Learn How Powerful a Design of Experiment (DOE) Can Be When Leveraged Correctly
TLDRThe video script introduces the concept of Design of Experiments (DoE), emphasizing its power in optimizing processes. It clarifies the misconception that one-factor-at-a-time (OFAT) experiments are equivalent to DoE, highlighting the importance of testing multiple factors and their interactions. The script outlines three types of DoE: screening, which identifies significant factors; full factorial, which tests all factor combinations for statistical significance; and optimization, which finds the best factor settings to meet specific goals. The video promises a deeper exploration of full factorial DoE in the next module, positioning it as a crucial tool for process improvement.
Takeaways
- π A Design of Experiments (DoE) is a structured method used to understand the impact of multiple factors on a process.
- π By the end of the module, you'll understand the key differences between DoE and One Factor At A Time (OFAT) experiments.
- π DoE is powerful for identifying the most significant inputs (X's) that affect a process, which is crucial for process optimization.
- β½οΈ An example given in the script involves a motorcycle manufacturer studying how tire pressure, speed, and gasoline octane impact fuel consumption.
- π« The script clarifies that One Factor At A Time (OFAT) experiments are not the same as DoE, despite a common misconception.
- π’ In a full factorial DoE, every possible combination of factors is tested, which can reveal interactions between factors that OFAT might miss.
- π The script uses a funnel analogy to describe the process of narrowing down critical inputs using various tools, with DoE being the final tool in the process.
- π§ Hypothesis testing is a review concept that's important for understanding DoE, as it helps make decisions about population parameters based on sample statistics.
- π οΈ Tools like process mapping, cause-and-effect matrices, and FMEA are used to initially identify and narrow down critical inputs before applying DoE.
- π Screening DoEs are used to quickly screen many factors to determine which are most significant, while optimization DoEs are used to find the optimal factor settings.
- π‘ Full factorial DoEs are more comprehensive but can be time-consuming and expensive, which is why screening designs are often used first to reduce the number of factors.
- βοΈ The next module will focus on full factorial DoEs, which are a more advanced form of experimentation that can provide robust insights into process optimization.
Q & A
What is the main topic of this module?
-The main topic of this module is the introduction to Design of Experiments (DoE), including its definition, power, and the difference between DoE and One Factor at a Time (OFAT) experiments.
What is the purpose of a Design of Experiments (DoE)?
-The purpose of a Design of Experiments is to provide a structured way to change multiple factors or settings to understand their impact on the process or system under study.
What is the difference between a full factorial DoE and a one factor at a time (OFAT) experiment?
-A full factorial DoE tests every possible combination of factor levels, allowing for the detection of interactions between factors. An OFAT experiment, on the other hand, changes only one factor at a time while keeping others constant, which does not account for factor interactions and can lead to incomplete or misleading conclusions.
Why is it important to understand the concept of two-way ANOVA when learning about DoE?
-Two-way ANOVA is an example of a full factorial DoE where two factors are studied at two levels each. Understanding this concept helps in grasping the more general principles of DoE, which can handle multiple factors and levels.
What are the three most popular types of DoE mentioned in the script?
-The three most popular types of DoE mentioned are screening DoE, full factorial DoE, and optimization DoE.
What is the primary goal of a screening DoE?
-The primary goal of a screening DoE is to quickly screen many factors at one time to determine which factors are significant and worthy of deeper investigation.
How does a full factorial DoE differ from a screening DoE in terms of the number of trials required?
-A full factorial DoE requires more trials because it tests every possible combination of factor levels. For example, with three factors at two levels each, a full factorial DoE would require eight trials, whereas a screening DoE might test fewer combinations to narrow down the significant factors.
What is the main advantage of an optimization DoE?
-The main advantage of an optimization DoE is that it helps identify the optimal factor settings to achieve specific targets or outcomes, making it highly robust and useful for fine-tuning processes.
Why are one factor at a time experiments considered dangerous and ineffective?
-One factor at a time experiments are considered dangerous and ineffective because they do not test the combination of factors and can miss important interactions between factors, leading to suboptimal conclusions about the system under study.
What tool or technique is used to narrow down the list of inputs identified during process mapping?
-A cause-and-effect matrix is used to identify critical inputs based on their impact to key customer requirements, which helps in narrowing down the list of inputs identified during process mapping.
How does the Failure Modes and Effects Analysis (FMEA) contribute to the process of narrowing down inputs?
-FMEA is used to identify potential ways in which a product or process can fail, which helps the team to identify necessary countermeasures. It also aids in further narrowing down the list of inputs through the analysis of the Risk Priority Number (RPN).
What statistical test was mentioned in the script that can help determine if the means of two different variables are statistically different?
-The two-sample t-test was mentioned as a statistical test that can help determine if the means of two different variables are statistically different.
Outlines
π Introduction to Design of Experiments (DoE)
This paragraph introduces the concept of Design of Experiments (DoE), emphasizing its power and importance in optimizing processes. It outlines the learning objectives: understanding what DoE is, its advantages over one-factor-at-a-time (OFAT) experiments, and familiarity with the three most popular types of DoE. The paragraph also reviews previously covered tools like process maps, cause-and-effect matrices, FMEA, and hypothesis testing, positioning DoE as the final tool for refining the critical inputs list. A formal definition of DoE is provided, explaining its structured approach to varying multiple factors to assess their impact on a process. The example of a motorcycle manufacturer's fuel consumption study illustrates the application of DoE, highlighting the need to control and optimize critical inputs for optimal performance.
π« Pitfalls of One-Factor-at-a-Time (OFAT) Experiments
This paragraph contrasts the DoE with the one-factor-at-a-time (OFAT) approach, which is often mistakenly considered a form of DoE. The narrative uses a motorcycle fuel efficiency example to demonstrate how OFAT can miss critical interactions between factors, as it only tests one variable at a time. The paragraph explains that a full factorial DoE, which tests all factor combinations, would require eight trials for three factors at two levels, whereas the OFAT example only conducted four. It emphasizes the danger and ineffectiveness of OFAT due to its inability to account for factor interactions. The paragraph concludes by introducing the three types of DoE to be explored: screening, full factorial, and optimization, each serving different purposes in the process of experimentation and analysis.
Mindmap
Keywords
π‘Design of Experiments (DoE)
π‘One Factor at a Time (OFAT)
π‘Two-Way ANOVA
π‘Process Maps
π‘Cause-and-Effect Matrix
π‘Failure Mode and Effects Analysis (FMEA)
π‘Hypothesis Testing
π‘Screening DoE
π‘Full Factorial DoE
π‘Optimization DoE
π‘Risk Priority Number (RPN)
Highlights
Introduction to Design of Experiments (DoE), a powerful method for understanding the impact of multiple factors on a process.
Understanding the key difference between DoE and One Factor at a Time (OFAT) experiments.
The three most popular types of DoE: Screening, Full Factorial, and Optimization.
Process mapping and Cause-and-Effect matrix (C&E) for identifying critical inputs.
Using Failure Modes and Effects Analysis (FMEA) to identify potential product or process failures.
The role of hypothesis testing in making decisions about population parameters using sample statistics.
The funnel approach to narrowing down critical inputs using various tools like process mapping, C&E matrix, FMEA, and hypothesis testing.
Formal definition of a Design of Experiment as a structured way to change multiple settings to understand their impact.
The concept of Full Factorial DoE through the example of Two-way ANOVA.
The importance of testing factor combinations, not just individual factors, in DoE.
The fictitious motorcycle manufacturer example to illustrate the limitations of OFAT compared to DoE.
The discovery of an interaction effect between factors that would have been missed with OFAT.
The dangers and ineffectiveness of one factor at a time experiments due to lack of combination testing.
Screening DoEs for screening many factors simultaneously to determine significant ones.
Full Factorial DoEs for testing factors across all possible combinations to find statistically significant factors.
Optimization DoEs for identifying optimal factor settings to achieve specific targets.
The robustness comparison between Screening and Optimization DoEs.
Summary of the introductory DoE module and a preview of the next lesson on Full Factorial DoEs.
Transcripts
Browse More Related Video

Design of Experiments (DoE) simply explained
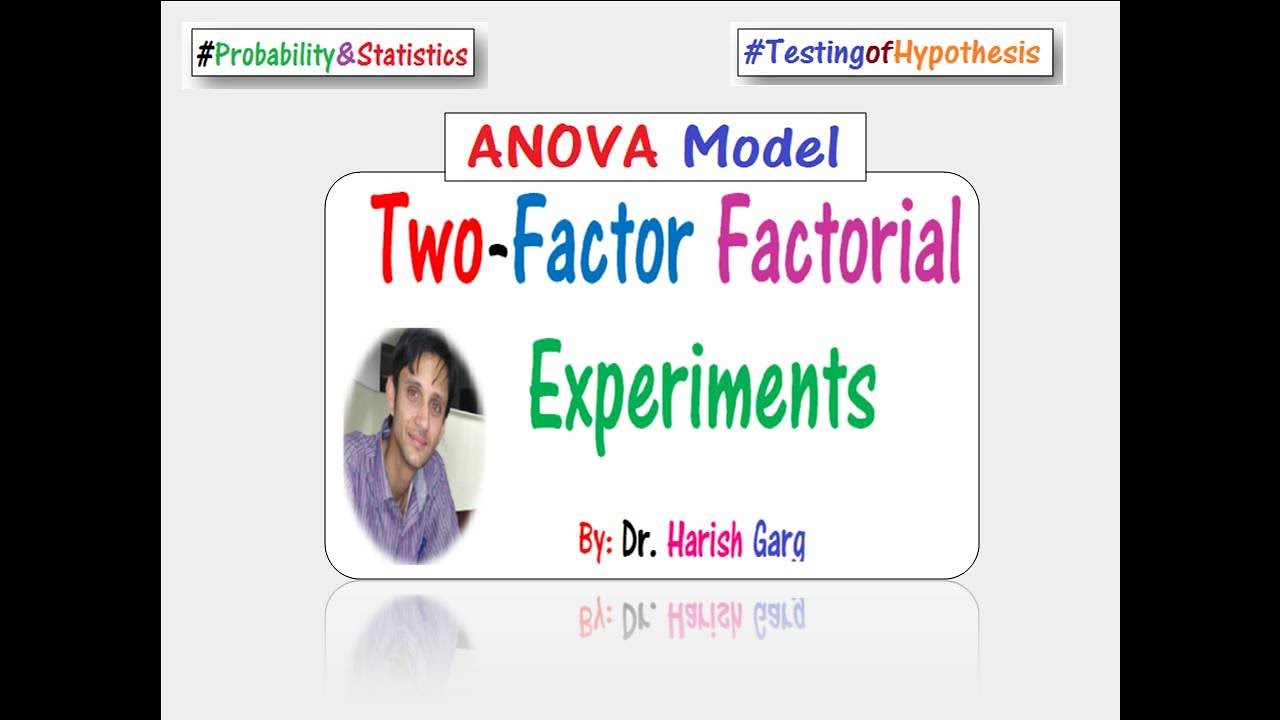
Two-Factor Factorial Design Experiments - ANOVA Model

Full Factorial Experiments Explained
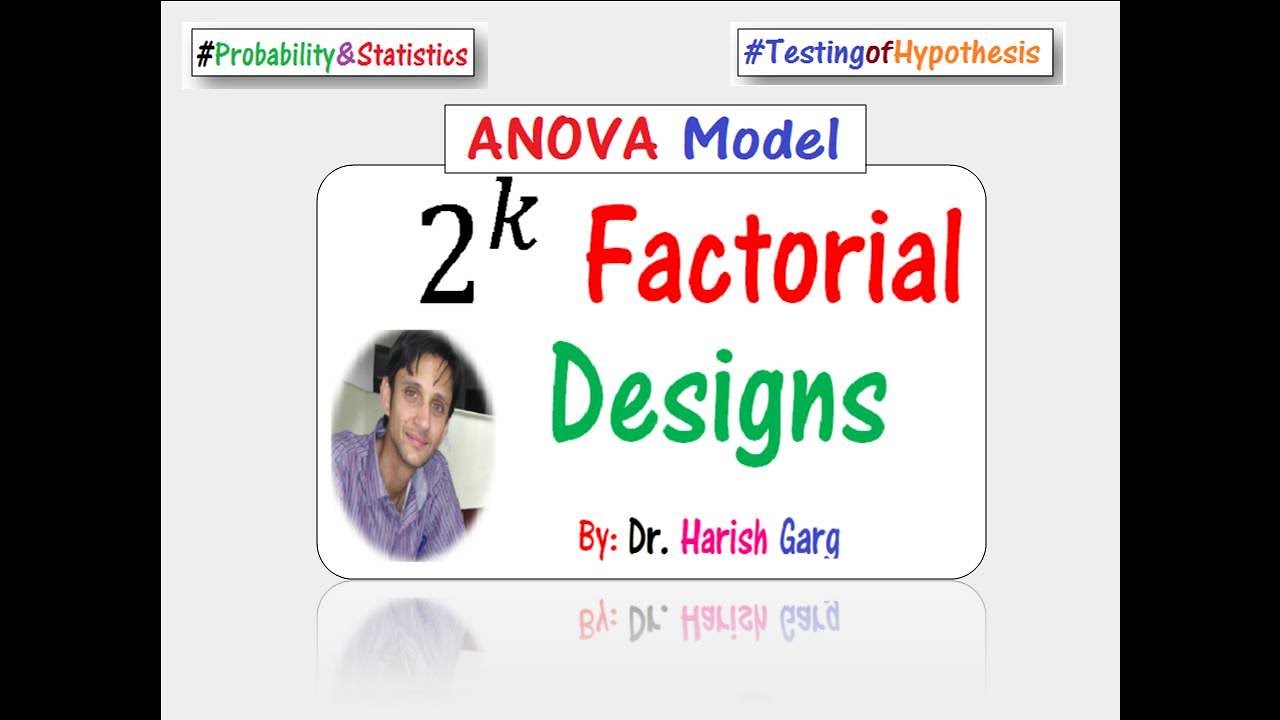
2^k Factorial Designs Experiment - ANOVA Model
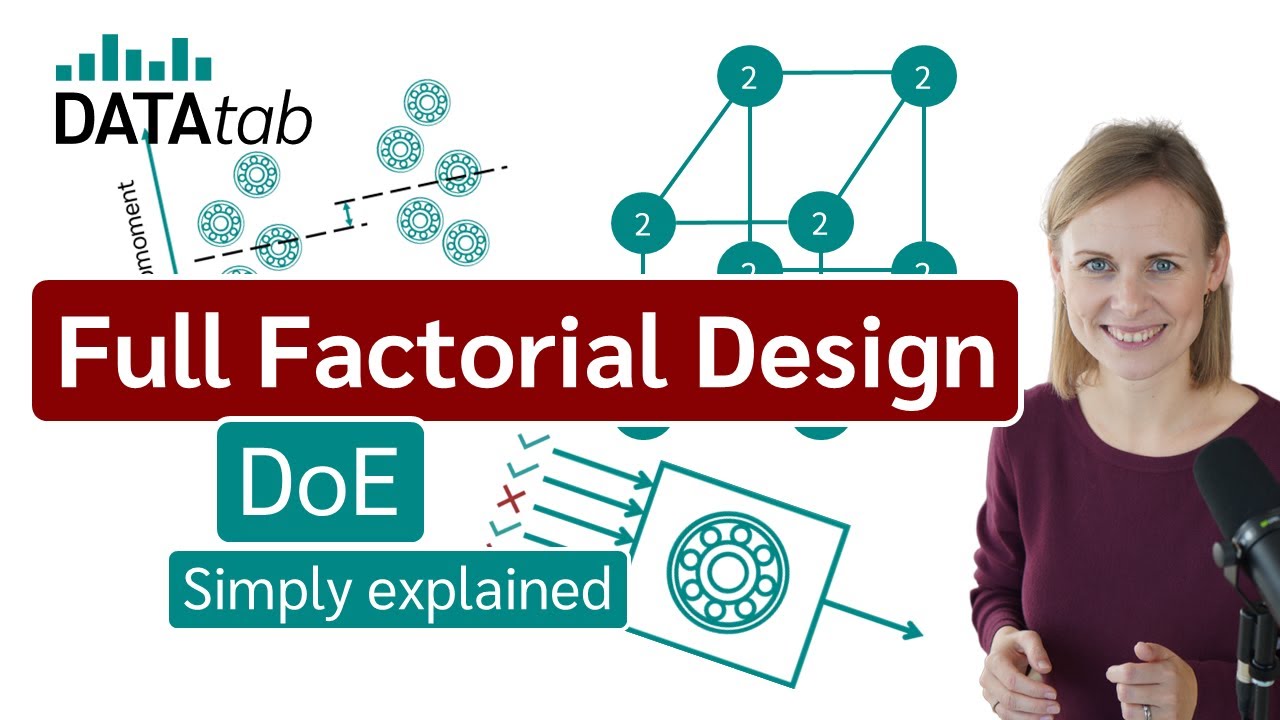
Full Factorial Design (DoE - Design of Experiments) Simply explained
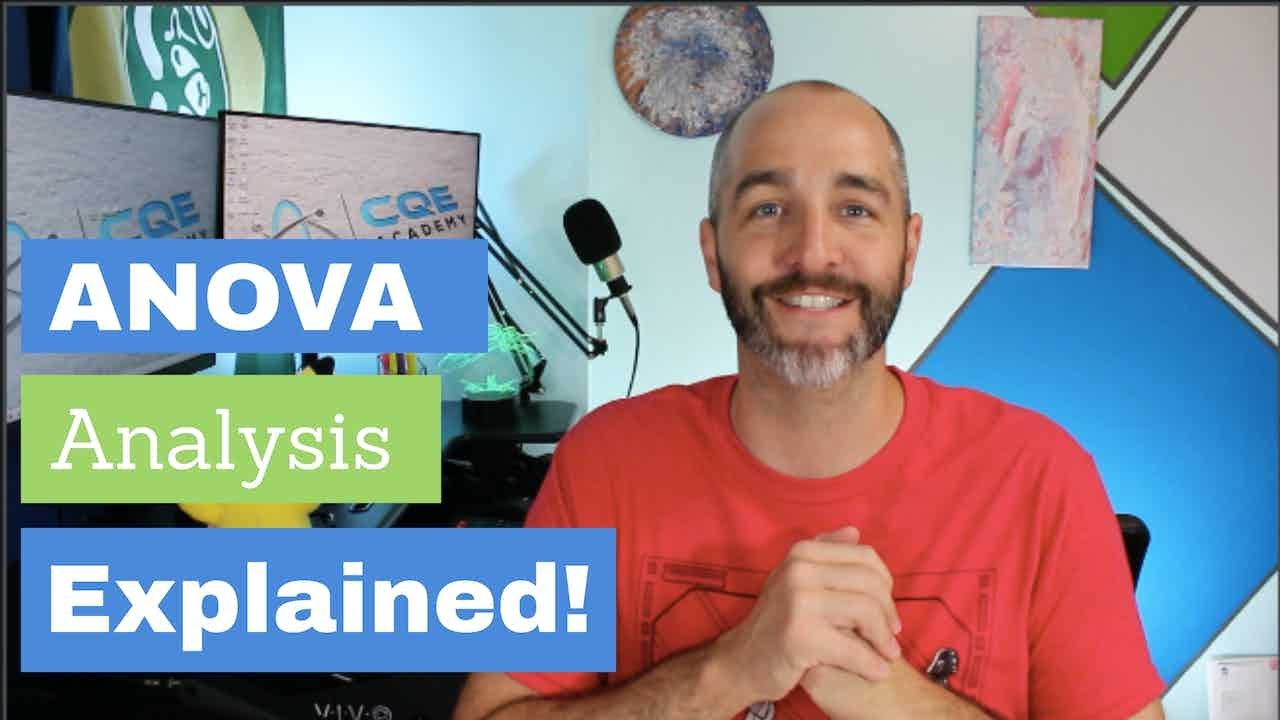
ANOVA (Analysis of Variance) Analysis β FULLY EXPLAINED!!!
5.0 / 5 (0 votes)
Thanks for rating: