Design of Experiments (DoE) simply explained
TLDRThis video script delves into the concept of Design of Experiments (DOE), outlining its systematic approach to experiment planning, execution, and analysis. It highlights the goal of identifying the impact of various factors on a response variable and discusses the process steps including planning, screening, optimization, and verification. The script also covers different types of DOE, such as full factorial and fractional factorial designs, and introduces online DOE creation with Data Tab, emphasizing the efficiency and cost-effectiveness of this method in reducing the number of necessary experiments.
Takeaways
- π¬ Design of Experiments (DOE) is a systematic approach to planning, conducting, and analyzing experiments.
- π― The aim of DOE is to analyze the relationship between multiple input variables (factors) and an output variable (response).
- π Factors are variables believed to affect the response variable, and each factor has at least two levels (specific values).
- π΄ An example given is investigating the frictional torque of a bike bearing, where frictional torque is the response variable, and lubrication (oil, grease) and temperature (low, medium, high) are the factors.
- π The process of a DOE project includes planning, screening, optimization, and verification.
- π Screening experiments are conducted to reduce the number of factors, which is crucial when there are many potential influencing factors.
- π Full factorial design involves testing all possible combinations of factor levels, but the number of experiments increases exponentially with the number of factors.
- βοΈ Fractional factorial design reduces the number of experiments by confounding some interactions with main effects or other interactions, sacrificing some resolution.
- βοΈ Main effects refer to the impact of a single factor on the response variable, while interaction effects occur when the effect of one factor depends on the level of another factor.
- π» DOE can be efficiently designed and analyzed using online tools like Data Tab, which supports various designs including full factorial, fractional factorial, Blacket Burman, Box Banking, and central composite designs.
Q & A
What is the Design of Experiments (DOE)?
-Design of Experiments (DOE) is a systematic approach to planning, conducting, and analyzing experiments with the aim to analyze the relationship between several input variables (factors) and an output variable (response variable).
What are the main objectives of using DOE?
-The main objectives of using DOE are to identify relevant factors that significantly influence the response variable and to find the optimal input variables that maximize or minimize the response variable.
What is the difference between a factor and a response variable in the context of DOE?
-In DOE, factors are the variables believed to affect the response, while the response variable is the output that is measured and analyzed to determine the effect of the factors.
What is the purpose of the screening step in a DOE project?
-The screening step is used to reduce the number of factors that could potentially influence the response variable, thus simplifying the experimental process and reducing the number of experiments required.
How does the number of factors affect the number of experiments needed in a full factorial design?
-In a full factorial design, the number of experiments or runs is calculated as 2 to the power of the number of factors (K). As the number of factors increases, the number of required experiments increases exponentially.
What is the significance of the verification step in a DOE project?
-The verification step is crucial as it involves checking whether the calculated optimal input variables indeed have the desired influence on the response variable, ensuring the reliability of the experimental results.
What is a fractional factorial design and when is it used?
-A fractional factorial design is used for screening experiments when there are more than approximately four to six factors. It reduces the number of runs by confounding some main effects and interaction effects, thus providing a more efficient way to test multiple factors.
What is the concept of resolution in fractional factorial designs?
-Resolution in fractional factorial designs is a measure of how well a DOE can distinguish between different effects. It indicates the extent to which main effects and interaction effects are confounded in a design.
What are the main differences between full factorial and fractional factorial designs?
-Full factorial designs test all possible combinations of factor levels, ensuring all main and interaction effects are considered but requiring more runs. Fractional factorial designs, on the other hand, reduce the number of runs by confounding some effects, sacrificing some resolution for efficiency.
How can one estimate the number of experiments needed using DOE?
-The number of experiments needed can be estimated using a formula that takes into account the standard deviation (Sigma) and the desired difference (Delta) to be detected. The larger the variability or the smaller the effect size, the more experiments are needed.
Can you provide an example of how to create a DOE using an online tool like DataTab?
-To create a DOE online with DataTab, one would go to the website, click on 'plus' and then 'DOE', select the design type, specify the number of factors, and enter the factor names and levels. The tool then generates the experimental plan, which can be exported to Excel for further use.
Outlines
π Introduction to Design of Experiments (DOE)
The first paragraph introduces the concept of Design of Experiments (DOE), a systematic method for planning, conducting, and analyzing experiments to understand the relationship between input variables (factors) and an output variable (response). It explains the aim of DOE, which is to determine the influence of factors on the response variable and to identify significant factors that affect this relationship. The paragraph also uses the example of a bike's frictional torque influenced by factors like lubrication and temperature, emphasizing the importance of DOE in creating efficient test plans, especially when dealing with multiple variables.
π DOE Process Steps and Types of Designs
This paragraph delves into the process of a DOE project, outlining the steps of planning, screening, optimization, and verification. It highlights the importance of identifying relevant factors and response variables during the planning phase. The screening phase is crucial for reducing the number of factors, which in turn reduces the number of experiments required. The paragraph also introduces different types of DOE, such as full factorial, fractional factorial, and Black-Burman designs, and mentions the online platform data.net for creating these designs.
π§ Efficiency of DOE in Reducing Experiments
The third paragraph discusses the efficiency of DOE in minimizing the number of experiments needed by providing a detailed example of how to estimate the number of runs required based on standard deviation and the desired level of accuracy (Delta). It contrasts the need for a large number of runs with the risk of missing relevant differences if the number of runs is too small. The paragraph also introduces the concept of full factorial design and its benefits over testing one factor at a time, such as gaining more information with fewer experiments.
π Understanding Main Effects and Interaction Effects
This paragraph explains the concepts of main effects and interaction effects in the context of DOE. Main effects refer to the influence of a single factor on the response variable, while interaction effects occur when the effect of one factor depends on the level of another factor. The paragraph uses the example of bearing lubrication and temperature to illustrate these effects and discusses the importance of distinguishing between them. It also introduces the concept of confounding in fractional factorial designs, where main effects and interaction effects cannot be separated.
π¬ Fractional Factorial Design and Resolution
The fifth paragraph explores fractional factorial designs, which are used for screening when there are many factors. It explains how these designs reduce the number of runs at the expense of resolution, which is a measure of the ability to distinguish between different effects. The paragraph discusses the implications of confounding effects at different resolutions and how it affects the analysis of results, using a table to illustrate the trade-off between the number of runs and resolution levels.
π Advanced DOE Techniques and Online Implementation
The final paragraph briefly touches on advanced DOE techniques such as Box-Behnken design and Central Composite design, which are used for detailed analysis and optimization of a few factors, including nonlinear dependencies. It also provides a step-by-step guide on how to create a DOE online using data.net, from selecting the design type and factors to exporting and evaluating the results, concluding the video with an invitation to learn more in the next video.
Mindmap
Keywords
π‘Design of Experiments (DOE)
π‘Factor
π‘Response Variable
π‘Screening
π‘Optimization
π‘Verification
π‘Full Factorial Design
π‘Fractional Factorial Design
π‘Interaction Effects
π‘Resolution
π‘Central Composite Design
π‘Box-Behnken Design
Highlights
Design of Experiments (DOE) is a systematic approach to planning, conducting, and analyzing experiments to understand the relationship between input variables (factors) and an output variable (response).
DOE aims to identify relevant factors that significantly influence the response variable and to find optimal input variables that maximize or minimize the response.
Factors in a system have at least two levels, which are the specific values a factor can take, to determine their influence on the response.
An example of applying DOE is investigating the frictional torque of a bike bearing, with lubrication and temperature as potential factors.
The process of a DOE project includes planning, screening, optimization, and verification stages.
Screening experiments are crucial when dealing with more than four to six factors to reduce the number of experiments needed.
The number of experiments required grows exponentially with the number of factors in a full factorial design, calculated as n = 2^K.
Fractional factorial designs and Plackett-Burman designs are used for screening experiments to reduce the number of runs while maintaining essential information.
After identifying significant factors, regression models are created to optimize the response variable based on input variables.
Verification ensures that the optimal input variables calculated indeed have the desired effect on the response variable.
Different types of designs like full factorial, fractional factorial, Plackett-Burman, Box-Behnken, and central composite designs serve various experimental needs.
Experiments cost time and money, hence the importance of reducing the number of runs while maintaining the integrity of the results.
The variability of the mean value can be reduced by increasing the sample size, leading to a more precise estimation of the mean.
A formula is provided to estimate the number of runs needed based on standard deviation and the desired difference (Delta).
Full factorial designs test all potential combinations of factors, providing more information about interactions between factors.
Fractional factorial designs confound interactions with other interactions or main effects to reduce the number of runs.
Resolution in DOE indicates the ability to distinguish between main effects and interaction effects, with higher resolution designs providing more detailed insights.
Black-Burman designs are suitable when many factors are involved and only main effects are of interest, distributing two-factor interactions over several factors.
Central composite and Box-Behnken designs are used for detailed analysis and optimization of a few factors, identifying nonlinear dependencies with fewer runs.
Data tab is an online platform that allows users to create and evaluate DOE designs, streamlining the experimental process.
Transcripts
Browse More Related Video
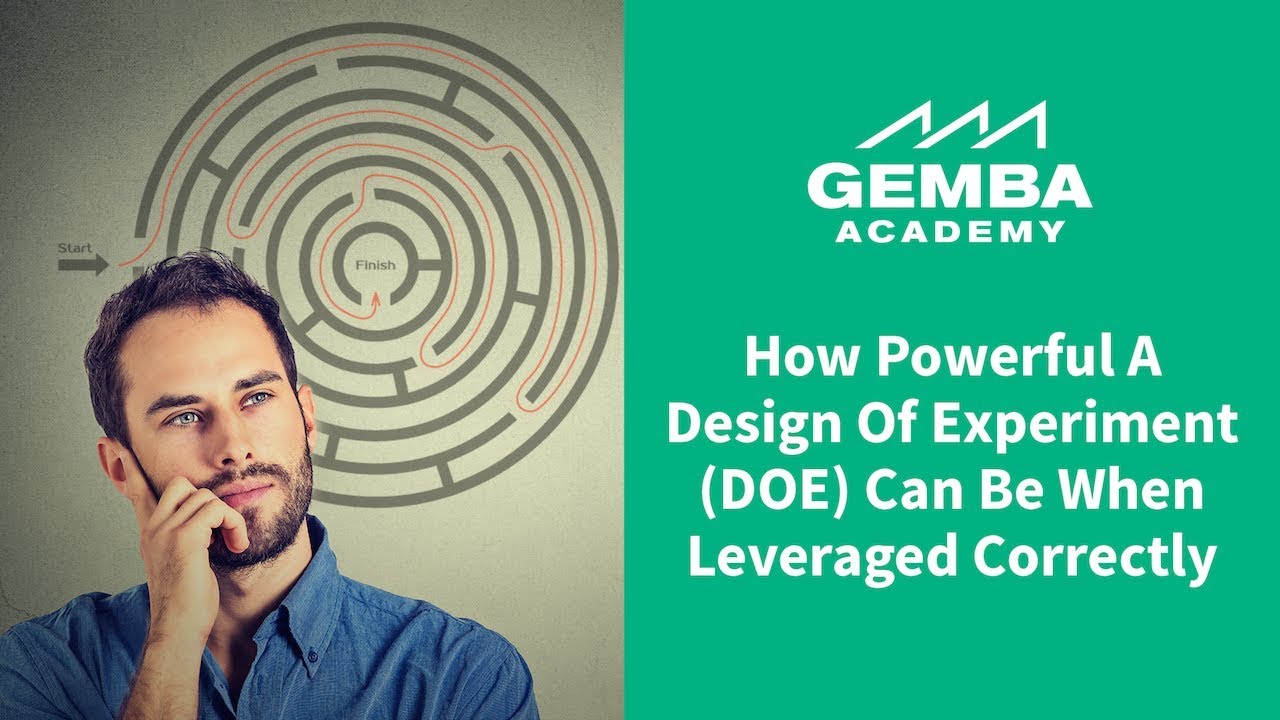
Learn How Powerful a Design of Experiment (DOE) Can Be When Leveraged Correctly
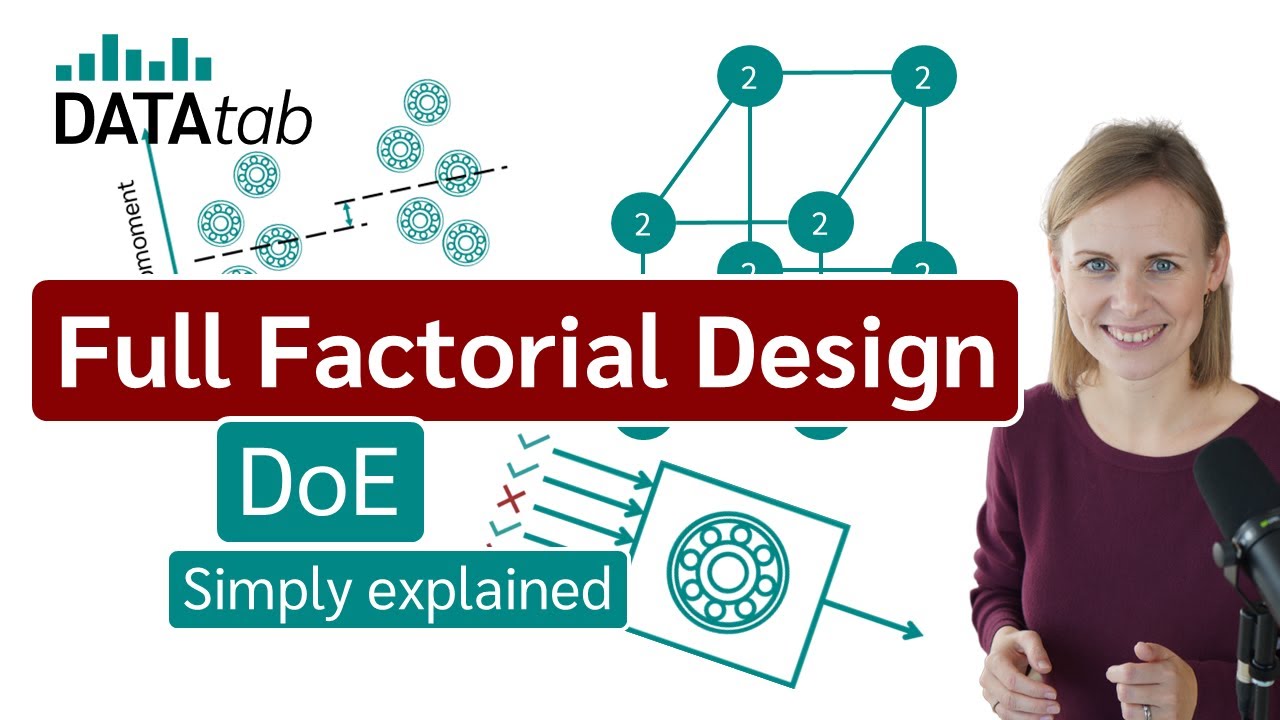
Full Factorial Design (DoE - Design of Experiments) Simply explained

Full Factorial Experiments Explained
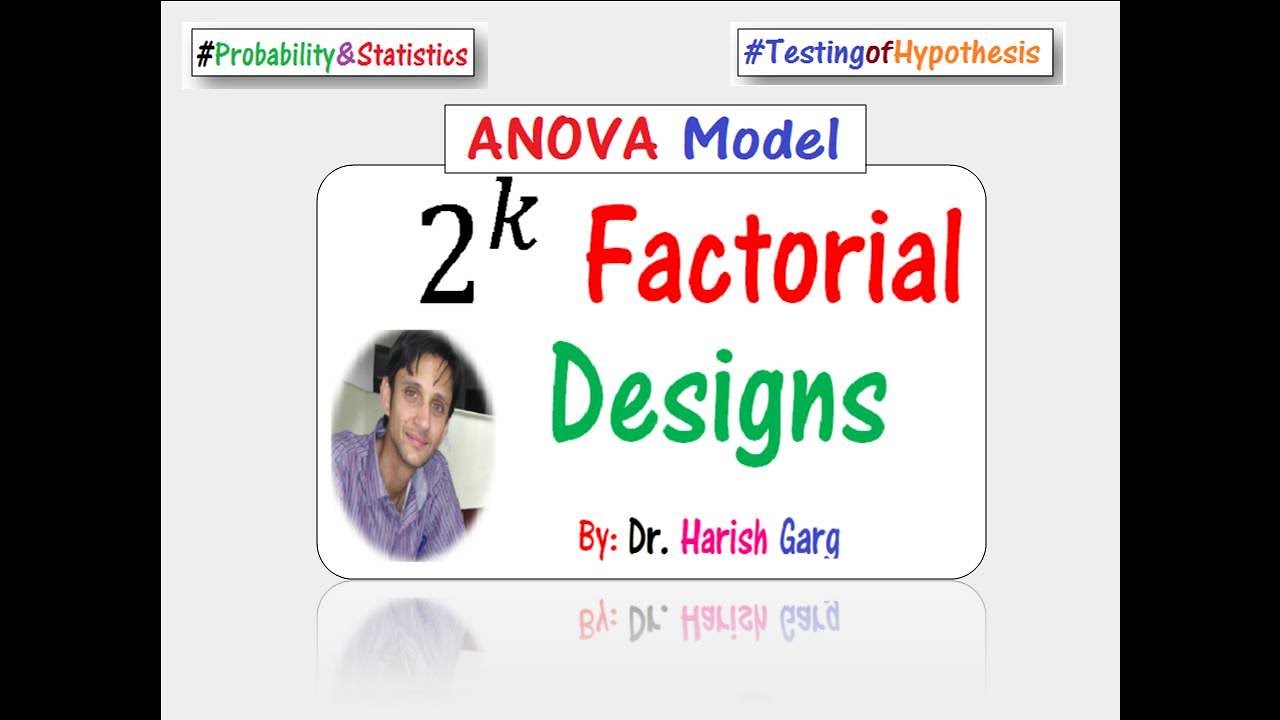
2^k Factorial Designs Experiment - ANOVA Model
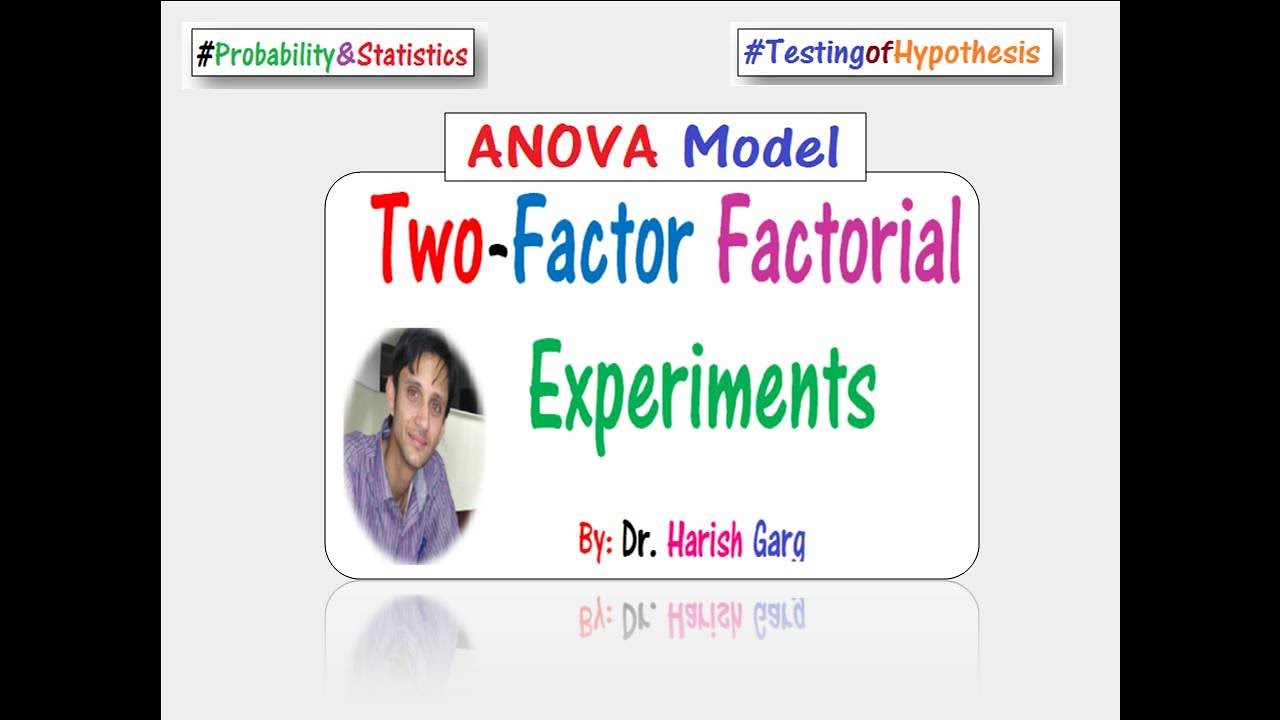
Two-Factor Factorial Design Experiments - ANOVA Model
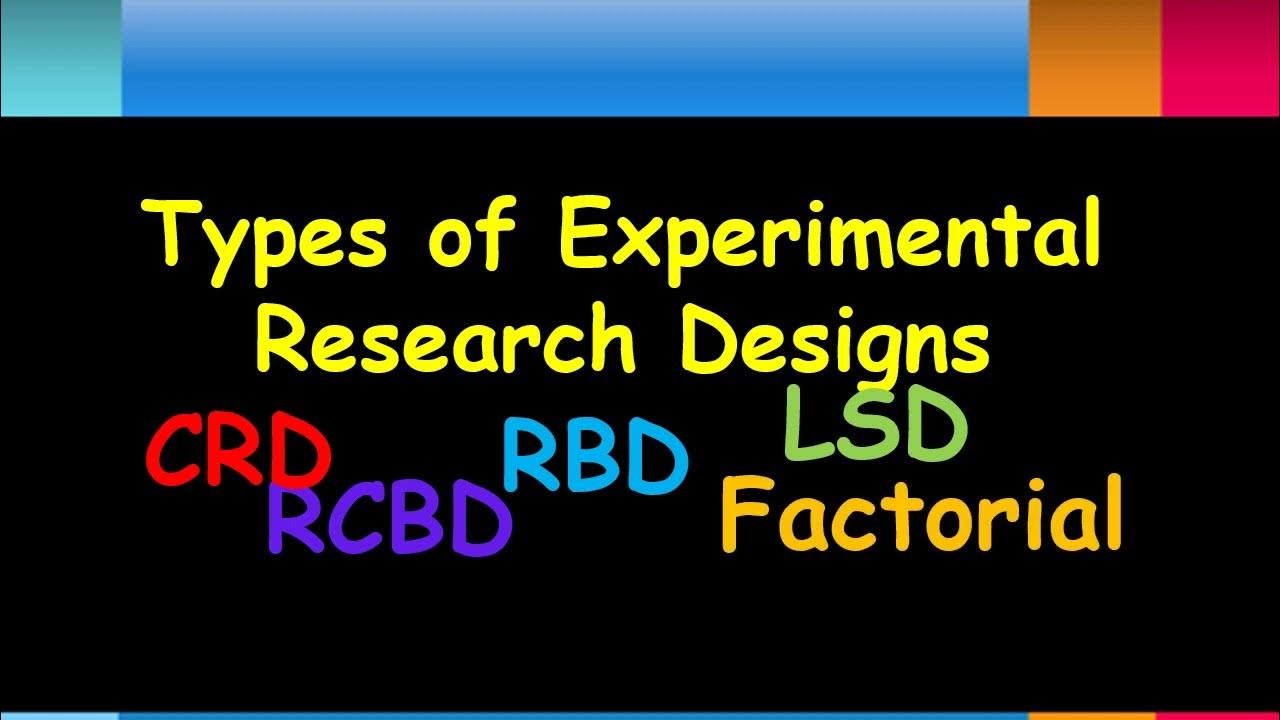
Types of Experimental Research Designs - CRD RBD RCBD LSD FD - Research Methods - Practical Research
5.0 / 5 (0 votes)
Thanks for rating: