Full Factorial Experiments Explained
TLDRThis video explores the concept of full factorial experiments, contrasting them with the traditional one-factor-at-a-time approach. It uses a gardening scenario to illustrate the limitations of not considering variable interactions, such as the combined effect of sunlight and water on rose growth. The full factorial method, though potentially leading to a combinatorial explosion with many factors, offers a more comprehensive understanding by capturing these interactions, providing insights that could be missed with simpler experimental designs.
Takeaways
- π± The video discusses the concept of a full factorial experiment and its alternatives, focusing on its use, advantages, and disadvantages.
- π An example of growing roses is used to illustrate the traditional one-factor-at-a-time (OFAT) approach versus the full factorial method.
- π The OFAT method involves varying one variable at a time, such as sunlight or water, while keeping other variables constant to measure their individual effects.
- π§ In the example, roses grew more with low sunlight and low water when tested separately, suggesting these conditions might be optimal.
- π The full factorial experiment, however, explores all possible combinations of variables, revealing interactions that OFAT might miss.
- π The full factorial approach uncovered that high sunlight and high water together resulted in the best growth, a finding not apparent with OFAT.
- π Full factorial experiments are advantageous for capturing variable interactions but can lead to a combinatorial explosion with many factors or levels.
- π§© The number of required experiments grows exponentially with the formula (level)^(factor), which can become impractical with numerous variables.
- π Despite the potential for combinatorial explosion, strategies exist to reduce the number of experiments needed, making full factorial feasible even with many factors.
- π€ The video acknowledges the support of patrons on Patreon, highlighting the community aspect and the role of patrons in enabling video production.
- π Viewers are encouraged to become patrons for early access to videos, behind-the-scenes content, and to join a community of like-minded individuals.
Q & A
What is a full factorial experiment?
-A full factorial experiment is a type of experimental design where all possible combinations of different levels of factors are tested. It allows for the study of the effects of multiple variables and their interactions on the outcome of an experiment.
What is the traditional alternative to a full factorial experiment?
-The traditional alternative is the one-factor-at-a-time (OFAT) experiment, where only one variable is changed at a time while others are held constant, to observe its effect on the outcome.
Why might a gardener be interested in conducting a full factorial experiment with roses?
-A gardener might be interested in a full factorial experiment to understand how different combinations of factors like sunlight and water affect the growth of roses, potentially discovering optimal conditions that are not evident when only one factor is varied at a time.
What is the main advantage of a full factorial experiment over the one-factor-at-a-time approach?
-The main advantage is that a full factorial experiment can capture interactions between variables, which can lead to a more comprehensive understanding of the system and reveal optimal conditions that might not be found using the one-factor-at-a-time approach.
What is the downside of conducting a full factorial experiment with many factors or levels?
-The downside is the potential for a combinatorial explosion, where the number of experiments required grows exponentially with the number of factors and levels, making the experiment impractical or too time-consuming.
What does the term 'interaction' refer to in the context of a full factorial experiment?
-In the context of a full factorial experiment, 'interaction' refers to the effect that one factor has on the outcome depending on the level of another factor, which can only be observed when both factors are varied simultaneously.
How many experimental runs are required for a two-factor interaction experiment with two levels each?
-A two-factor interaction experiment with two levels for each factor requires 2 x 2, or 4, experimental runs to cover all possible combinations.
What is the term used to describe the situation where the number of required experiments grows exponentially with the number of factors and levels?
-The term used to describe this situation is 'factorial explosion' or 'combinatorial explosion'.
Can you provide an example of how a full factorial experiment might reveal different results compared to a one-factor-at-a-time experiment?
-In the script, the example shows that when sunlight and water were tested separately, low levels of both were thought to be optimal. However, the full factorial experiment revealed that high levels of both actually resulted in the best growth, a finding that would have been missed with the one-factor-at-a-time approach.
What strategies can be used to reduce the number of experiments in a full factorial design when dealing with many factors or levels?
-Strategies such as fractional factorial designs, response surface methodology, or using statistical techniques like Taguchi methods can be employed to reduce the number of experiments while still capturing important interactions between factors.
Outlines
π± Full Factorial Experiments: An Introduction
This paragraph introduces the concept of full factorial experiments, contrasting them with the traditional one-factor-at-a-time (OFAT) approach. It uses the analogy of growing roses to explain the process, focusing on two variables: sunlight and water. The OFAT method is described, where sunlight and water are adjusted independently to observe their effects on rose growth. The paragraph highlights the limitations of OFAT, such as missing potential interactions between variables, which can lead to suboptimal conclusions about the best conditions for growth.
π Advantages of Full Factorial Experiments
This paragraph delves into the advantages of full factorial experiments over the OFAT method. It illustrates how varying all possible combinations of factors, in this case, water and sunlight, can reveal interactions between them. The example given shows that the best growth condition for roses is a combination of high sunlight and high water, which would not have been discovered using the OFAT method. The paragraph emphasizes the ability of full factorial experiments to capture these interactions and provide more comprehensive insights, despite the increased number of experimental runs required.
π Factorial Explosion and Efficient Experimental Design
The final paragraph discusses the potential downside of full factorial experiments, which is the combinatorial explosion that occurs when there are many factors and levels to test. It explains that this can lead to an impractical number of experiments. However, it also introduces the concept of being 'clever' with experimental design to reduce the number of runs without losing valuable information. The paragraph concludes with a call to action for patrons on Patreon, acknowledging their support as crucial for the creation of such educational content.
Mindmap
Keywords
π‘Full factorial experiment
π‘Advantages
π‘Disadvantages
π‘Traditional experiment
π‘Variables
π‘Sunlight
π‘Water
π‘Interactions
π‘Combinatorial explosion
π‘One-factor-at-a-time (OFAT)
π‘Experimental runs
Highlights
Introduction to the concept of full factorial experiments and their alternatives.
Explanation of a traditional experiment setup with roses, focusing on sunlight and water as variables.
Description of a one-factor-at-a-time (OFAT) experiment method with its limitations.
Illustration of how OFAT experiments might lead to incorrect conclusions about optimal conditions.
Introduction of full factorial experiments as an alternative to capture interactions between variables.
Demonstration of how full factorial experiments can reveal optimal conditions missed by OFAT.
Explanation of two-factor interactions and their significance in full factorial experiments.
Advantage of full factorial experiments in measuring interactions with fewer experimental runs.
Discussion on the potential downside of factorial experiments with many factors leading to combinatorial explosion.
Mention of strategies to reduce the number of experiments needed in the presence of many factors or levels.
Clarification of factorial notation, such as 'three to the five factorial', to explain the scale of experiments.
Highlight of the practical applications of full factorial experiments in various scientific studies.
Emphasis on the importance of considering interactions between variables in experimental design.
Acknowledgment of the role of patrons in supporting the creation of educational content.
Invitation for viewers to become patrons for early access and exclusive content.
Transcripts
Browse More Related Video
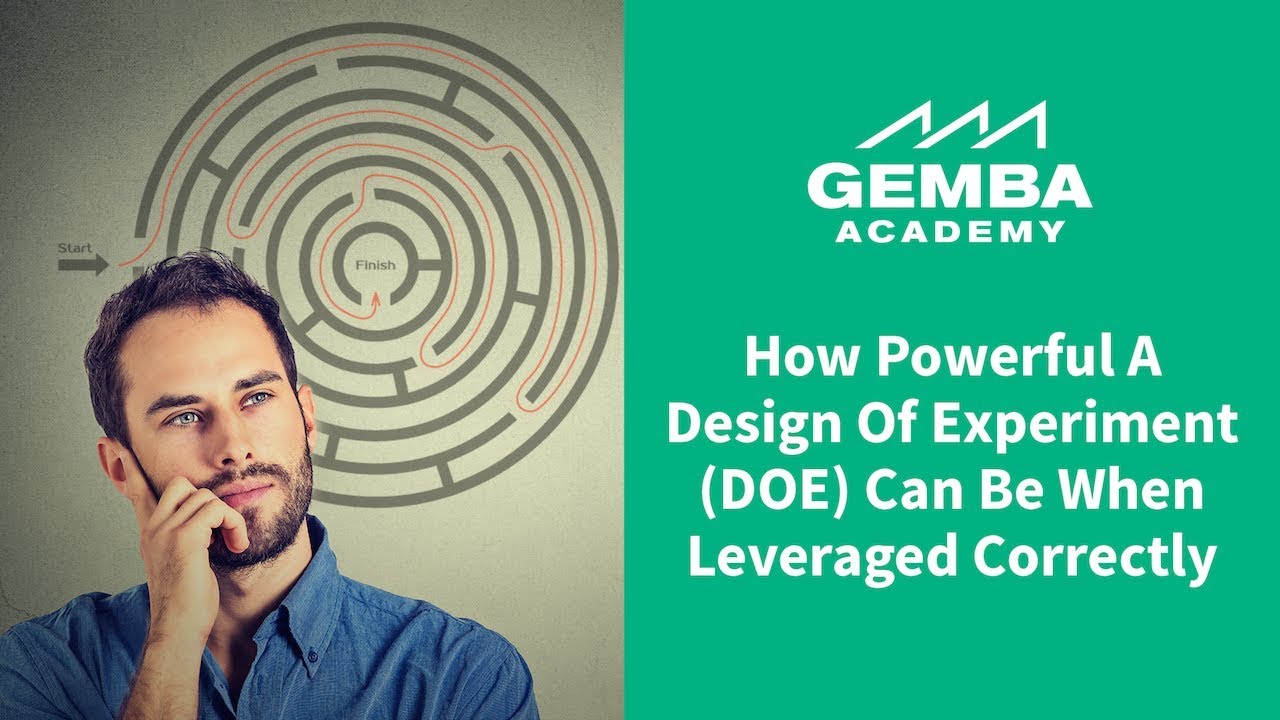
Learn How Powerful a Design of Experiment (DOE) Can Be When Leveraged Correctly
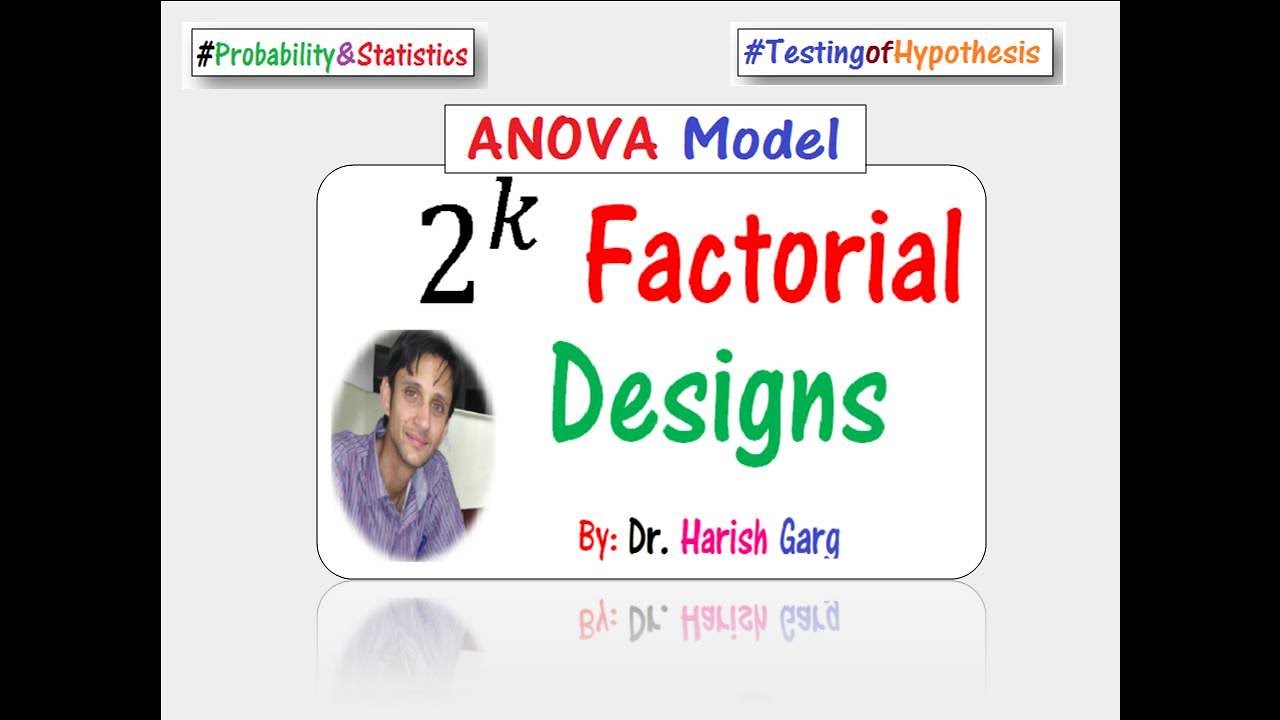
2^k Factorial Designs Experiment - ANOVA Model
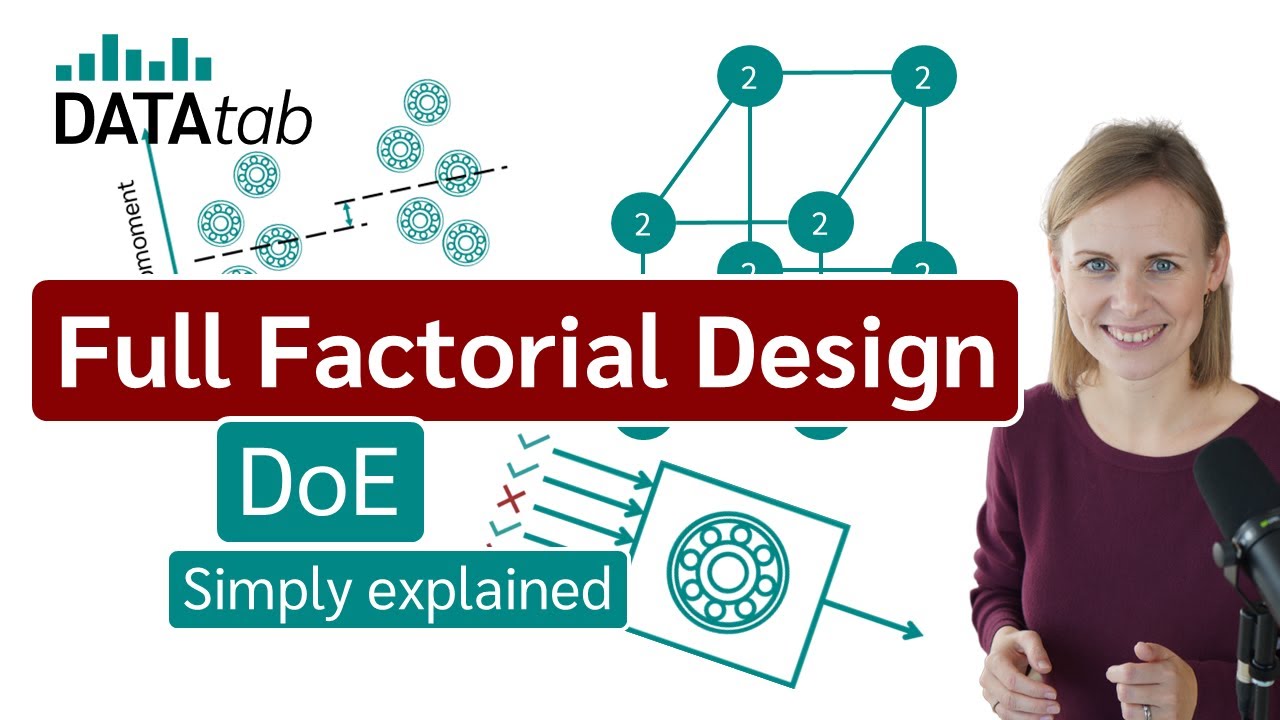
Full Factorial Design (DoE - Design of Experiments) Simply explained

Design of Experiments (DoE) simply explained
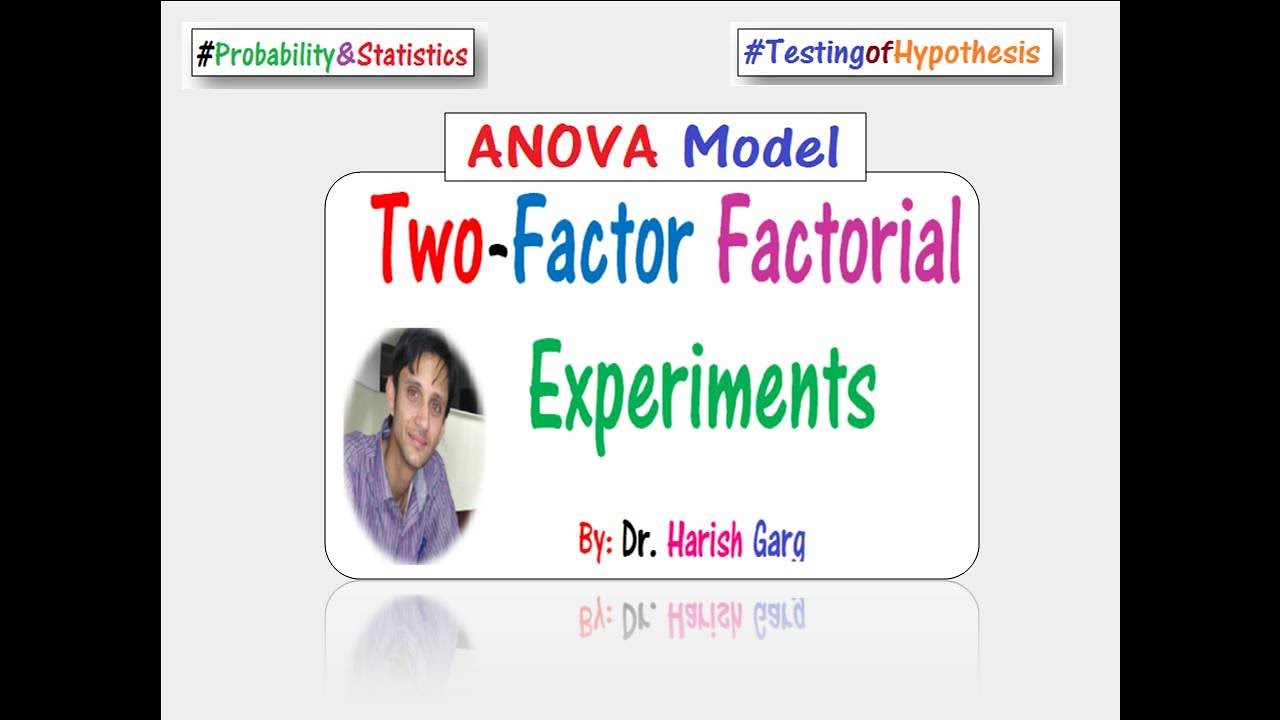
Two-Factor Factorial Design Experiments - ANOVA Model
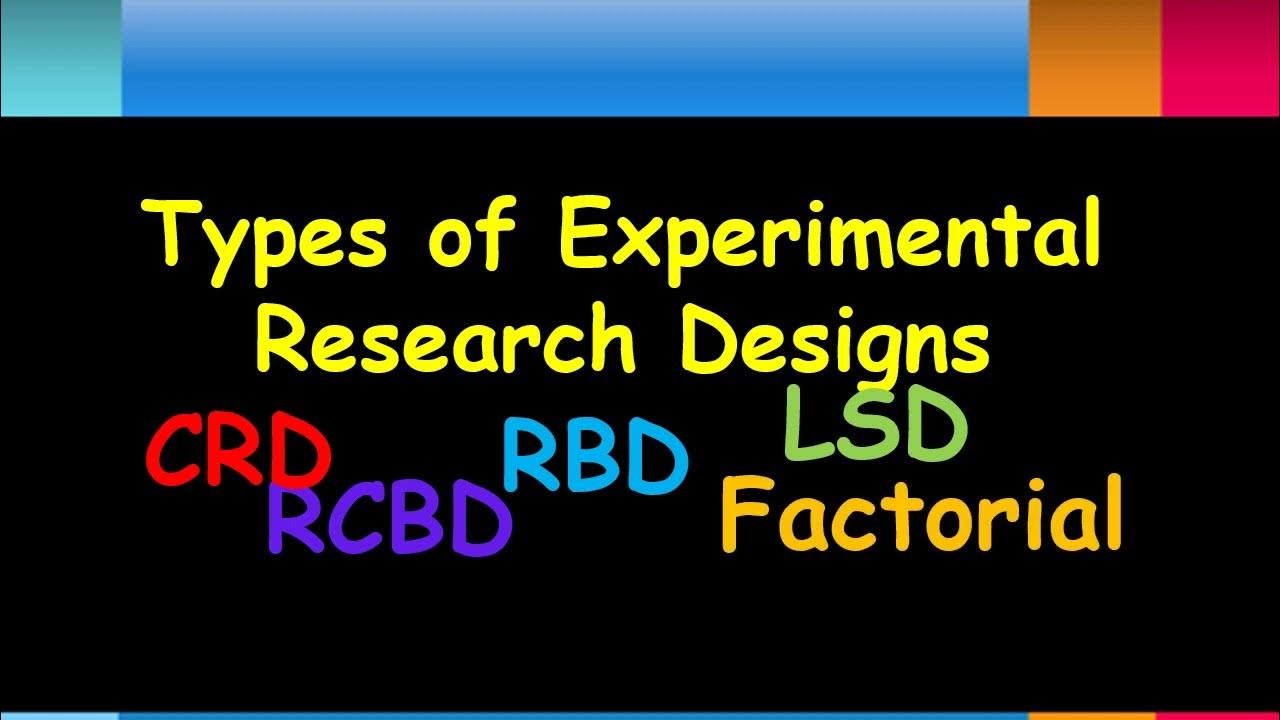
Types of Experimental Research Designs - CRD RBD RCBD LSD FD - Research Methods - Practical Research
5.0 / 5 (0 votes)
Thanks for rating: