Understanding Statistical Significance - Statistics help
TLDRDr. Mike from Statistics Learning Center and Creative Mess explains the concept of statistical significance, which is often misunderstood. In everyday language, 'significant' can mean 'big' or 'important,' but in statistics, it has a different meaning. Statistical significance indicates that the effect observed in a sample likely exists in the population, not necessarily that it is large or important. A common measure for this is the p-value, where a value less than 0.05 typically indicates statistical significance. However, with a very large sample, even a small effect can be statistically significant but may not be practically meaningful. Conversely, a small sample size may not show statistical significance unless the effect is very large. Dr. Mike advises using the term 'statistically significant' in reports to avoid confusion and suggests alternative words like 'notable,' 'remarkable,' 'compelling,' 'measurable,' or 'large' for clarity.
Takeaways
- π Statistical significance is a term with a specific meaning in statistics, different from its everyday use.
- π In everyday language, 'significant' can mean 'big' or 'important', but in statistics, it refers to the likelihood that an observed effect is not due to chance.
- π The word 'significant' in statistical analysis does not necessarily imply that the effect is large or important.
- βοΈ A statistically significant result means there is evidence from a sample that the effect exists in the population.
- π§ Understanding the concepts of 'sample' and 'population' is crucial to grasp the idea of statistical significance.
- π A p-value is used to determine statistical significance; typically, a p-value less than 0.05 indicates significance.
- β Rejecting the null hypothesis (which states there is no effect) when the p-value is low suggests that there is an effect in the population.
- π’ With a very large sample, even a small effect can be statistically significant due to the sample's good representation of the population.
- π Conversely, with a very small sample, it's unlikely to find statistical significance unless the effect is remarkably large.
- π When reporting statistical findings, use the term 'statistically significant' instead of just 'significant' to avoid confusion.
- π In statistical reports, it's better to use terms like 'notable', 'remarkable', 'compelling', 'measurable', or 'large' to describe the size or importance of an effect.
Q & A
What does the term 'statistical significance' refer to in the context of statistical analysis?
-In statistical analysis, 'statistical significance' refers to evidence from a sample that suggests an effect or relationship exists in the population. It does not necessarily imply that the effect is large or important, which is a common misconception.
What is the everyday language meaning of the word 'significant'?
-In everyday language, 'significant' can mean 'big' or 'important'. For example, saying 'my earnings are significantly more this year' implies a large difference, while 'a significant increase in sea temperature' indicates an important change, which might be small in magnitude.
Why is it problematic when news reports use the term 'significant' without clarification?
-It's problematic because the general public may interpret 'significant' to mean 'large and important', whereas in statistics, it only indicates that the result is unlikely to have occurred by chance. This can lead to misunderstandings about the actual size and importance of the effect.
What is a p-value and how is it used in determining statistical significance?
-A p-value is a statistical measure that indicates the strength of the evidence against the null hypothesis. If the p-value is less than a predetermined threshold, typically 0.05, the result is considered statistically significant, suggesting that the effect is not due to chance.
What is the null hypothesis in statistical testing?
-The null hypothesis is a statement that there is no effect or no relationship between variables being studied. It is used as a basis for statistical testing, and if the p-value is low, the null hypothesis is rejected, indicating evidence of an effect.
Why might a large sample size make a result statistically significant even if the effect is small?
-A large sample size provides a better representation of the population, increasing the likelihood that any observed effect is not due to random chance. Even a small effect can become statistically significant because the sample size amplifies the visibility of the effect.
What is the potential issue with a very small sample size in determining statistical significance?
-A very small sample size may not capture the variability present in the population well. As a result, it is less likely to show a statistically significant result unless the effect is remarkably large. Small sample sizes can lead to unreliable conclusions about the population.
What are some alternative terms to 'significant' that can be used when writing up a statistical analysis to avoid confusion?
-Instead of using 'significant', which can be misleading, one can use terms like 'statistically significant', 'notable', 'remarkable', 'compelling', 'measurable', or 'large' to convey the magnitude and importance of the findings more accurately.
What is the importance of understanding the concepts of 'sample' and 'population' in the context of statistical significance?
-Understanding 'sample' and 'population' is crucial because statistical significance is about inferring the existence of an effect in the population based on the evidence from the sample. Without this understanding, the interpretation of statistical results can be flawed.
What does it mean to 'reject the null hypothesis' in statistical terms?
-Rejecting the null hypothesis means that the data provides enough evidence to suggest that the default assumption of no effect or no relationship is unlikely to be true. It is a conclusion drawn when the p-value is below the significance level, often 0.05.
Why is it important to distinguish between statistical significance and practical significance?
-It's important because a result can be statistically significant but have a negligible effect size, making it of little practical importance. Distinguishing between the two helps avoid overestimating the impact of findings and makes research more relevant to real-world applications.
What are confidence intervals and how do they relate to statistical significance?
-Confidence intervals provide a range within which the true population parameter is estimated to lie, with a certain level of confidence. They are related to statistical significance in that they can give an indication of the effect size and its precision, which are important considerations in determining the practical significance of a result.
Outlines
π Understanding Statistical Significance
Dr. Mike from the Statistics Learning Center and Creative Mess introduces the concept of statistical significance, explaining the difference between its everyday meanings ('big' or 'important') and its specific use in statistical analysis. He clarifies that statistical significance indicates evidence from a sample that an effect exists in the population, which is often misunderstood as being 'big' or 'important'. The video emphasizes the importance of the p-value, which is used to determine if the observed effect in a sample is indicative of a real effect in the population or could have occurred by chance. A p-value less than 0.05 is typically considered statistically significant, leading to the rejection of the null hypothesis. Dr. Mike also discusses the impact of sample size on statistical significance, noting that a large sample size can render even small effects statistically significant, while a very small sample may not show significance unless the effect is very large. He advises on the appropriate use of the term 'statistically significant' in reports and suggests alternative words like 'notable', 'remarkable', 'compelling', 'measurable', or 'large' to convey the magnitude of the effect.
Mindmap
Keywords
π‘Statistical Significance
π‘P-value
π‘Sample
π‘Population
π‘Null Hypothesis
π‘Effect Size
π‘Sampling Error
π‘Inference
π‘Confidence Intervals
π‘Large Sample
π‘Small Sample
π‘Language and Context
Highlights
Statistical significance is often misunderstood due to its different meanings in everyday language compared to statistical language.
In everyday language, 'significant' can refer to something being big or important.
Statistically, 'significant' refers to evidence from a sample that an effect exists in the population.
Understanding the concepts of 'sample' and 'population' is crucial to grasp statistical significance.
A p-value is used to determine statistical significance, with a common threshold of less than 0.05.
A statistically significant result does not necessarily mean the effect is large or important.
Large sample sizes can make even small effects statistically significant due to better representation of the population.
Small sample sizes require a large effect to be considered statistically significant.
The misuse of the term 'significant' in news reports can lead to confusion about the actual size and importance of an effect.
When writing up an analysis, it's recommended to use 'statistically significant' instead of just 'significant' to avoid ambiguity.
Using 'significant' to mean big or important in a statistical report can be misleading.
Alternative terms such as 'notable', 'remarkable', 'compelling', 'measurable', or 'large' can be used for clarity.
The video emphasizes the importance of correctly interpreting and reporting statistical significance.
Dr. Mike from Statistics Learning Center explains the concept in an accessible manner.
Viewers are encouraged to like, share, subscribe, and turn on notifications for more informative videos.
Understanding statistical significance is a fundamental concept in statistical analysis.
The video aims to clarify misconceptions about the term 'significant' in a statistical context.
The importance of sample size in determining statistical significance is discussed.
Transcripts
Browse More Related Video
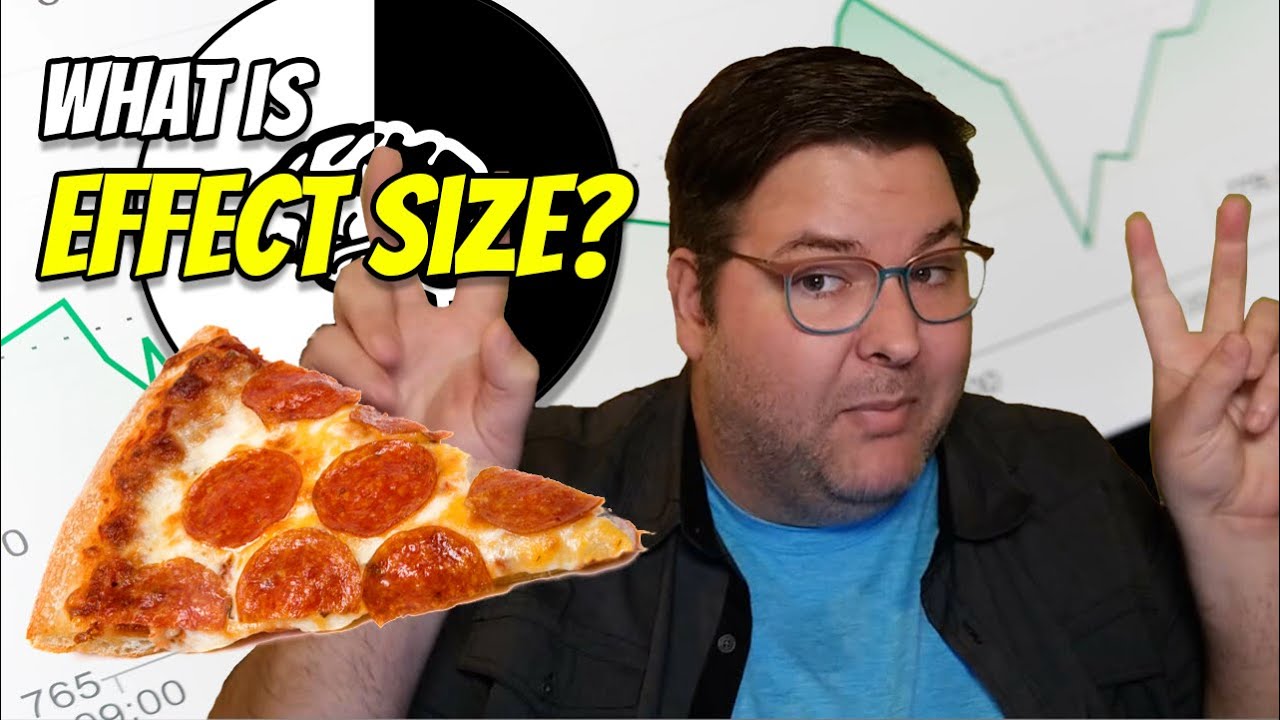
What is Effect Size? Statistical significance vs. Practical significance
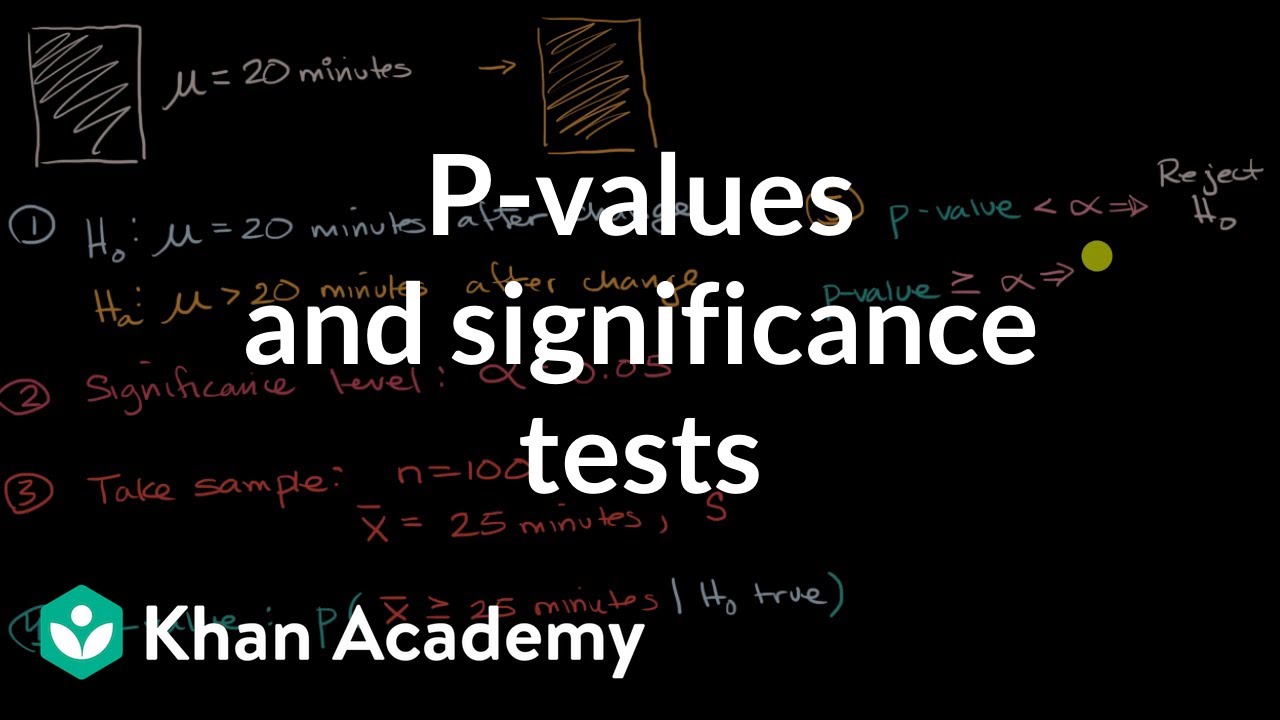
P-values and significance tests | AP Statistics | Khan Academy

Statistical vs Practical Significance Compared

P value versus effect size
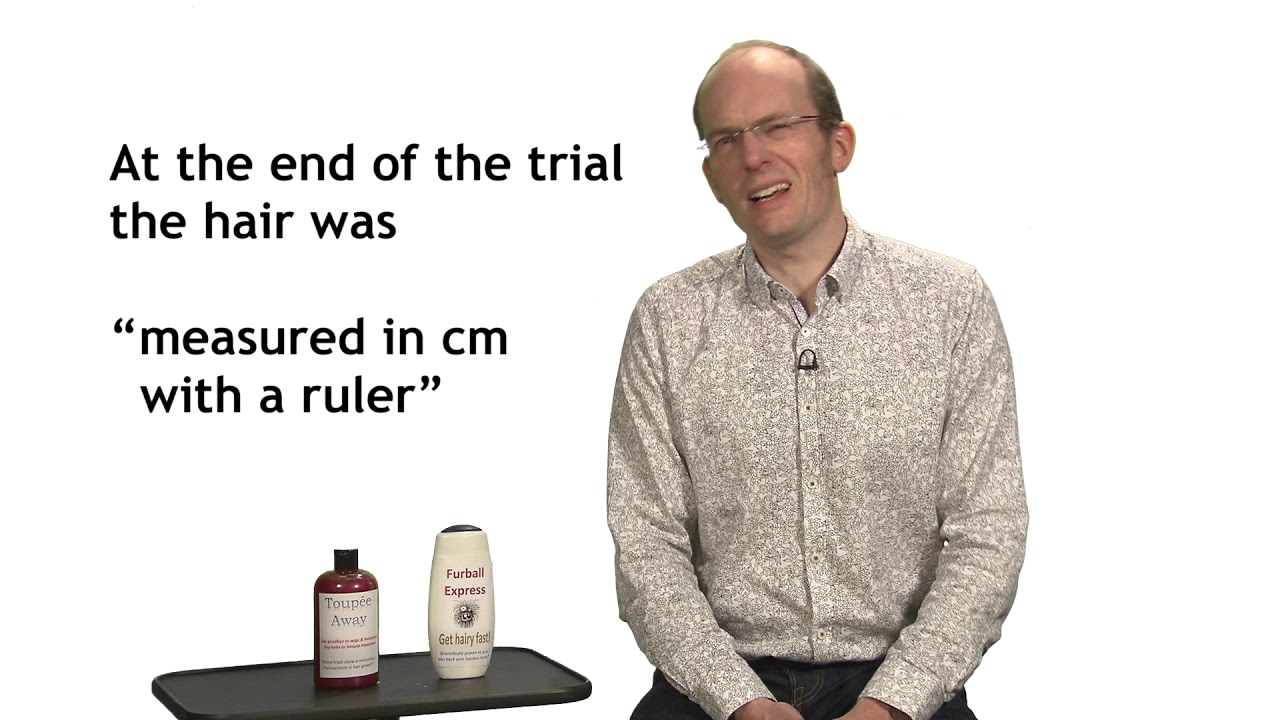
What do my results mean Effect size is not the same as statistical significance. With Tom Reader.
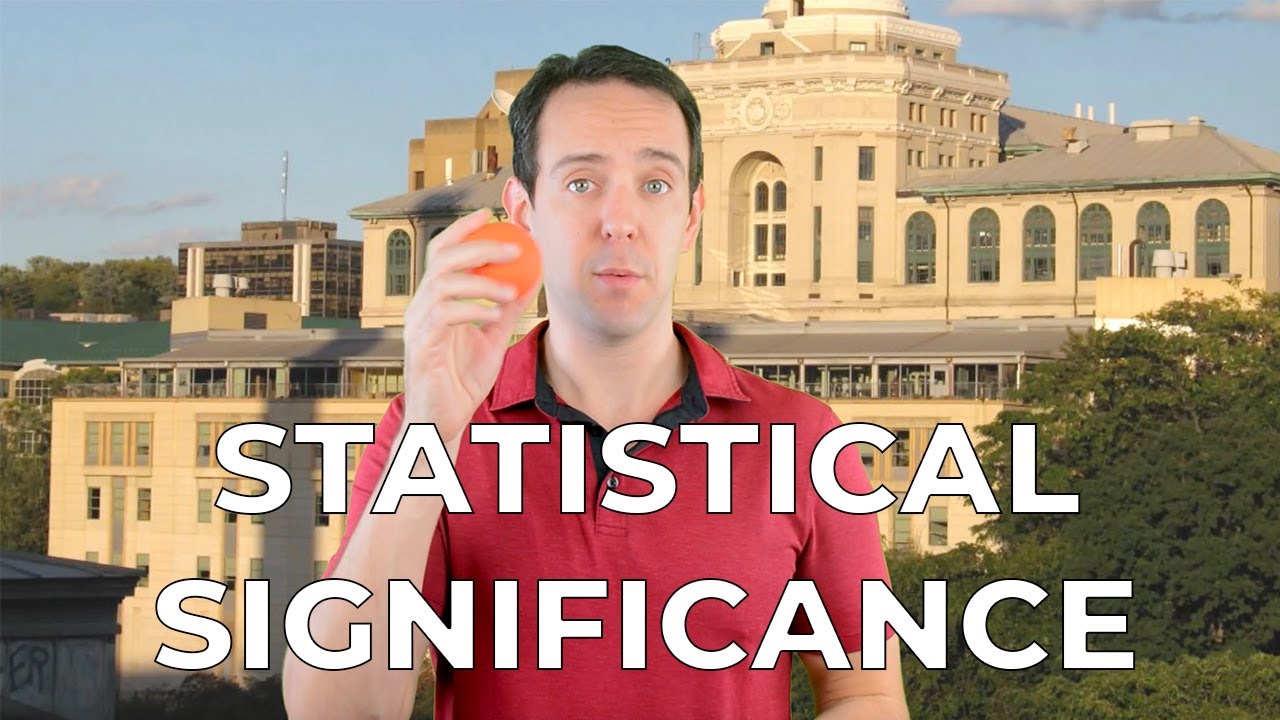
Statistical Significance and p-Values Explained Intuitively
5.0 / 5 (0 votes)
Thanks for rating: