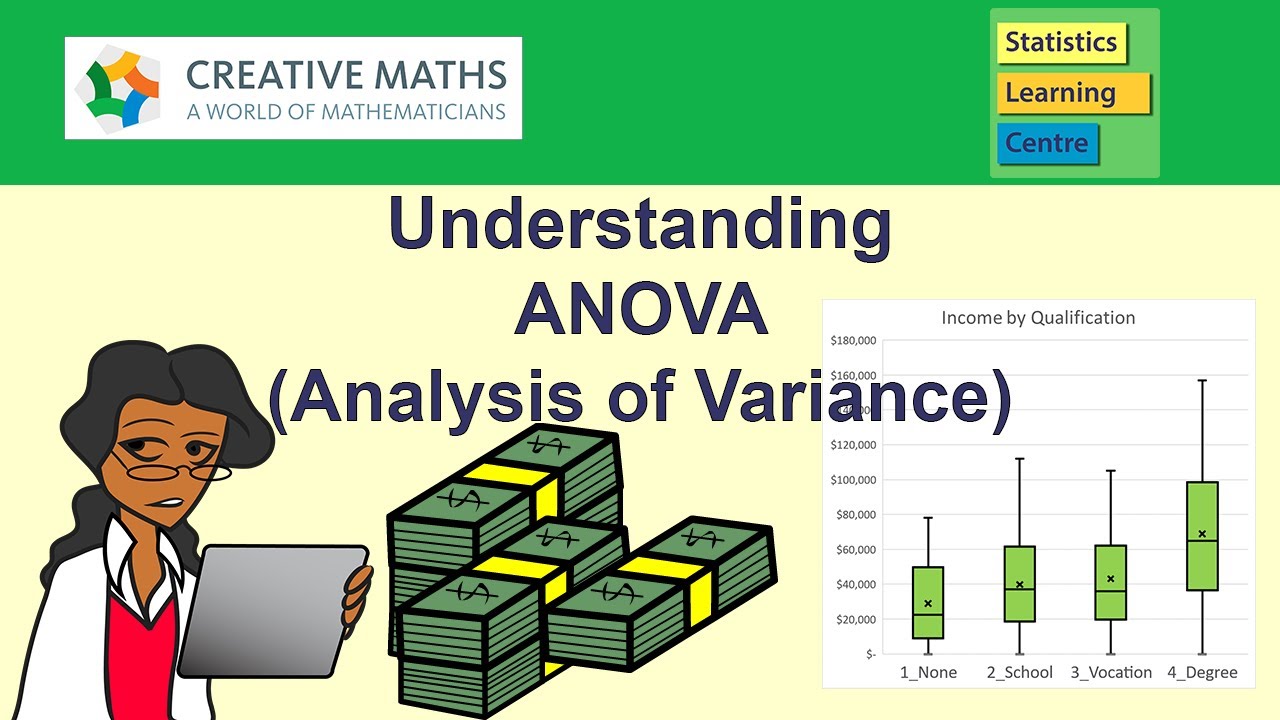
Understanding Analysis of Variance (ANOVA) including Excel - Statistics Help
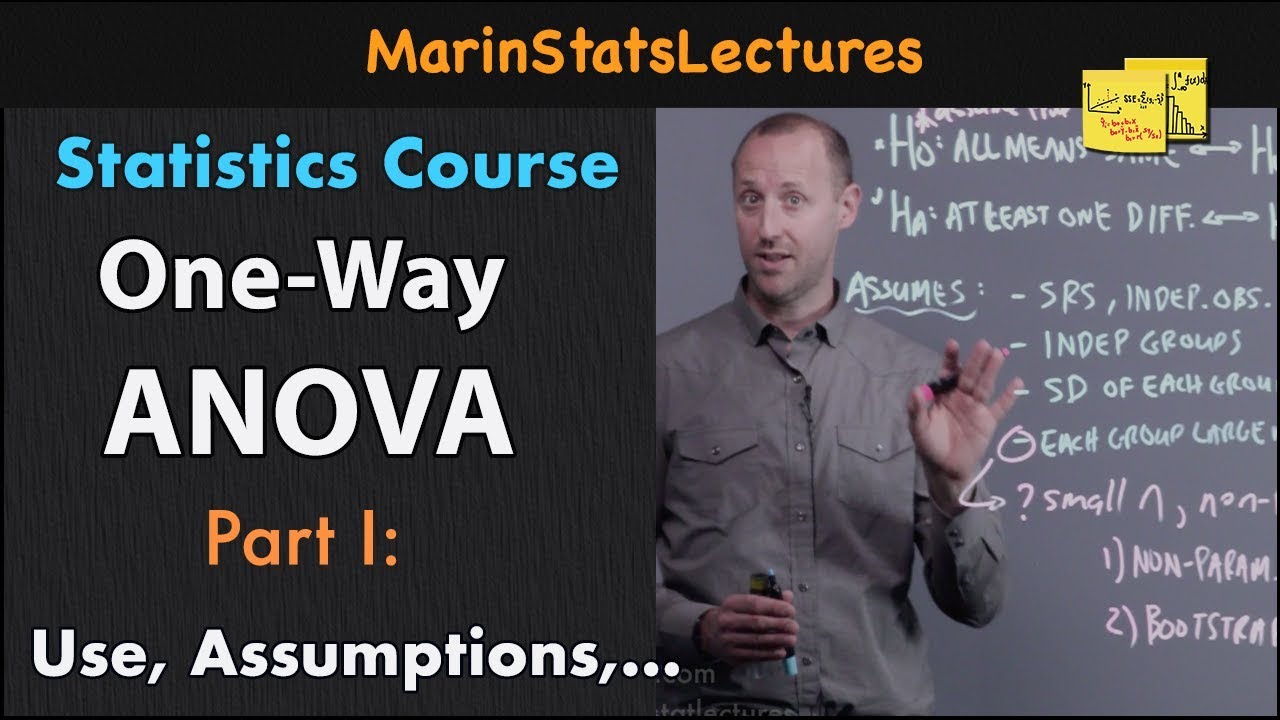
One Way ANOVA (Analysis of Variance): Introduction | Statistics Tutorial #25 | MarinStatsLectures
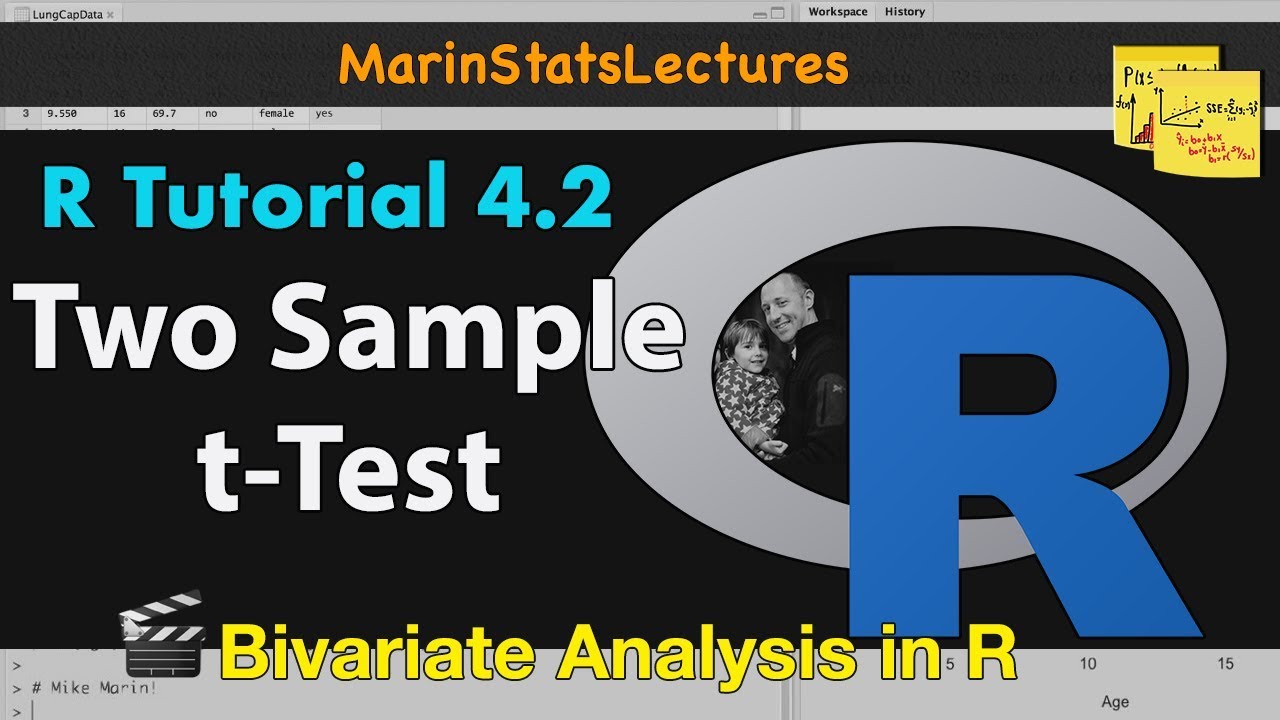
Two-Sample t Test in R (Independent Groups) with Example | R Tutorial 4.2 | MarinStatsLectures

Paired t-Test in R with Examples | R Tutorial 4.7 | MarinStatsLectures

ANOVA, ANOVA Multiple Comparisons & Kruskal Wallis in R | R Tutorial 4.9 | MarinStatsLectures|
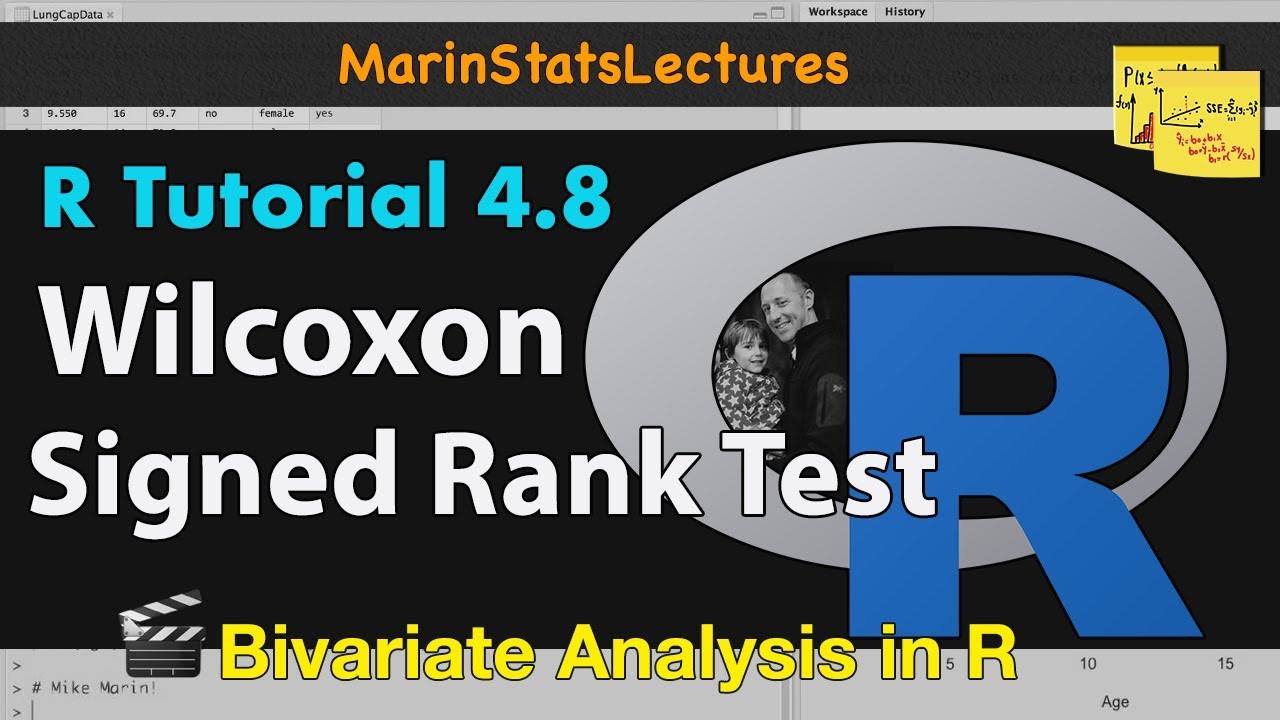
Wilcoxon Signed Rank Test in R with Example | R Tutorial 4.8 | MarinStatsLectures
5.0 / 5 (0 votes)
Thanks for rating: