Standard Deviation & Mean Absolute Deviation Explained - 6-8-19]
TLDRThe lesson focuses on understanding the concepts of mean absolute deviation and standard deviation, which are crucial for analyzing data sets. It explains how to calculate these measures and their significance in determining the spread of data around the mean. The video uses real-life examples, such as classroom grades and tree heights, to illustrate the concepts and emphasizes the importance of knowing not only the center of the data but also its dispersion. The mean absolute deviation is calculated by finding the average of the absolute deviations of data points from the mean, while the standard deviation involves squaring the deviations, averaging them, and then taking the square root to maintain the original units of measurement.
Takeaways
- ๐ Understanding data involves finding the mean (center) and the spread (deviation) of a data set.
- ๐ Mean Absolute Deviation (MAD) measures the average distance of data points from the mean, without considering direction.
- ๐ To calculate MAD, find the absolute deviations of each data point from the mean, sum them up, and divide by the number of data points.
- ๐ข Even sets of data with the same mean can have different spreads, hence the need for deviation measures to fully understand data.
- ๐ Standard Deviation is another measure of spread similar to MAD, but it involves squaring the deviations before averaging and then taking the square root.
- ๐ค MAD is easier to understand and calculates the average distance from the mean, while Standard Deviation is more commonly used in statistical analysis.
- ๐ When calculating MAD or Standard Deviation, negative deviations become positive after taking the absolute value, simplifying the calculations.
- ๐ The mean of a data set is calculated by adding all data points and dividing by the number of points.
- ๐ Both MAD and Standard Deviation provide insights into how data is clustered around the mean, but they do so in slightly different ways.
- ๐ In real life, these statistical measures help condense large data sets into understandable information about central tendency and dispersion.
- ๐ Understanding and applying these statistical concepts enables better interpretation and communication of data findings.
Q & A
What is the main focus of the lesson?
-The main focus of the lesson is to understand the concepts of mean absolute deviation and standard deviation, and how they help in understanding the spread of a data set around the mean.
What are the two key aspects of a data set that statistics aims to understand?
-The two key aspects of a data set that statistics aims to understand are the center of the data set (the mean) and how spread out the data set is (the deviation).
How does the mean absolute deviation help in understanding a data set?
-Mean absolute deviation helps in understanding how spread out the data is around the mean by calculating the average distance of the data points from the mean, disregarding the direction (positive or negative) of the deviation.
What is the difference between mean absolute deviation and standard deviation?
-Mean absolute deviation is the average of the absolute values of the deviations of data points from the mean, while standard deviation is the square root of the average of the squared deviations of data points from the mean. Standard deviation is more commonly used in actual statistics and math.
Why is it important to know both the mean and the deviation of a data set?
-Knowing both the mean and the deviation of a data set provides a more complete understanding of the data. The mean gives the center of the data, while the deviation indicates how spread out the data is around the mean. This helps in making more informed conclusions about the data set.
How do you calculate the mean absolute deviation of a data set?
-To calculate the mean absolute deviation, first find the mean of the data set. Then, for each data point, find the absolute deviation from the mean (without considering the sign). Finally, average these absolute deviations to get the mean absolute deviation.
What is the significance of a high mean absolute deviation in a data set?
-A high mean absolute deviation indicates that the data points are generally far from the mean, suggesting a greater spread or variability in the data set.
What is the significance of a low mean absolute deviation in a data set?
-A low mean absolute deviation indicates that the data points are generally close to the mean, suggesting that the data set has less variability and the values are more consistently clustered around the mean.
How does the presence of outliers affect the mean and mean absolute deviation?
-Outliers can significantly affect the mean, often pulling it away from the central tendency of the rest of the data. They also increase the mean absolute deviation as they represent extreme distances from the mean.
Can you provide an example of how mean absolute deviation and standard deviation are used in real-life scenarios?
-In a classroom setting, the mean absolute deviation and standard deviation of students' grades can provide insights into the overall performance and consistency of scores. A low mean absolute deviation and standard deviation would indicate that most students scored close to the average grade, while a high mean absolute deviation and standard deviation would suggest a wide range of grades, with some students performing much better or worse than others.
Outlines
๐ Introduction to Mean Absolute Deviation and Standard Deviation
This paragraph introduces the concepts of mean absolute deviation and standard deviation, emphasizing their importance in understanding data sets in statistics and math. It explains that while the mean indicates the center of a data set, deviations reveal how spread out the data is around the mean. The discussion includes a real-life example of exam grades in a classroom to illustrate how different distributions of grades can have the same mean but different spreads, highlighting the need to consider both measures for a complete data analysis.
๐ Mean Absolute Deviation: Definition and Calculation
This section delves into the definition of mean absolute deviation, explaining that it measures how much data points deviate from the mean, regardless of whether they are above or below it. The word 'absolute' signifies that both positive and negative deviations are considered without their signs. The paragraph provides a step-by-step guide on calculating mean absolute deviation using a simple data set of tree heights, illustrating how to find the mean, calculate individual deviations, and then average these deviations to find the mean absolute deviation.
๐ Interpreting Mean Absolute Deviation
This paragraph focuses on interpreting the mean absolute deviation. It clarifies that this measure provides an average distance of data points from the mean, indicating where most of the data lies in relation to the mean. The explanation includes a visual representation, showing that if the mean absolute deviation is 2, most data points will fall within plus or minus 2 units of the mean. The paragraph also discusses the practical significance of knowing the mean absolute deviation, as it offers insights into the data distribution that the mean alone cannot provide.
๐งฎ Calculating Mean Absolute Deviation with Example Data
This section presents a detailed example of calculating mean absolute deviation using the ages at which individuals had their first child. The paragraph walks through the process of finding the mean, determining deviations from the mean, applying absolute values to these deviations, and finally averaging these absolute deviations to arrive at the mean absolute deviation. The example illustrates how this statistical measure helps in understanding the central tendency and dispersion of the given data set.
๐ Standard Deviation: An Alternative Measure
This paragraph introduces standard deviation as an alternative to mean absolute deviation, explaining that it is a widely used measure in statistics and math. The standard deviation is calculated by squaring the deviations from the mean, averaging these squared values, and then taking the square root of the average. The paragraph demonstrates this process using the same data points from the previous example, showing that the standard deviation is slightly larger than the mean absolute deviation, indicating a slightly wider range around the mean. The discussion highlights the close relationship between the two measures and their respective applications.
๐ Mean Absolute Deviation and Standard Deviation: A Comparative Analysis
This section compares and contrasts mean absolute deviation and standard deviation, showing how both measures provide insights into the spread of a data set around the mean. The paragraph reiterates the calculation process for both measures, using the ages of when children get braces as an example. It emphasizes that while both measures are similar, the standard deviation is more commonly used in statistical analysis due to its mathematical properties and ease of interpretation. The summary underscores the importance of understanding both measures for a comprehensive analysis of data distribution.
๐ก๏ธ Applying Mean Absolute Deviation to Temperature Data
The final paragraph applies the concept of mean absolute deviation to a new data set: daily temperatures in Celsius. It guides through the calculation of the mean temperature and the subsequent determination of deviations from this mean for each day. The paragraph then averages these absolute deviations to find the mean absolute deviation, which in this case is 3. This indicates that most of the temperature data falls within a range of plus or minus 3 units from the mean temperature. The summary illustrates the practical application of mean absolute deviation in analyzing and summarizing data, providing a clear understanding of the data's central tendency and dispersion.
Mindmap
Keywords
๐กMean Absolute Deviation
๐กStandard Deviation
๐กMean
๐กData Set
๐กDispersion
๐กDeviation
๐กOutliers
๐กCenter of Data
๐กStatistical Analysis
๐กVariability
Highlights
The lesson focuses on understanding the mean absolute deviation and standard deviation of a data set.
Mean absolute deviation and standard deviation are critical for understanding any data set in math or statistics.
Mean absolute deviation is easier to understand and calculates the average distance data points are from the mean.
Standard deviation is more commonly used in actual statistics and math but is slightly more complex to calculate.
The mean of a data set represents the center of the data, while mean absolute deviation and standard deviation represent how spread out the data is around the mean.
Real-life examples, such as grades in a classroom, can help illustrate the concepts of mean, mean absolute deviation, and standard deviation.
Two data sets can have the same mean but completely different spreads, which is why understanding deviation is crucial.
Mean absolute deviation is calculated by finding the absolute value of the distance each data point is from the mean, then averaging these values.
Standard deviation involves squaring the distances from the mean, averaging them, and then taking the square root of the result.
The concepts of mean absolute deviation and standard deviation provide clarity on the distribution of data around the mean.
Understanding the spread of data is essential for grasping the full picture of a data set rather than just knowing the mean.
The lesson provides a step-by-step guide on how to calculate mean absolute deviation and standard deviation using examples.
The use of absolute values in calculating mean absolute deviation ensures that all deviations are treated as positive, regardless of their direction from the mean.
Squaring the deviations in the calculation of standard deviation allows for the handling of negative values and the preservation of positive values.
Both mean absolute deviation and standard deviation provide insights into the variability of a data set and its relationship to the mean.
The lesson emphasizes the importance of understanding not just the center of the data but also its spread for a comprehensive data analysis.
Transcripts
Browse More Related Video

What is Standard Deviation and Mean Absolute Deviation | Math, Statistics for data science, ML

Standard Deviation and Variance
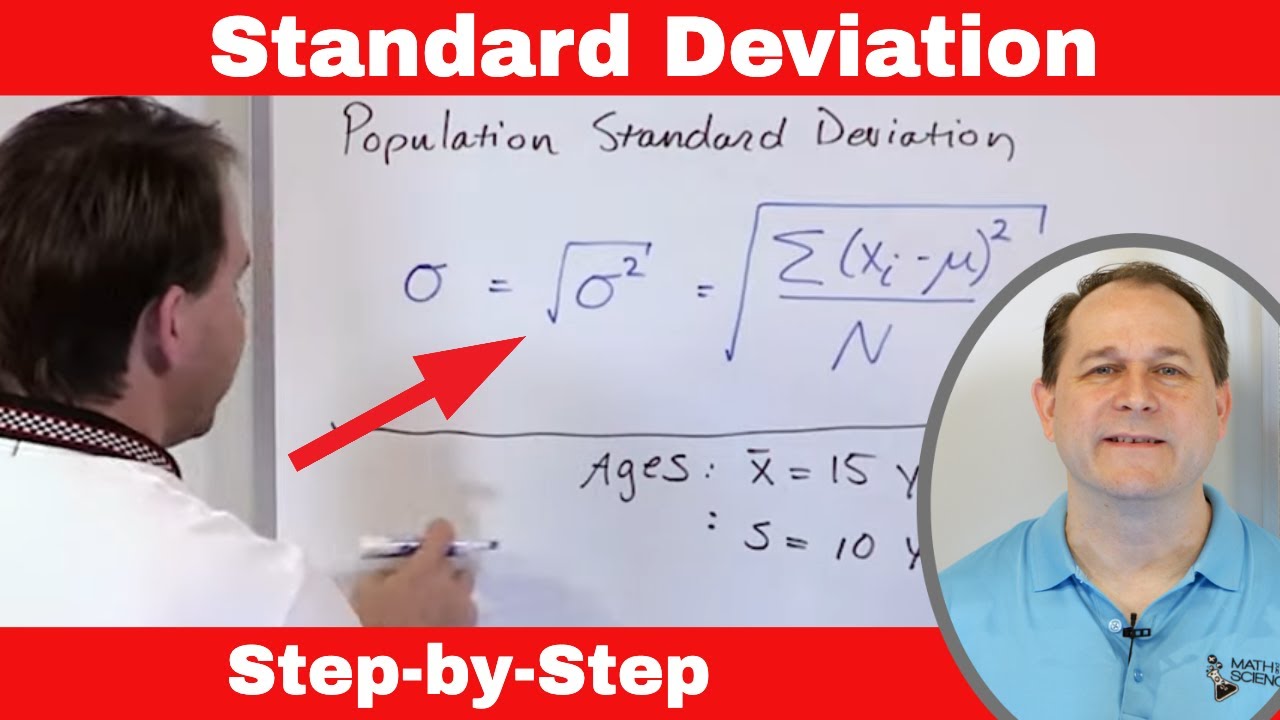
Learn Standard Deviation - Formula & Examples | Step-by-Step
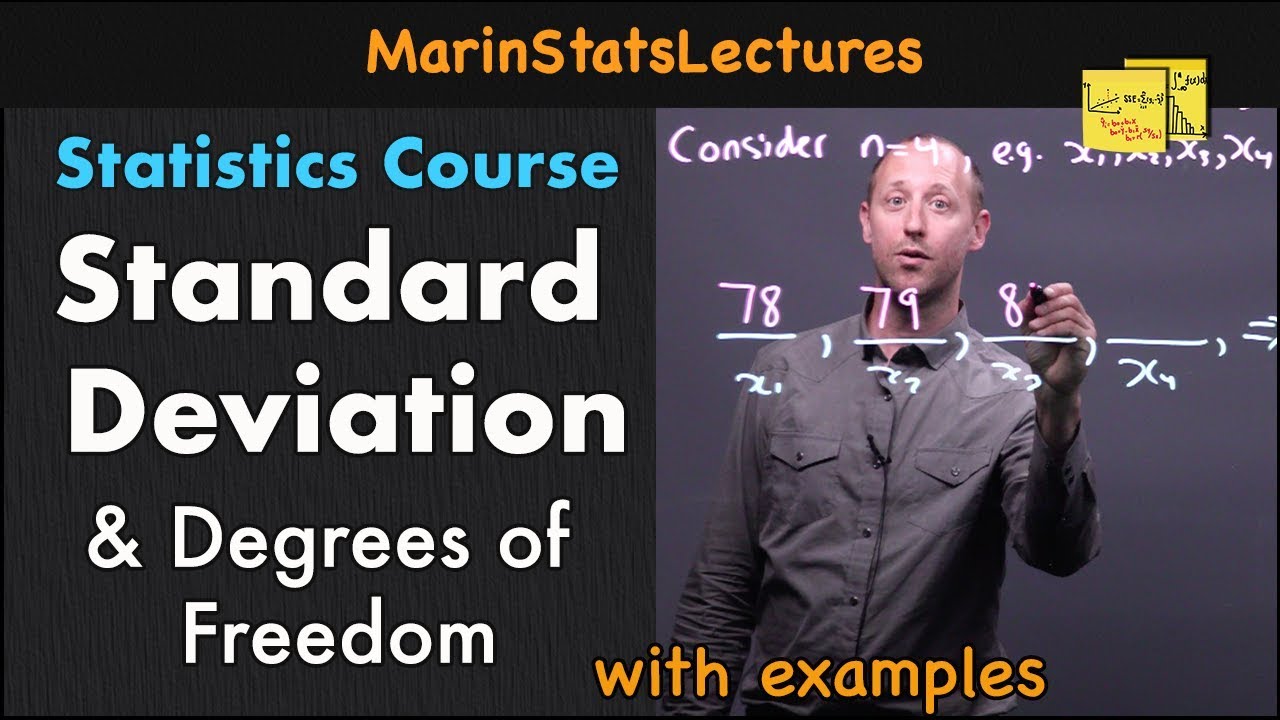
Standard Deviation & Degrees of Freedom Explained | Statistics Tutorial | MarinStatsLectures

Standard Deviation - Explained and Visualized
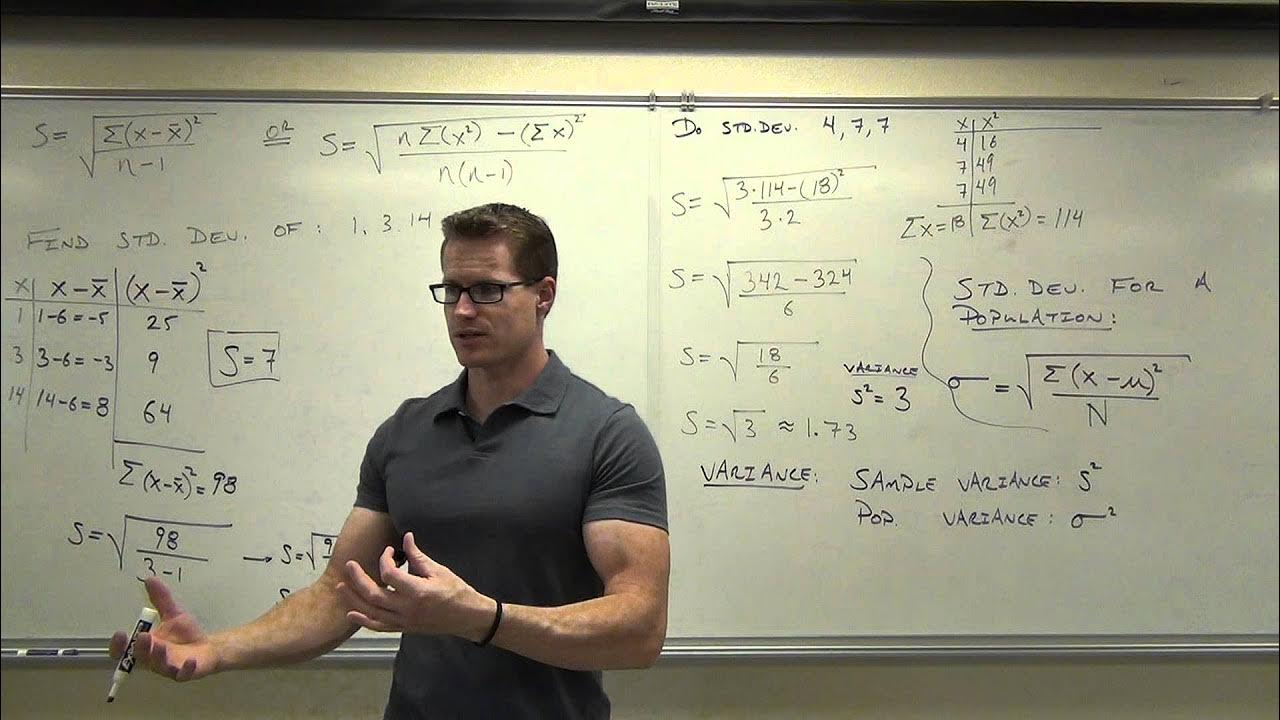
Statistics Lecture 3.3: Finding the Standard Deviation of a Data Set
5.0 / 5 (0 votes)
Thanks for rating: