Sampling: Simple Random, Convenience, systematic, cluster, stratified - Statistics Help
TLDRThe video script discusses the common practice of sampling to understand a population of interest. It introduces five sampling methods: simple random sampling, convenience sampling, systematic sampling, cluster sampling, and stratified sampling, highlighting their processes, advantages, and disadvantages. The importance of representative and unbiased samples is emphasized, acknowledging the inevitability of sampling error and the necessity to account for known bias in analysis and reporting.
Takeaways
- π **Sampling Process**: A sample is a subset of objects or observations taken from a larger population to infer information about the whole.
- π― **Objective of Sampling**: The goal is to obtain an unbiased and representative sample that accurately reflects the population's characteristics.
- βοΈ **Ideal Sampling Conditions**: Each member of the population should have an equal chance of being selected for the sample to ensure unbiased results.
- π **Sampling Example**: Measuring the size of apples in an orchard by taking a sample rather than measuring every single apple exemplifies the sampling process.
- π’ **Random Sampling Methods**: Simple random sampling uses random numbers to select sample members and is considered the theoretical ideal for unbiased sampling.
- π **Practicality of Sampling**: Simple random sampling is more practical for populations that are geographically concentrated and have a readily available sampling frame.
- πΆββοΈ **Convenience Sampling**: This method involves selecting samples based on what is easily accessible or convenient, which can often lead to biased results.
- π **Systematic Sampling**: A starting point is chosen randomly, and then every kth element is selected for the sample, making it an easier administration than simple random sampling.
- π **Cluster Sampling**: The population is divided into clusters, which are then randomly selected. This method is more convenient but can be biased if clusters differ significantly.
- π’ **Stratified Sampling**: This method involves dividing the population into strata based on specific characteristics and then taking a random sample from each stratum, aiming for a highly representative sample.
- π‘ **Sampling Method Selection**: Choose a sampling method that best fits the available resources and consider potential biases when analyzing and reporting the results.
Q & A
What is the definition of a sample in the context of a population of interest?
-A sample is a selection of objects and observations taken from the population of interest, which is used to infer information about the entire population.
Why is it impractical to measure every single object in a population?
-It is impractical due to constraints such as time, resources, and cost. Measuring or observing every single object is often not feasible, hence a representative sample is taken instead.
What is an unbiased sample and why is it important?
-An unbiased sample is one where each object in the population has an equal chance of being selected. It is important because it helps ensure that the sample accurately represents the population, thereby leading to reliable and valid conclusions.
What is the concept of sampling error?
-Sampling error, or variation due to sampling, refers to the difference between the characteristics of the sample and the actual characteristics of the entire population. It occurs because we are only examining a subset of the population.
What are the advantages and disadvantages of simple random sampling?
-Simple random sampling is theoretically the ideal method as it gives each object an equal chance of selection, potentially leading to an unbiased and representative sample. However, it can be difficult and expensive to implement, especially with human populations, and requires a good sampling frame.
How does convenience sampling work and what are its potential biases?
-Convenience sampling involves selecting participants or objects that are easily accessible or convenient. It is quick and cost-effective but often biased because it may not accurately represent the population due to non-random selection and potential self-selection bias.
Explain the process of systematic sampling and its possible limitations.
-Systematic sampling involves selecting a random starting point and then choosing every kth object in a list or sequence. While it's easier to administer than simple random sampling, it can lead to bias if there's a pattern in the population that corresponds to the sampling interval.
What is cluster sampling and how does it differ from stratified sampling?
-Cluster sampling divides the population into clusters, which are then selected at random, and all objects within each chosen cluster are included in the sample. It is more convenient and practical than simple random sampling. In contrast, stratified sampling involves dividing the population into strata based on specific characteristics and then sampling each stratum. Cluster sampling can be biased if the clusters are not representative of the population.
How does stratified sampling ensure a representative sample?
-Stratified sampling divides the population into homogeneous subgroups (strata) based on certain characteristics and then takes a random sample from each stratum, often proportional to the stratum's size in the population. This method can lead to a very representative sample by ensuring that the sample mirrors the diversity within the population.
What should be considered when choosing a sampling method?
-When choosing a sampling method, one should consider the nature of the population, the resources available in terms of time and money, and the desired level of accuracy. The method should be practical and yield the best possible results with the given constraints.
How should known bias in a sample be addressed in analysis and reporting?
-Known bias in a sample should be acknowledged and taken into account during analysis and reporting. This can involve adjusting the results or providing context to help interpret the findings accurately and understand their limitations.
Outlines
π Introduction to Sampling
This paragraph introduces the concept of sampling as a method to gather information about a population of interest. It explains that a sample consists of a subset of objects and observations selected from the larger population. The importance of selecting a sample that is representative and unbiased is emphasized, noting that there will always be some degree of sampling error since only a part of the population is observed. The paragraph also outlines that the method of sampling is influenced by the nature of the population and the resources available, with an unbiased sample being ideal but often challenging and costly to obtain, especially with human populations. It sets the stage for the discussion of five different sampling methods that will be presented in the video.
Mindmap
Keywords
π‘population of interest
π‘sample
π‘unbiased sample
π‘sampling error
π‘simple random sampling
π‘convenience sampling
π‘systematic sampling
π‘cluster sampling
π‘stratified sampling
π‘sampling frame
π‘resources
Highlights
The concept of sampling is introduced as a common practice to study a population of interest.
A sample is defined as a selection of objects and observations taken from the population of interest.
The importance of measuring the size of apples in an orchard is used as an example to illustrate the concept of sampling.
The method of sampling depends on the nature of the population and the resources available.
An unbiased sample is ideal, where each object in the population has an equal chance of being selected.
Representativeness of the sample is also crucial, as exemplified by the red and green apple split.
Sampling error is acknowledged as an inherent part of the process since only a part of the population is observed.
Simple random sampling is considered the theoretical ideal method of sampling, providing an unbiased sample.
Challenges and expenses associated with simple random sampling, especially with human populations, are discussed.
Convenience sampling is described as a quick and easy method, but it often leads to biased results.
Self-selection bias in convenience sampling is highlighted as a potential issue.
Systematic sampling is explained as a method involving a random starting point and regular intervals.
Cluster sampling is introduced as a method dividing the population into clusters and selecting them at random.
Stratified sampling is detailed as a method that involves dividing the population into strata representing different characteristics.
The complexity and resource requirements of stratified sampling are discussed.
The importance of choosing the best sampling method based on available resources is emphasized.
The need to account for known bias in the sample during analysis and reporting is stressed.
Transcripts
Browse More Related Video
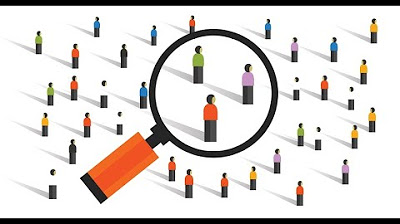
Probability and Non-Probability Sampling in Research Methods

Statistics Lecture 1.5: Sampling Techniques. How to Develop a Random Sample
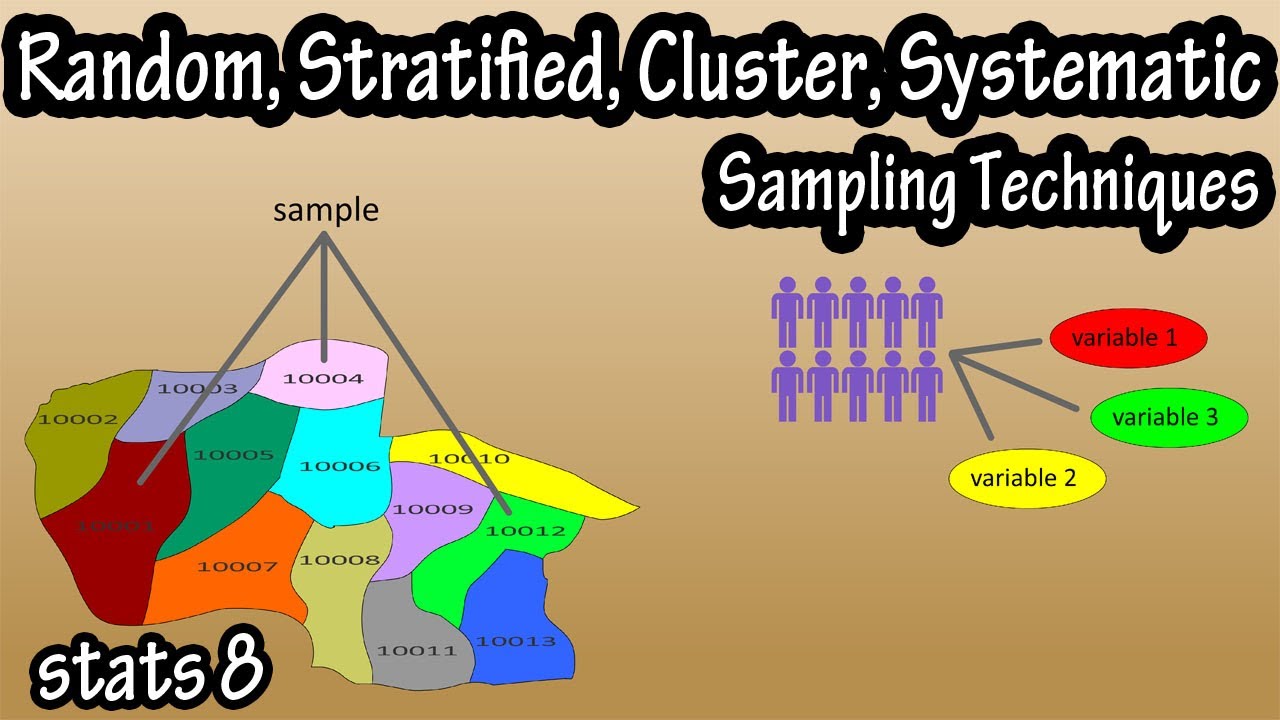
What Are The Types Of Sampling Techniques In Statistics - Random, Stratified, Cluster, Systematic
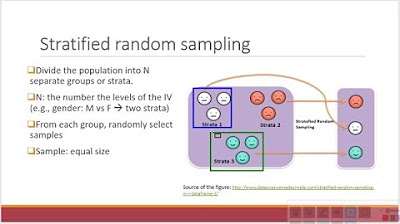
Research Methods 1: Sampling Techniques
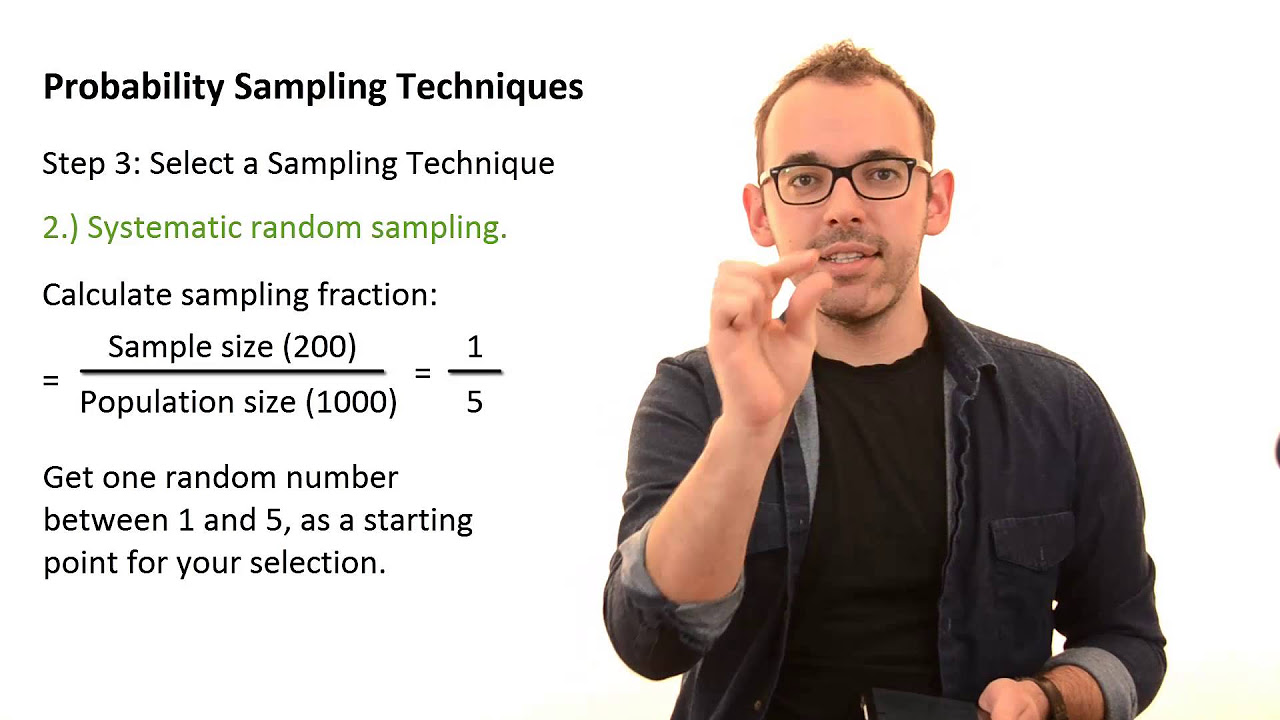
4.2 Probability Sampling Techniques

Tutorial 2- What is Population And Sample And Sampling Techniques In Hindi?
5.0 / 5 (0 votes)
Thanks for rating: