R-squared or coefficient of determination | Regression | Probability and Statistics | Khan Academy
TLDRThe video script discusses the concept of finding the best-fit line for a set of data points in a Cartesian coordinate system. It introduces the formula for the line, y = mx + b, and explains how to calculate the squared error, which represents the total variation in y not described by the line. The script then introduces the coefficient of determination, also known as R-squared, which measures the percentage of the total variation in y that is explained by the variation in x. The explanation is clear and engaging, providing a solid foundation for understanding linear regression and its applications.
Takeaways
- π The concept of finding a line that minimizes the squared distance between data points is introduced, which is the basis for linear regression analysis.
- π The line of best fit is described by the equation y = mx + b, where m is the slope and b is the y-intercept.
- π§ The squared error, or the difference between the actual data points and the predicted values on the line, is a measure of how well the line fits the data.
- π The total variation in y-values is calculated by summing the squared differences between each y-value and the mean of all y-values.
- π― The coefficient of determination, also known as R-squared, is a statistical measure that represents the proportion of the variance for the dependent variable that's explained by the independent variable(s).
- π A high R-squared value (close to 1) indicates that a large proportion of the variance in the dependent variable is explained by the independent variable(s), suggesting a good fit of the model.
- π A low R-squared value (close to 0) implies that the independent variable(s) explain little of the variance in the dependent variable, indicating a poor fit of the model.
- π The R-squared value is calculated as 1 minus the ratio of the sum of squared residuals (errors of the regression line) to the total sum of squares (variation of y-values around their mean).
- π The script emphasizes the importance of understanding the relationship between the variation in the dependent variable and the independent variable(s) in regression analysis.
- π The process of calculating the regression line and R-squared is demonstrated through a step-by-step explanation, providing clarity on how to interpret these statistical measures.
- π₯ The script concludes with a preview of applying these concepts to actual data samples in the subsequent video, highlighting the practical application of the discussed theories.
Q & A
What is the main concept discussed in the video?
-The main concept discussed in the video is the relationship between n points with x and y coordinates and finding a line that minimizes the squared distance to these points, known as the regression line.
How is the equation of the regression line represented?
-The equation of the regression line is represented as y = mx + b, where m is the slope and b is the y-intercept.
What is the term used to describe the error between the line and a point?
-The error between the line and a point is referred to as the squared error.
What is the total squared error?
-The total squared error is the sum of the squared distances (errors) between each data point and the regression line.
How is the total variation in y calculated?
-The total variation in y is calculated by summing the squares of the differences between each y value and the mean of all y values.
What does the coefficient of determination measure?
-The coefficient of determination, also known as R-squared, measures the percentage of the total variation in y that is explained by the variation in x as described by the regression line.
What does a high R-squared value indicate?
-A high R-squared value indicates that a large proportion of the variation in y is explained by the variation in x, suggesting that the regression line is a good fit for the data points.
What does a low R-squared value indicate?
-A low R-squared value indicates that a small proportion of the variation in y is explained by the variation in x, suggesting that the regression line is not a good fit for the data points.
How is the coefficient of determination (R-squared) calculated?
-The coefficient of determination (R-squared) is calculated as 1 minus the ratio of the total squared error of the regression line to the total variation in y (the squared error from the mean of y).
What will be discussed in the next video?
-In the next video, the discussion will involve looking at actual data samples, calculating their regression line, and determining their R-squared to assess the quality of the fit.
Why is understanding the concept of the regression line and R-squared important?
-Understanding the concept of the regression line and R-squared is important because it allows us to quantify the relationship between variables and assess how well one variable explains the variation in another, which is crucial in statistical analysis and modeling.
Outlines
π Introduction to Linear Regression
This paragraph introduces the concept of linear regression with n points, each having x and y coordinates. It explains the process of finding a line that minimizes the squared distance to all the points, known as the least squares method. The line is represented as y = mx + b, where m is the slope and b is the y-intercept. The paragraph also reviews the concept of squared error, which is the difference between the line and each point, and how it contributes to the total squared error. The goal is to find the line that best fits the data points by minimizing this error.
π Calculating Total Variation and Error
This paragraph delves into the calculation of total variation in y values and how it relates to the error of the regression line. It explains that the total variation is the sum of the squared distances of each y value from the mean of all y values. The paragraph then contrasts this with the squared error of the line, which measures how much of the total variation is not described by the regression line. The key takeaway is understanding that the squared error from the average represents the unexplained variation, while the remainder of the total variation is explained by the line.
π’ Interpreting the Coefficient of Determination (R-squared)
The final paragraph discusses the coefficient of determination, also known as R-squared, which is a measure of how well the regression line fits the data. It explains that if the squared error of the line is small, it indicates a good fit, and thus a large portion of the variation in y is described by the variation in x. Conversely, a large squared error indicates a poor fit and a small portion of the variation is described by the line. The paragraph emphasizes the practical significance of R-squared in interpreting the results of a regression analysis and provides a preview of applying these concepts to actual data in the next video.
Mindmap
Keywords
π‘n points
π‘best-fit line
π‘squared distance
π‘total variation
π‘squared error
π‘coefficient of determination
π‘variance
π‘regression line
π‘R-squared
π‘linear regression
π‘least squares method
Highlights
The concept of finding a line that minimizes the squared distance to a set of points is introduced.
The line of best fit is represented by the equation y = mx + b, where m is the slope and b is the y-intercept.
The total squared error between the points and the line is referred to as the squared error.
The method to calculate the squared error for an individual point is explained by subtracting the y-value of the line at the x-coordinate of the point from the actual y-value of the point and squaring the result.
The total squared error is the sum of the squared errors for each point.
The concept of total variation in y is defined as the sum of the squared distances of each y-value from the mean of all y-values.
The variation in y not described by the regression line is measured by the squared error of the line.
The coefficient of determination, also known as R-squared, is introduced as a measure of how well the regression line fits the data.
R-squared is calculated as 1 minus the ratio of the squared error of the line to the total variation in y.
A high R-squared value indicates that a large proportion of the variation in y is explained by the variation in x.
A low R-squared value suggests that the regression line does not fit the data well, and a small proportion of the variation in y is explained by the variation in x.
The transcript discusses the practical application of these concepts by mentioning that future videos will include calculations of regression lines and R-squared for actual data samples.
The importance of understanding the relationship between the variation in x and y is emphasized for accurately modeling data.
The process of calculating the squared error from the average helps in visualizing the total variation in y.
The transcript provides a clear explanation of how the total variation in y can be measured and used to evaluate the effectiveness of the regression line.
The concept of variance is related to the total variation in y, providing a statistical context for the discussion.
The transcript explains the mathematical relationship between the squared error of the line and the total variation not described by the regression line.
Transcripts
Browse More Related Video
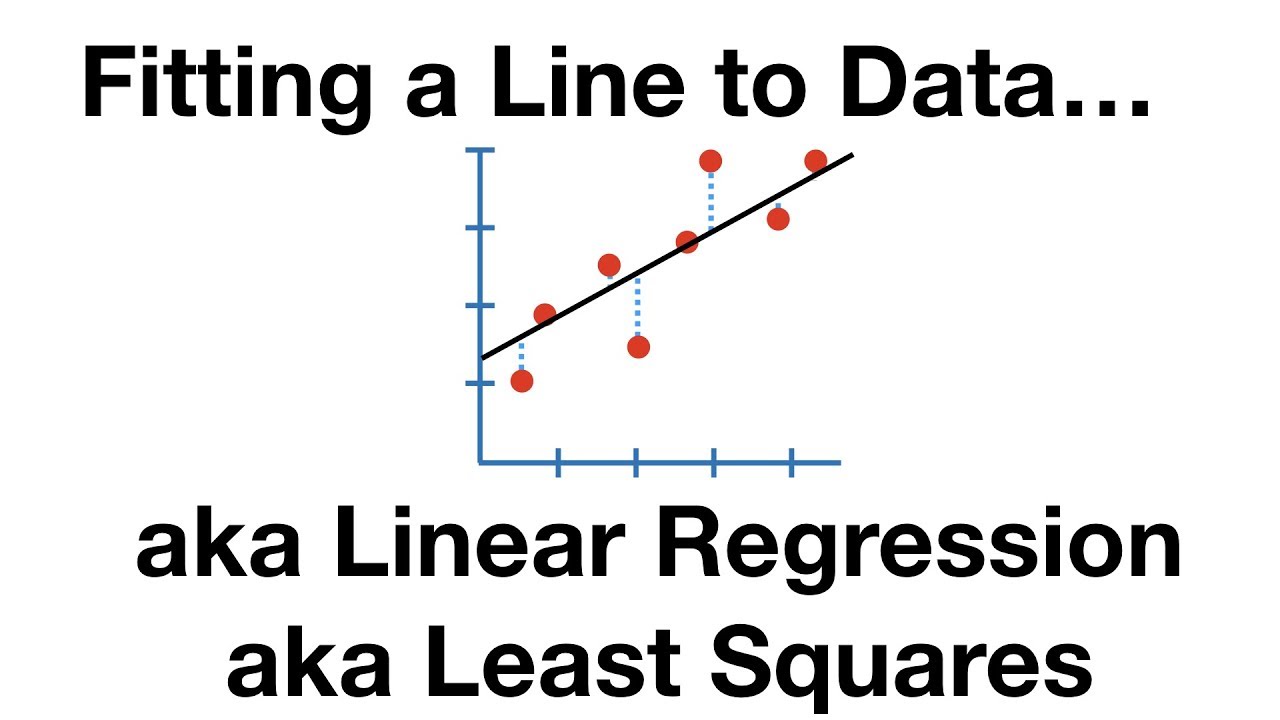
The Main Ideas of Fitting a Line to Data (The Main Ideas of Least Squares and Linear Regression.)
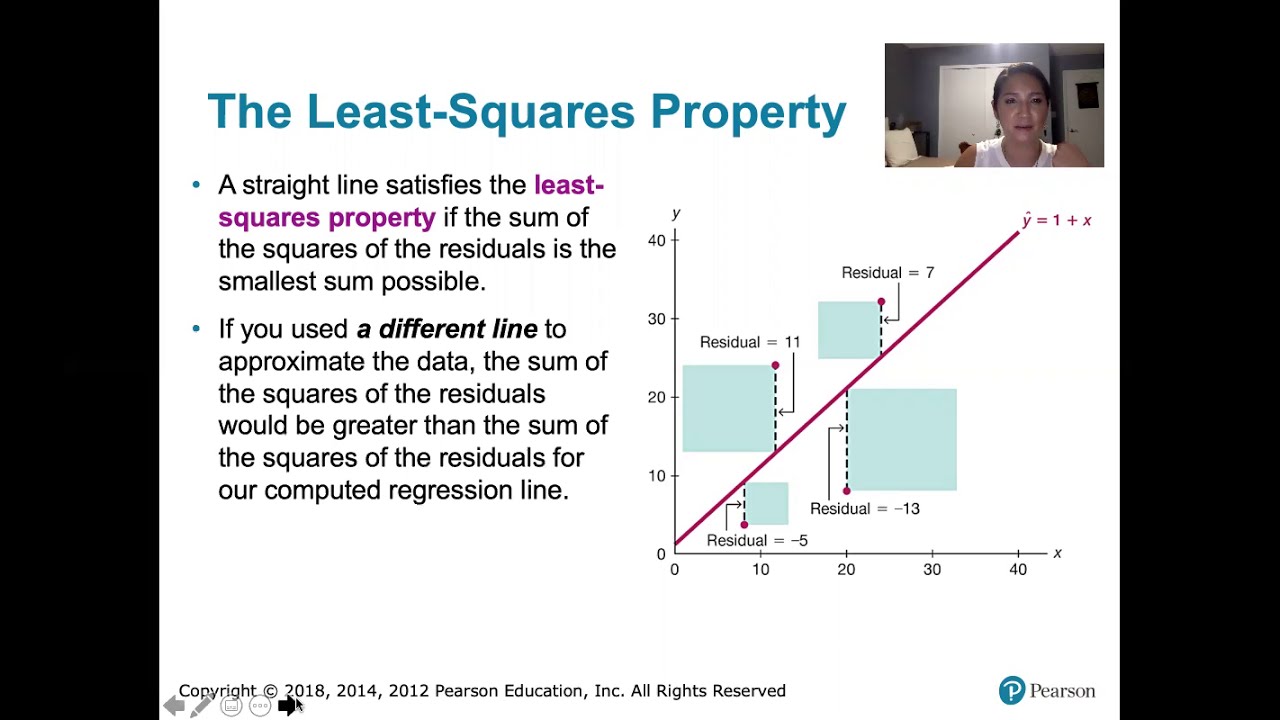
10.2.5 Regression - Residuals and the Least-Squares Property
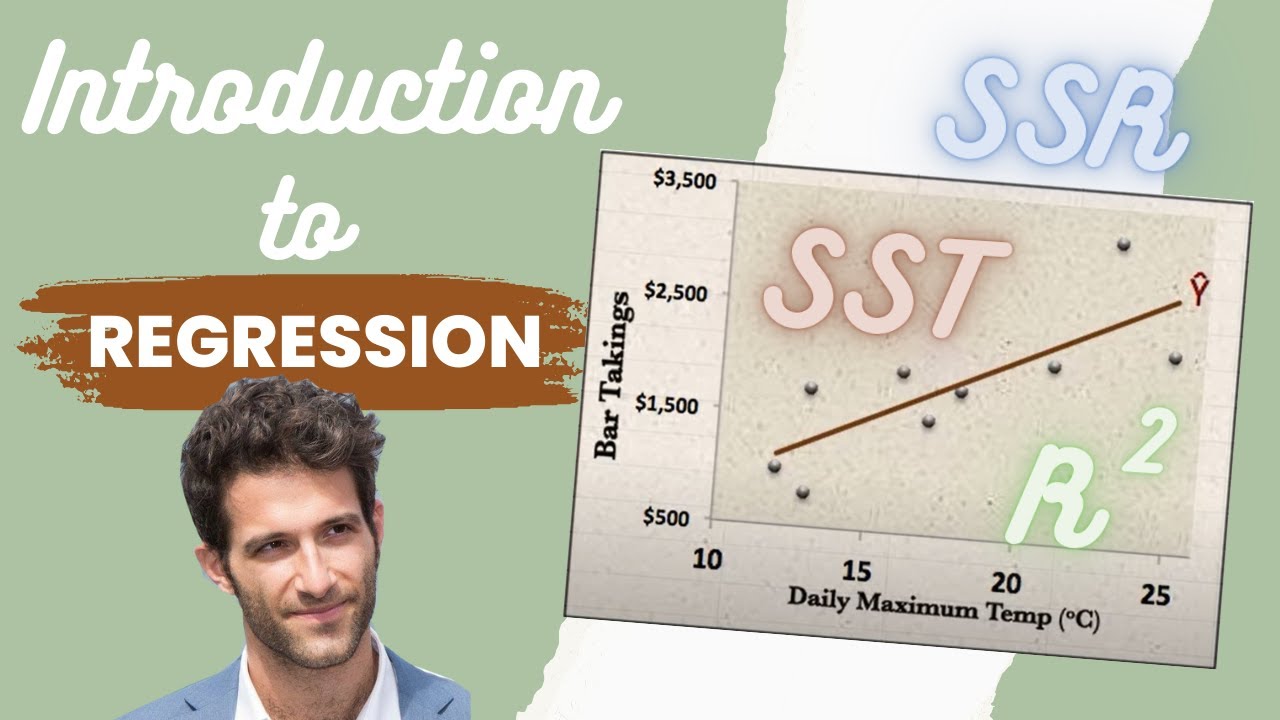
Introduction to REGRESSION! | SSE, SSR, SST | R-squared | Errors (Ξ΅ vs. e)

Interpreting Graphs in Chemistry
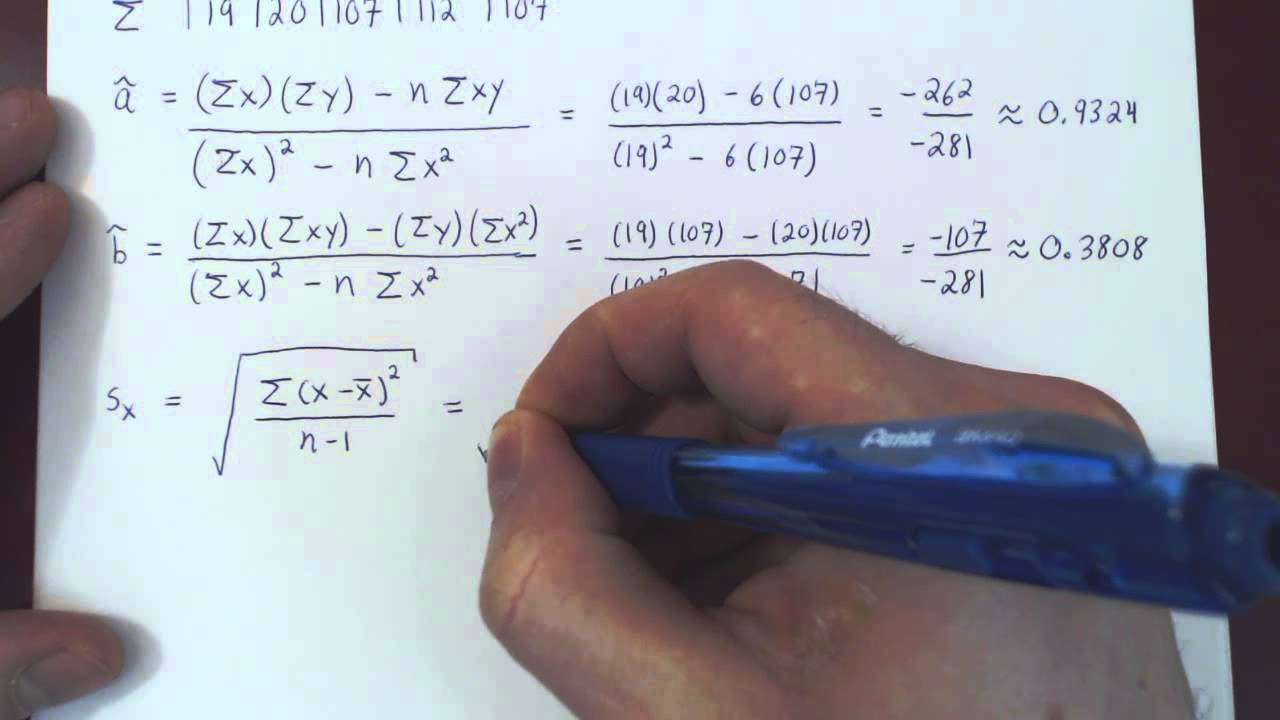
Linear Regression and Correlation - Example
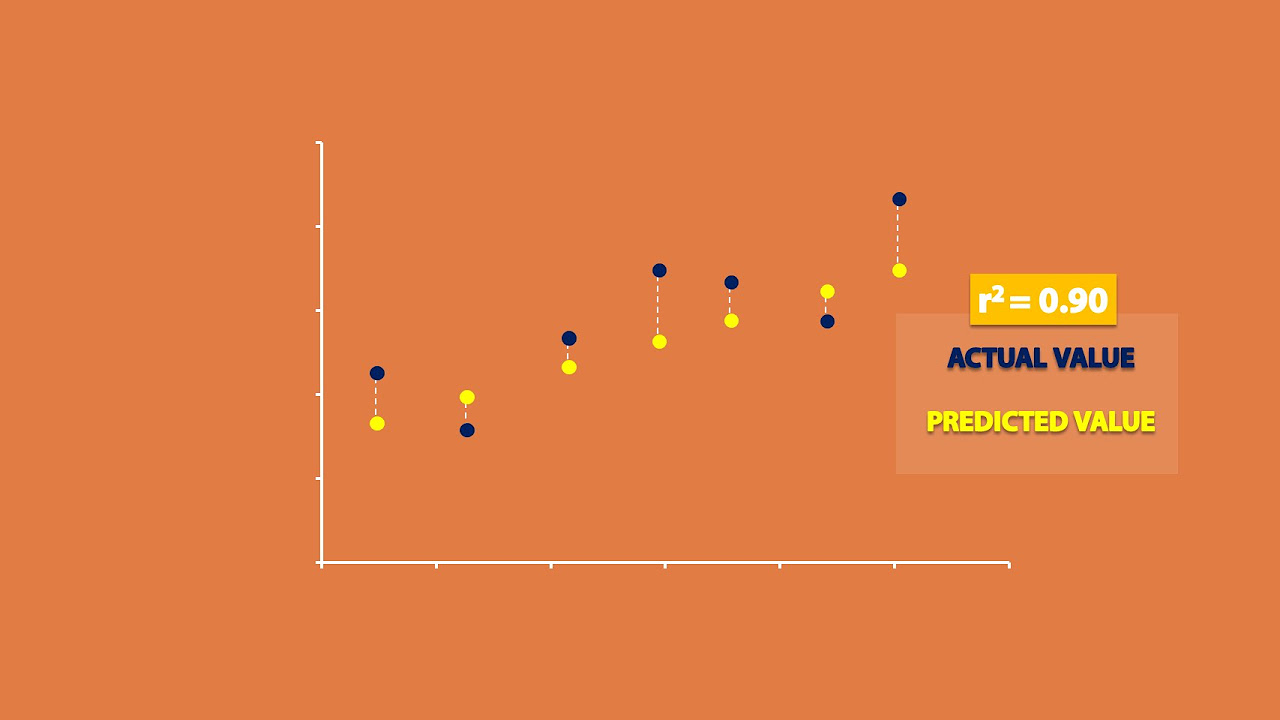
Regression and R-Squared (2.2)
5.0 / 5 (0 votes)
Thanks for rating: