The Turing Lectures: What is generative AI?
TLDRProfessor Mirella Lapata delivered a comprehensive lecture on generative AI at the Turing Institute, exploring its history, current capabilities, and future implications. She discussed the evolution from single-purpose AI like Google Translate to more sophisticated models like ChatGPT, the importance of fine-tuning, and the challenges of bias and misinformation. The lecture also touched on the potential societal impacts, including job displacement and the need for regulation, emphasizing AI's role as a tool with both benefits and risks.
Takeaways
- ๐ The Turing Lectures on generative AI are a flagship series by the Turing Institute, featuring leading speakers on data science and AI since 2016.
- ๐ Generative AI, such as ChatGPT and Dali, can produce content like essays or emails, and has both beneficial and concerning implications, which the lecture series aims to explore.
- ๐ง Professor Mirella Lapata, a renowned expert in natural language processing (NLP), discussed the technology behind generative AI and its evolution from single-purpose systems like Google Translate to more sophisticated models like ChatGPT.
- ๐ The core technology behind generative AI is language modeling, which predicts the most likely continuation of text based on the context provided.
- ๐ The success and capability of generative AI models have increased significantly with the scale of data and model size, with ChatGPT-4 claiming to outperform 90% of humans on the SAT.
- ๐ก Language models are trained using a large corpus of text from various sources like Wikipedia, StackOverflow, and social media, predicting words that have been removed to teach the model.
- ๐ข The number of parameters in AI models has grown exponentially, with some models like GPT-54 reaching 1 trillion parameters, though still not at the level of the human brain's complexity.
- ๐ The training and fine-tuning of AI models involve self-supervised learning and human preference feedback, which can influence the model's behavior and reduce biases.
- ๐ผ The development and training of large AI models like ChatGPT are costly and resource-intensive, raising questions about accessibility and the potential societal impacts.
- ๐ There are risks associated with generative AI, including the propagation of misinformation, biases, and the potential for job displacement, highlighting the need for regulation and ethical considerations.
- ๐ฎ The future of AI is uncertain, with possibilities ranging from beneficial applications to risks of misuse, emphasizing the importance of ongoing research and public discourse on AI's role in society.
Q & A
What is the main focus of the Turing Lectures on generative AI?
-The Turing Lectures on generative AI focus on exploring how AI has impacted the Internet, particularly the role of generative AI technologies, their potential benefits, and the challenges they pose.
What is generative AI, and how does it differ from traditional AI?
-Generative AI refers to AI systems that can create new content, such as text, images, or audio, that the system has not seen before. It differs from traditional AI in that it is not just about processing existing data but also about synthesizing new content based on learned patterns.
Can you provide an example of an early application of generative AI?
-An early example of generative AI is Google Translate, which launched in 2006 and uses AI to translate text from one language to another, creating new content in the target language.
What are the potential benefits of generative AI technologies?
-Generative AI technologies can revolutionize various industries by enabling the creation of new content, automating creative processes, and enhancing human creativity through AI collaboration.
Outlines
๐ Introduction and Opening Remarks
Hari introduces himself and the event, expressing excitement for the Turing lecture series on generative AI. He engages the audience with a light-hearted joke about his name and gauges their familiarity with Turing lectures. Hari outlines the format of the event, including a Q&A session.
๐ค What is Generative Artificial Intelligence?
Professor Mirella Lapata begins by defining generative AI, explaining its componentsโartificial intelligence and generative content. She provides examples of generative AI, such as Google Translate and Siri, to illustrate its longstanding presence. The focus of the lecture will be on text generation within natural language processing (NLP).
๐ Evolution of AI Models
Mirella traces the development of AI models, highlighting the shift from single-purpose systems like Google Translate to more sophisticated models like GPT-4. She discusses the core technology behind these models, emphasizing language modeling and the ability to predict the next word in a sequence based on context.
๐ง Building and Training AI Models
The process of building and training language models is detailed. Mirella explains the need for vast amounts of data and the use of neural networks to predict the next word in a sentence. The concept of fine-tuning pre-trained models for specific tasks is introduced, along with the challenges and costs associated with training large models like GPT-4.
๐ Neural Networks and Transformers
Mirella explains the structure and function of neural networks, focusing on the role of layers and weights in learning. She introduces the transformer architecture, a key component of GPT models, and discusses its ability to process and predict language. The importance of self-supervised learning in training these models is emphasized.
๐ Scaling AI Models
The lecture covers the importance of scale in AI models, illustrating how increasing the number of parameters enhances the model's capabilities. Mirella presents graphs showing the growth in model sizes and the number of words processed. She discusses the high costs and energy consumption associated with training large models.
๐ Fine-Tuning and Human Preferences
Fine-tuning is revisited as a critical step in aligning AI models with human needs. Mirella explains how user preferences and human feedback are incorporated to improve model performance. The challenges of ensuring AI systems are helpful, honest, and harmless are highlighted, along with the HHH framework for aligning AI with human values.
๐ก AI Demonstrations and Limitations
Live demonstrations of ChatGPT's capabilities are presented, showcasing its ability to answer questions, generate poems, and more. Mirella addresses the limitations of AI, including issues with accuracy, bias, and the potential for misuse. Examples of misinformation and the risks of generative AI are discussed.
๐ The Future of AI
Mirella discusses the potential future developments in AI, including the balance between benefits and risks. She addresses the challenges of regulating AI, the environmental impact of training large models, and the societal implications of job displacement. The importance of ethical considerations and responsible AI use is emphasized.
๐ Closing Remarks and Q&A
The lecture concludes with a Q&A session, where Mirella addresses audience questions on various topics, including AI bias, detection of AI-generated content, and the role of humans in AI development. She underscores the importance of continued human involvement in fine-tuning and ethical oversight of AI technologies.
Mindmap
Keywords
๐กGenerative AI
๐กTuring Lectures
๐กNatural Language Processing (NLP)
๐กLanguage Modelling
๐กTransformers
๐กFine-tuning
๐กSelf-supervised Learning
๐กParameter Size
๐กBias
๐กRegulation
๐กMisinformation
Highlights
Introduction and background of the Turing Lecture series, highlighting its significance since 2016.
Explanation of generative AI and its applications, including examples like ChatGPT and DALL-E.
Overview of the history of generative AI, mentioning early examples like Google Translate and Siri.
Description of how generative AI models work, including the concept of language modeling and neural networks.
Discussion on the importance of large datasets in training AI models and the role of neural networks in prediction.
Explanation of the Transformer architecture, which underpins many modern AI models.
Insights into the scalability of AI models, including the increasing number of parameters and the impact on performance.
The role of fine-tuning in enhancing AI models' capabilities, particularly for specific tasks.
Challenges of AI, including the alignment problem and ensuring models are helpful, honest, and harmless.
Live demonstration of ChatGPT's capabilities, showcasing both its strengths and limitations.
Discussion on the environmental impact of training large AI models, including energy consumption and emissions.
Potential societal impacts of AI, such as job displacement and the generation of fake content.
Future perspectives on AI, including the importance of regulation and the potential risks and benefits.
Audience questions addressing topics like bias in AI, emergent properties, and the role of human oversight.
Closing remarks emphasizing the need for ongoing research, ethical considerations, and public engagement in AI development.
Transcripts
Browse More Related Video
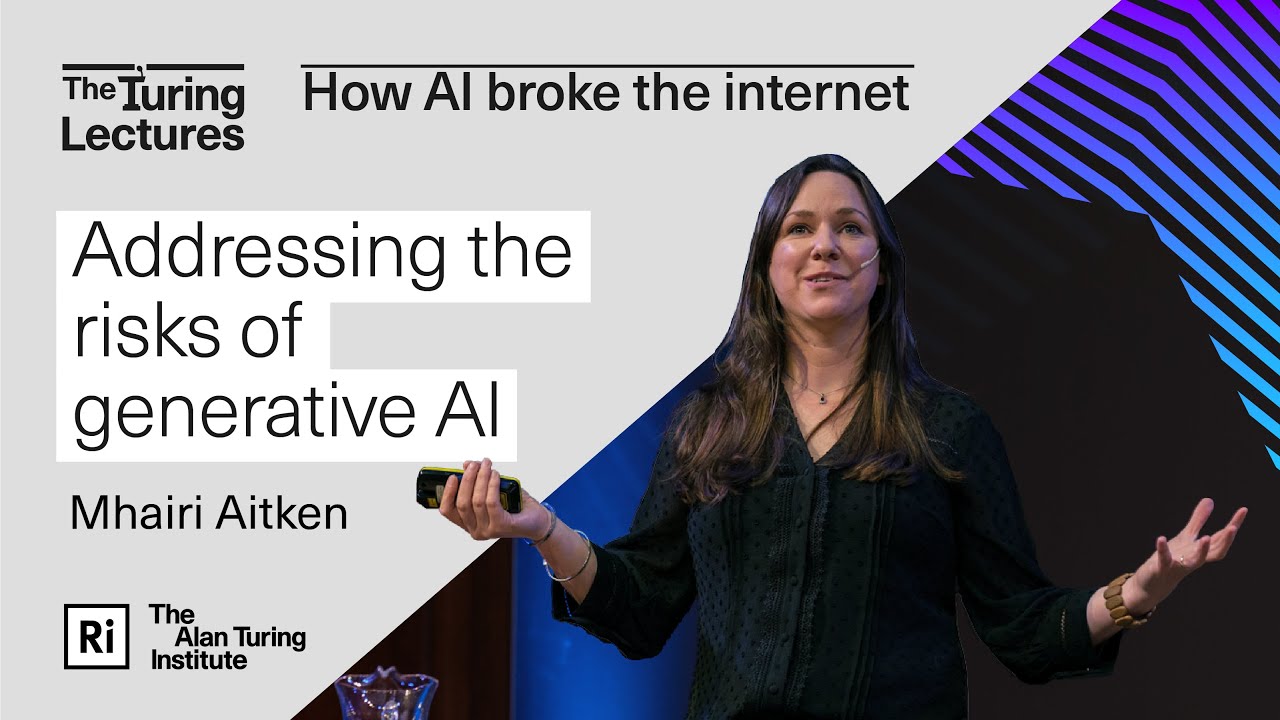
The Turing Lectures: Addressing the risks of generative AI

What is generative AI and how does it work? โ The Turing Lectures with Mirella Lapata
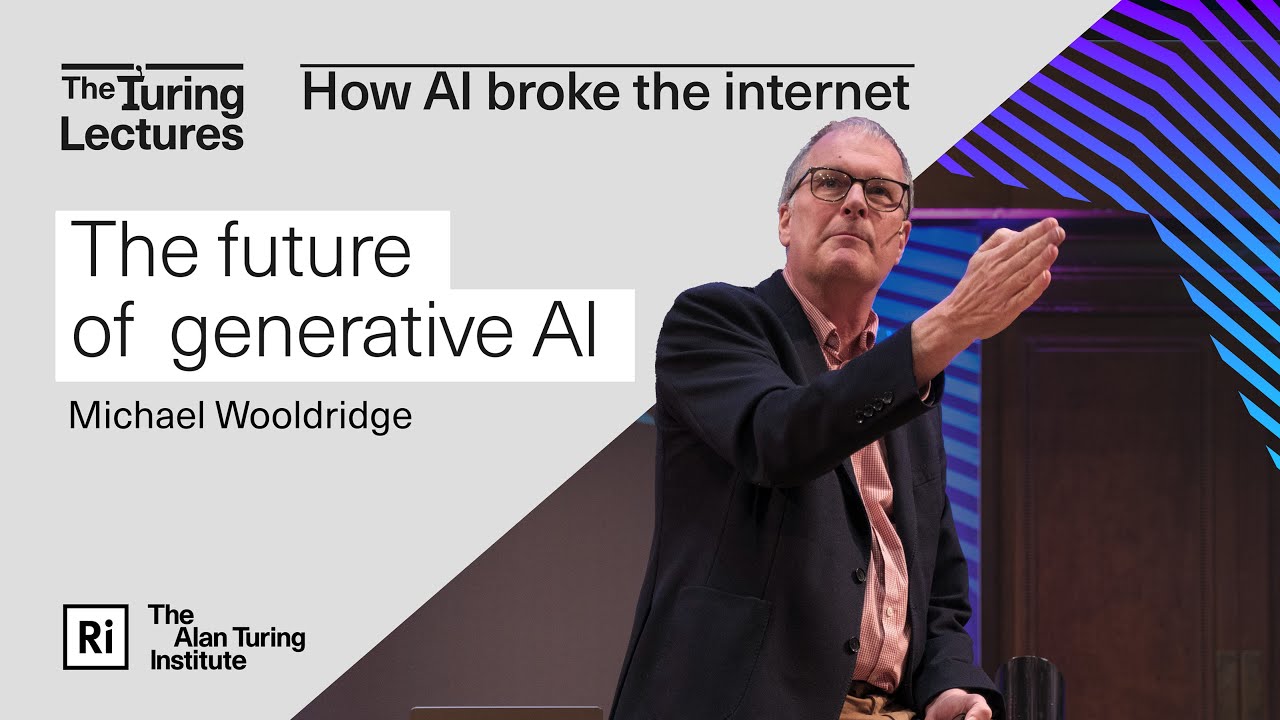
The Turing Lectures: The future of generative AI
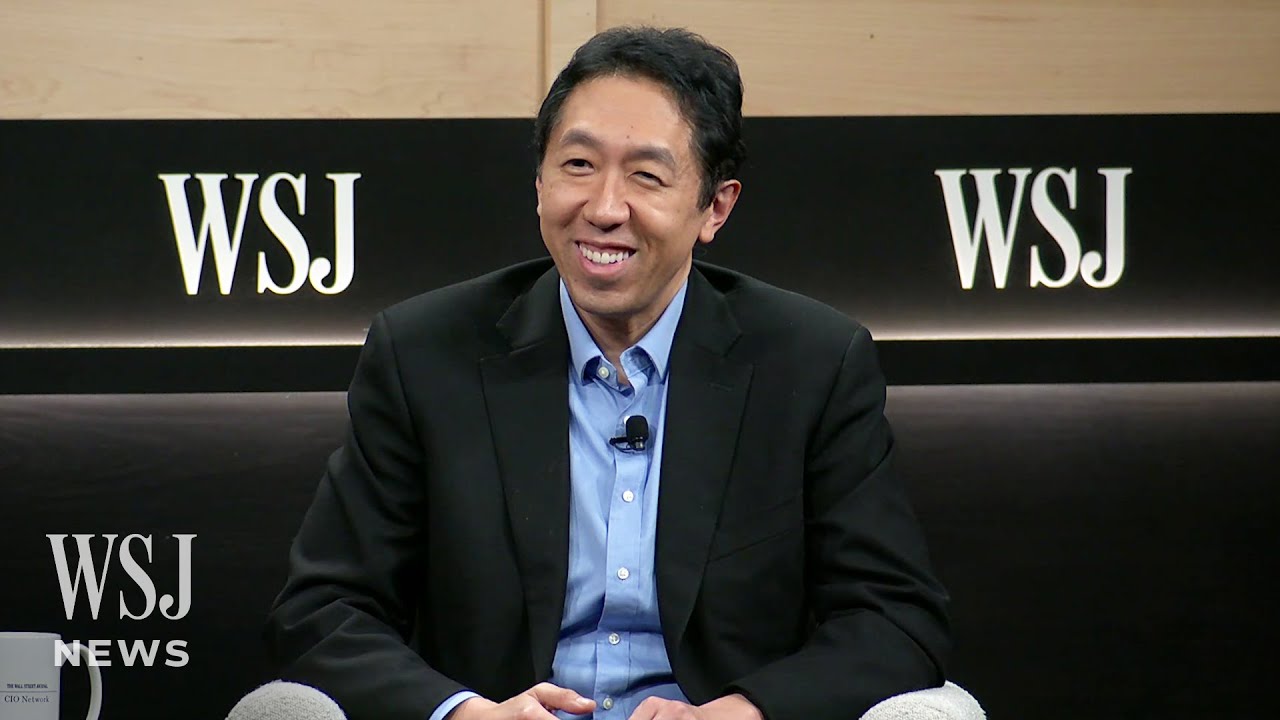
Andrew Ng on AI's Potential Effect on the Labor Force | WSJ
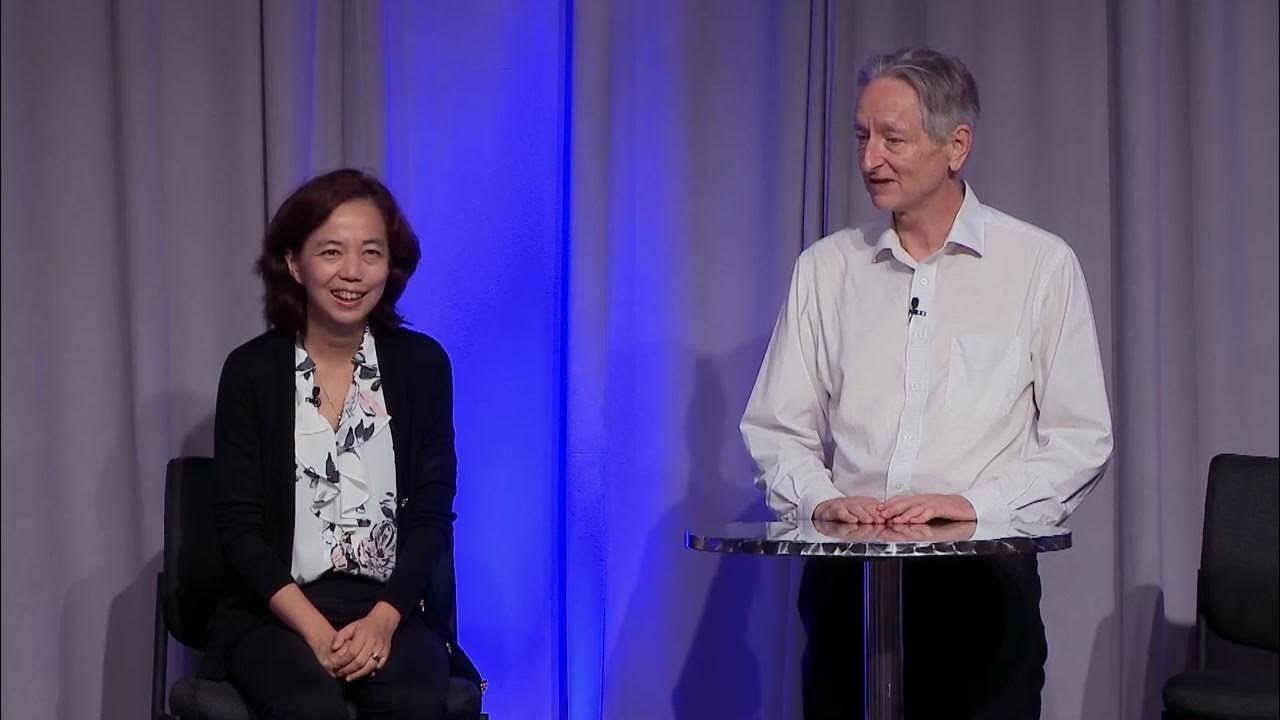
Geoffrey Hinton in conversation with Fei-Fei Li โ Responsible AI development
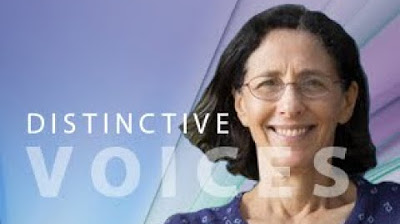
The Future of Artificial Intelligence
5.0 / 5 (0 votes)
Thanks for rating: