Statistical Significance and p-Values Explained Intuitively
TLDRIn this video, Jeff Gallick from 'Date Demystified' explains the concept of statistical significance in scientific studies. He clarifies that while we cannot prove something to be true, we can provide evidence against a null hypothesis, which is the assumption of no effect or no difference. The video delves into the meaning of 'statistically significant results', typically indicated by a p-value less than 0.05. This p-value represents the probability of observing the data (or more extreme) if the null hypothesis were true. A p-value below 0.05 suggests that the observed results are unlikely if the null hypothesis holds, thus providing enough evidence to reject it. Gallick also discusses the arbitrary nature of the 0.05 threshold, which is a convention established by Ronald Fisher in the early 20th century. He suggests that while some argue for the abandonment of p-value significance testing in favor of confidence intervals and Bayesian methods, the convention aids in consistent scientific communication.
Takeaways
- π§ **Statistical Significance**: In science, we can't prove something to be true; instead, we provide evidence against a null hypothesis, which is a statement of no effect or no difference.
- π **P-Value**: A p-value is a statistic that measures the strength of the evidence against the null hypothesis. A smaller p-value indicates stronger evidence to reject the null hypothesis.
- π **Threshold of 0.05**: The convention of considering a p-value less than 0.05 as statistically significant was chosen arbitrarily and is not an absolute measure of truth.
- π **Repeatability**: Repeated experiments that show a consistent result increase our confidence in rejecting the null hypothesis, though we never achieve absolute certainty.
- π **Data-Rich World**: The video aims to equip viewers with the knowledge to thrive in a world where data and statistical analysis play a crucial role.
- π **Null Hypothesis**: In statistics, the null hypothesis is a default position that assumes no effect or no relationship between variables, which is what we test against.
- π **Evidence Against Null**: When we observe a result that strongly suggests the null hypothesis is false, we gather evidence to reject it, not prove it wrong.
- π **Example of Drug Efficacy**: The video uses the example of testing a drug's effectiveness against heart disease to illustrate the process of statistical significance testing.
- π **Confidence and P-Value**: A lower p-value (e.g., 0.01) means that if the null hypothesis were true, the observed result would be very unlikely, thus increasing confidence in rejecting the null hypothesis.
- π€ **Arbitrary Nature of 0.05**: The choice of 0.05 as a threshold for statistical significance is a historical convention without a scientific basis for being the ideal cutoff.
- βοΈ **Alternatives to P-Values**: Some argue for focusing on confidence intervals, Bayesian estimates, and effect sizes as alternatives to traditional p-value significance testing for a more nuanced understanding of evidence.
Q & A
What is the main idea behind statistical significance testing in science?
-The main idea behind statistical significance testing is that we can never prove something to be true; instead, we provide evidence against a null hypothesis, which is an assumption of no effect or no difference.
What does the term 'statistically significant result' imply?
-A 'statistically significant result' implies that there is enough evidence to reject the null hypothesis, suggesting that the observed effect or difference is unlikely to have occurred by chance alone.
What is the role of the null hypothesis in statistical testing?
-The null hypothesis serves as a default assumption of no effect or no relationship between variables. It is what we assume to be true until evidence from data analysis suggests otherwise.
Why can't we prove something to be true in science?
-We can't prove something to be true in science because it would require observing every possible condition across all of time, which is impossible. Instead, we can only provide evidence against a claim or assumption.
What is the significance of the p-value in determining statistical significance?
-The p-value indicates the probability of observing the data (or more extreme data) if the null hypothesis were true. A smaller p-value suggests stronger evidence against the null hypothesis, with a common threshold for significance being p < 0.05.
Why is the threshold for statistical significance often set at 0.05?
-The threshold of 0.05 is arbitrary and was chosen by convention, likely by Ronald Fisher in the early 20th century. It is used as a standard to determine if the evidence is strong enough to reject the null hypothesis.
What is the difference between proving a hypothesis and rejecting a null hypothesis?
-Proving a hypothesis would require demonstrating its truth in every possible scenario, which is not feasible. Rejecting a null hypothesis, on the other hand, involves showing that the observed data is unlikely under the assumption of the null hypothesis, providing evidence against it.
How does the concept of 'innocent until proven guilty' relate to the null hypothesis in statistics?
-The concept of 'innocent until proven guilty' is analogous to the null hypothesis in that it represents the default position that is assumed to be true unless sufficient evidence is presented to the contrary.
What are some criticisms of using statistical significance testing with a p-value threshold?
-Critics argue that the reliance on a p-value threshold can be misleading, as it does not account for the size of the effect, the power of the study, or the potential for false positives. Some suggest focusing on confidence intervals, Bayesian methods, or effect sizes instead.
What is the meaning of a p-value of 0.01 in the context of a study?
-A p-value of 0.01 means that if the null hypothesis were true, there is only a 1% chance of observing a result as extreme as the one obtained in the study, suggesting strong evidence against the null hypothesis.
Why might someone choose a different p-value threshold, such as 0.01 or 0.15?
-A researcher might choose a different p-value threshold to adjust the stringency of the evidence required to reject the null hypothesis. A lower threshold like 0.01 indicates a higher standard for evidence, while a higher threshold like 0.15 indicates a more lenient standard.
What are some alternatives to traditional statistical significance testing that are sometimes recommended?
-Some alternatives to traditional significance testing include confidence intervals, which provide a range of values within which the true effect is likely to fall; Bayesian estimates, which incorporate prior knowledge and update it with new data; and effect sizes, which measure the magnitude of the difference or strength of the relationship between variables.
Outlines
π Understanding Statistical Significance
This paragraph introduces the concept of statistical significance in scientific studies. The host, Jeff Gallick, explains that while we can't prove something to be true, we can provide evidence against a null hypothesis. He uses the example of a ball falling to the ground to illustrate how repeated observations can build evidence against a hypothesis. The paragraph also touches on the idea that statistical significance is about comparing results to a null hypothesis and the role of p-values in determining if results are statistically significant.
π The Role of P-Values in Statistical Significance
In this paragraph, Jeff discusses the role of p-values in statistical testing. He explains that a p-value represents the probability of observing a given result if the null hypothesis were true. A smaller p-value indicates stronger evidence against the null hypothesis. He uses the example of a drug trial to illustrate how different p-values can lead to different conclusions about the drug's effectiveness. Jeff also explains that a p-value of less than 0.05 is traditionally considered statistically significant, meaning there's less than a 5% chance of observing the results if the null hypothesis were true. He notes that the choice of 0.05 as a cutoff is arbitrary and that different disciplines might use different thresholds. Finally, he mentions that while statistical significance testing is a common approach, some argue for focusing on confidence intervals, Bayesian estimates, and effect sizes instead.
Mindmap
Keywords
π‘Statistical Significance
π‘P-Value
π‘Null Hypothesis
π‘Data-Rich World
π‘Evidence
π‘Confidence Intervals
π‘Bayesian Estimates
π‘Effect Size
π‘Randomized Experiment
π‘False Positive Rate
π‘Ronald Fisher
Highlights
Jeff Galick, the host of Date Demystified, aims to equip viewers with the information needed to thrive in a data-rich world.
The concept of 'statistical significance' is often mentioned in scientific studies and refers to a p-value less than 0.05.
Statistical significance testing is about providing evidence against a null hypothesis, not proving it true.
Science provides evidence against claims rather than absolute proof, due to the impossibility of observing every condition across time.
The null hypothesis is an assumption of innocence until proven guilty, which is what we assume to be true until evidence suggests otherwise.
Statistical significance is determined by comparing the result of an analysis to a null hypothesis, similar to a jury convicting a defendant.
The p-value represents the probability of observing the data (or more extreme) if the null hypothesis were true.
A p-value less than 0.05 indicates that there is strong enough evidence to reject the null hypothesis.
The threshold of 0.05 for statistical significance is arbitrary and was chosen by convention, likely by Ronald Fisher in the early 20th century.
Choosing a smaller p-value cutoff like 0.01 provides stronger evidence to reject the null hypothesis.
A higher p-value, such as 0.60, suggests that the observed results are likely even if the null hypothesis is true, indicating weak evidence.
The p-value of 0.01 means that if the null hypothesis were true, there's only a 1% chance of observing the data or more extreme results.
The video discusses the importance of shared scientific language for effective communication and knowledge growth.
Some argue that statistical significance testing should be abandoned in favor of confidence intervals, Bayesian estimates, and effect sizes.
The video simplifies complex statistical concepts to provide an intuitive understanding of significance testing.
The host encourages viewers to engage by liking, subscribing, and turning on notifications for new content.
The video ends with a call to action for viewers to comment if they are interested in learning more about specific statistical topics.
Transcripts
Browse More Related Video
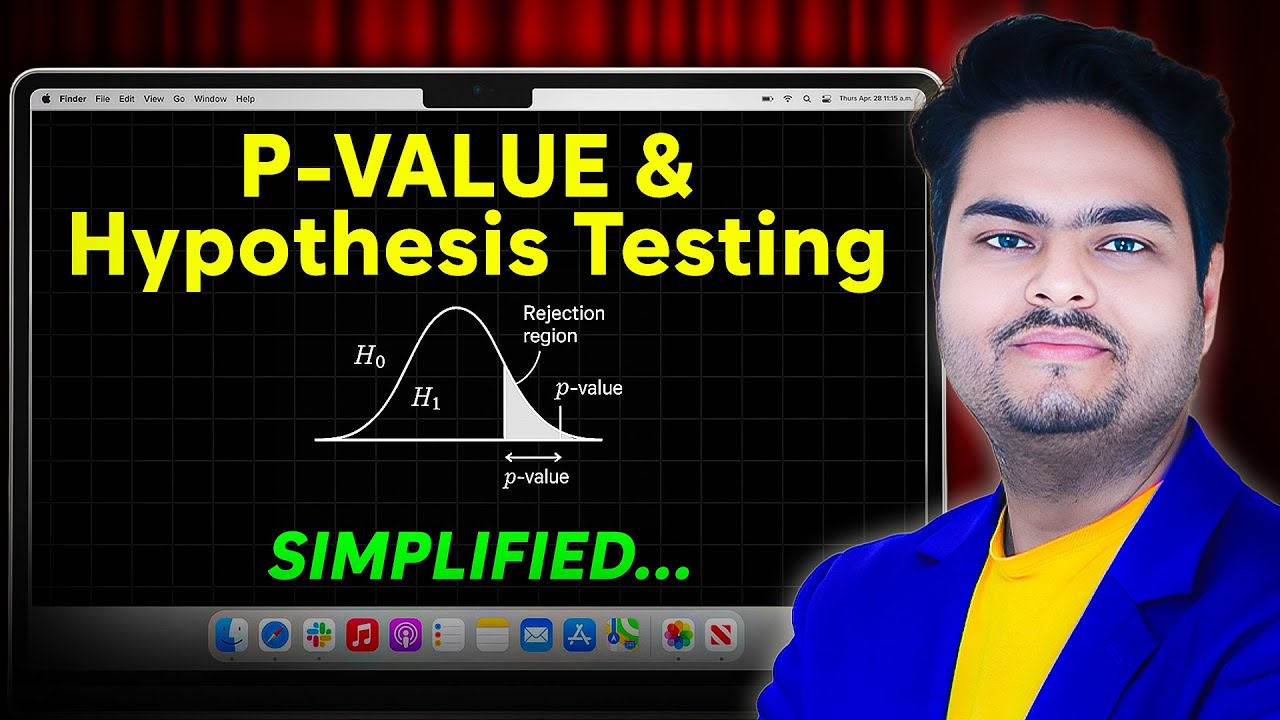
P Value and Hypothesis Testing Simplified|P-value and Hypothesis testing concepts in Statistics

p-values: What they are and how to interpret them
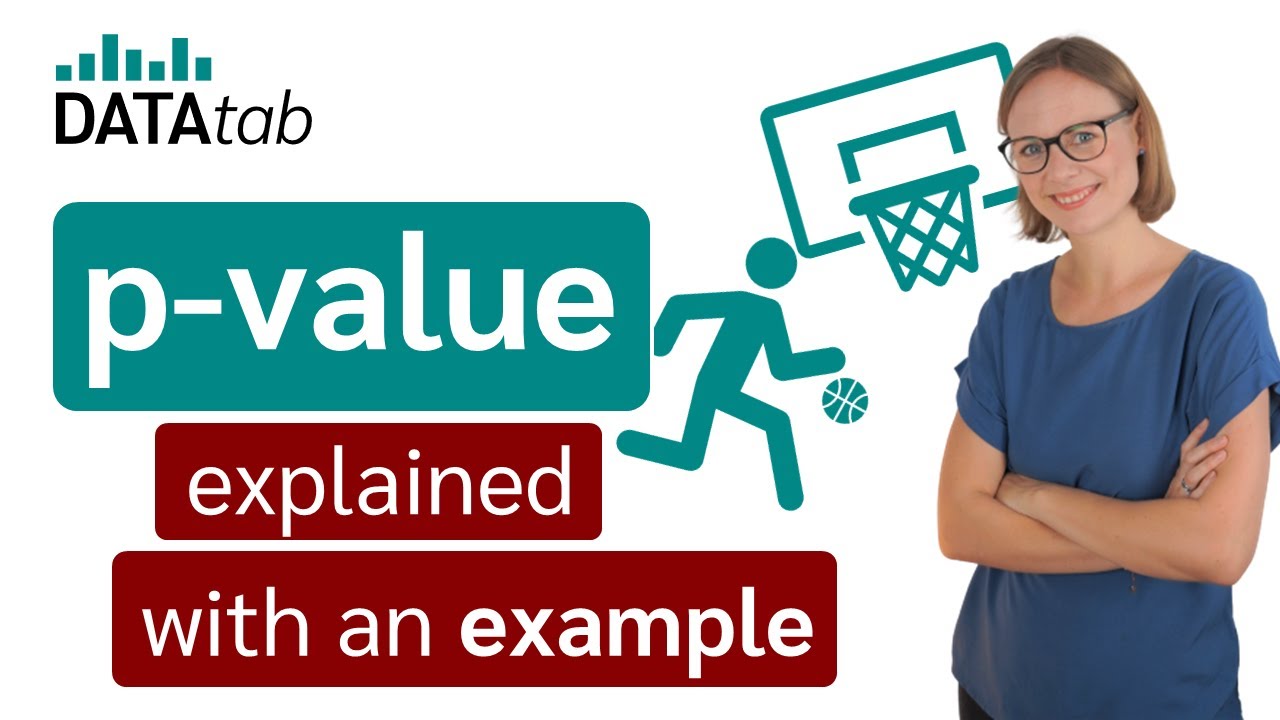
p-value - easily explained with an example
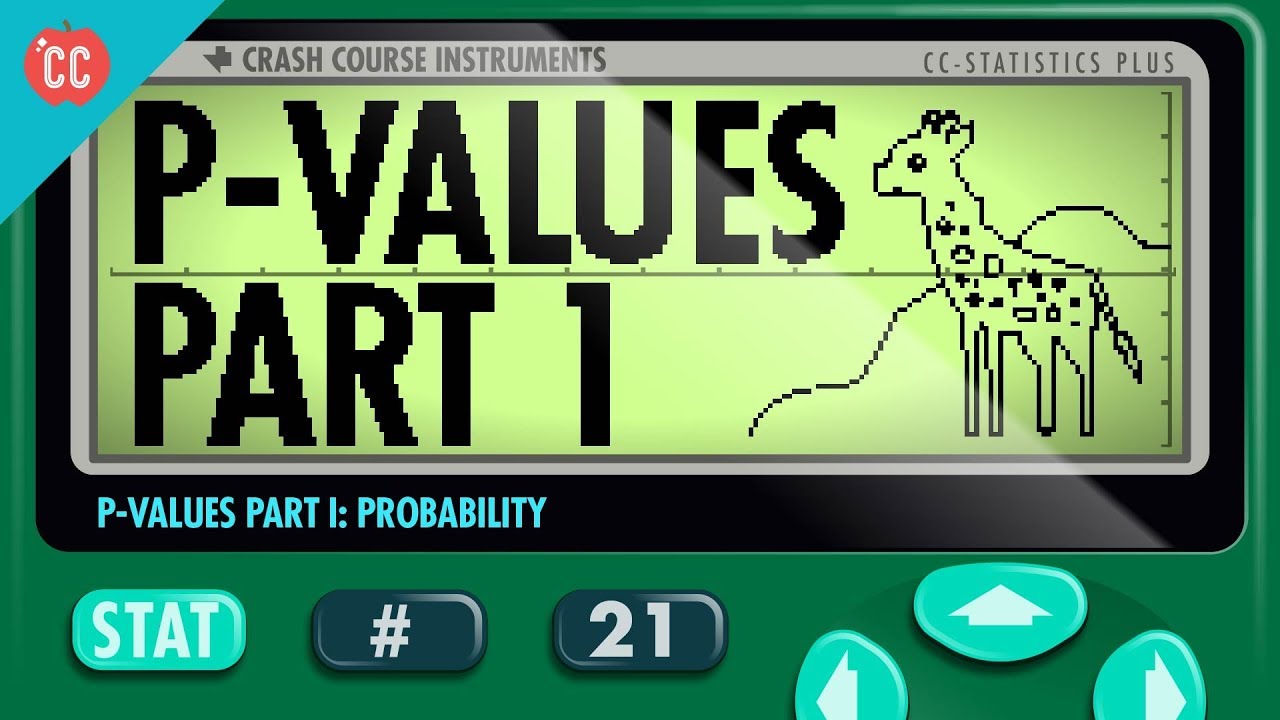
How P-Values Help Us Test Hypotheses: Crash Course Statistics #21
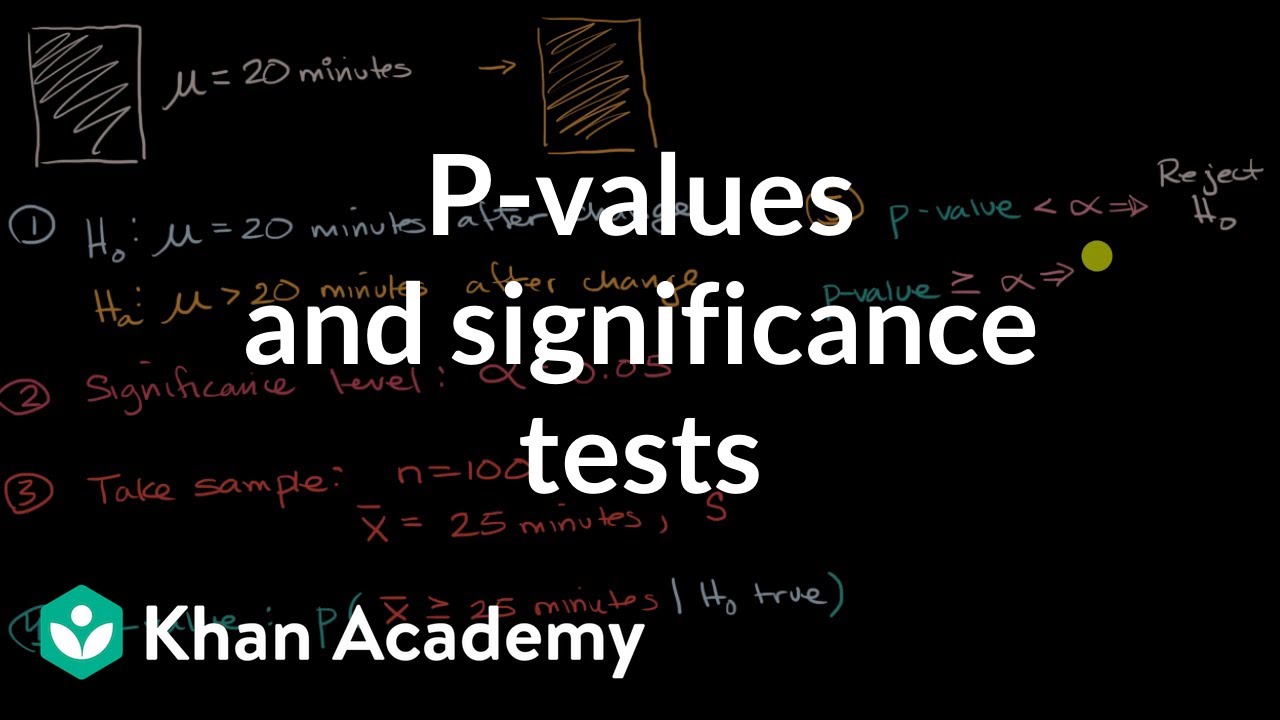
P-values and significance tests | AP Statistics | Khan Academy

What Is A P-Value? - Clearly Explained
5.0 / 5 (0 votes)
Thanks for rating: