p-values: What they are and how to interpret them
TLDRIn this engaging StatQuest episode, Josh Starmer explains the concept of p-values in the context of comparing two drugs, Drug A and Drug B. He uses a hypothetical experiment to illustrate how p-values, which range between 0 and 1, quantify the confidence level that the observed effects are not due to random chance. A commonly used threshold for p-values is 0.05, meaning that if there's no actual difference between the drugs, only 5% of the time would we incorrectly conclude there is a difference (a false positive). Josh clarifies that a small p-value does not necessarily indicate a large effect size; it simply suggests that the observed difference is unlikely to be due to random variation. He also touches on hypothesis testing, where the null hypothesis assumes no difference between the drugs, and the p-value aids in deciding whether to reject this null hypothesis. The episode concludes by emphasizing the importance of p-values in statistical analysis and their role in scientific decision-making.
Takeaways
- π€ P-values are used to determine how confident we can be that there is a difference between two treatments, like Drug A and Drug B.
- π A p-value is a number between 0 and 1 that quantifies the strength of the evidence against the null hypothesis, which assumes no difference between treatments.
- π« A common threshold for p-values is 0.05, meaning that if there is no real difference, only 5% of experiments would incorrectly suggest there is one (false positive).
- π§ A smaller p-value indicates stronger evidence against the null hypothesis, suggesting a more likely real difference between treatments.
- π A p-value of 0.24 suggests that we are not confident that there is a difference between Drug A and Drug B, as it is higher than the commonly used threshold.
- π The size of the p-value does not reflect the size of the difference between treatments; it only indicates the strength of the evidence for a difference.
- 𧬠In hypothesis testing, the null hypothesis typically states that there is no difference between treatments, and a small p-value can lead to its rejection.
- π Repeating the same experiment many times helps to account for random variations and can lead to more reliable p-values.
- π¨ A false positive occurs when a small p-value is obtained when there is actually no difference between treatments, which can happen by chance.
- π‘οΈ Using a smaller threshold for p-values (like 0.00001) reduces the chance of false positives but may not be practical due to increased costs or complexity.
- β±οΈ The choice of p-value threshold depends on the importance of avoiding false positives; a higher threshold (like 0.2) is acceptable when the stakes are lower.
- π Understanding p-values is crucial for interpreting the results of experiments and making informed decisions based on statistical evidence.
Q & A
What is the main topic of the video?
-The main topic of the video is to explain what p-values are and how to interpret them in the context of statistical analysis.
Why is it not sufficient to test two drugs on just one person each?
-It is not sufficient because there could be many different reasons for the outcome, such as medication interactions, allergies, improper dosage, or placebo effects, which could skew the results.
What does a p-value represent in statistical terms?
-A p-value is a number between 0 and 1 that quantifies how confident we should be that there is a difference between two treatments or conditions.
What is the commonly used threshold for p-values in statistical analysis?
-The commonly used threshold for p-values is 0.05, which means that if there is no difference between the conditions, only 5% of the experiments would result in a wrong decision.
What is the null hypothesis in hypothesis testing?
-The null hypothesis is the default assumption that there is no difference between the conditions or treatments being tested.
What is a false positive in the context of p-values?
-A false positive occurs when a small p-value is obtained even though there is no actual difference between the conditions, which happens when random chance leads to significant results.
How does the p-value help in deciding whether to reject the null hypothesis?
-A small p-value (typically less than the chosen threshold, such as 0.05) indicates strong evidence against the null hypothesis, suggesting that we should reject it.
What does a p-value not tell us about the difference between two treatments?
-A p-value does not tell us the size of the difference between the treatments; it only indicates the probability of observing the data under the null hypothesis.
Why might a study with a larger effect size have a larger p-value than expected?
-A study with a larger effect size might have a larger p-value if the sample size is small, leading to less statistical power and a higher chance of random variation affecting the results.
What is the significance of a p-value of 0.24 in the context of the experiment?
-A p-value of 0.24 suggests that there is not enough evidence to conclude that drug A is different from drug B, as it is higher than the commonly used threshold of 0.05.
How can the threshold for p-values be adjusted to account for the importance of the experiment?
-The threshold for p-values can be adjusted lower (e.g., 0.00001) for experiments where it is crucial to be correct, reducing the chance of false positives but potentially increasing costs or complexity. Conversely, a higher threshold (e.g., 0.2) can be used for less critical decisions, accepting a higher rate of false positives.
What are some ways viewers can support StatQuest?
-Viewers can support StatQuest by subscribing to the channel, contributing to the Patreon campaign, becoming a channel member, purchasing original songs or merchandise, or making a direct donation through the links provided in the video description.
Outlines
π Understanding P-Values and Their Significance
In this paragraph, Josh Starmer introduces the concept of p-values and their role in statistical analysis. He uses the example of comparing two drugs, Drug A and Drug B, to illustrate how p-values help determine if there's a significant difference between the two. The discussion covers the limitations of small sample sizes, the impact of random occurrences, and the need for larger sample sizes to draw reliable conclusions. It concludes with an example that shows a significant difference in effectiveness between the two drugs, emphasizing that a low p-value indicates a high level of confidence that the observed effects are not due to random chance.
π P-Value Thresholds and Decision Making
This section delves into the practical use of p-values in decision making. A threshold of 0.05 is commonly used, which means that if there is no actual difference between the drugs, only 5% of experiments would incorrectly indicate a difference. The paragraph explains the concept of false positives and how adjusting the threshold can reduce the likelihood of such errors. It also touches on the idea of hypothesis testing, where a null hypothesis assumes no difference between the drugs, and a small p-value provides evidence to reject this null hypothesis. The summary also clarifies that a small p-value does not necessarily mean a large effect size, as the p-value is about the probability of the observed data under the null hypothesis, not the magnitude of the difference.
π Summary of P-Value Analysis
The final paragraph summarizes the discussion on p-values. It contrasts two experiments with different p-values and effect sizes to show that a smaller p-value does not automatically imply a larger difference between the drugs. The summary reinforces the idea that p-values are about the statistical significance of the results, not the size of the observed effect. The paragraph ends with a call to action for viewers to subscribe for more content, support the channel through Patreon, or purchase merchandise, providing links in the video description for further engagement.
Mindmap
Keywords
π‘P-value
π‘Null Hypothesis
π‘Drug A and Drug B
π‘Confidence Level
π‘False Positive
π‘Effect Size
π‘Random Chance
π‘Placebo Effect
π‘Sample Size
π‘Hypothesis Testing
π‘Statistical Significance
Highlights
P-values are discussed to determine if drug A is different from drug B.
A single person's result is insufficient to conclude the effectiveness of a drug due to possible random factors.
Increasing the number of test subjects improves the reliability of the drug comparison.
A large sample size of drug A showing a 99.7% cure rate suggests a significant difference from drug B.
A p-value close to zero indicates high confidence that drug A and drug B are different.
The commonly used threshold for p-value is 0.05, which means only 5% of experiments would result in a wrong decision if no difference exists.
A p-value of 0.9 indicates no significant difference between two groups, suggesting a high chance of random factors.
A false positive occurs when a small p-value is obtained despite no actual difference, which happens 5% of the time with a 0.05 threshold.
A smaller p-value threshold like 0.00001 reduces the chance of false positives but may not be necessary for less critical decisions.
The most common threshold of 0.05 is used because reducing false positives further often isn't worth the cost.
A p-value of 0.24 in the experiment suggests that drug A is not significantly different from drug B.
Hypothesis testing involves the null hypothesis that the drugs are the same, which the p-value helps to reject or accept.
A small p-value does not necessarily imply a large effect size or difference between the drugs.
The difference between drug A and drug B can be tiny or huge, regardless of the p-value.
A larger sample size can lead to a smaller p-value even with a smaller observed difference due to increased statistical power.
The video concludes by emphasizing the importance of understanding p-values in statistical analysis and their practical applications.
The presenter invites viewers to subscribe for more content and to support the channel through Patreon or other contributions.
Transcripts
Browse More Related Video

What Is A P-Value? - Clearly Explained
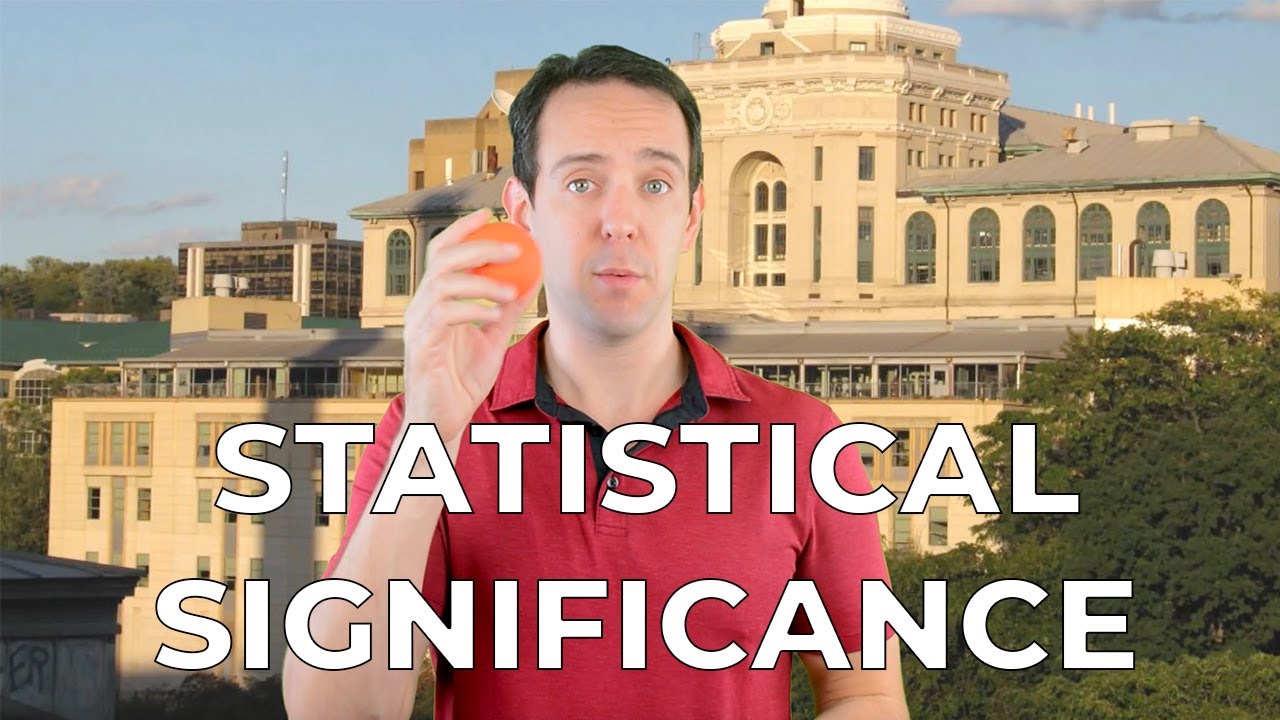
Statistical Significance and p-Values Explained Intuitively
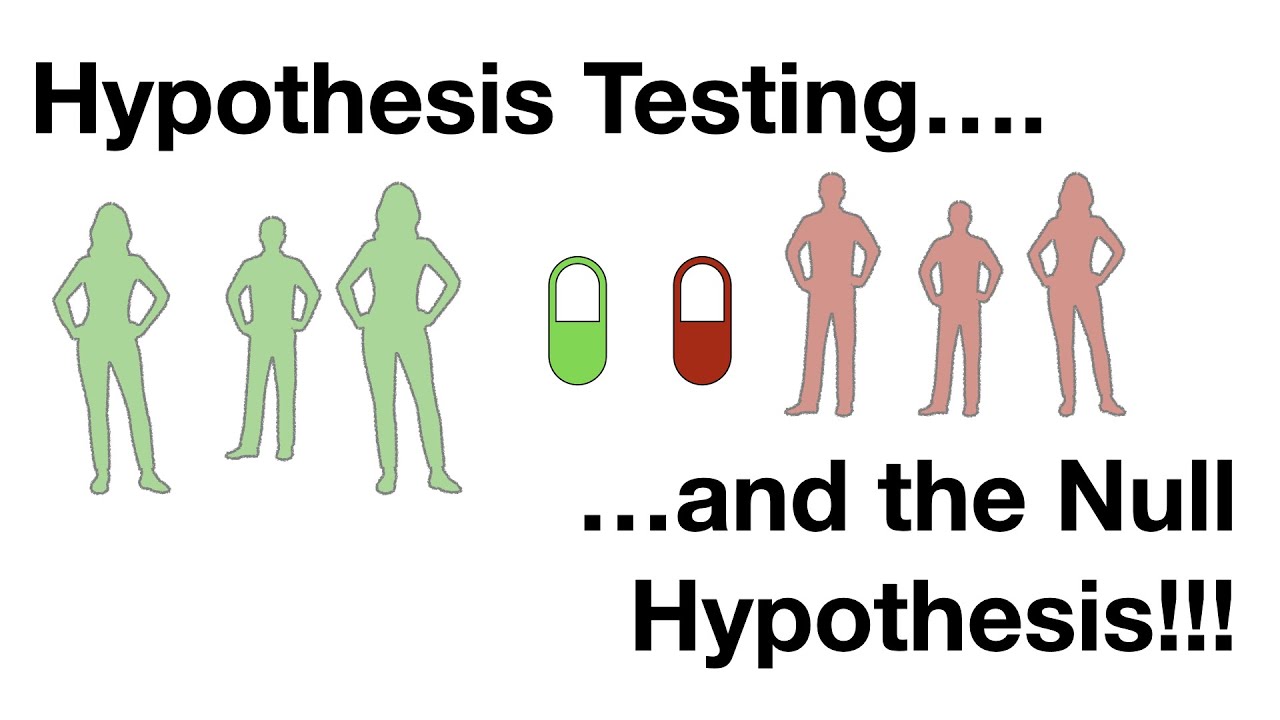
Hypothesis Testing and The Null Hypothesis, Clearly Explained!!!
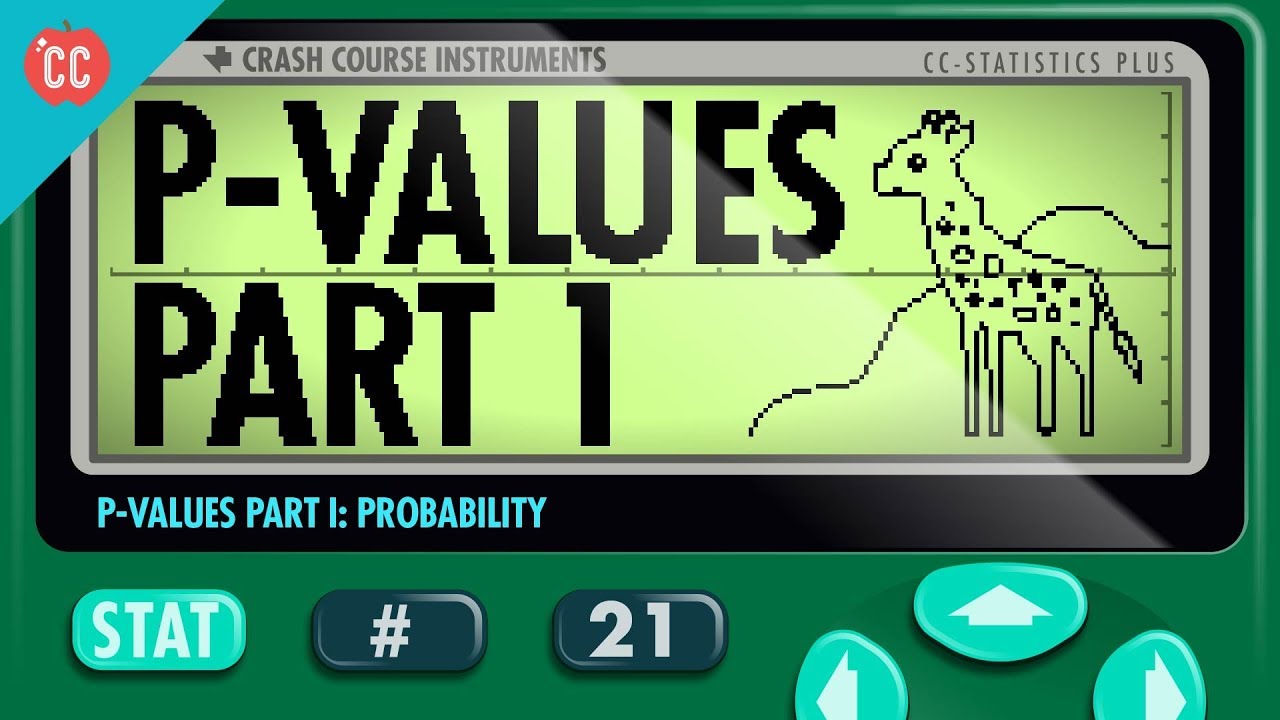
How P-Values Help Us Test Hypotheses: Crash Course Statistics #21
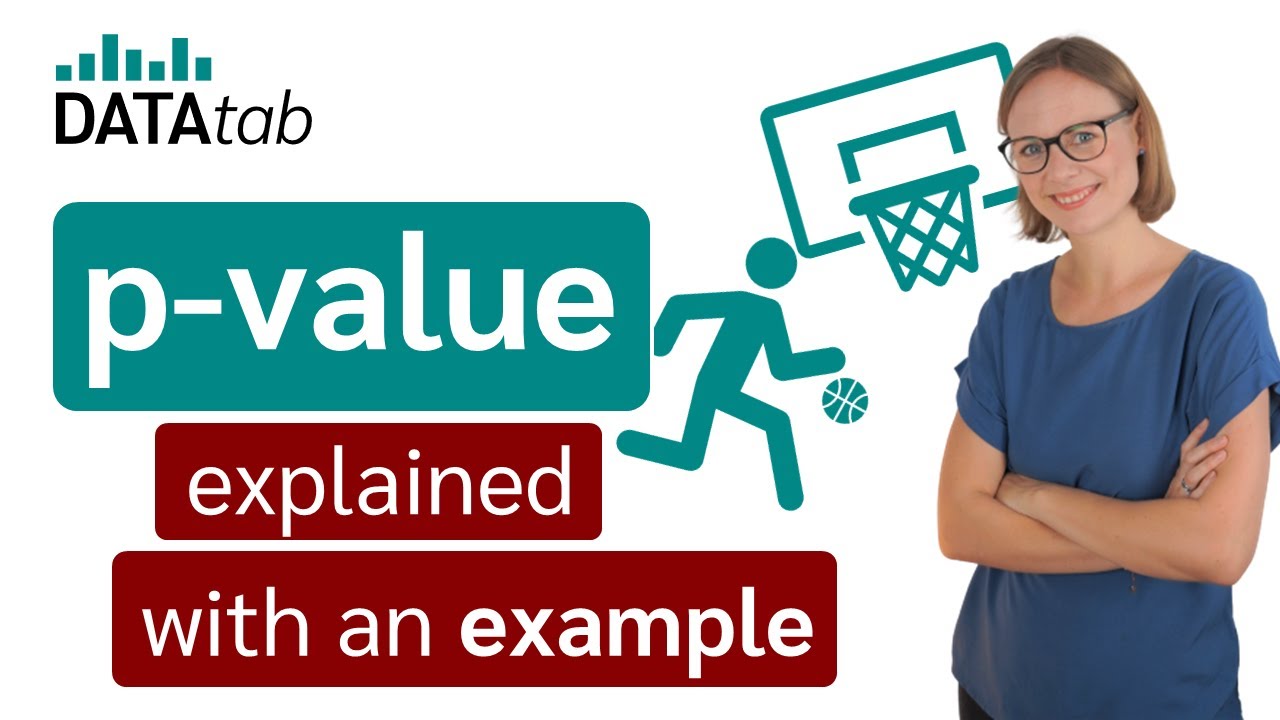
p-value - easily explained with an example
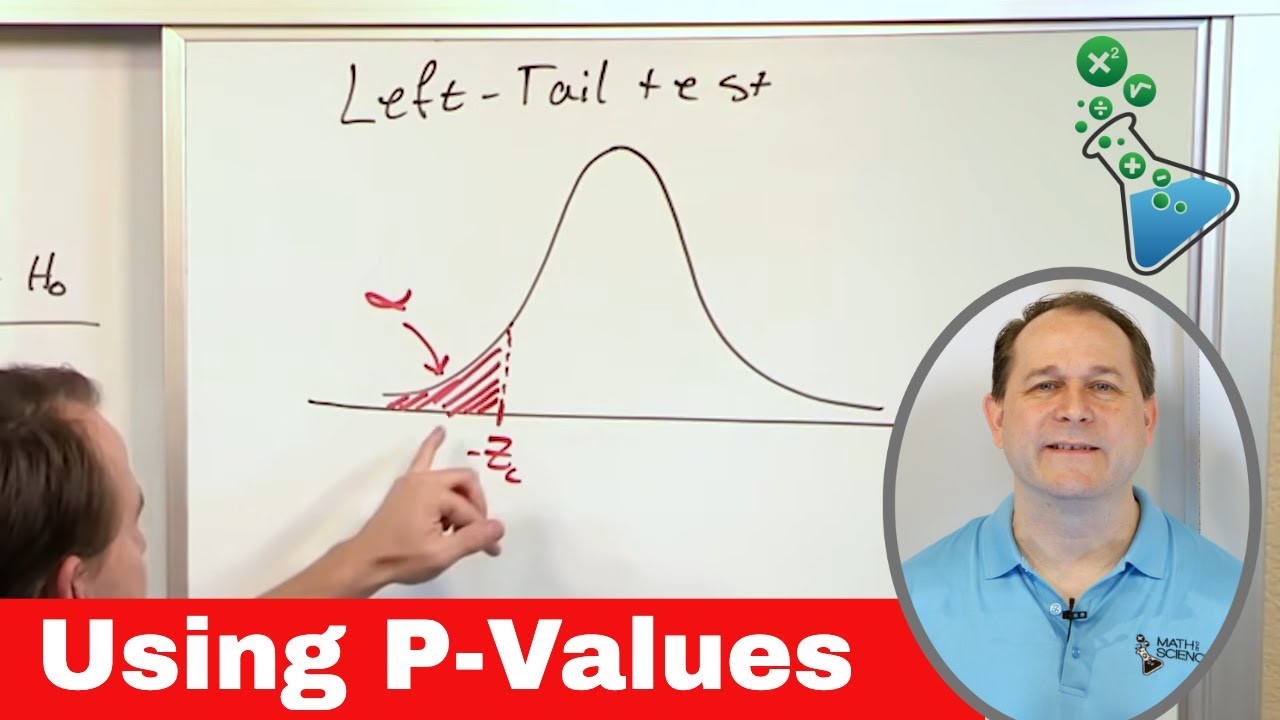
05 - Using P-Values in Hypothesis Testing (Compare P Value to Level of Significance)
5.0 / 5 (0 votes)
Thanks for rating: