Automatic Differentiation with TensorFlow โ Topic 64 of Machine Learning Foundations
TLDRThe video script introduces the concept of automatic differentiation using TensorFlow, contrasting it with the process in PyTorch. It begins by importing TensorFlow and initializing a scalar tensor with the value of 5. Unlike PyTorch, TensorFlow uses a GradientTape context to track gradients locally. The script demonstrates tracking gradients on a variable by using the 'watch' method within the GradientTape scope. The function y=x^2 is then defined and used to calculate the derivative dy/dx at x=5. The TensorFlow 'gradient' function is applied to find the slope, resulting in the same answer as PyTorch and manual calculation. The presenter expresses a preference for PyTorch's more intuitive and Pythonic approach. The video concludes by encouraging viewers to try TensorFlow as an exercise and hints at applying autodiff to implement a simple machine learning algorithm in future content.
Takeaways
- ๐ The video demonstrates the use of TensorFlow for automatic differentiation.
- ๐ TensorFlow is used to calculate the derivative of y with respect to x for the function y = x^2 at x = 5.
- ๐ฆ The process begins by importing the TensorFlow library and initializing a scalar tensor with the value 5.
- ๐ TensorFlow uses a GradientTape context to track gradients, unlike PyTorch which tracks them globally.
- ๐ Within the GradientTape, the `watch_method` is used to specify which variables to track gradients for.
- ๐งฎ The forward pass of the x tensor is tracked, and it is passed into the function y = x^2.
- ๐ The `gradient` method in TensorFlow is used to find the slope (derivative) between two variables.
- ๐ข The result of the derivative calculation is 10, which matches the manual calculation and the result from PyTorch.
- ๐ค The presenter finds PyTorch more intuitive and Pythonic compared to TensorFlow.
- ๐ The video suggests using PyTorch for automatic differentiation in the machine learning foundation series.
- ๐ TensorFlow is recommended for exercises to provide practice in differentiating using alternative methods.
- ๐ The next step is to apply automatic differentiation to implement a simple machine learning algorithm, specifically a regression line.
Q & A
What is the main focus of the video?
-The video focuses on performing automatic differentiation using the TensorFlow library.
What is the function used in the video to demonstrate automatic differentiation?
-The function used is y = x squared.
How does TensorFlow handle gradient tracking differently from PyTorch?
-In TensorFlow, gradient tracking is done within a gradient tape context, whereas in PyTorch, gradients are tracked globally.
What is the name of the TensorFlow method used to track gradients?
-The TensorFlow method used to track gradients is called 'gradient tape'.
How is the gradient tape method typically initialized in TensorFlow?
-The gradient tape method is typically initialized with the statement 'with tf.GradientTape() as t:'.
What method is used in TensorFlow to specify which variables to track gradients on?
-The 'watch' method is used to specify variables for gradient tracking in TensorFlow.
What is the result of the derivative calculation in the video?
-The result of the derivative calculation is 10, which is the slope of the curve at x equals 5.
How does the speaker feel about the TensorFlow syntax compared to PyTorch?
-The speaker finds PyTorch to be more intuitive and Pythonic than TensorFlow.
What library will the speaker primarily use for automatic differentiation in the rest of the machine learning foundation series?
-The speaker will primarily use PyTorch for automatic differentiation in the rest of the series.
What is the next topic the speaker plans to cover in the machine learning foundation series?
-The next topic is implementing a simple machine learning algorithm using autodiff to fit a regression line.
What is the method used in TensorFlow to calculate the gradient of one variable with respect to another?
-The 'gradient' method is used in TensorFlow to calculate the gradient of one variable with respect to another.
How does the speaker suggest viewers practice their understanding of TensorFlow's automatic differentiation?
-The speaker suggests viewers practice by trying to perform the automatic differentiation in TensorFlow as an exercise, after learning it in PyTorch.
Outlines
๐ค Introduction to Automatic Differentiation with TensorFlow
This paragraph introduces the use of TensorFlow for automatic differentiation, contrasting it with previous work done using PyTorch. The video begins by importing TensorFlow and initializing a scalar tensor with the value of 5. Unlike PyTorch, TensorFlow uses a GradientTape context to track gradients locally. The 'watch' method is used to specify which variables to track gradients for, analogous to PyTorch's 'requires_grad'. The forward pass is then tracked on the 'x' tensor, which is input into the function y = x^2. To calculate the derivative, TensorFlow's 'gradient' method is used, providing the same result as PyTorch and manual calculations, which is 10 for dy/dx at x=5. The presenter expresses a preference for PyTorch's intuitive and Pythonic approach over TensorFlow's.
Mindmap
Keywords
๐กTensorFlow
๐กAutomatic Differentiation
๐กGradient Tape
๐กScalar Tensor
๐กWatch Method
๐กGradient Method
๐กPyTorch
๐กMachine Learning Foundation Series
๐กRegression Line
๐กPythonic
๐กComputational Graph
Highlights
The video demonstrates the use of TensorFlow for automatic differentiation.
A comparison is made between TensorFlow and PyTorch for calculating the derivative of y with respect to x.
TensorFlow uses a gradient tape to track gradients, unlike PyTorch which tracks them globally.
The gradient tape is initiated with the statement 'with tf.GradientTape() as t:', a TensorFlow-specific approach.
In TensorFlow, the 'watch' method is used to specify variables to track gradients on.
The forward pass is tracked on the x tensor within the gradient tape context.
The function y = x^2 is used as an example for differentiation.
The 'tf.GradientTape.gradient()' method is used to compute the gradient of y with respect to x.
The result of the differentiation in TensorFlow matches the manual calculation and PyTorch's result, yielding 10.
The presenter expresses a preference for PyTorch's more intuitive and Pythonic approach over TensorFlow.
The video suggests using PyTorch for the rest of the machine learning foundation series.
TensorFlow's method is described as less intuitive and less enjoyable compared to PyTorch.
The video encourages viewers to try the TensorFlow exercise as a challenge after learning it in PyTorch.
The next video will focus on applying autodiff to implement a simple machine learning algorithm.
A simple regression line fitting will be the subject of the next tutorial, using PyTorch.
The presenter emphasizes the importance of understanding the theory before jumping into practical implementations.
The video concludes with a teaser for the upcoming regression tutorial in the machine learning series.
Transcripts
Browse More Related Video
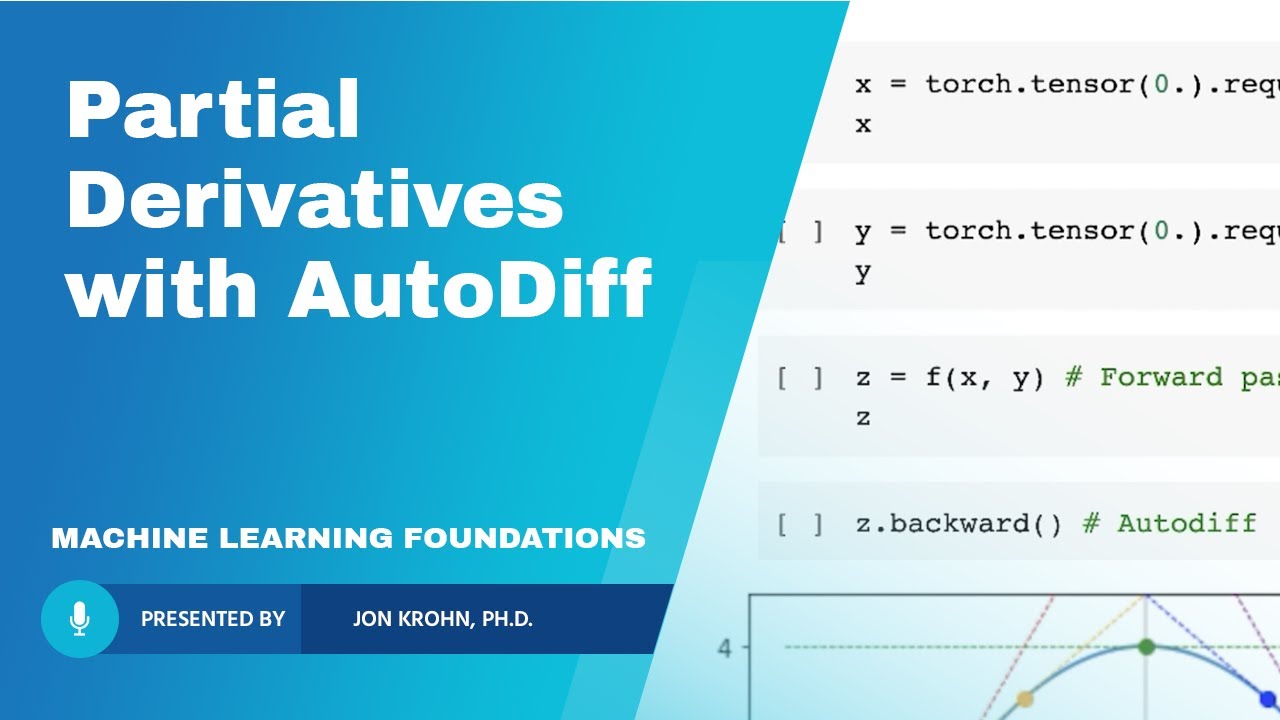
Calculating Partial Derivatives with PyTorch AutoDiff โ Topic 69 of Machine Learning Foundations
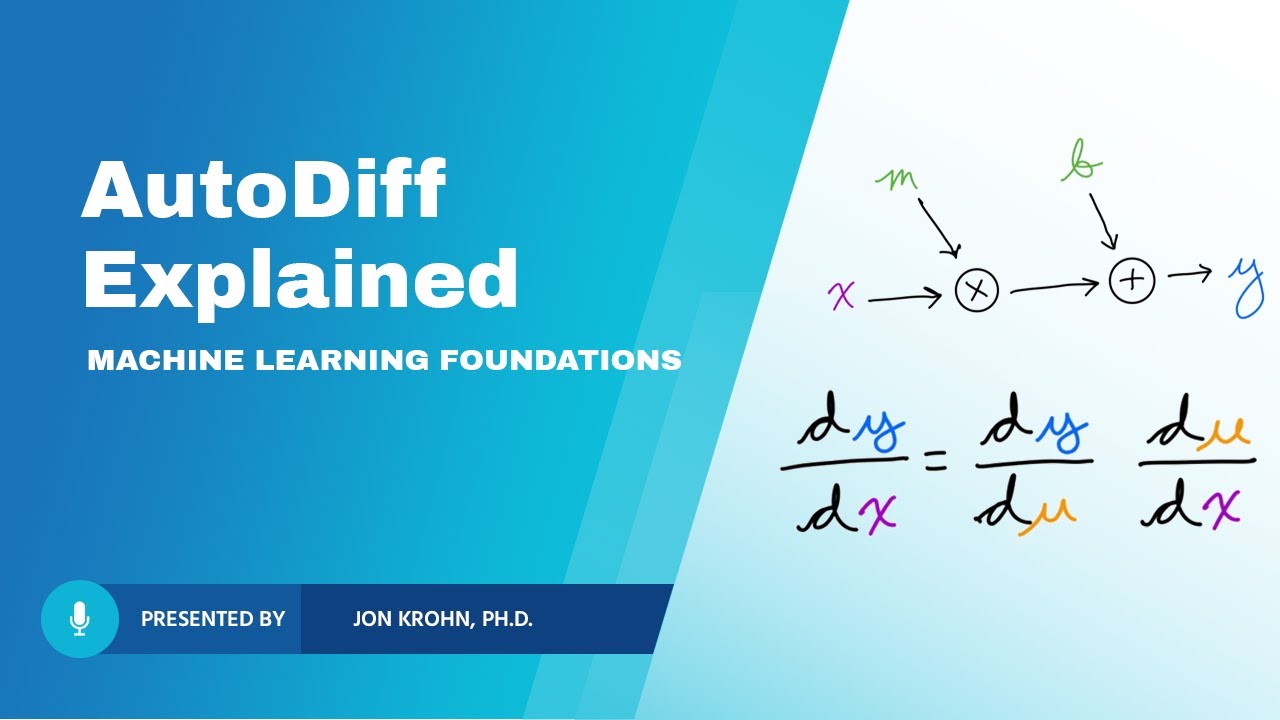
What Automatic Differentiation Is โ Topic 62 of Machine Learning Foundations
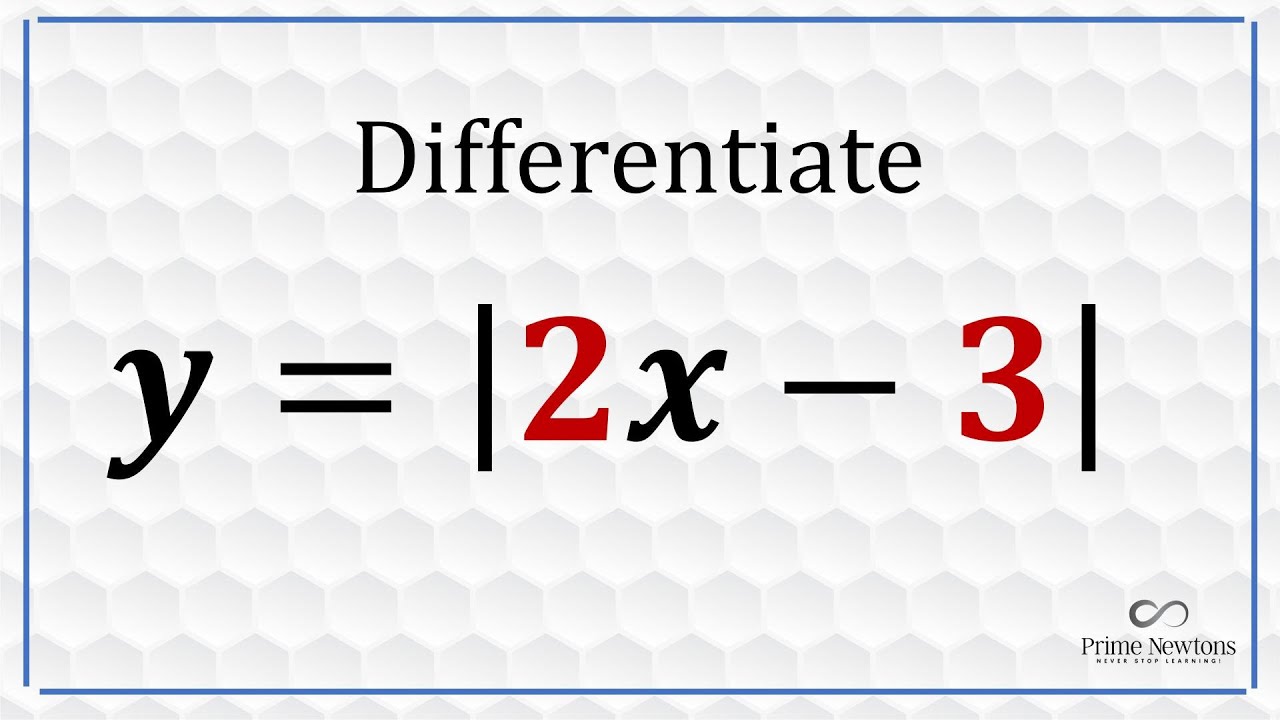
Derivative of absolute value function
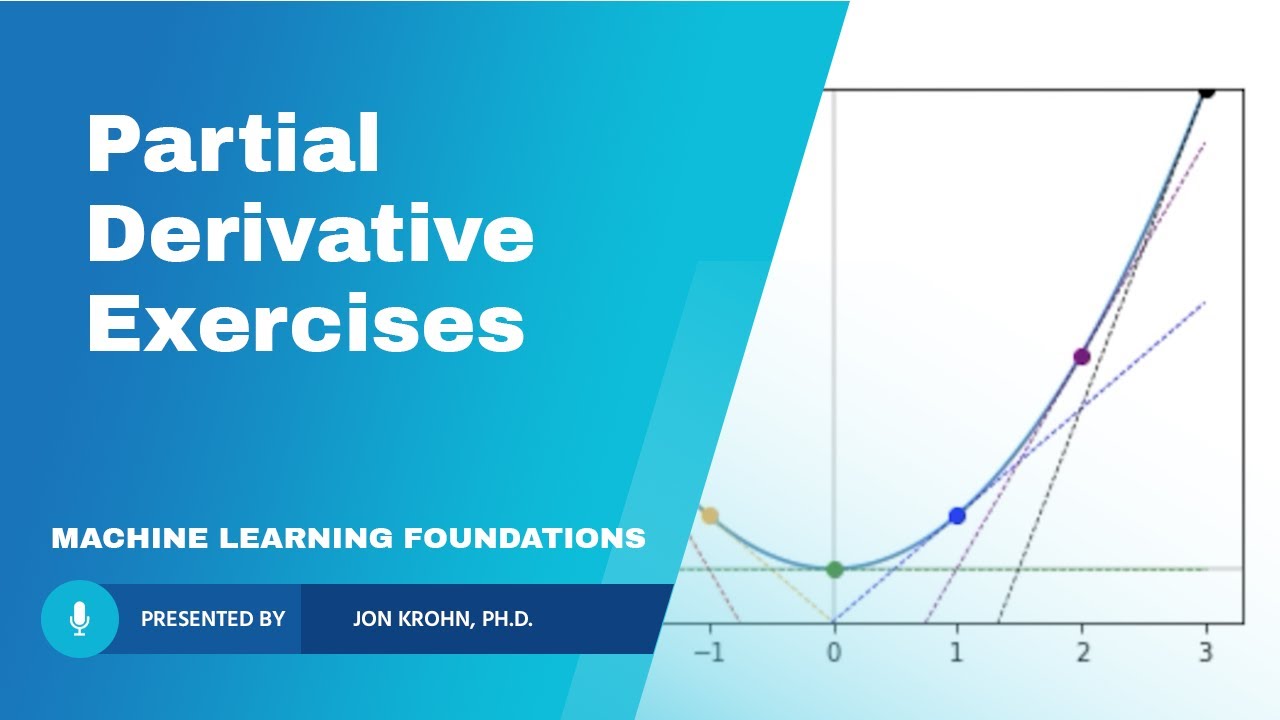
Partial Derivative Exercises โ Topic 68 of Machine Learning Foundations

Implicit Differentiation - Find The First & Second Derivatives
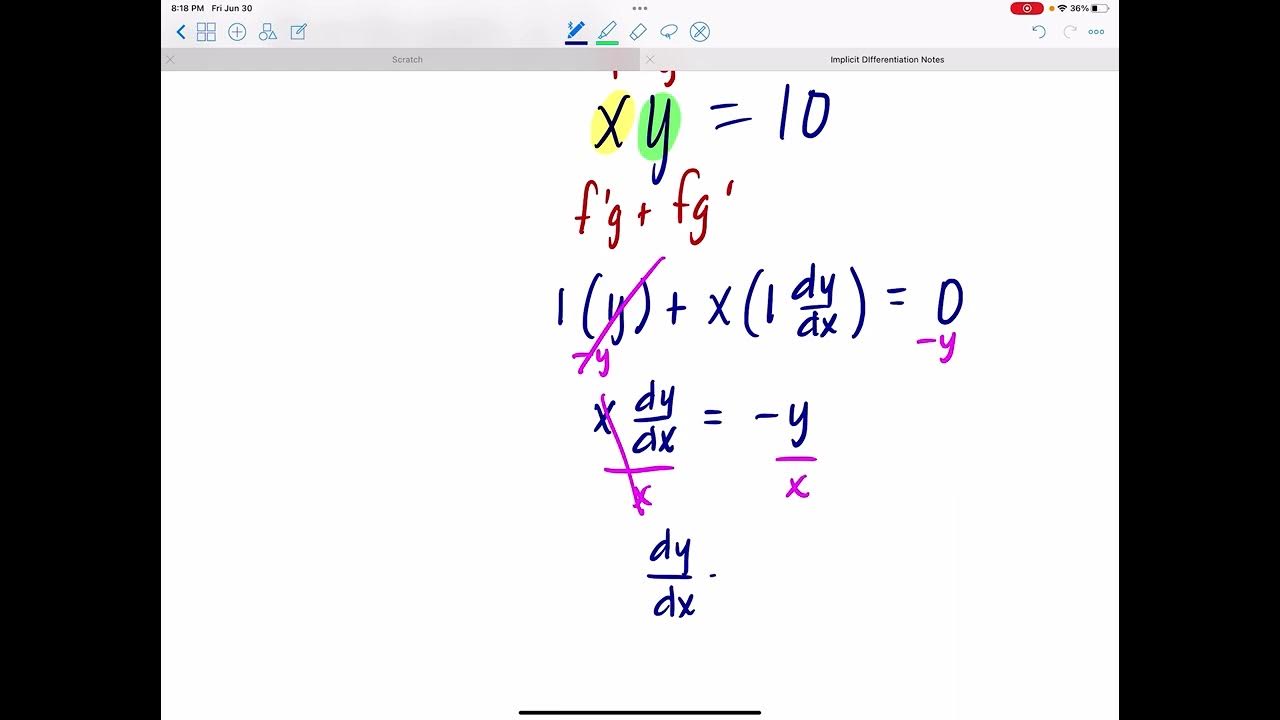
Implicit Differentiation
5.0 / 5 (0 votes)
Thanks for rating: