The Central Limit Theorem - understanding what it is and why it works
TLDRThe central limit theorem, a foundational concept in statistics, posits that the sampling distribution of the mean becomes more normal and less spread out as sample size increases. Dr. Nic illustrates this with a dragon strength example, showing how sample means cluster around the population mean and how larger samples produce a more precise estimate. The video emphasizes the theorem's applicability to real-world studies and the importance of adequate sample size for accurate statistical inferences.
Takeaways
- π The central limit theorem is a fundamental concept in statistics with wide applications in traditional tests and procedures.
- π’ It deals with the sampling distribution of the mean, which is the distribution of the means obtained from samples taken from a population.
- π― The mean of the sample (x-bar), the size of the sample (n), and the standard deviation of the sample (s) are key components used to make inferences about the population mean.
- π Aspect 1: The sampling distribution of the mean is less spread out than the values in the original population.
- π Aspect 2: The sampling distribution of the mean is well-modeled by a normal distribution, even with small sample sizes.
- π Aspect 3: The spread of the sampling distribution is related to the spread of the values in the population, with greater variability in the population leading to a greater spread in the sample means.
- π Aspect 4: Larger sample sizes lead to a smaller spread in the sampling distribution, reducing the likelihood of extreme values.
- π€ The central limit theorem is demonstrated through a hypothetical example of a dragon population, illustrating how sample means cluster around the population mean.
- π§ The theorem is illustrated with a computer simulation due to the impracticality of manually obtaining thousands of samples.
- β οΈ The central limit theorem applies under certain conditions and requires sufficiently large sample sizes; samples of 30 are usually sufficient for most cases.
Q & A
What is the central limit theorem concerned with?
-The central limit theorem is concerned with the sampling distribution of the mean, which is the distribution of means of samples taken from a population.
How does the central limit theorem relate to statistical tests and procedures?
-The central limit theorem is fundamental to many traditional statistical tests and procedures because it provides a basis for inferring population parameters from sample data, especially when sample sizes are large.
What are the four aspects of the central limit theorem mentioned in the script?
-The four aspects are: 1) The sampling distribution of the mean will be less spread than the values in the population. 2) The sampling distribution will be well modeled by a normal distribution. 3) The spread of the sampling distribution is related to the spread of the population values. 4) Bigger samples lead to a smaller spread in the sampling distribution.
Why is the mean of a sample (x-bar), the sample size (n), and the standard deviation of the sample (s) important?
-These values are important because they are used to estimate the population mean and to calculate confidence intervals, which help in making inferences about the population from the sample data.
How does the likelihood of getting an exact mean strength of one or eight for a sample of four dragons illustrate the central limit theorem?
-The very low probability of getting an exact mean strength of one or eight for a sample of four dragons illustrates that the mean of a sample is unlikely to be at the extreme ends of the population distribution, supporting the central limit theorem's aspect that the sampling distribution will be less spread than the population values.
What does it mean when the sampling distribution is well-modeled by a normal distribution?
-When the sampling distribution is well-modeled by a normal distribution, it means that the distribution of sample means will have a bell shape, with most of the sample means clustered around the true population mean and fewer sample means at the extremes.
How does the spread of the population values affect the spread of the sampling distribution?
-The spread of the population values directly affects the spread of the sampling distribution. If the population values have a larger range, the spread of the sample means will also be greater, as seen in the example with the dragon population strengths ranging from 1 to 20.
What is the relationship between the sample size and the spread of the sampling distribution?
-The spread of the sampling distribution is inversely related to the square root of the sample size. As the sample size increases, the spread of the sampling distribution decreases, leading to a more focused distribution around the population mean.
What are the conditions under which the central limit theorem applies?
-The central limit theorem applies when the sample sizes are large enough, typically samples of 30 or more are sufficient under most conditions. It also assumes that the population has a finite level of variance.
How does the computer simulation of 4,000 samples of four dragons help in understanding the central limit theorem?
-The computer simulation helps to illustrate the central limit theorem by showing the distribution of thousands of sample means, which would be impractical to calculate by hand. It visually demonstrates how the sampling distribution becomes less spread and more normally distributed as the number of samples increases.
What is the significance of the normal distribution in the context of the central limit theorem?
-The significance of the normal distribution is that it provides a mathematical model for the shape of the sampling distribution. As the sample size increases, the sampling distribution of the mean becomes more closely approximated by a normal distribution, regardless of the shape of the original population distribution.
Outlines
π Introduction to the Central Limit Theorem
This paragraph introduces the central limit theorem and its significance in statistics. Dr Nic explains the theorem's role in traditional statistical tests and procedures, and outlines four key aspects that will be discussed in the video. The central limit theorem is about the sampling distribution of the mean, which is the distribution of the means from samples taken from a population. The video aims to illustrate how the mean of a sample can be used to infer the mean of the population, and how the central limit theorem applies to this process. The aspects covered include the sampling distribution being less spread than the population, the normal distribution modeling of the sampling distribution, the relationship between the spread of the sampling distribution and the population values, and how bigger samples result in a smaller spread in the sampling distribution. An example involving a population of dragons and their strengths is used to illustrate these concepts.
π Implications of Sample Size on Distribution Spread
This paragraph delves into the impact of sample size on the spread of the sampling distribution. It explains that the spread of the sample means is expected to be greater if the population has a wider range of values, as illustrated by comparing two different dragon populations with varying strength ranges. The paragraph also discusses how the shape of the sampling distribution becomes more like a normal distribution as the sample size increases. This is evidenced by graphs showing the spread of sample means from different sample sizes. The central limit theorem's applicability is noted to depend on certain conditions and sufficiently large sample sizes, with a general recommendation that samples of 30 are usually sufficient. The video concludes with a reminder that the central limit theorem only applies to one sample from a population, and that the thousands of samples used in the video are for illustrative purposes only.
Mindmap
Keywords
π‘Central Limit Theorem
π‘Sampling Distribution
π‘Mean
π‘Standard Deviation
π‘Inference
π‘Population
π‘Sample
π‘Confidence Interval
π‘Normal Distribution
π‘Spread
π‘Sample Size
Highlights
The central limit theorem is a fundamental concept in statistics with wide applications in traditional tests and procedures.
The theorem deals with the sampling distribution of the mean, which is the distribution of means from samples taken from a population.
The population mean is usually unknown, and we rely on sample data to make inferences about it.
The central limit theorem states that the sampling distribution of the mean will be less spread out than the population values.
The sampling distribution of the mean is well modeled by a normal distribution, regardless of the shape of the population distribution.
The spread of the sampling distribution is related to the spread of the population values, with greater spread in the population leading to greater spread in the sample means.
Larger sample sizes result in a smaller spread in the sampling distribution, reducing the likelihood of extreme values.
The central limit theorem is illustrated with an example involving a population of dragons and their strengths.
The example demonstrates that the mean strength of small samples can vary widely, but the central limit theorem suggests a normal distribution with larger samples.
The video also discusses the conditions under which the central limit theorem applies and notes that sample sizes of 30 are generally sufficient.
The central limit theorem is a powerful tool for making statistical inferences, especially when the population distribution is unknown or difficult to determine.
Understanding the central limit theorem is crucial for researchers and analysts who rely on sample data to draw conclusions about populations.
The video provides a clear and accessible explanation of the central limit theorem, suitable for learners at various levels of statistical knowledge.
The use of a fictional population of dragons in the example helps to clarify the abstract concepts of the central limit theorem in a more engaging and memorable way.
The video emphasizes the importance of sample size in achieving a sampling distribution that closely resembles a normal distribution.
The central limit theorem has practical implications for designing experiments and studies, as it informs decisions about sample sizes and the reliability of results.
Transcripts
Browse More Related Video
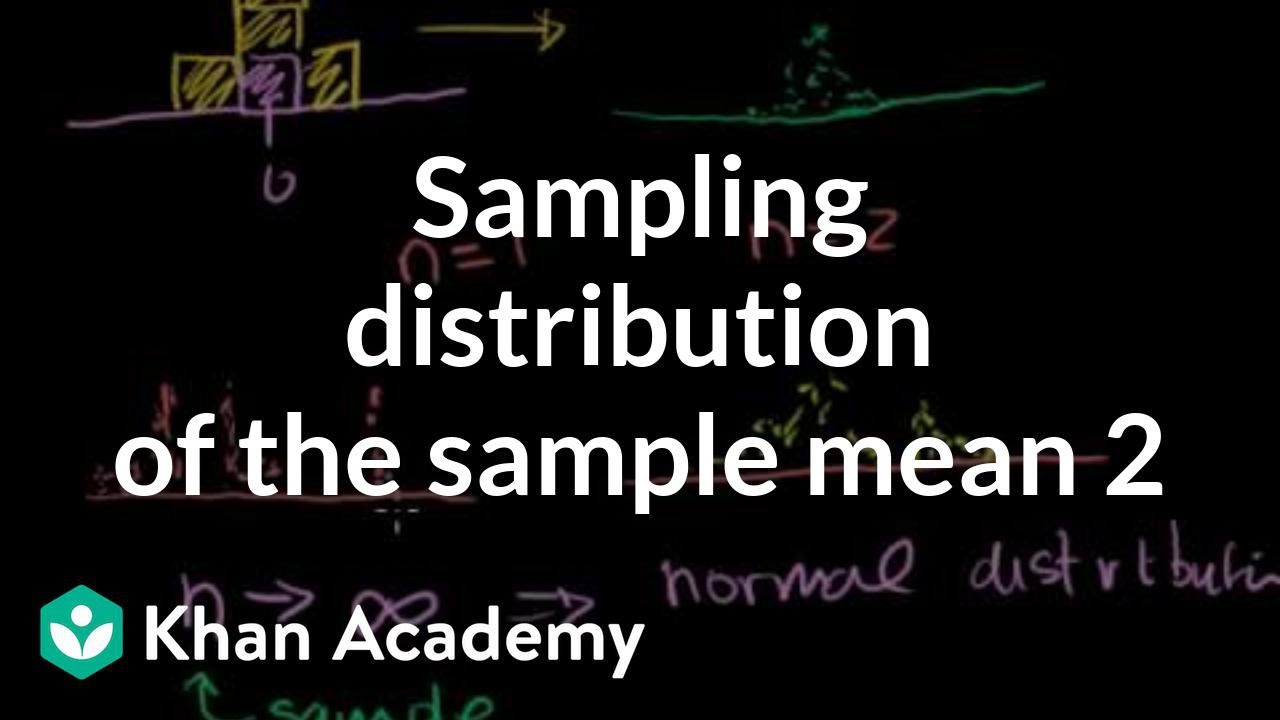
Sampling distribution of the sample mean 2 | Probability and Statistics | Khan Academy
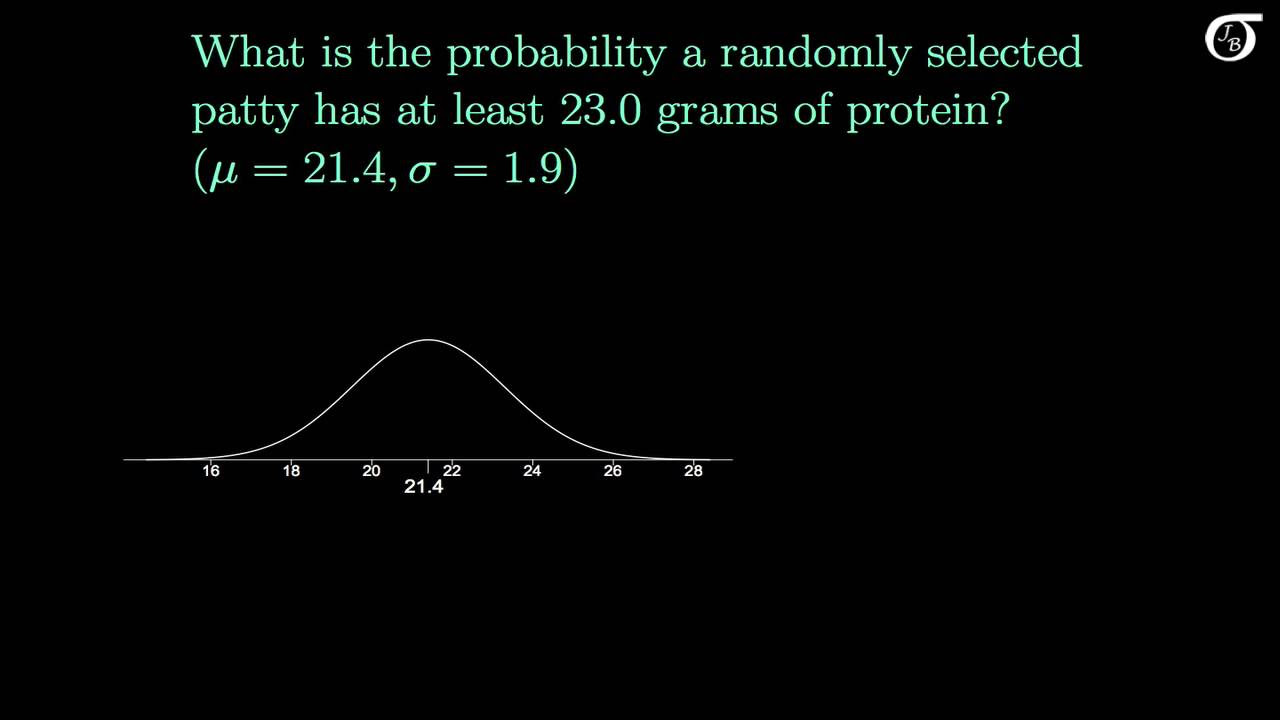
The Sampling Distribution of the Sample Mean
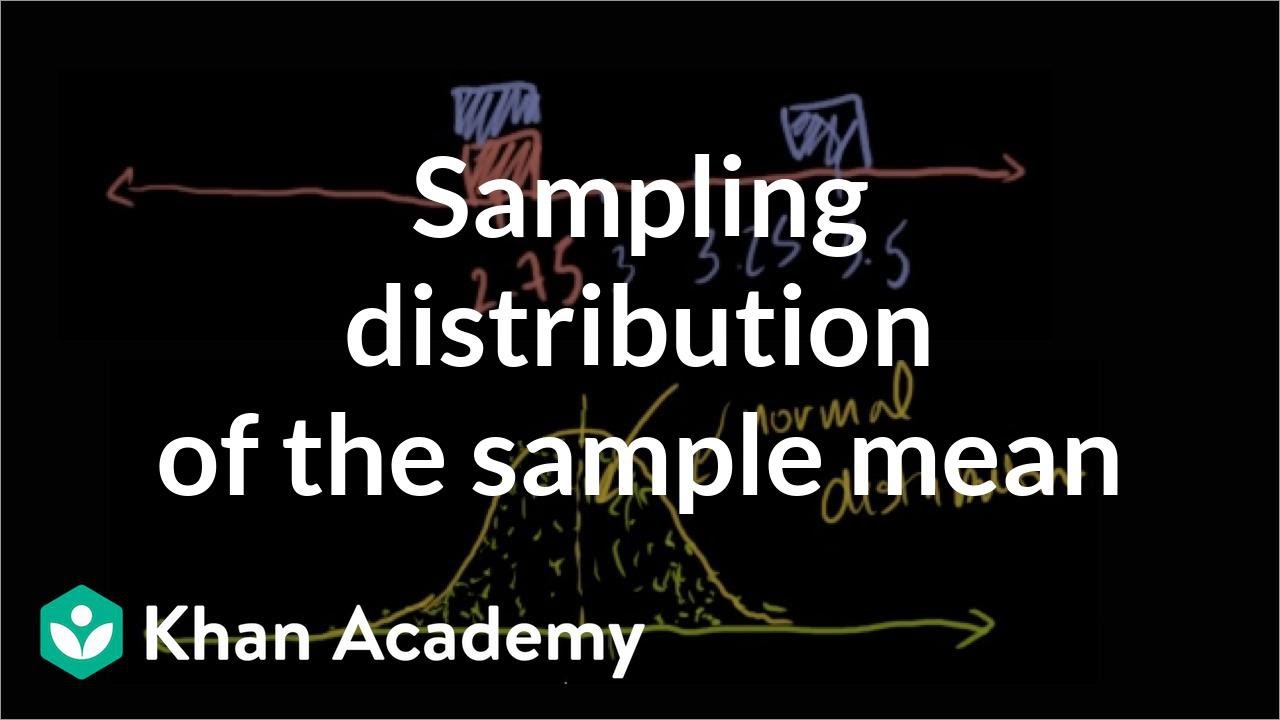
Sampling distribution of the sample mean | Probability and Statistics | Khan Academy

Elementary Stats Lesson #13
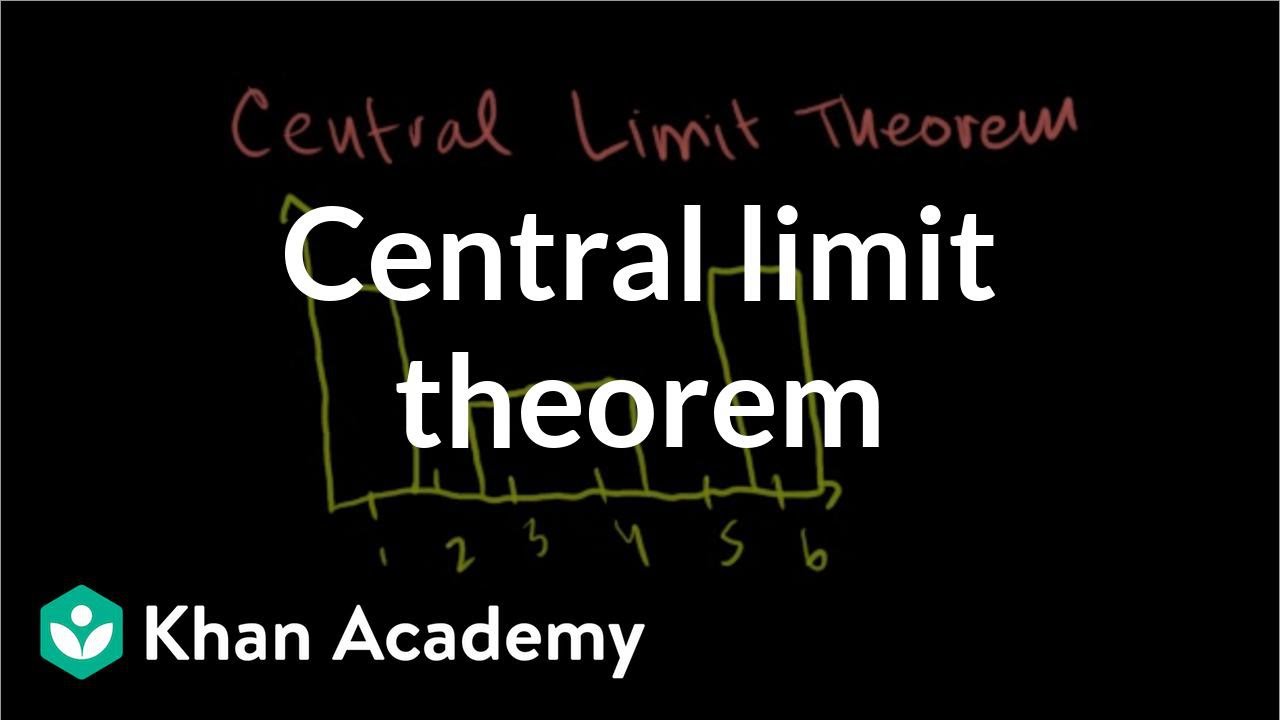
Central limit theorem | Inferential statistics | Probability and Statistics | Khan Academy
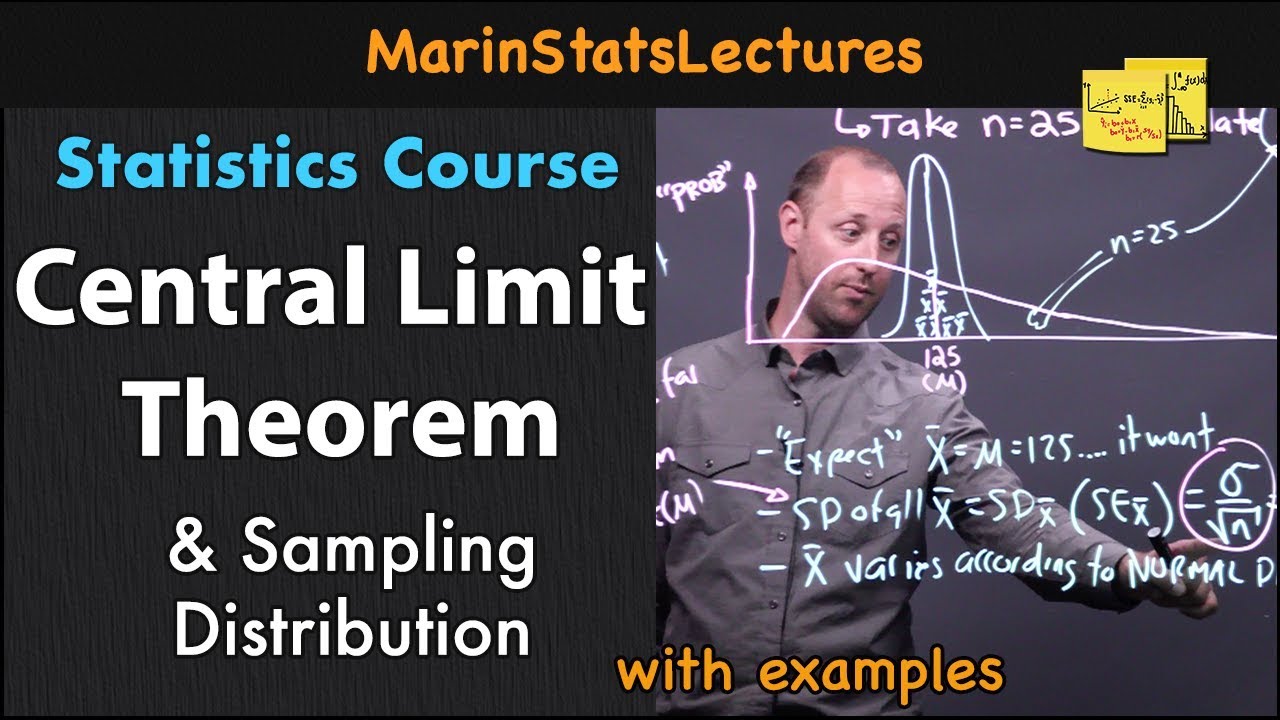
Central Limit Theorem & Sampling Distribution Concepts | Statistics Tutorial | MarinStatsLectures
5.0 / 5 (0 votes)
Thanks for rating: