Quantiles and Percentiles, Clearly Explained!!!
TLDRIn this episode of Stat Quest, Josh Star delves into the intricacies of quantiles and percentiles, explaining their definitions and practical applications. He clarifies that quantiles are lines dividing data into equal groups, with the median being a quintessential example. Percentiles are a specific type of quantile, dividing data into 100 groups. Despite the technical definitions, the terms are often used interchangeably, even when data sets are too small to be divided into 100 parts. Josh also discusses the various methods for calculating quantiles, highlighting the variability that can occur with small data sets and the convergence of results as sample sizes increase. He promises upcoming videos on quantile-quantile plots and quantile normalization, emphasizing the importance and prevalence of quantiles in statistical analysis.
Takeaways
- ๐งฌ Stat Quest is a series focused on statistics, particularly in the context of genetics.
- ๐ The video discusses quantiles and percentiles, which are concepts that can be confusing due to varying definitions and calculation methods.
- ๐ข Quantiles are defined as values that divide a dataset into equal groups, with the median being a quintessential example as it splits the data into two equal parts.
- ๐ Percentiles are a specific type of quantile that divides the data into 100 equal groups, with the median being the 50th percentile.
- ๐ The script clarifies that the terms 'quantile' and 'percentile' are often used interchangeably, even when the dataset is not large enough to be divided into 100 groups.
- ๐ The video script highlights that there are multiple methods to calculate quantiles, with R's quantile function offering nine different approaches.
- ๐ค It cautions that with small datasets, different quantile calculation methods can yield significantly different results.
- ๐ For larger datasets, the different methods tend to produce similar quantile results, indicating greater stability.
- ๐ The script explains that quantiles and percentiles are determined by the number of values less than the value of interest.
- ๐ The video promises further exploration of quantiles in upcoming Stat Quest episodes, including quantile-quantile plots and quantile normalization.
- ๐ฅ The host, Josh Star, invites viewers to subscribe for updates on future episodes and to share suggestions for new topics.
Q & A
What is the main topic of discussion in this StatQuest video?
-The main topic of discussion in this StatQuest video is quantiles and percentiles, and how they are defined and used in practice.
Why did Josh Star find it challenging to create this StatQuest episode?
-Josh Star found it challenging to create this StatQuest episode because every webpage he looked at had a slightly different explanation of quantiles and there are many different methods to calculate them, which led him down a 'crazy rabbit hole'.
What is the strict definition of a quantile according to the video?
-The strict definition of a quantile is a value that splits a data set into groups that contain the same number of data points. For example, the median is a quantile because it divides the data into two equal groups.
What is the median also known as in terms of quantiles?
-The median is also known as the 0.5 quantile or the 50% quantile because it splits the data into two equal parts.
How does the video illustrate the concept of quantiles?
-The video illustrates the concept of quantiles by measuring the expression of genes and using lines to divide the data into equally sized groups, such as the 0.25 (25%) and 0.75 (75%) quantiles.
What is the 0.25 quantile in the given example of gene expression data?
-In the given example, the 0.25 quantile, which represents 25% of the data points, is 2.5.
What is the 0.75 quantile in the given example of gene expression data?
-In the given example, the 0.75 quantile, which represents 75% of the data points, is 7.3.
Why are quantiles and percentiles often used interchangeably in practice?
-In practice, the terms quantile and percentile are often used interchangeably, even though technically percentiles are quantiles that divide the data into 100 equally sized groups.
How does the video explain the calculation of quantiles and percentiles?
-The video explains that calculating quantiles and percentiles involves determining how many values are less than the value of interest. For example, if one data point is less than a certain value out of fifteen, it is the 1/15 or approximately 7% quantile.
What does the video suggest about the reliability of quantiles in small datasets?
-The video suggests that in small datasets, quantiles can vary significantly depending on the method used to calculate them, so they should not be overly relied upon.
What is the significance of having multiple methods to calculate quantiles as mentioned in the video?
-The significance of having multiple methods to calculate quantiles is that they can result in slightly different outcomes, especially in small datasets, which highlights the importance of understanding the context and method when interpreting quantile values.
What future topics will be covered in the StatQuest series on quantiles?
-Future topics in the StatQuest series on quantiles will include quantile-quantile plots and quantile normalization, which will be covered in separate episodes.
Outlines
๐ Understanding Quantiles and Percentiles
In this segment, Josh Star introduces the topic of quantiles and percentiles, explaining their importance and the confusion surrounding their definitions. He clarifies that quantiles are lines that divide data into equal groups, with the median being a quintessential example of a quantile, as it splits the data into two equal parts. The video also discusses how quantiles are labeled, either by their position (e.g., 0.25 for the first quartile) or as a percentage (e.g., 25%). Percentiles are a specific type of quantile that divides data into 100 equal parts, but in practice, the terms are used interchangeably even when the data set is not large enough to be divided into 100 groups. Josh emphasizes the variability in calculating quantiles, noting that there are nine different methods in R, which can yield slightly different results, especially in small datasets. However, with larger datasets, the methods converge to provide more consistent results.
๐ Quantile Calculation Methods and Future Topics
This paragraph delves into the complexities of calculating quantiles and percentiles, acknowledging that there are multiple methods available in statistical software like R, which can lead to varying results. Josh warns against placing too much reliance on quantiles when working with small datasets due to their potential variability. He contrasts this with larger datasets, where different methods yield more consistent quantile values. The paragraph concludes with a teaser for future Stat Quest episodes, promising a series on quantiles, including discussions on quantile-quantile plots and quantile normalization. Josh encourages viewers to subscribe for updates on these upcoming videos and to leave suggestions for future topics in the comments section.
Mindmap
Keywords
๐กQuantiles
๐กPercentiles
๐กMedian
๐กExpression
๐กData Points
๐กQuantile Function
๐กR Programming Language
๐กQuantile-Quantile Plots
๐กQuantile Normalization
๐กStatistical Analysis
Highlights
Stat Quest is a special series focusing on quantiles and percentiles.
Quantiles and percentiles are often misunderstood due to varying definitions and calculation methods.
The strict definition of quantiles is to divide data into equal groups.
The median is a quantile, specifically the 0.5 or 50% quantile, dividing data into two equal parts.
Quantiles can be labeled by their decimal or percentage representation.
The 0.25 or 25% quantile divides the data such that 25% of points are below it.
The 0.75 or 75% quantile divides the data such that 75% of points are below it.
Percentiles are quantiles that divide data into 100 equal groups.
In practice, the terms quantile and percentile are used interchangeably.
Small datasets can lead to variability in quantile calculations due to different methods.
Large datasets yield more consistent quantile results across different methods.
Quantiles can be calculated using various methods, with R offering nine different options.
Quantile-quantile plots and quantile normalization will be covered in future Stat Quest episodes.
The importance of quantiles in statistical analysis and their wide application.
Stat Quest aims to clarify the confusion around quantiles and percentiles through a series of episodes.
The series will delve deeper into quantiles with a focus on their practical applications.
The transcript emphasizes the need for understanding quantiles due to their frequent use in statistics.
Transcripts
Browse More Related Video
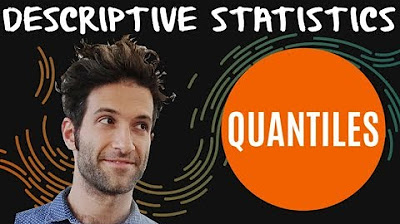
What are Quartiles? Percentiles? Deciles?
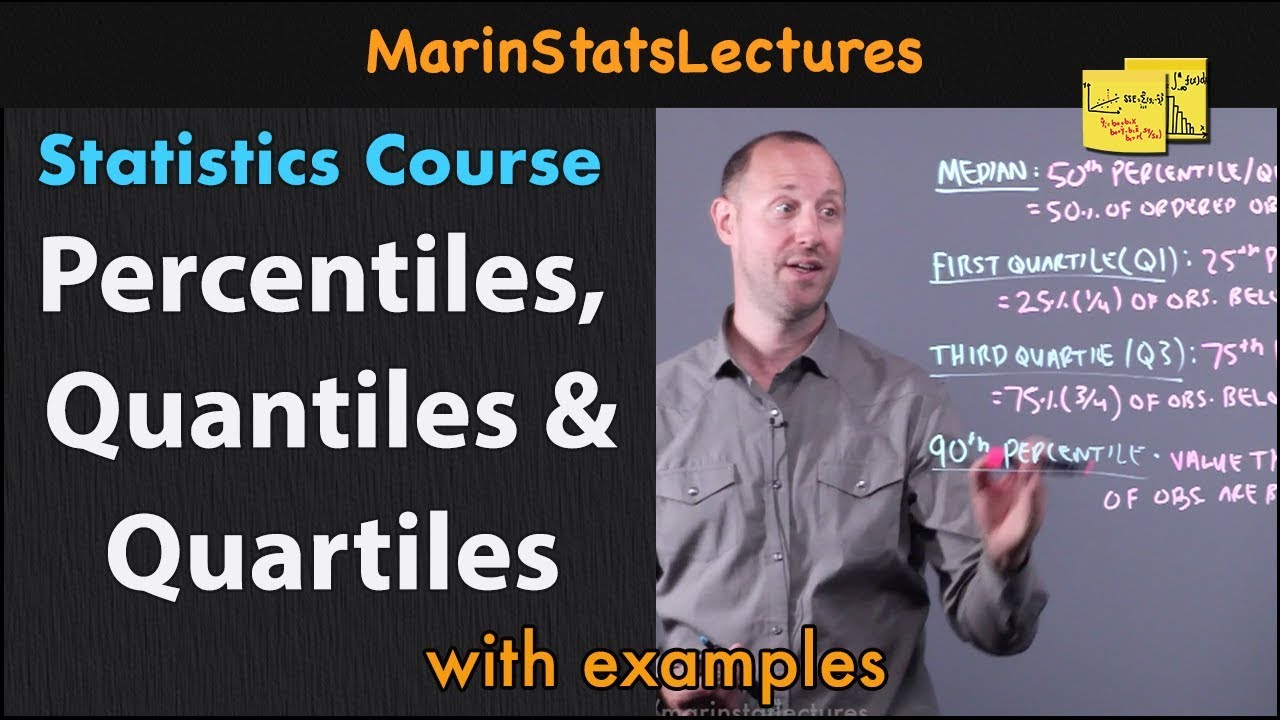
Percentiles, Quantiles and Quartiles in Statistics | Statistics Tutorial | MarinStatsLectures
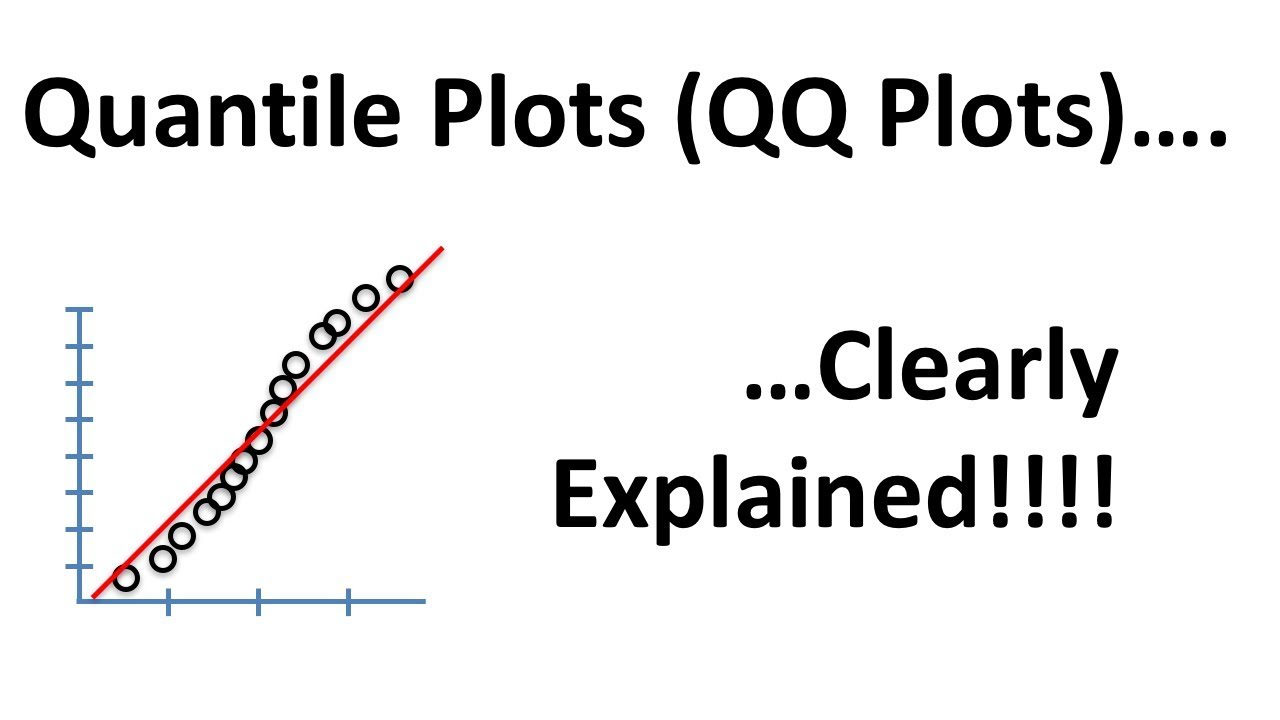
Quantile-Quantile Plots (QQ plots), Clearly Explained!!!

Quartiles, Deciles, & Percentiles With Cumulative Relative Frequency - Data & Statistics
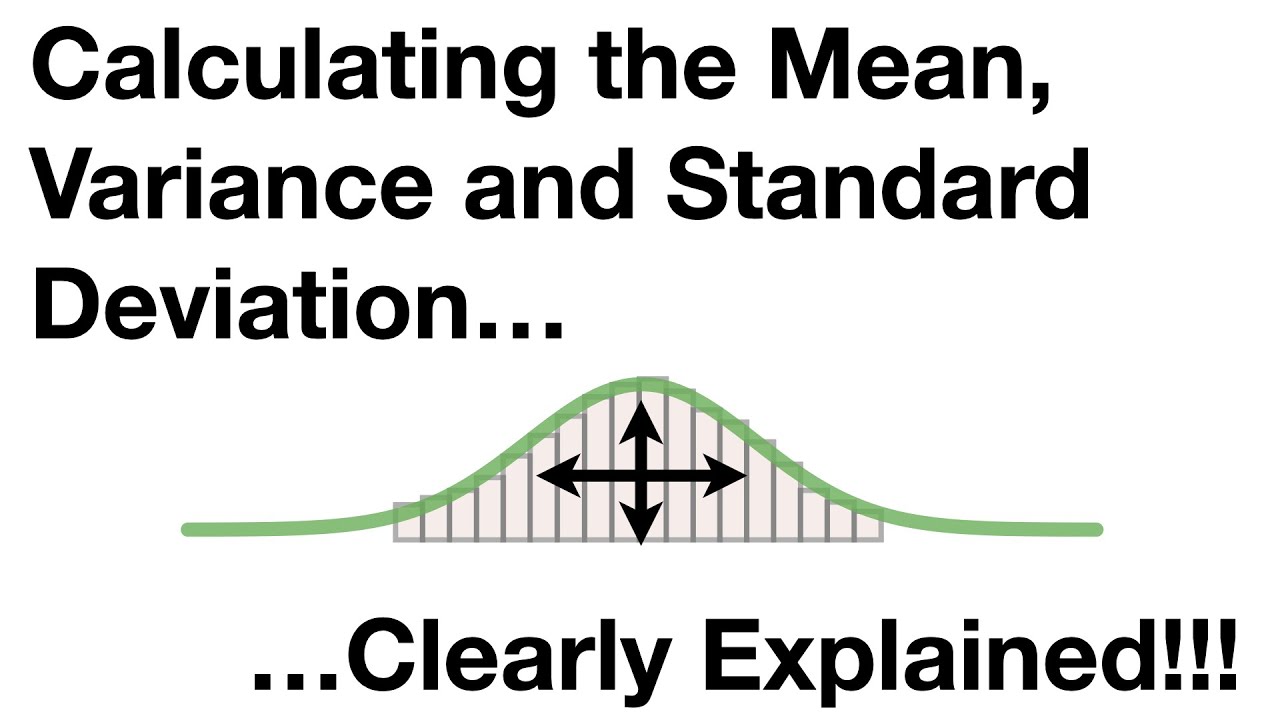
Calculating the Mean, Variance and Standard Deviation, Clearly Explained!!!
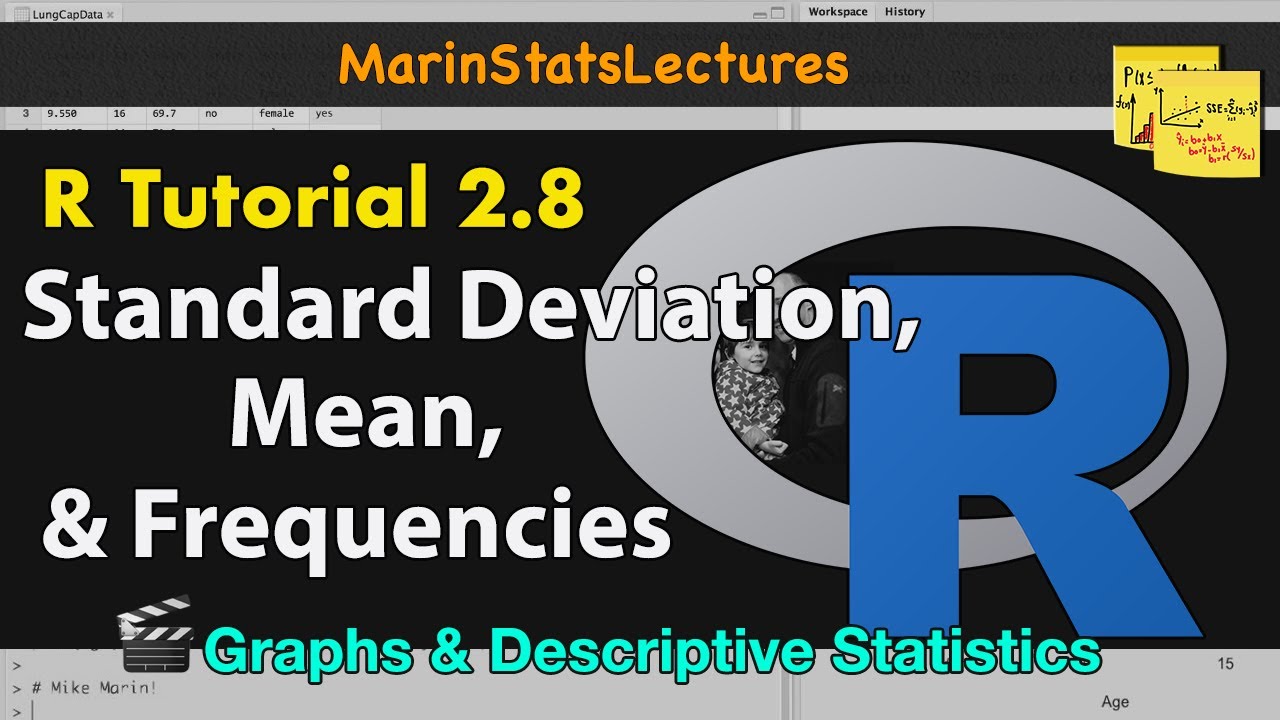
Calculating Mean, Standard Deviation, Frequencies and More in R | R Tutorial 2.8| MarinStatsLectures
5.0 / 5 (0 votes)
Thanks for rating: