Significance vs. Effect Size for One Way ANOVA using SPSS
TLDRDr. Grande's video offers a detailed tutorial on comparing significance to effect size using SPSS. The example uses fictitious data with an independent variable 'treatment' having two levels (psychodynamic and REBT) and a dependent variable 'depression' measured continuously. The video demonstrates how to conduct an ANOVA to test the null hypothesis and introduces the concept of effect size through partial eta squared, explaining its importance over mere statistical significance. It highlights the practical significance of differences between groups, the impact of sample size on statistical significance, and the comparison of effect sizes across studies. The tutorial also covers how to calculate partial eta squared and interpret its values, emphasizing the need to consider both significance and effect size in data analysis.
Takeaways
- π The video is a tutorial by Dr. Grande on comparing significance to effect size using SPSS.
- π The example data in SPSS includes a continuous dependent variable 'depression' and an independent variable 'treatment' with two levels: psychodynamic and REBT.
- π§ The video demonstrates how to conduct an ANOVA to analyze the significance of the difference between the two treatment groups.
- π The significance level (p-value) obtained from the ANOVA is 0.024, indicating a 2.4% chance of observing the results by random error if the null hypothesis were true.
- β Rejecting the null hypothesis with a p-value less than 0.05 (common alpha level in social sciences) suggests that the two treatment groups are significantly different in terms of depression.
- π Beyond significance, the video emphasizes the importance of effect size to understand the practical importance of the observed difference.
- π Effect size is measured by 'partial eta squared' in this context, which represents the proportion of variance in the dependent variable explained by group membership.
- π The partial eta squared value of 0.106 indicates that 10.6% of the variance in depression scores is due to the treatment type.
- π The video explains that a statistically significant result does not necessarily imply a meaningful difference, which is where effect size plays a crucial role.
- π Effect size can also be used to compare findings across studies and is particularly important when sample sizes are large, as statistical significance can be easily achieved.
- π The tutorial also covers how to calculate effect size using SPSS output and Excel, providing a step-by-step guide for understanding the calculations.
Q & A
What is the purpose of the video by Dr. Grande?
-The purpose of the video is to demonstrate how to compare significance to effect size using SPSS, specifically with a focus on ANOVA.
What type of data does Dr. Grande use in the video?
-Dr. Grande uses fictitious data in the SPSS data editor, with an independent variable named 'treatment' having two levels (psychodynamic and REBT) and a dependent variable 'depression' measured at the interval level.
Why does Dr. Grande choose to perform an ANOVA instead of a t-test despite having only two groups?
-Dr. Grande chooses to perform an ANOVA to compare significance and effect size, rather than just testing for statistical significance with a t-test.
What is the significance level (p-value) obtained in the ANOVA for the treatment variable?
-The significance level (p-value) obtained for the treatment variable in the ANOVA is 0.024, which indicates a 2.4% chance of observing the results by random error if the null hypothesis were true.
What does the p-value of 0.024 suggest about the null hypothesis in this context?
-The p-value of 0.024 suggests that there is a low probability (2.4%) that the observed difference in depression levels between the two treatment groups is due to random error, leading to the rejection of the null hypothesis that the groups are equal.
Why is it important to consider effect size in addition to significance?
-Considering effect size is important because it provides information about the practical significance of the observed difference between groups, which mere statistical significance does not convey.
What additional statistic is provided when Dr. Grande re-runs the ANOVA with options for effect size?
-The additional statistic provided is 'partial eta squared', which measures the proportion of variance in the dependent variable explained by the independent variable.
What is the value of partial eta squared obtained in the example, and what does it represent?
-The value of partial eta squared obtained is 0.106, which represents 10.6% of the variance in depression levels explained by group membership, indicating the effect size.
Why might statistical significance not be enough to determine the importance of a result?
-Statistical significance alone might not be enough because as sample sizes increase, it becomes easier to find statistically significant results that may not have practical importance, which is where effect size comes into play.
How can effect size help in comparing findings across different studies?
-Effect size helps in comparing findings across studies by providing a standardized measure of the importance or magnitude of the difference between groups, allowing for a more meaningful comparison than significance levels alone.
What are the different thresholds for small, medium, and large effect sizes for partial eta squared?
-For partial eta squared, small, medium, and large effect sizes are categorized as follows: small (1% to 6%), medium (6% to 14%), and large (14% or greater).
Outlines
π¬ Introduction to ANOVA and Effect Size in SPSS
Dr. Grande introduces a video on comparing significance to effect size using SPSS. The example uses fictitious data with an independent variable 'treatment' having two levels: psychodynamic and REBT, and a continuous dependent variable 'depression' measured at the interval level. The video demonstrates how to conduct an ANOVA to determine if there's a significant difference between the two treatment groups. The significance level (p-value) of 0.024 indicates a 2.4% chance that the observed difference is due to random error, leading to the rejection of the null hypothesis. However, the video emphasizes that significance alone doesn't convey the importance of the difference, which is where effect size comes into play.
π Understanding Effect Size and Its Importance
This paragraph delves into the concept of effect size, explaining its importance in determining the practical significance of observed differences between groups. It contrasts statistical significance with effect size, noting that large sample sizes can easily yield significant results that may not be meaningful. The paragraph introduces different measures of effect size, such as partial eta squared and Cohen's D, explaining that partial eta squared represents the proportion of variance in the dependent variable explained by group membership. The video provides a step-by-step guide on calculating partial eta squared using SPSS output and Excel. It also discusses the interpretation of effect size values, highlighting the thresholds for small, medium, and large effect sizes. The video concludes by emphasizing the importance of considering both significance and effect size in statistical analysis.
Mindmap
Keywords
π‘ANOVA
π‘Effect Size
π‘Significance Level
π‘Null Hypothesis
π‘Dependent Variable
π‘Independent Variable
π‘Psychodynamic
π‘REBT
π‘Partial Eta Squared
π‘Cohen's D
Highlights
Introduction to the video by Dr. Grande on comparing significance to effect size using SPSS.
Use of fictitious data with an independent variable 'treatment' and a dependent variable 'depression'.
Explanation of the difference between interval and ratio level measurements for continuous variables.
Decision to conduct an ANOVA despite having only two groups to compare significance and effect size.
Description of the general linear model univariate analysis setup in SPSS.
Interpretation of the significance level (p-value) of 0.024 indicating a 2.4% chance of random error.
Discussion on rejecting the null hypothesis when p-value is less than the alpha value (0.05).
Importance of effect size in determining the practical significance of observed differences.
Introduction of partial eta squared as a measure of effect size in ANOVA.
Calculation and interpretation of partial eta squared value of 0.106 indicating 10.6% variance explained.
The difference between statistical significance and practical significance in research.
The impact of increased sample sizes on the ease of finding statistical significance.
Comparison of findings across studies using effect size as a common metric.
Different types of effect sizes, such as partial eta squared and Cohen's D, and their calculations.
Guidelines for interpreting effect sizes as small, medium, or large based on their values.
The importance of considering both significance and effect size in inferential statistics.
Conclusion emphasizing the different purposes of p-value and effect size in statistical analysis.
Transcripts
Browse More Related Video
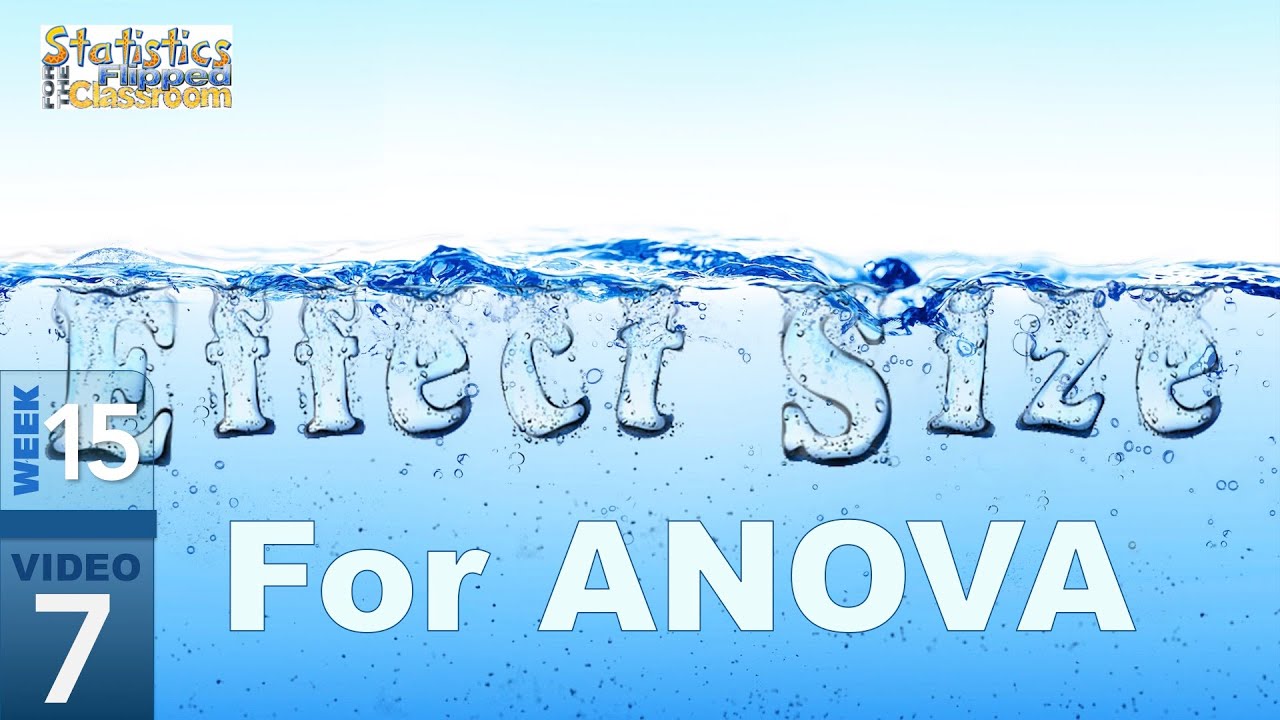
Eta Squared Effect Size for One-Way ANOVA (12-7)
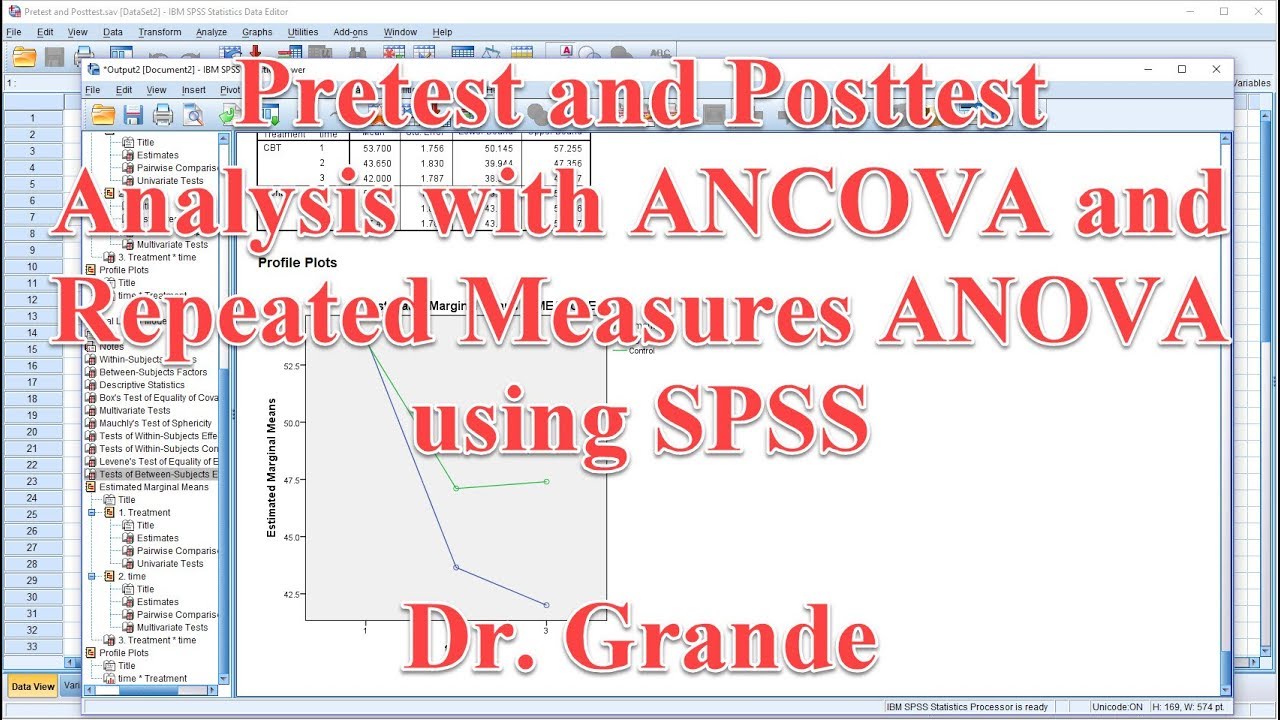
Pretest and Posttest Analysis with ANCOVA and Repeated Measures ANOVA using SPSS

P value versus effect size
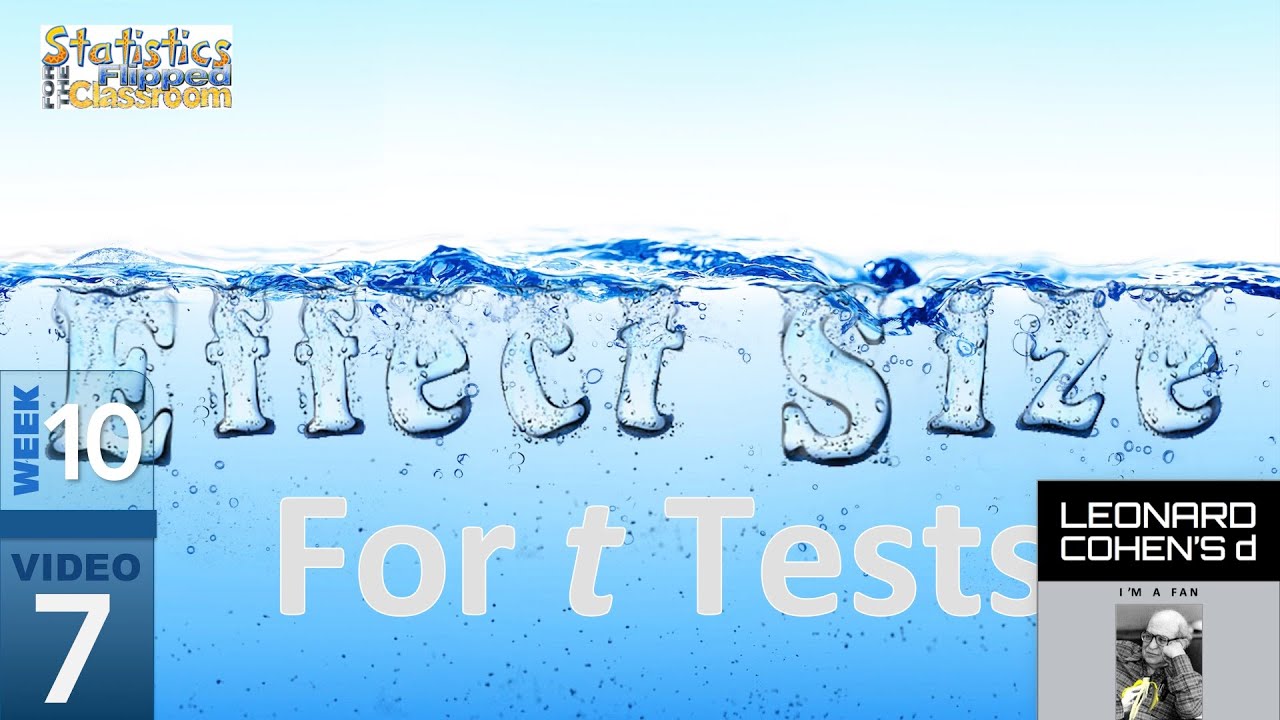
Cohenβs d Effect Size for t Tests (10-7)

One-Way ANOVA with LSD (Least Significant Difference) Post Hoc Test in Excel
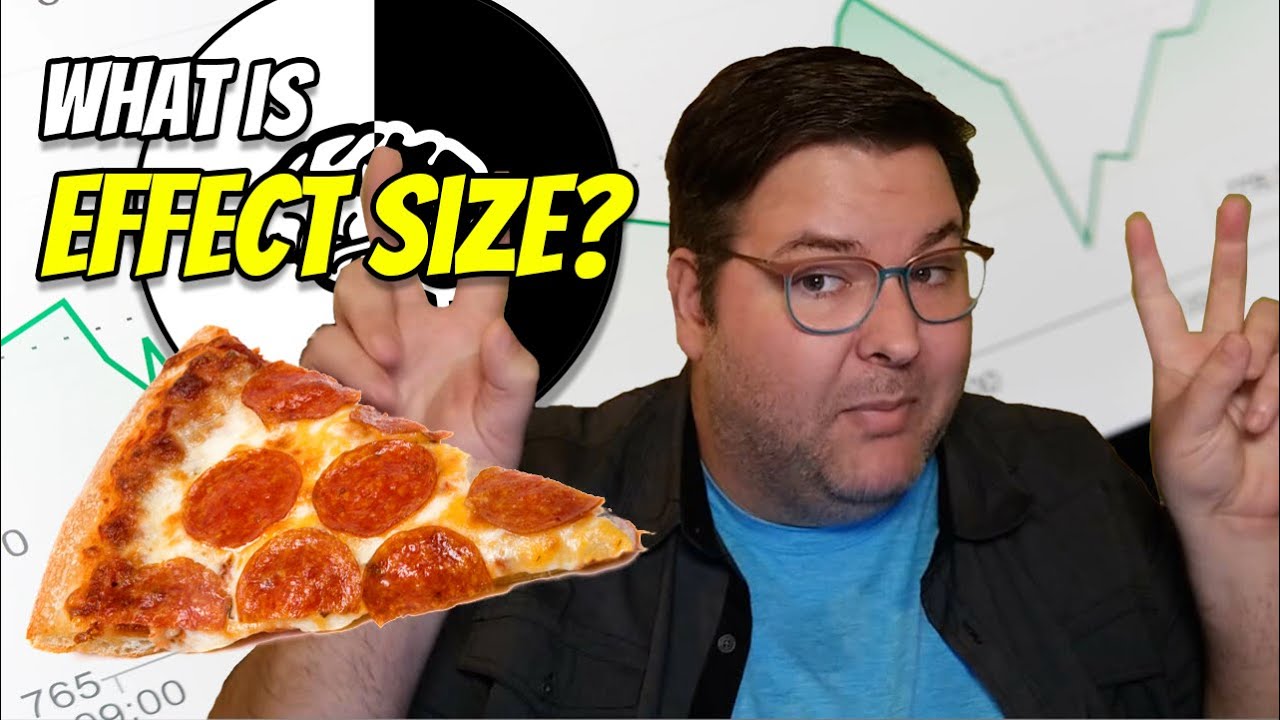
What is Effect Size? Statistical significance vs. Practical significance
5.0 / 5 (0 votes)
Thanks for rating: