Lecture 14: Location, Scale, and LOTUS | Statistics 110
TLDRThe video script delves into the intricacies of the standard normal distribution, denoted as Z, and its properties. It covers the probability density function (PDF), cumulative distribution function (CDF), mean, and variance, highlighting that the mean of Z is 0 and the variance is 1. The讲师 (instructor) discusses the concept of moments, emphasizing that all odd moments of the standard normal distribution are zero due to symmetry. The general normal distribution is introduced as X = μ + σZ, where μ is the mean (or location) and σ is the standard deviation (or scale). The讲师 demonstrates how to derive the PDF of the general normal distribution using standardization and the properties of variance. The script also touches on the properties of variance, explaining its calculation and the common mistake of omitting the square when multiplying by a constant. The讲师 further explains the variance of the sum and difference of independent normal random variables and introduces the empirical rule (68-95-99.7% rule) for normal distributions. The script concludes with a discussion on the variance of the Poisson distribution and a method for calculating the variance of the binomial distribution using indicator random variables and linearity. The讲师 also explains the Law of the Unconscious Statistician (LOTUS) for both discrete and general cases, providing an intuitive understanding of why it holds true.
Takeaways
- 👨🔬 The standard normal distribution, often denoted as Z, has a probability density function (PDF) that is symmetrical around zero, and its cumulative distribution function (CDF) is represented by Φ.
- 🔨 Key properties of the standard normal distribution include a mean (E[Z]) of 0 and a variance (Var[Z]) of 1, highlighting its symmetry since any odd moment like E[Z^3] equals 0.
- ✅ When deriving distributions from the standard normal, transforming Z by adding a mean (μ) and multiplying by a standard deviation (σ) creates a new normal distribution X with mean μ and variance σ^2.
- ⚠️ The variance of a distribution does not change when a constant is added (Var[X+c] = Var[X]), but scales by the square of the factor when multiplied (Var[cX] = c^2 Var[X]).
- 📈 Discusses how variance properties differ from expectations: variance is not linear, and Var[X+Y] ≠ Var[X] + Var[Y] unless X and Y are independent.
- ➡️ The transformation of a normal variable X (X = μ + σZ) back to the standard normal form is called standardization (Z = (X-μ)/σ).
- 📝 Highlights the importance of the LOTUS (Law of the Unconscious Statistician), which is used to compute expectations of transformations of random variables without knowing their distribution.
- ✏️ Explains how to derive the PDF of a generalized normal distribution through differentiation of its CDF, demonstrating practical application of calculus in probability.
- 📉 Introduces the 68-95-99.7% rule for normal distributions, explaining how it describes the probability of a value falling within certain standard deviations from the mean.
- 📖 Emphasizes practical approaches to understanding distributions, such as exploring variances through summing independent variables, and stresses the importance of recognizing how operations affect variance and mean.
Q & A
What is the standard notation for the cumulative distribution function (CDF) of the standard normal distribution?
-The standard notation for the CDF of the standard normal distribution is capital Phi (Φ).
What is the mean of a standard normal random variable Z?
-The mean of a standard normal random variable Z is 0, which is evident due to the symmetry of the distribution around the y-axis.
How is the variance of a standard normal distribution calculated, and what is its value?
-The variance is calculated using integration by parts, and for a standard normal distribution, it equals 1.
What is the expected value of Z cubed for a standard normal random variable Z?
-The expected value of Z cubed is 0 because it is an odd function, and by symmetry, all odd moments of the normal distribution are 0.
What is the term used to describe the property that all odd moments of the normal distribution are zero?
-This property is referred to as the 'third moment' in the context of moments of a distribution.
How is the general normal distribution defined in terms of the standard normal distribution?
-The general normal distribution is defined as X = μ + σZ, where μ is the mean (or location) and σ is the standard deviation (or scale), and Z is a standard normal random variable.
What is the effect of adding a constant (mu) to a normal random variable on its variance?
-Adding a constant (mu) to a normal random variable does not change its variance. The variance remains the same because variance is unaffected by changes in location.
How does multiplying a normal random variable by a constant (sigma) affect its variance?
-Multiplying a normal random variable by a constant (sigma) results in the variance being multiplied by the square of that constant (sigma squared).
What is the rule of standardization for converting a general normal random variable X to a standard normal random variable Z?
-The rule of standardization is Z = (X - μ) / σ, where X is a normal random variable with mean μ and standard deviation σ, and Z is the resulting standard normal random variable.
What is the 68-95-99.7% rule for the normal distribution, and what does it signify?
-The 68-95-99.7% rule states that for a normal distribution, approximately 68% of the data falls within one standard deviation of the mean, 95% falls within two standard deviations, and 99.7% falls within three standard deviations.
How is the variance of a Poisson random variable with parameter lambda derived, and what is its value?
-The variance of a Poisson random variable is derived using the law of the unconscious statistician (LOTUS) and is found to be equal to lambda, the parameter of the Poisson distribution.
What is the variance of a binomial random variable with parameters n and p?
-The variance of a binomial random variable is npq, where q = 1 - p, and both n and p are the parameters of the binomial distribution.
Outlines
📚 Introduction to Standard Normal Distribution
The paragraph begins with a review of the standard normal distribution, denoted as Z, which has a mean of 0 and a variance of 1. The cumulative distribution function (CDF) of the standard normal is symbolized by the capital Phi and cannot be expressed in closed form. The properties of the standard normal are discussed, including its mean and variance, and the fact that all odd moments (E[Z^n] for odd n) are zero due to symmetry. The concept of moments is introduced, and the importance of symmetry in understanding the distribution is highlighted. The general normal distribution is then introduced as X = μ + σZ, where μ is the mean (or location) and σ is the standard deviation (or scale).
🔍 Properties of Variance and Transformations
This section delves into the properties of variance, emphasizing that variance is not linear and cannot be negative. It explains how adding a constant to a random variable does not affect its variance, but multiplying by a constant c results in the variance being multiplied by c^2. The importance of squaring the constant when applying it to the variance is stressed to avoid computational errors. The paragraph also clarifies that the variance of a sum of two random variables is not simply the sum of their variances unless the variables are independent. The concept of standardization for normal distributions is introduced as X = μ + σZ, and the reverse process is also explained.
🧮 Deriving the PDF of the General Normal Distribution
The paragraph focuses on deriving the probability density function (PDF) of a general normal distribution with mean μ and variance σ^2. It uses the standardization technique to transform the random variable X into a standard normal Z, and then applies the chain rule from calculus to find the derivative of the CDF, which yields the PDF. The process is demonstrated to be straightforward once the PDF of the standard normal distribution is known. The concept of the 68-95-99.7% rule is introduced as a rule of thumb for the normal distribution, indicating the probability of a normal random variable falling within a certain number of standard deviations from the mean.
📉 Variance of the Poisson Distribution
The discussion shifts to the Poisson distribution, which is characterized by having a mean and variance both equal to lambda (λ). The process of finding the variance involves using the moment-generating function (MGF) of the Poisson distribution and applying derivatives to find E[X^2]. The sum of λ^k/k! is derived using the Taylor series expansion of e^λ, and the variance is ultimately found by subtracting the square of the mean from E[X^2]. The properties of the Poisson distribution are highlighted, including the fact that the mean equals the variance.
🎯 Variance of the Binomial Distribution
The paragraph tackles the calculation of the variance for a binomial distribution. It outlines three potential methods for finding the variance: a direct but tedious calculation using the binomial PMF, an easy method that relies on the variance of independent sums (which is not yet proven), and a compromise method using indicator random variables. The compromise method involves squaring the binomial expression, summing over all terms, and then applying expected values to simplify the expression. The result is the variance of the binomial distribution, which is given by np(1-p), where n is the number of trials and p is the probability of success in each trial.
🧩 LOTUS and Its Proof for Discrete Random Variables
The final paragraph provides an explanation for the Law of the Unconscious Statistician (LOTUS), particularly for discrete random variables. LOTUS states that the expected value of a function of a random variable can be computed as a sum of the function times the probability mass function (PMF) without first determining the distribution of the function. The proof involves considering the sum over all possible values of the random variable and grouping them into 'super pebbles' based on their values. The double sum representation is used to show that the expected value can be computed by first summing over all values of the random variable and then summing over the corresponding probabilities, leading to the conclusion that LOTUS holds true.
Mindmap
Keywords
💡Standard Normal
💡PDF (Probability Density Function)
💡CDF (Cumulative Distribution Function)
💡Variance
💡Moment
💡LOTUS (Law of the Unconscious Statistician)
💡Standardization
💡General Normal Distribution
💡Independence
💡68-95-99.7% Rule
Highlights
The standard normal distribution, often denoted as Z, has a mean (E[Z]) of 0 and a variance of 1.
All odd moments (E[Z^n] for odd n) of the standard normal distribution are zero due to symmetry.
The even moments of the standard normal distribution can be computed using integration techniques.
The concept of 'moments' in statistics refers to the expected values of powers of a random variable.
Minus Z is also standard normal, highlighting the symmetry of the standard normal distribution.
General normal distribution X can be expressed as X = μ + σZ, where μ is the mean and σ is the standard deviation.
The mean and variance of a general normal distribution X are μ and σ^2, respectively.
Standardization of a normal variable X is achieved by subtracting the mean and dividing by the standard deviation (X - μ) / σ.
The PDF of a general normal distribution can be derived from the standard normal PDF using standardization.
The variance of a scaled random variable is the square of the scaling factor times the original variance.
The sum of independent normal random variables is itself normal, with a mean equal to the sum of the means and variance equal to the sum of the variances.
The empirical rule (68-95-99.7% rule) provides a quick way to estimate the probability of a normal variable falling within certain standard deviation ranges.
The variance of a Poisson random variable with parameter λ is equal to λ, which is also its mean.
The binomial variance can be calculated using the formula npq, where n is the number of trials, p is the probability of success, and q = 1 - p.
Linearity of expectation (LOTUS) allows for the computation of the expected value of a function of a random variable without needing the distribution of that function.
The proof of LOTUS for a discrete sample space involves grouping and ungrouping pebbles (outcomes) in a way that maintains the average value.
The double sum technique used in the proof of LOTUS rearranges terms to show that the expected value of a function of a random variable can be computed directly from the random variable's distribution.
Transcripts
Browse More Related Video

Elementary Statistics - Chapter 6 Normal Probability Distributions Part 1

6.1.3 The Standard Normal Distribution - Normal Dist. and Properties of the Standard Normal Dist.
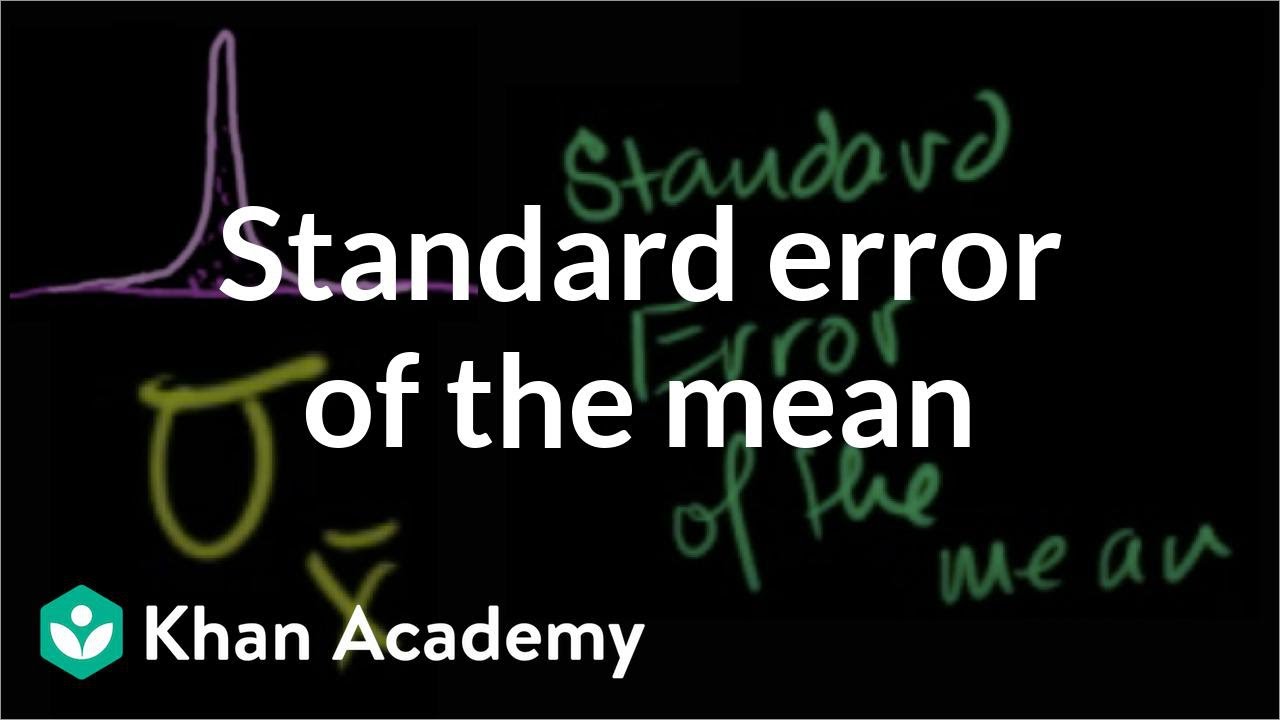
Standard error of the mean | Inferential statistics | Probability and Statistics | Khan Academy
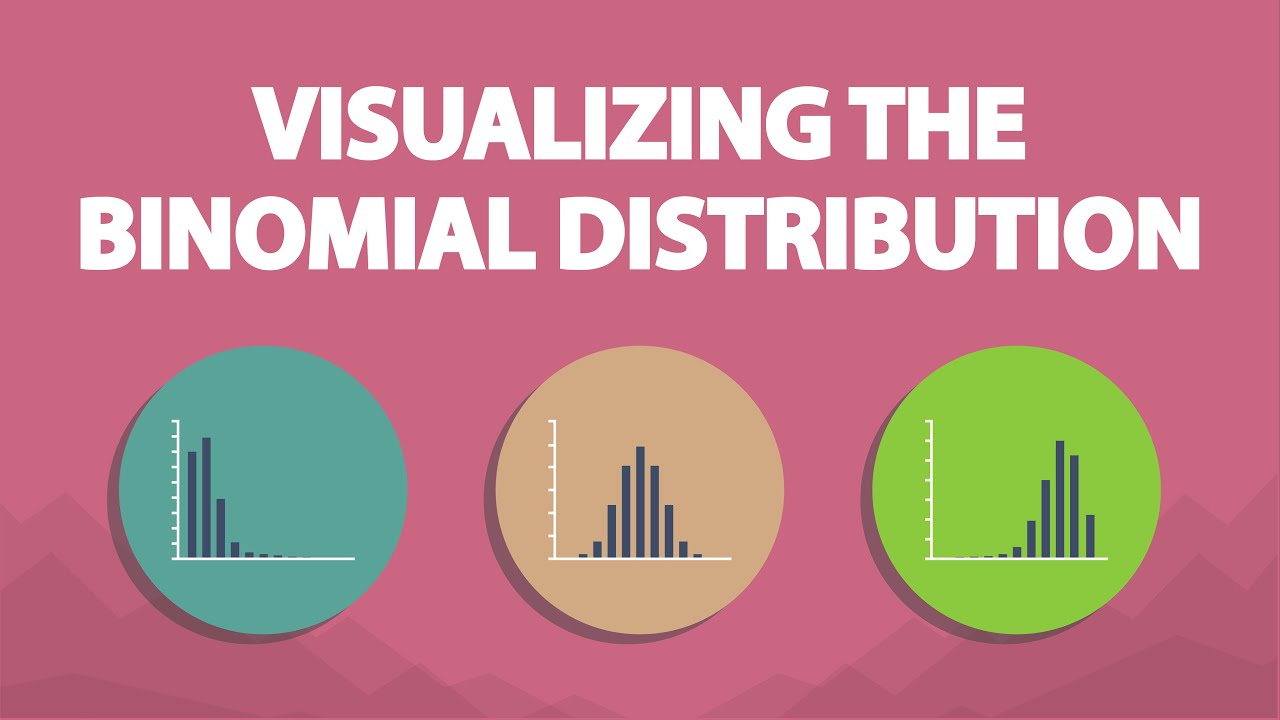
Visualizing the Binomial Distribution (6.6)

Elementary Stats Lesson #11
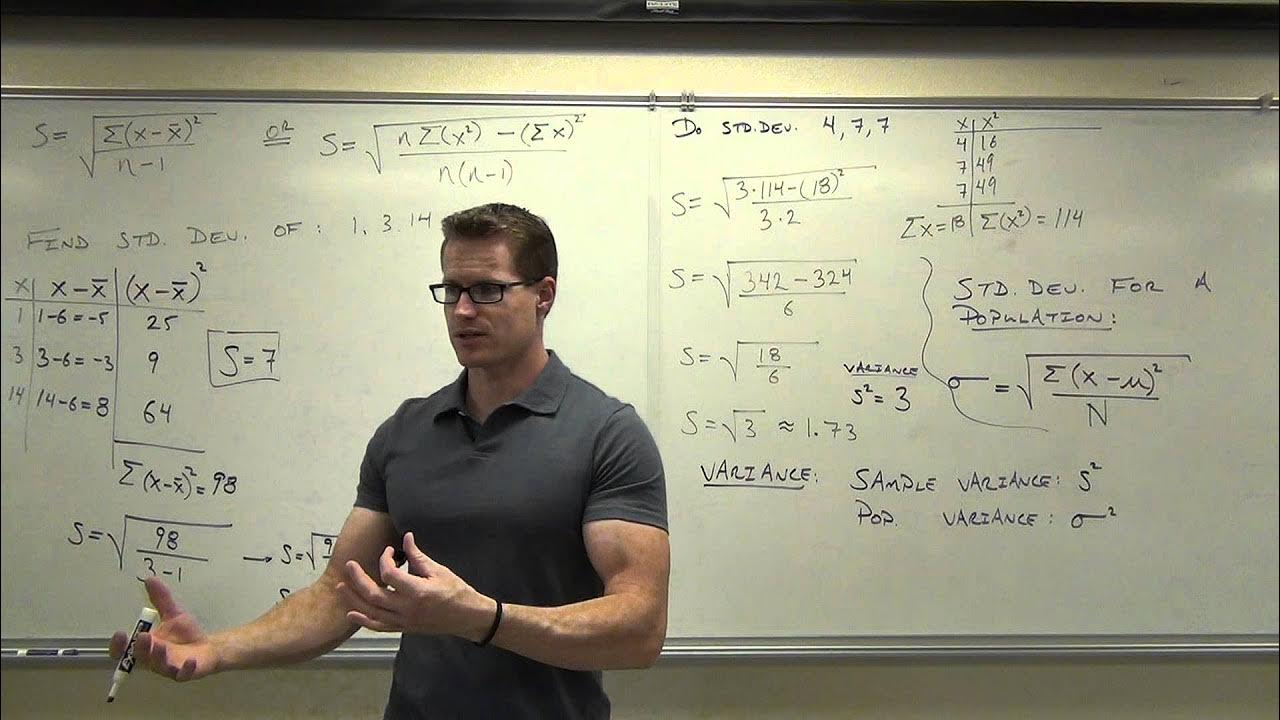
Statistics Lecture 3.3: Finding the Standard Deviation of a Data Set
5.0 / 5 (0 votes)
Thanks for rating: