Full Factorial Design (DoE - Design of Experiments) Simply explained
TLDRThis video script delves into the concept and application of full factorial designs, a systematic approach to examine multiple factors' effects on a response variable. It illustrates how to create and analyze such designs, using the example of a bike's frictional torque influenced by factors like lubrication and temperature. The script guides through estimating experiment numbers, the benefits of factorial designs in reducing experiments, and analyzing results using statistical tools, concluding with optimizing input parameters for desired outcomes.
Takeaways
- π A full factorial design is a systematic method used to examine the effects of multiple factors and their interactions on a response variable.
- π§ The goal is to analyze the relationship between several input variables (factors) and an output variable (response variable).
- π Each factor in the design has at least two levels, representing specific values that the factor can take, to determine their influence on the response.
- π΄ββοΈ An example given in the script involves examining the frictional torque of a bike bearing under different lubrication conditions and temperatures.
- π The number of experiments needed should be minimized to save time and money, but not so small that important differences may be overlooked.
- π A full factorial design can significantly reduce the number of experiments required compared to testing one factor at a time.
- βοΈ The formula provided in the script can help estimate the number of runs needed based on the standard deviation and the effect size.
- π Full factorial designs can reveal interactions between factors, such as how lubrication and temperature might interact to affect frictional torque.
- π The number of runs in a full factorial design is calculated as 2^K, where K is the number of factors, and grows exponentially with the addition of factors.
- π The script demonstrates how to create and analyze a full factorial design using an online tool, with a focus on interpreting results and determining significant factors.
- π The Pareto diagram and regression coefficients are used to identify significant factors and interactions that affect the response variable, with a focus on p-values and T-values.
Q & A
What is a full factorial design?
-A full factorial design is a systematic method for examining the effects and possible interactions of several factors on a response variable. It aims to analyze the relationship between multiple input variables (factors) and an output variable (response variable).
What are the factors in a full factorial design?
-Factors in a full factorial design are the variables believed to affect the response variable. Each factor has at least two levels, which are the specific values the factor can take.
What is the purpose of using a full factorial design?
-The purpose of using a full factorial design is to determine whether changes in the levels of factors have an influence on the response variable, and to identify interactions between factors that may affect the response.
How can a full factorial design help reduce the number of experiments needed?
-A full factorial design can help reduce the number of experiments by testing multiple factors and their combinations simultaneously, rather than testing one factor at a time, thus providing more information with fewer runs.
What is the formula used to estimate the number of experiments needed in a full factorial design?
-The formula used to estimate the number of experiments needed is n = 2^k, where n is the number of runs and k is the number of factors. This formula applies when each factor has two levels.
How does the number of runs increase with the number of factors in a full factorial design?
-The number of runs increases exponentially with the number of factors. Specifically, the number of runs is 2 raised to the power of the number of factors, which means adding more factors significantly increases the number of required experiments.
What is the role of sample size in estimating the mean value of the response variable?
-The sample size plays a crucial role in estimating the mean value of the response variable. A larger sample size reduces the variability of the mean value, leading to a more precise estimation and making it easier to identify specific differences or effects.
How can the effects of factors and their interactions be analyzed in a full factorial design?
-The effects of factors and their interactions can be analyzed using statistical methods such as regression analysis, Pareto diagrams, and analysis of variance (ANOVA) to determine which factors and interactions have a significant influence on the response variable.
What is the significance of the P-value in analyzing the results of a full factorial design?
-The P-value is used to determine the statistical significance of factors and interactions. A P-value smaller than 0.05 indicates that the factor or interaction has a statistically significant influence on the response variable.
How can the optimal input parameters be determined to minimize or maximize the target value?
-The optimal input parameters can be determined by analyzing the significant factors and interactions from the full factorial design and adjusting them to achieve the desired outcome for the target variable, often using optimization techniques.
Can a full factorial design be used with more than two levels per factor?
-Yes, a full factorial design can be used with more than two levels per factor. However, this increases the number of runs required, as the total number of runs is equal to the product of the number of levels for each factor.
Outlines
π Introduction to Full Factorial Design
This paragraph introduces the concept of a full factorial design, a methodical approach to studying the effects of multiple factors on a response variable. It explains the purpose of using such a design to understand the relationship between input variables (factors) and an output variable. The paragraph uses the example of a bike's bearings to illustrate how different factors like lubrication and temperature can influence the frictional torque. It also raises questions about estimating the number of experiments needed, the workings of a full factorial design, its benefits in reducing the number of experiments, and the analysis of results.
π Estimating Experiments and Factorial Design Efficiency
This section delves into how to estimate the number of experiments required for a full factorial design, emphasizing the balance between cost and the risk of missing significant differences. It introduces a formula for calculating the number of runs based on standard deviation, desired effect size, and sample size. The paragraph also explains how a full factorial design can reduce the number of required experiments by testing multiple factors simultaneously, rather than one at a time. It provides an example with lubrication and temperature factors, showing how combining these in a full factorial design can yield more information with fewer runs. The concept of interactions between factors is introduced, and the benefits of full factorial designs in terms of efficiency and information gain are highlighted.
π Creating and Analyzing Full Factorial Designs
The final paragraph discusses the practical steps for creating and analyzing a full factorial design using an online tool like Data Tab. It outlines the process of defining factors and their levels, creating a test plan, conducting experiments, and recording results. The paragraph explains how to analyze the data using DOE (Design of Experiments) analysis, interpret regression coefficients, and identify significant factors and interactions. It also touches on the difference between coded and uncoded regression coefficients and how to use Pareto diagrams and ANOVA results to determine the optimal input parameters for minimizing or maximizing the target variable. The summary concludes with a note on the limitations of full factorial designs in terms of the number of factors and levels, and a teaser for the next video.
Mindmap
Keywords
π‘Full Factorial Design
π‘Factors
π‘Response Variable
π‘Levels
π‘Interactions
π‘Experiments
π‘Sample Size
π‘Standard Deviation
π‘Regression Coefficients
π‘Pareto Chart
π‘Analysis of Variance (ANOVA)
Highlights
A full factorial design is a systematic method for examining the effects and interactions of multiple factors on a response variable.
The aim of a full factorial design is to analyze the relationship between several input variables (factors) and an output variable (response variable).
Each factor in a full factorial design has at least two levels, representing specific values the factor can take.
The example of bike wheel frictional torque is used to illustrate the application of a full factorial design.
Factors such as lubrication and temperature, with levels like oiled/greased and low/medium/high, can be studied using a full factorial design.
Full factorial designs can lead to significant savings in experiments, especially with more than two variables.
The number of experiments needed can be estimated using a formula involving standard deviation, effect size, and desired precision.
Increasing the sample size reduces the variability of the mean value, leading to a more precise estimation of the mean.
A full factorial design can reduce the number of experiments by testing multiple factor combinations simultaneously.
The number of runs in a full factorial design is calculated as 2^K, where K is the number of factors, each with two levels.
Full factorial designs are generally limited to six or seven factors due to the exponential increase in the number of runs required.
Online tools like data.net can be used to create and analyze full factorial designs.
The significance of factors and interactions is determined by analyzing P-values, with values less than 0.05 indicating significance.
Pareto diagrams can visualize the impact of factors and interactions on the response variable.
Regression coefficients with coded values represent the impact of categorical factor levels as -1 and +1.
The analysis of variance (ANOVA) provides a statistical test to determine the significance of factors and interactions.
Optimal input parameters can be calculated to minimize or maximize the target variable, especially when factors have been measured with three levels.
Transcripts
Browse More Related Video

Design of Experiments (DoE) simply explained
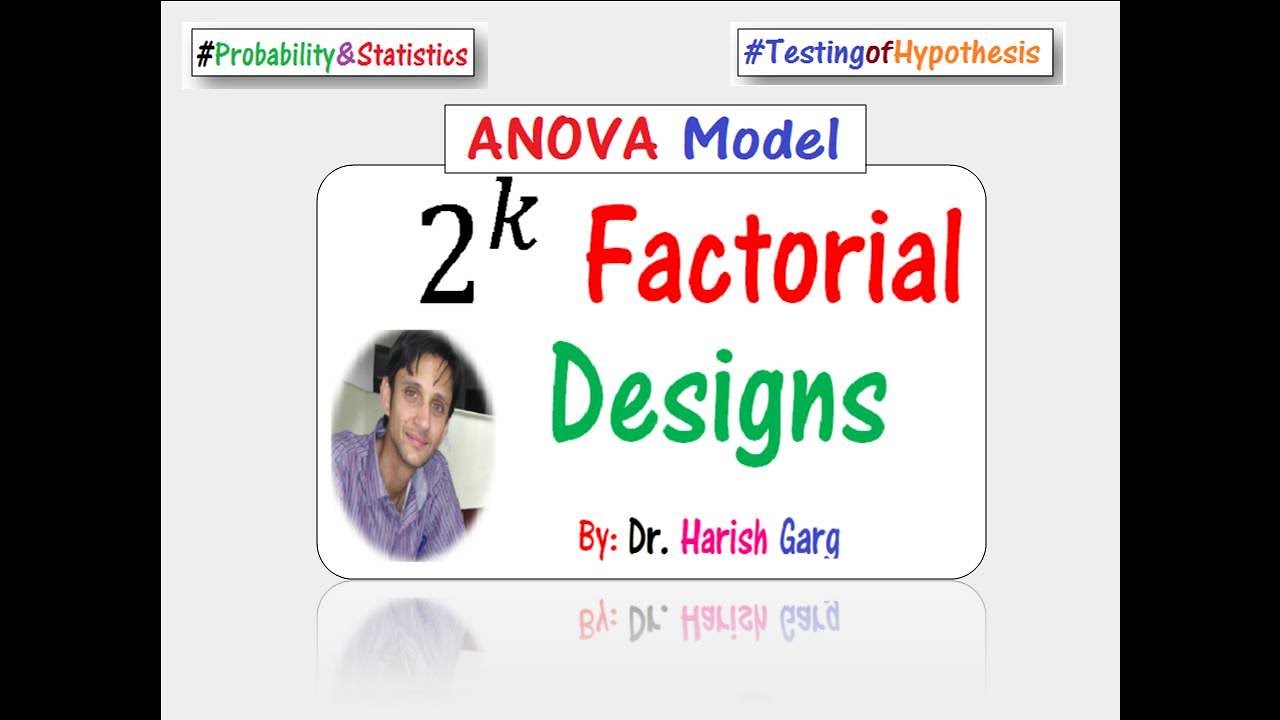
2^k Factorial Designs Experiment - ANOVA Model
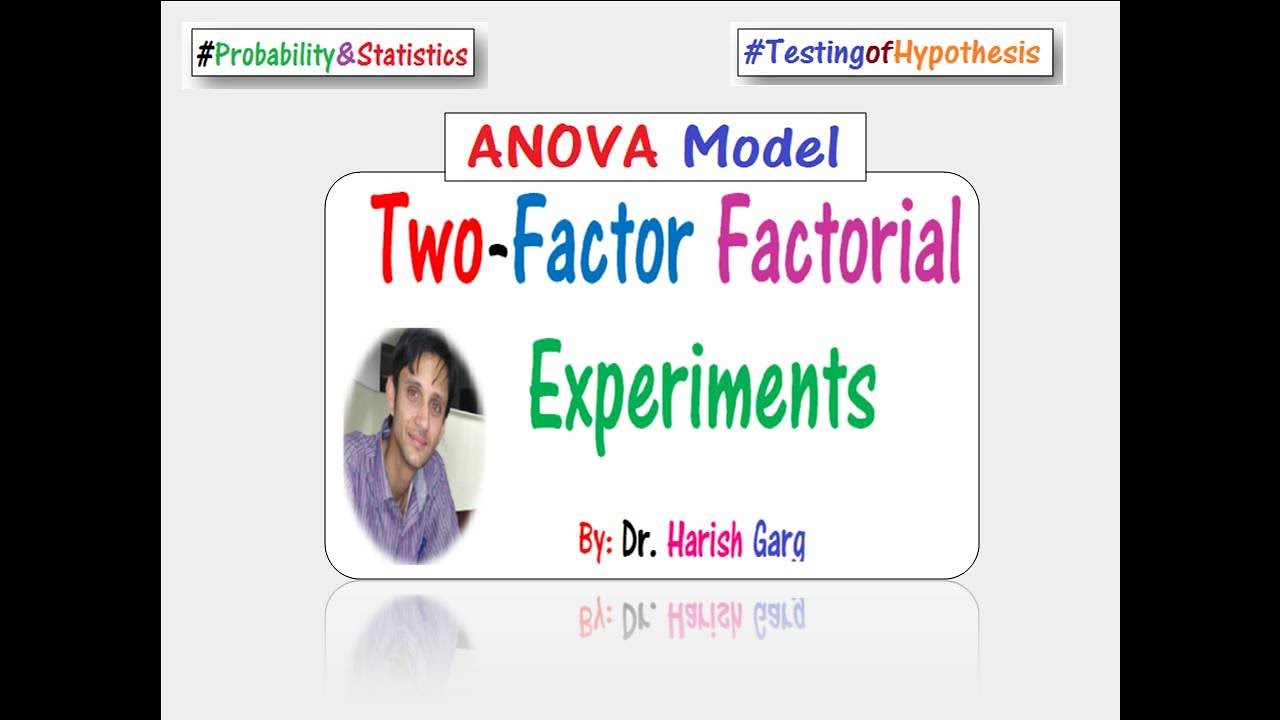
Two-Factor Factorial Design Experiments - ANOVA Model
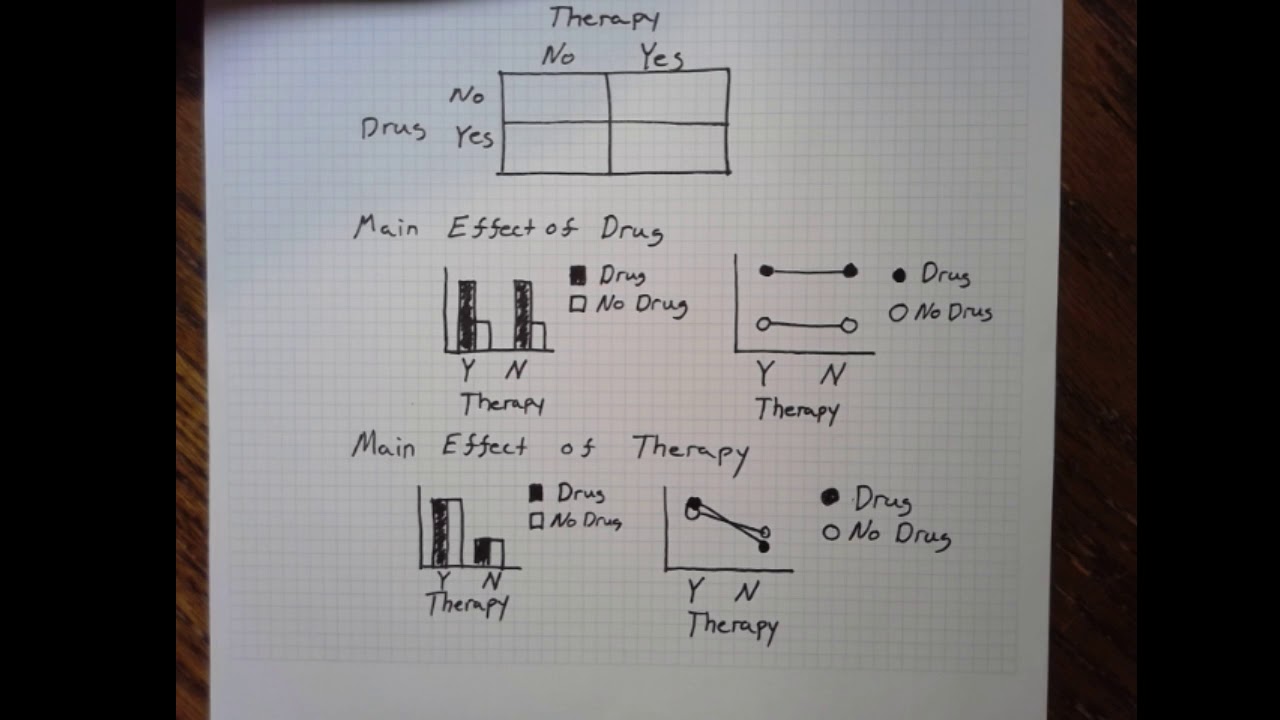
Factorial Designs: Main Effects & Interactions

Full Factorial Experiments Explained
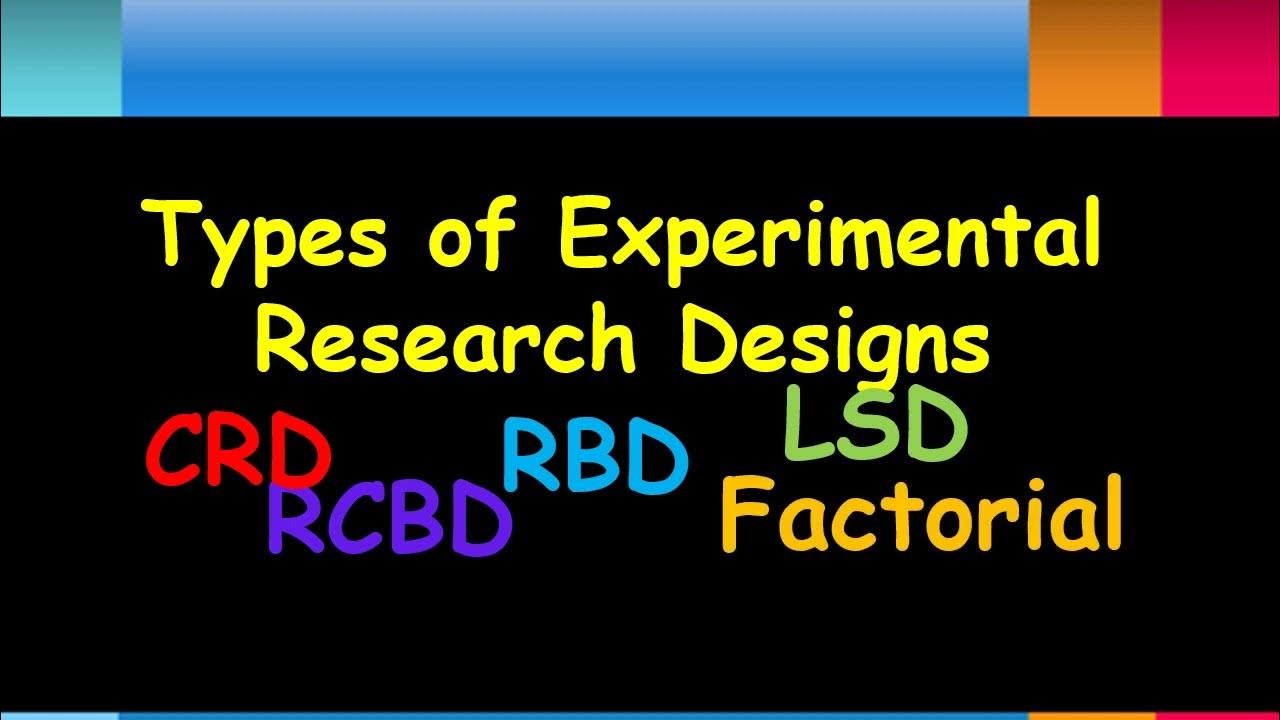
Types of Experimental Research Designs - CRD RBD RCBD LSD FD - Research Methods - Practical Research
5.0 / 5 (0 votes)
Thanks for rating: