9.1.5 Two Proportions - Hypothesis Testing and Confidence Intervals When Requirements Are Not Met
TLDRThis video lesson delves into alternative methods for testing hypotheses about two proportions when the conditions for using the critical value, p-value, or confidence interval methods are not met. It revisits the necessary requirements for these methods, such as having two independent simple random samples with at least five successes and failures each. The video introduces Fisher's exact test for hypothesis testing and bootstrap resampling for constructing confidence intervals when the sample size condition is not satisfied. It also highlights the limitations of non-generalizable data from voluntary or convenience samples.
Takeaways
- π The video discusses learning outcome number five from lesson 9.1, focusing on testing hypotheses about two proportions when certain statistical methods are not applicable.
- π The prerequisites for using the critical value method, p-value method, or the confidence interval method include having two simple random samples that are independent of each other.
- π« Independence means values from one population should not be related to or paired with values from the other, avoiding scenarios like twins or before and after data.
- π A normal distribution can be used as an approximation for the binomial distribution if there are at least five successes and five failures in each sample, represented as n*p_hat β₯ 5 and n*q_hat β₯ 5.
- π« If the sample size requirement is not met, the normal distribution should not be used as an approximation, but the exact binomial distribution can still be applied.
- π If the sample requirements are not met, there's no way to salvage the data from a voluntary response or convenience sample for generalization.
- π§ Fisher's exact test is an alternative for hypothesis testing when the sample size requirement is not met, utilizing the binomial distribution without normal approximation.
- π€ Technology can assist with the complex computations involved in Fisher's exact test, which is not detailed in the textbook but is an available option.
- π Bootstrap resampling methods can be used to construct a confidence interval for the difference between two population proportions if the sample size requirement is not met.
- π Bootstrap methods are discussed in section 7.4 of the textbook for constructing confidence intervals when the sample size requirement is not met.
- π The next video will cover testing hypotheses about two population means, focusing on the difference between population means rather than proportions.
Q & A
What are the two main requirements for testing hypotheses about two proportions using the critical value method, p-value method, or the confidence interval method?
-The two main requirements are: 1) having two simple random samples, and 2) ensuring those samples are independent from each other, meaning the values from one population are not related to or naturally paired with values from the other population.
What does it mean for samples to be 'independent' in the context of hypothesis testing?
-Samples are considered 'independent' when the values from one population are not influenced by or related to the values from the other population. This prevents issues like twins, partners in a romantic couple, or before-and-after data from skewing the results.
What is the minimum number of successes and failures required in each sample for using a normal distribution as an approximation to the binomial distribution?
-There should be at least five successes and five failures in each sample, which can be represented as n times p hat being greater than or equal to five, and n times q hat being greater than or equal to five.
What is the alternative method for hypothesis testing if the requirement of having at least five successes and five failures in each sample is not met?
-If the requirement is not met, Fisher's exact test can be used, which is more complex and uses the binomial distribution without the normal approximation.
What is Fisher's exact test and how is it different from the normal approximation method?
-Fisher's exact test is a statistical test used when the sample sizes are too small for the normal approximation to be valid. It is more computationally intensive and does not rely on the normal approximation to the binomial distribution.
If the sample requirements are not met, can the results from a hypothesis test still be generalizable?
-No, if the sample requirements are not met, such as having a voluntary response or convenience sample, the results from the hypothesis test will not be generalizable to anyone outside that specific sample.
What is the alternative method for constructing a confidence interval when the requirement of having at least five successes and five failures in each sample is not met?
-Bootstrap resampling methods can be used to construct a confidence interval for the difference between two population proportions when the sample requirements are not met.
Where in the textbook can I find information about bootstrap resampling methods?
-Bootstrap resampling methods are discussed in lesson 7.4 or section 7.4 of the textbook.
What will be the topic of the next video in the series?
-The next video will discuss testing hypotheses about two samples, specifically focusing on two population means and the difference between population means, as opposed to the difference between two population proportions.
What is the symbolic representation of the requirement for the number of successes in a sample?
-The symbolic representation of the requirement for the number of successes in a sample is n times p hat being greater than or equal to five, where n is the sample size and p hat is the sample proportion of successes.
What is the symbolic representation of the requirement for the number of failures in a sample?
-The symbolic representation of the requirement for the number of failures in a sample is n times q hat being greater than or equal to five, where n is the sample size and q hat is the sample proportion of failures.
Outlines
π Hypothesis Testing for Two Proportions
This paragraph introduces the topic of hypothesis testing for two proportions when the conditions for using the critical value method, p-value method, or confidence interval method are not met. The script explains the prerequisites for these methods, which include having two independent simple random samples with at least five successes and five failures in each. If these conditions are not satisfied, the normal distribution cannot be used as an approximation for the binomial distribution. The paragraph also touches on the limitations of sample types like voluntary response or convenience samples, which do not allow for generalizable results.
π Alternative Methods for Hypothesis Testing
The script discusses alternative methods for hypothesis testing when the requirement of having at least five successes and five failures in each sample is not met. Fisher's exact test is introduced as a more complex method that uses the binomial distribution without relying on the normal approximation. It is noted that technology can assist with the computations involved in this test, which is not detailed in the textbook. The paragraph also mentions the possibility of constructing a confidence interval using bootstrap resampling methods, which are covered in a different section of the book, and suggests looking up these methods for further information.
Mindmap
Keywords
π‘Testing Hypotheses
π‘Two Proportions
π‘Critical Value Method
π‘P-Value Method
π‘Confidence Interval Method
π‘Simple Random Samples
π‘Independence of Samples
π‘Binomial Distribution
π‘Fisher's Exact Test
π‘Bootstrap Resampling
π‘Population Proportions
Highlights
Lesson 9.1 discusses learning outcome number five, addressing testing hypotheses about two proportions when standard methods are not applicable.
Critical value, p-value, and confidence interval methods require specific conditions to be met for their use.
Two simple random samples are needed, ensuring independence from each other.
Independence means values from one population are not related to or paired with values from the other.
At least five successes and five failures in each sample are required for the normal approximation of the binomial distribution.
The condition n * p hat β₯ 5 and n * q hat β₯ 5 must be satisfied for the normal distribution approximation to be appropriate.
If the sample conditions are not met, the exact binomial distribution can still be used instead of the normal approximation.
Fisher's exact test is an alternative for hypothesis testing when the sample conditions are not met.
Fisher's exact test is more complex and computationally intensive but can be performed with technological assistance.
Bootstrap resampling methods can be used to construct a confidence interval for the difference between two population proportions.
Bootstrap methods are discussed in section 7.4 of the textbook for cases where the sample conditions are not met.
The video does not cover examples of Fisher's exact test or bootstrap methods but informs viewers of their existence and utility.
The next video will discuss testing hypotheses about two population means, not about two sample means.
The upcoming lesson will focus on the difference between population means rather than the difference between two population proportions.
The video aims to make viewers aware of the available options for hypothesis testing when standard requirements are not satisfied.
The transcript emphasizes the importance of understanding the prerequisites for applying statistical methods correctly.
The video provides guidance on where to find more information on alternative statistical methods in the textbook.
Transcripts
Browse More Related Video
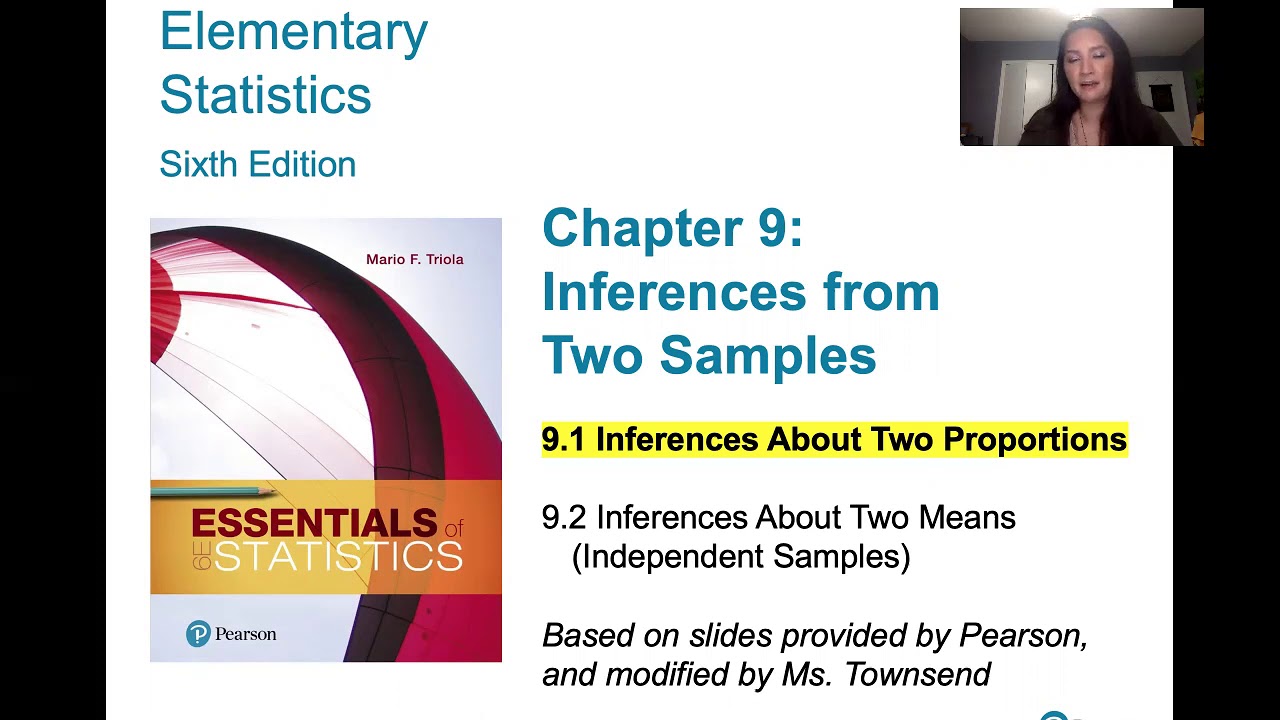
9.1.0 Two Proportions - Lesson Overview, Key Concepts, Learning Outcomes
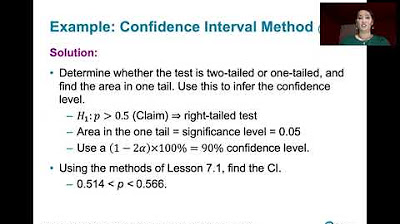
8.2.2 Testing a Claim About A Proportion - Confidence Interval Method, Comparison to Other Methods

Bootstrap Hypothesis Testing in Statistics with Example |Statistics Tutorial #35 |MarinStatsLectures

9.2.0 Two Means, Independent Samples - Lesson Overview, Learning Outcomes, Key Concepts
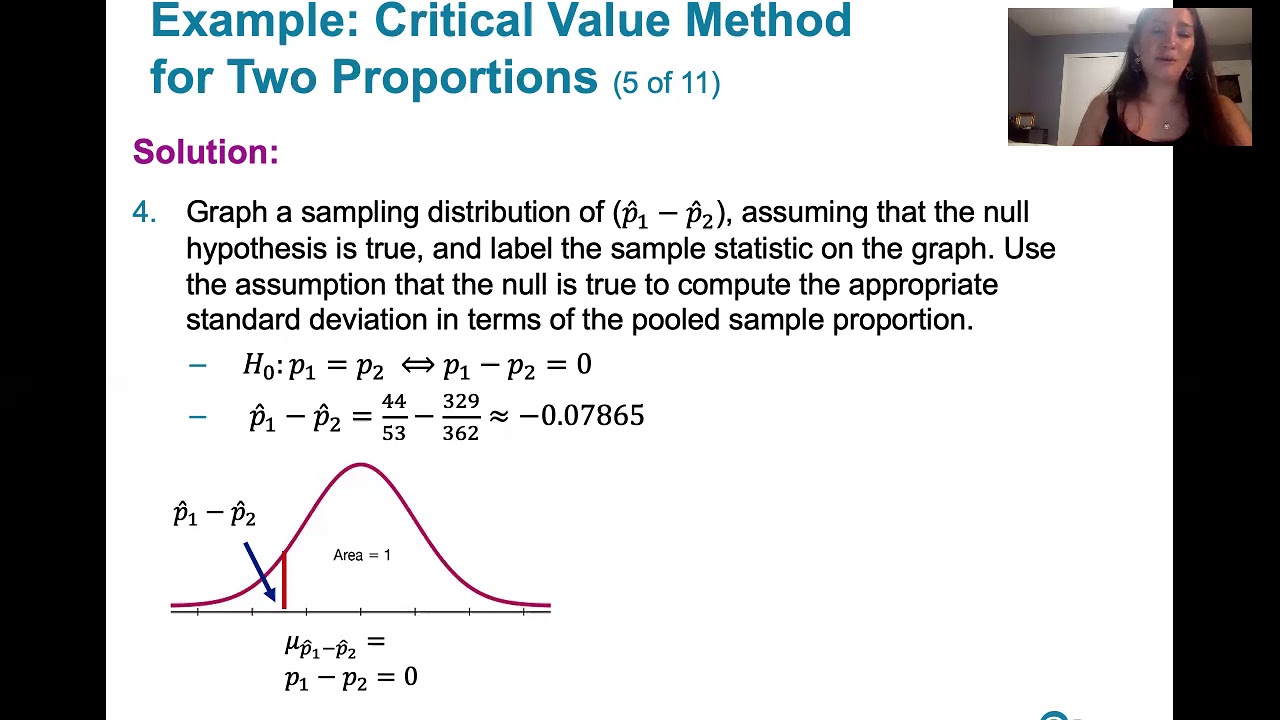
9.1.3 Two Proportions - Example, Critical Value Method, Normal Approximation
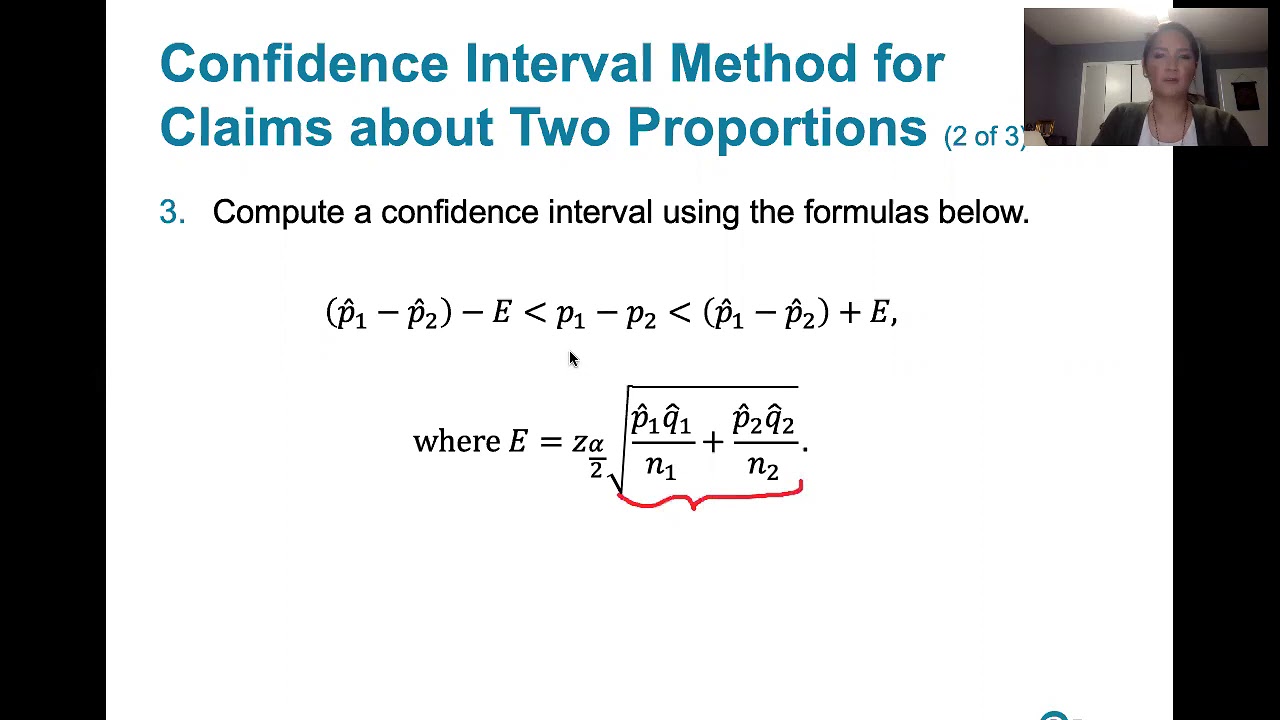
9.1.4 Two Proportions - Confidence Intervals for the Difference Between Two Proportions
5.0 / 5 (0 votes)
Thanks for rating: