How to read a Forest Plot in a meta analysis
TLDRIn this educational lecture, Dr. Ashish Kumar, a professor of gastroenterology and hepatology, explains the concept and importance of meta-analysis, specifically focusing on how to interpret forest plots. He describes the forest plot as a visual tool that consolidates results from multiple studies into one figure, illustrating each study's weight and overall evidence. Dr. Kumar details the components of a forest plot, including the horizontal axis for effect size, the vertical line of null effect, individual study lines with confidence intervals, and the summary diamond representing combined effect size. He also discusses the significance of p-values and heterogeneity, particularly the I-squared statistic, in evaluating study outcomes and the reliability of meta-analysis results.
Takeaways
- π A forest plot is a graphical representation used in meta-analysis to summarize the results from multiple studies into one figure.
- π The horizontal axis of a forest plot represents the effect size or outcome effect, which can be odds ratio, relative risk, hazard ratio, etc., depending on the statistics used.
- π The vertical line, known as the line of null effect, is placed at one or zero based on the statistics used and represents the null hypothesis of no difference between intervention and control.
- π Individual studies are represented by horizontal lines with a box indicating the study's result and a horizontal line for the 95% confidence interval.
- π The size of the box corresponds to the weight of the study, typically related to the sample size or number of patients.
- βοΈ A study's result is considered statistically significant if its confidence interval does not cross the line of null effect.
- π The diamond shape represents the combined effect size and confidence interval of all the individual studies when averaged together.
- π Each study on the forest plot is labeled with the lead author's name and year of publication, providing context for the findings.
- π The forest plot includes numerical data such as the number of patients, events in each group, and the actual effect size (e.g., risk ratios or odds ratios).
- π The p-value indicates the significance of the overall effect, and the I-squared value measures heterogeneity among the studies.
- π§ If heterogeneity is high (I-squared value > 50%), additional analysis such as subgroup analysis or exploration of publication bias may be necessary.
- π Subgroup analysis can help explore heterogeneity by categorizing studies based on specific parameters and examining the combined results within each subgroup.
Q & A
What is a forest plot in the context of a meta-analysis?
-A forest plot is a graphical representation used in meta-analysis to summarize the results from multiple studies into one figure. It displays the effect size and 95% confidence intervals for each study, as well as a summary statistic that combines the results of all studies.
Why is the forest plot important in a meta-analysis?
-The forest plot is important because it not only provides statistical data but also indicates the weight of each study, showing how influential each one is in the overall analysis. It helps in understanding whether the intervention or outcome in question yields a clear-cut answer.
What is the significance of the horizontal axis in a forest plot?
-The horizontal axis in a forest plot represents the effect size or outcome effect. The effect size depends on the statistics used in the studies, which can include odds ratio, relative risk, hazard ratio, absolute risk reduction, standardized mean difference, or proportion.
What does the vertical line in a forest plot signify?
-The vertical line in a forest plot, often called the line of null effect, represents the null hypothesis where no difference is expected between the intervention and control groups. Its position depends on the type of statistics used; it's at one for odds ratio or relative risk and at zero for absolute risk reduction or standardized mean difference.
How is the size of the box in a forest plot related to the study it represents?
-The size of the box in a forest plot represents the weight of the study, which is often determined by the number of patients or the sample size. Larger boxes indicate studies with bigger sample sizes, and they carry more weight in the overall analysis.
What does the 95% confidence interval signify in a forest plot?
-The 95% confidence interval in a forest plot indicates the range within which the true effect size is likely to lie with 95% certainty. It provides an estimate of the precision of the study results, with narrower intervals suggesting more precise studies.
What does it mean if a study's confidence interval line crosses the line of null effect?
-If a study's confidence interval line crosses the line of null effect, it suggests that the study's result is statistically not significant for that treatment outcome, meaning there is no clear difference between the intervention and control groups.
What is the purpose of the diamond shape in a forest plot?
-The diamond shape in a forest plot represents the summary of all the studies. It shows the combined effect size and its 95% confidence interval, providing an overall estimate of the intervention's effect based on the pooled data from all studies.
How can the heterogeneity of studies be assessed in a forest plot?
-Heterogeneity among studies in a forest plot can be assessed using the I-squared statistic. A lower I-squared value indicates less heterogeneity and more consistency among the studies, with values less than 50% generally considered acceptable.
What is the role of subgroup analysis in addressing heterogeneity in a meta-analysis?
-Subgroup analysis is used to explore the reasons behind heterogeneity by dividing studies into different groups based on certain parameters. This can help identify why there is variability in study results and provide a clearer understanding of the intervention's effect on specific subgroups.
Why might the line of null effect be omitted in a forest plot?
-The line of null effect might be omitted in a forest plot, especially when dealing with proportions, where the concept of a null effect does not apply as it would with other statistics. Proportions are inherently bounded between 0 and 1, making the line of null effect unnecessary.
Outlines
π Introduction to Forest Plots in Meta-Analysis
Dr. Ashish Kumar, a professor of gastroenterology and hepatology, introduces the concept of forest plots in meta-analysis. He explains that forest plots are crucial for summarizing results from multiple studies into a single figure, which not only shows statistical data but also indicates the importance or weight of each study. The lecture focuses on the components of a forest plot, starting with the horizontal axis representing the effect size or outcome, which depends on the statistics used, such as odds ratio, relative risk, or hazard ratio. Dr. Kumar also discusses the importance of the line of null effect, which is analogous to the null hypothesis and varies depending on the statistics used.
π Components and Interpretation of Forest Plots
This paragraph delves deeper into the individual components of a forest plot. It describes how each study's result is represented by a horizontal line with a box indicating the study's main result and a horizontal line for the 95% confidence interval. The size of the box corresponds to the study's weight, often related to the sample size. The paragraph explains how the position of these lines and boxes helps interpret whether a study's result is statistically significant or not by comparing it to the line of null effect. Additionally, it introduces the concept of the summary diamond, which represents the combined effect size and confidence interval of all studies, indicating the overall result of the meta-analysis.
π Detailed Explanation of Forest Plot Elements
The speaker provides a detailed explanation of the elements found in a forest plot, including the labels for individual studies, the number of patients and events in treatment and control groups, and the actual effect sizes such as risk ratios or odds ratios with their confidence intervals. The paragraph also discusses the importance of the p-value for determining the significance of the overall effect and the heterogeneity of the studies, measured by the i-squared value. It highlights the need for subgroup analysis and exploration of heterogeneity when the i-squared value is high, and mentions the importance of considering publication bias in meta-analysis.
π Examples and Summary of Forest Plot Analysis
The final paragraph provides examples of different types of forest plots, such as those showing standardized mean differences, death incidence, and proportions. It emphasizes the importance of the line of null effect's position depending on the type of outcome being measured. The paragraph also discusses the use of subgroup analysis to explore heterogeneity, as demonstrated by a meta-analysis that grouped studies by gender. The summary reiterates the key points about forest plots, including the representation of individual studies, the significance of the diamond's position, and the importance of the i-squared statistic for assessing heterogeneity. The lecture concludes with an acknowledgment of the audience's patience and an invitation for questions.
Mindmap
Keywords
π‘Meta-analysis
π‘Forest plot
π‘Effect size
π‘Odds ratio
π‘Relative risk
π‘Hazard ratio
π‘Confidence interval
π‘Null effect
π‘Weight of a study
π‘Heterogeneity
Highlights
Dr. Ashish Kumar, a professor of gastroenterology and hepatology, discusses how to read a forest plot in meta-analysis.
Forest plot is a crucial tool in meta-analysis, summarizing results from multiple studies into one figure.
The forest plot displays the effect size, which depends on the statistics used, such as odds ratio or relative risk.
A vertical line in the forest plot, called the line of null effect, represents the null hypothesis of no difference between intervention and control.
Individual studies are represented by horizontal lines with boxes indicating the study's result and lines showing the 95% confidence interval.
The size of the box in a forest plot correlates with the study's weight, often determined by the sample size.
A narrower confidence interval indicates a more precise study, while a wider interval suggests greater uncertainty.
If a study's confidence interval line crosses the line of null effect, the study is statistically insignificant for the treatment outcome.
The diamond shape in the forest plot represents the combined effect size and confidence interval of all individual studies.
The forest plot also includes labels for individual study names, publication years, and detailed statistics such as event counts and total patients.
The p-value indicates the significance of the overall effect, with the diamond's position relative to the null effect line providing a visual representation.
The I-squared value measures heterogeneity among studies, with lower values indicating more consistency.
High heterogeneity may require subgroup analysis to explore differences and understand the reasons behind variability.
Subgroup analysis can help in understanding heterogeneity by categorizing studies based on specific parameters.
Publication bias should also be considered when interpreting forest plots and meta-analysis results.
Examples provided include forest plots for standardized mean difference, incidence of death, and proportions in specific patient populations.
The importance of understanding the position of individual studies and the summary diamond in relation to the line of null effect.
The forest plot's ability to visually represent the combined result of multiple studies and its confidence in the findings.
Dr. Kumar's gratitude towards Dr. Rakesh Agrawal for teaching him meta-analysis and his thanks to the audience for their attention.
Transcripts
Browse More Related Video
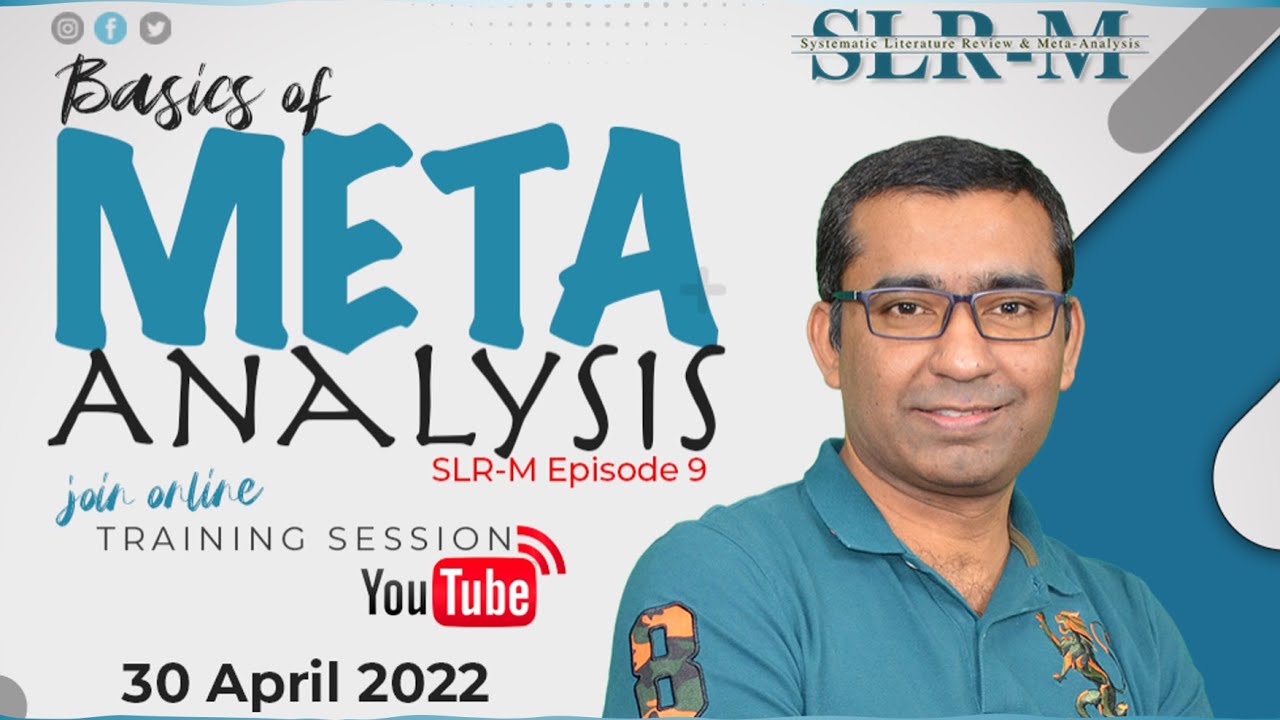
Basics of Meta-Analysis

4 Course Meta-Analyses VU: Calculating and pooling effect sizes

Heterogeneity - Meta-Analysis Workshop Online Video Series Course
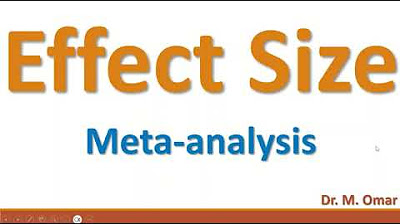
Effect size in meta analysis
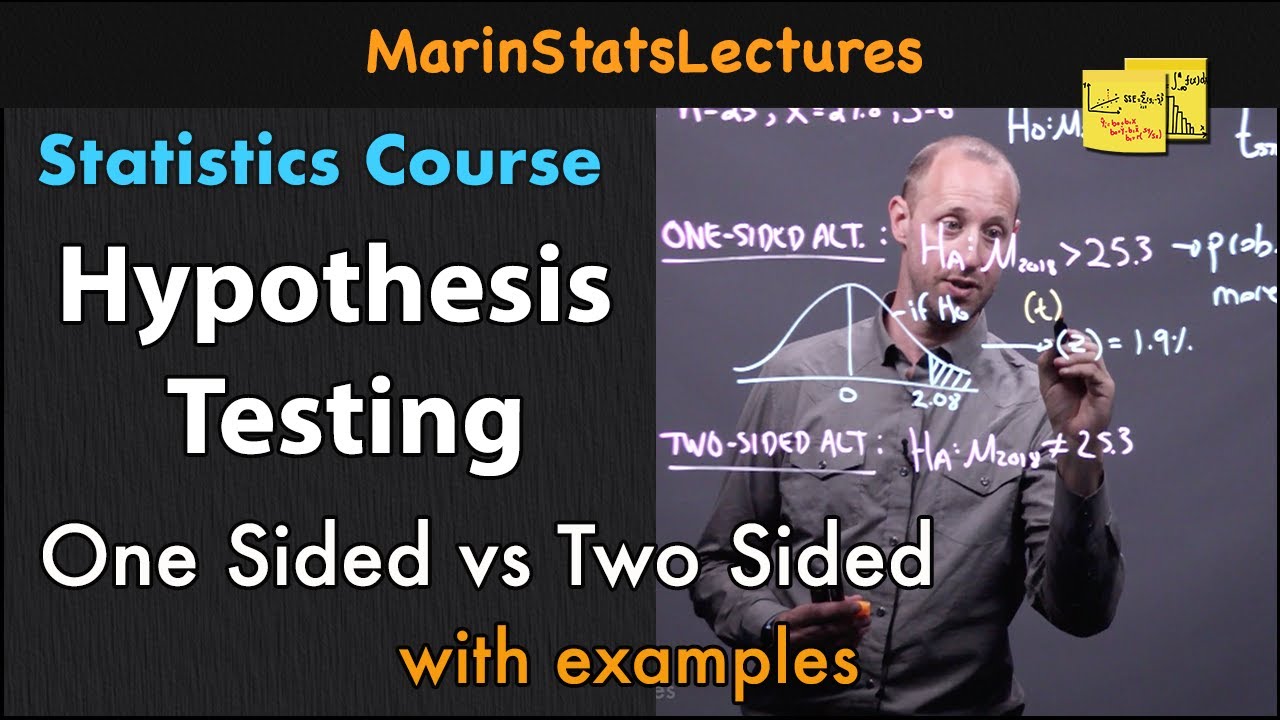
Hypothesis Testing: One Sided vs Two Sided Alternative | Statistics Tutorial #14 |MarinStatsLectures

Significance vs. Effect Size for One Way ANOVA using SPSS
5.0 / 5 (0 votes)
Thanks for rating: