The Turing Lectures: The future of generative AI
TLDRProfessor Michael Wooldridge delivers a comprehensive lecture on the current state and future of artificial intelligence, particularly focusing on large language models like GPT-3 and their implications. He discusses the transformative impact of these models, their ability to generate human-like text, and the ethical and practical challenges they present, such as the potential for spreading bias and misinformation. Wooldridge also addresses the concept of general AI, the potential for AI to achieve consciousness, and the responsibility humans hold in the development and use of AI technologies. The lecture concludes with an engaging Q&A session where he further elaborates on the intricacies of AI and its potential integration with human society.
Takeaways
- π **Generative AI's Impact**: Generative AI, such as ChatGPT and DALL-E, can produce a wide range of content, from text to images, and has various applications including professional writing, creative prompts, and even legal filings.
- π§ **Human-like AI**: Despite the impressive capabilities of AI, it lacks human-like understanding and consciousness. AI operates on pattern recognition rather than comprehension or reasoning.
- π **AI's Evolution**: AI has evolved significantly since the post-WWII era, with major advancements in machine learning around 2005 and a significant boost in 2012 due to the use of GPUs.
- π **Data-Driven AI**: Modern AI systems are heavily reliant on vast amounts of data. Training a large language model like GPT3 requires processing up to 500 billion words of text.
- βοΈ **Neural Networks**: Neural networks, inspired by the human brain, are at the core of machine learning. They consist of interconnected neurons that perform simple pattern recognition tasks.
- π **Bias and Toxicity**: AI models can inherit biases and toxic content from the data they are trained on, highlighting the need for careful filtering and the introduction of 'guardrails'.
- π **Accuracy Concerns**: AI can generate fluent but factually incorrect information. It is essential to fact-check AI-generated content, as it cannot distinguish truth from falsehood.
- π§ **Generative AI's Limitations**: While AI has made strides in natural language processing, it still struggles with tasks like logical reasoning, planning, and real-world manipulation.
- βοΈ **Responsibility and Ethics**: The responsibility for AI outcomes lies with the users and developers. It is crucial to consider legal, ethical, and moral implications when deploying AI systems.
- π³ **Model Collapse**: AI-generated content, when used to train further AI models, can lead to a degradation of quality, emphasizing the value of authentic human-generated content.
- β **The Future of AI**: The future of AI is likely to involve more sophisticated models that can handle multiple modalities (text, images, sound) and potentially create immersive virtual experiences.
Q & A
What is the Turing Institute's focus in terms of research applications?
-The Turing Institute focuses on finding real-world use cases and users for its research outputs, particularly in the domains of data science and AI.
Why is the Turing Lecture series significant?
-The Turing Lecture series is significant as it is the institute's flagship lecture series that has been running since 2016, welcoming world-leading experts in data science and AI to share their insights.
How does generative AI, like ChatGPT and DALL-E, differ from other AI applications?
-Generative AI differs as it involves algorithms capable of generating new content, such as text, images, and more, which can be used for a wide range of applications, from professional work to creative endeavors.
What is the role of training data in machine learning?
-Training data is crucial for machine learning as it provides the input-output pairs that the algorithms learn from. It helps the system make predictions or classifications based on patterns it has learned during the training phase.
How do neural networks function in the context of AI?
-Neural networks function by mimicking the human brain's structure, using interconnected neurons to recognize patterns. Each neuron performs a simple pattern recognition task, and when a pattern is detected, it sends a signal to connected neurons.
What are the implications of large language models like GPT3?
-Large language models like GPT3 represent a significant leap in AI capabilities, offering more accurate and sophisticated text generation and understanding. They are trained on vast amounts of data and can perform tasks like language translation, content creation, and more.
Why did the development of AI technologies experience a surge around 2005 and 2012?
-The surge in AI development around 2005 and 2012 was due to the rise of machine learning techniques that began to work on more practical and useful problems. The discovery that graphics processing units (GPUs) were highly effective for the mathematical operations required for AI further accelerated progress.
What are the potential ethical concerns with AI systems like ChatGPT?
-Potential ethical concerns include the generation of incorrect or misleading information, biases present in the training data leading to unfair or prejudiced outputs, and the potential misuse of the technology for harmful purposes.
How does the transformer architecture contribute to the capabilities of large language models?
-The transformer architecture, introduced in the paper 'Attention Is All You Need', allows for more efficient processing of sequences in the data, enabling the models to better understand the context in which words appear, thus improving the quality of text generation and understanding.
What is the significance of the Turing Test in the context of AI?
-The Turing Test, proposed by Alan Turing, is a measure of a machine's ability to exhibit intelligent behavior that is indistinguishable from that of a human. It has historically been a benchmark for AI development, although modern AI has moved beyond this test to more complex and nuanced tasks.
How does the speaker view the future of AI in terms of general intelligence and consciousness?
-The speaker believes that while we are not close to achieving general AI that can perform any task a human can, we are making progress in specific areas like natural language processing. Regarding machine consciousness, the speaker asserts that current AI systems are not conscious and do not possess a mind or self-awareness.
Outlines
π Introduction to the Turing Lecture
The speaker, Hari Sood, welcomes the audience to a special Turing Lecture, the last in the series of 2023. He introduces himself as a research application manager at the Turing Institute, focusing on real-world applications of the institute's research. The event is a hybrid lecture and discourse, marking a new tradition. Sood engages the audience with a quick show of hands, recognizing both newcomers and returning attendees. He highlights the significance of the Turing Lectures, which have hosted world-leading experts in data science and AI since 2016. The institute is named after Alan Turing, a prominent British mathematician known for his role in cracking the Enigma code during World War II. The lecture series aims to advance data science and AI research for societal benefit. Sood also outlines the format of the evening, including a Q&A session and the use of social media for audience engagement, and sets the stage for a discussion on generative AI.
π The Evolution of Artificial Intelligence
The lecture delves into the history of AI, noting its slow progress until the 21st century. It emphasizes the pivotal role of machine learning in advancing AI, particularly after 2005. Machine learning, despite its misleading name, does not involve self-study but rather the use of training data to teach AI systems. The talk uses the example of facial recognition to illustrate supervised learning, where a system is shown input-output pairs to learn to recognize faces. The importance of training data is underscored, with social media contributing significantly to this data pool. The lecture also touches on the risks and potential future of generative AI, which is the focus of the lecture series.
π§ Neural Networks and Pattern Recognition
The discussion shifts to the biological inspiration behind neural networks, which mimic the human brain's vast network of neurons. Each neuron performs a simple pattern recognition task, and when a pattern is detected, it signals other connected neurons. This mechanism is harnessed in AI to recognize complex patterns, such as images. The process of training a neural network involves adjusting the network's connections using training data until it can reproduce the desired output. The lecture also highlights the importance of data in training these networks and the significant advancements made possible by the availability of big data and increased computing power.
π The Rise of Big Data and AI
The lecture explores the concept of big data and its impact on AI, particularly in the training of neural networks. The rise of deep learning, the abundance of data, and affordable computing power are credited with the significant progress in AI. Training a neural network involves adjusting its structure to produce the desired output when presented with training data. The lecture also discusses the role of GPUs in training large neural networks and the commercial interest from Silicon Valley, leading to substantial investments in AI technology.
π The Internet as a Training Ground for AI
The lecture describes the process of training AI systems using vast amounts of data from the internet. The training data for a system like GPT3 consists of 500 billion words from various sources, including web pages, PDF documents, and more. This data is used to train the AI in language tasks, such as predicting the next word in a sequence. The lecture also touches on the legal and ethical considerations surrounding the use of copyrighted material in AI training data.
π€ Limitations and Ethical Concerns of AI
The speaker addresses the limitations of AI, including its tendency to produce incorrect information that is presented in a plausible and fluent manner. The lecture discusses the need for fact-checking and the ethical implications of AI's use, particularly concerning bias, toxicity, and copyright issues. The speaker also highlights the challenges AI faces in understanding and reasoning about complex or abstract concepts, emphasizing that AI lacks the mental processes and consciousness of humans.
π§ The Quest for General Artificial Intelligence
The lecture contemplates the concept of general AI, which would possess a broad range of cognitive abilities similar to humans. It outlines various levels of general AI, from a system capable of any intellectual task to one that can perform any language-based task. The speaker expresses skepticism about the imminence of full general AI, citing the limitations of current technology and the complexities of tasks like loading a dishwasher. The lecture also considers the potential for AI to become 'superhuman' and the ethical considerations this raises.
π The Future of AI and Human Interaction
The lecture concludes with a discussion on the future of AI, suggesting that while large language models are powerful tools, they do not provide deep insights into human nature or mental processes. The speaker also addresses the potential for AI to augment human capabilities, the importance of understanding the brain's functional organization, and the ongoing research into integrating neural and symbolic AI approaches. The lecture ends with a reminder of the Turing Lecture series' upcoming Christmas Lecture and an invitation to follow the Turing Institute for future events.
Mindmap
Keywords
π‘Artificial Intelligence (AI)
π‘Machine Learning
π‘Neural Networks
π‘Generative AI
π‘Large Language Models (LLMs)
π‘Supervised Learning
π‘Bias and Toxicity
π‘General Artificial Intelligence (AGI)
π‘Transformer Architecture
π‘Model Collapse
π‘Multimodal AI
Highlights
Introduction to the Turing Lecture series, emphasizing its significance as the institute's flagship event featuring world-leading experts in data science and AI.
Hari Sood's role as a research application manager at the Turing Institute, focusing on real-world applications for research outputs.
The Turing Institute's mission to make significant advancements in data science and AI research for societal benefit.
The hybrid nature of the Turing Lecture, combining both in-person and online participation.
The importance of training data in supervised machine learning, illustrated through the example of facial recognition.
The concept of neural networks and their inspiration from the human brain, including the role of neurons and connections.
The rise of big data and affordable computing power as key factors in the advancement of AI, particularly in the training of neural networks.
The introduction of the Transformer Architecture, which has been pivotal for the development of large language models like GPT3.
GPT3's unprecedented scale, with 175 billion parameters, and its training on 500 billion words of ordinary English text.
The practical applications of generative AI, from creating content to aiding creativity and even generating legal filings.
The ethical considerations and potential issues with AI, including the risk of spreading misinformation and the challenge of bias in training data.
The limitations of current AI systems, particularly their lack of understanding and consciousness, despite their powerful autocomplete-like capabilities.
The potential future of AI, including the concept of augmented large language models and the possibility of AI systems that can perform a wide range of cognitive tasks.
The ongoing debate and research around the potential sentience of AI, following high-profile claims and subsequent discussions.
The need for a new science to fully understand and explore the capabilities of large language models, given their emergent properties.
The impact of AI on climate change, both in terms of the energy consumed by large models and the potential for AI to contribute solutions.
The future trajectory of AI, with predictions of more capable language models, multimodal interactions, and generative AI creating personalized content.
Transcripts
Browse More Related Video
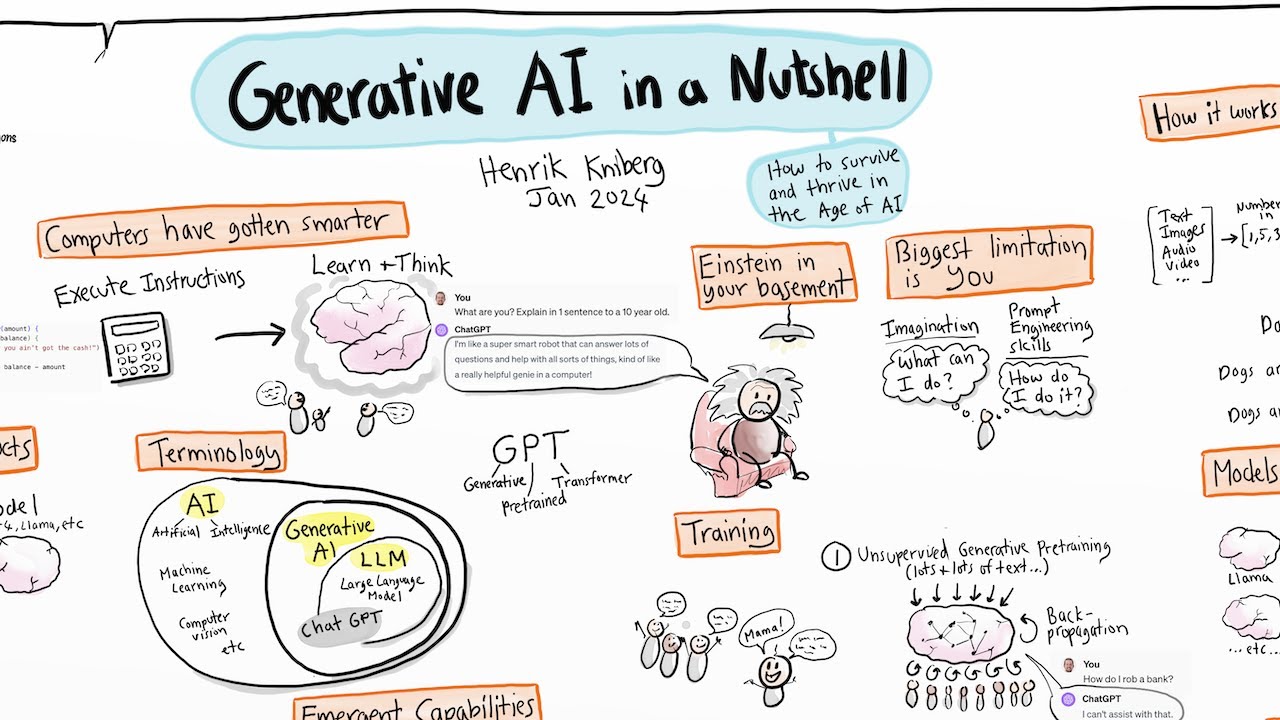
Generative AI in a Nutshell - how to survive and thrive in the age of AI
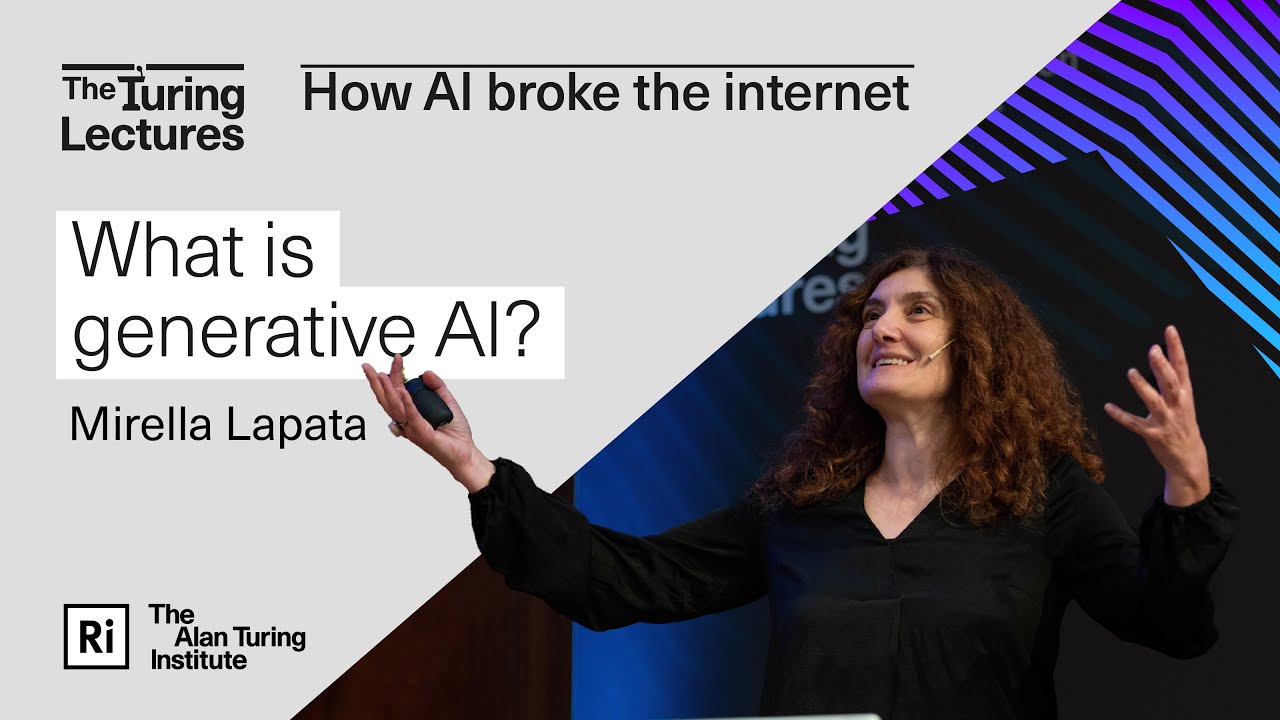
The Turing Lectures: What is generative AI?
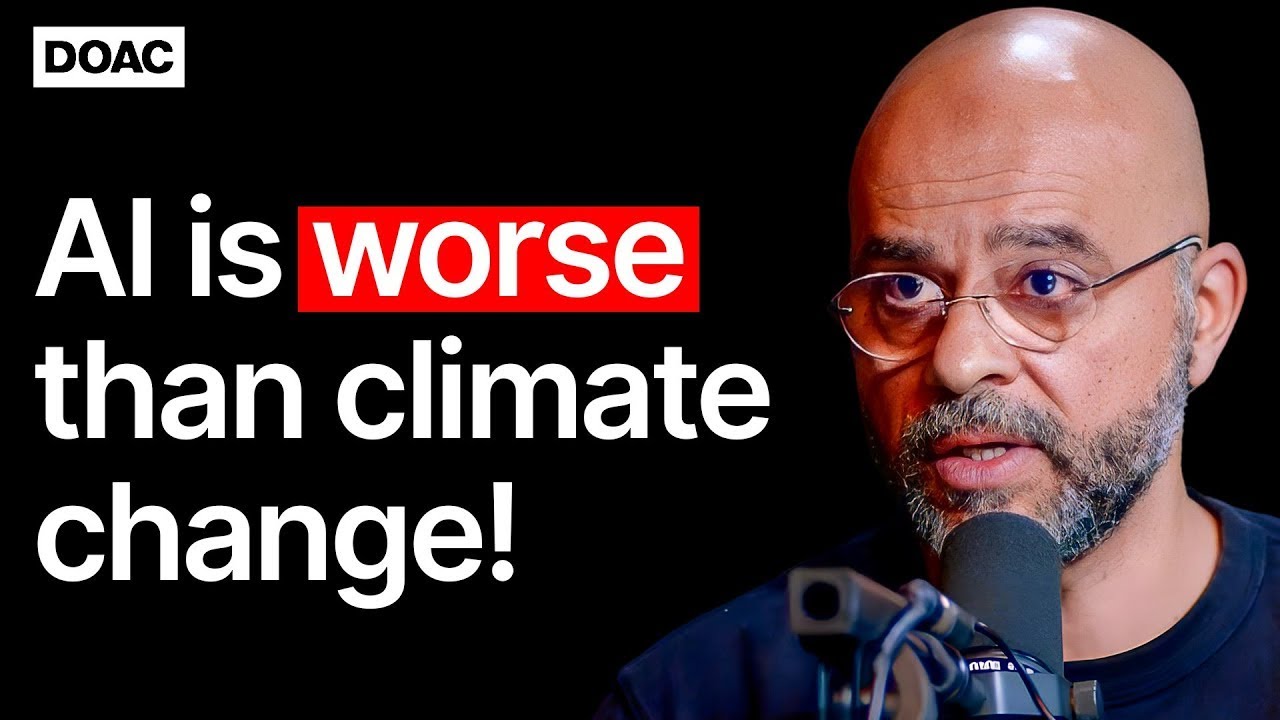
EMERGENCY EPISODE: Ex-Google Officer Finally Speaks Out On The Dangers Of AI! - Mo Gawdat | E252
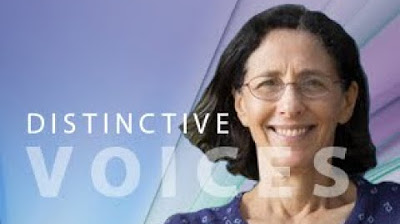
The Future of Artificial Intelligence
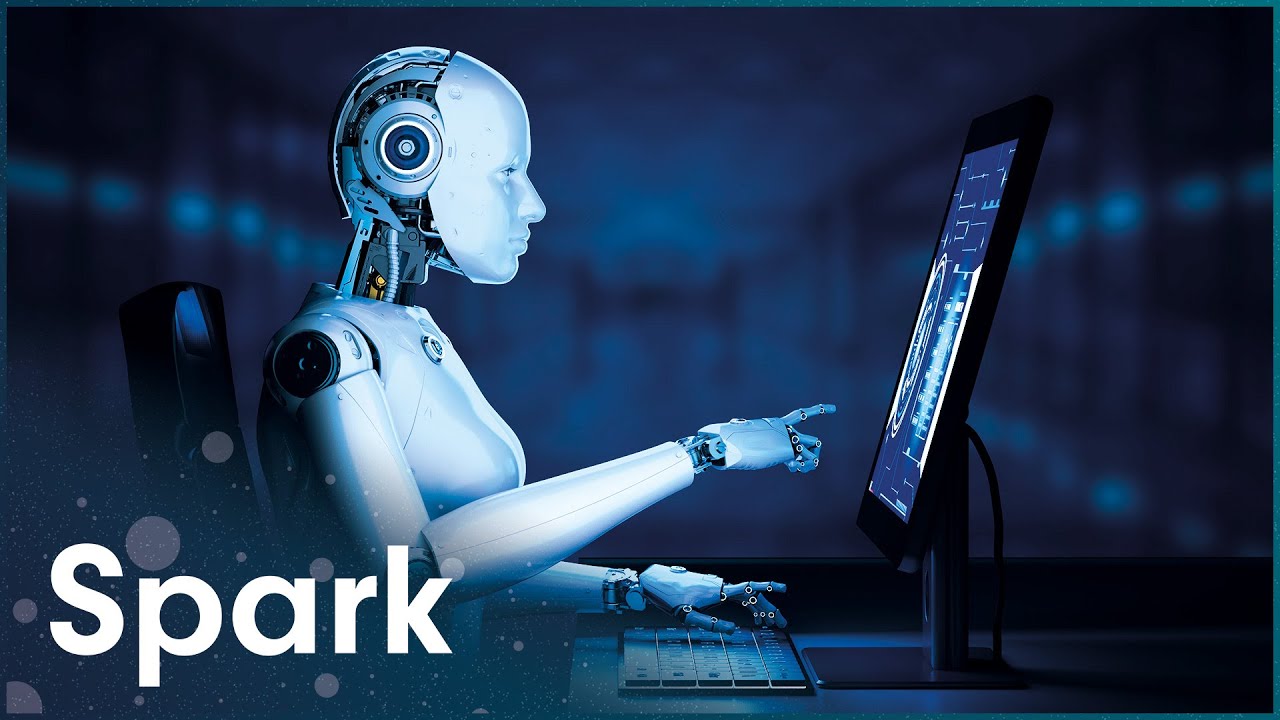
How AI Is Changing The Future Of The Human Race | Spark
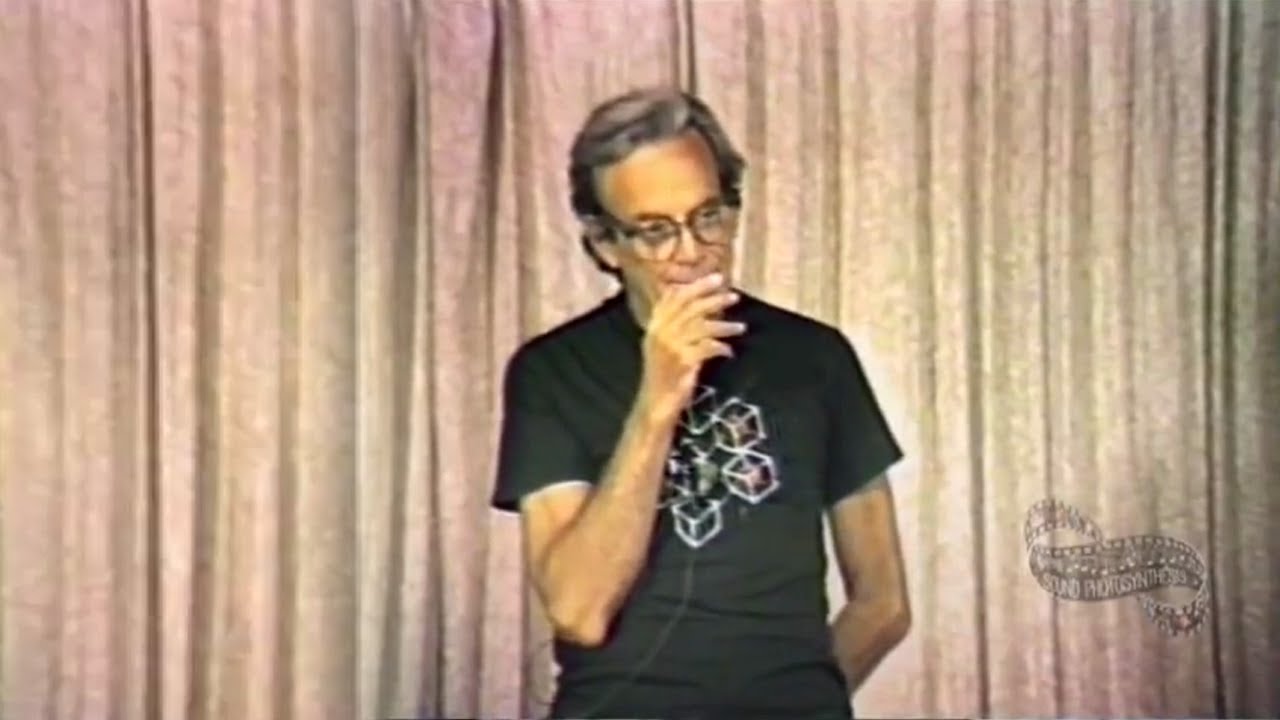
Richard Feynman: Can Machines Think?
5.0 / 5 (0 votes)
Thanks for rating: