Statistical vs Practical Significance Compared
TLDRIn this insightful video, Jeff Gallick from 'Data Demystified' challenges the conventional reliance on statistical significance as the sole determinant of a study's validity. He introduces the concept of 'meaningful significance,' emphasizing that while statistical significance establishes whether an observed effect is likely due to chance, it doesn't necessarily convey the practical importance of the result. Gallick illustrates this with examples from medicine, economics, and education, where interventions might show statistically significant benefits but may not be worth the investment if the impact is minimal. He encourages viewers to consider both statistical and meaningful significance when evaluating the value of a study, policy, or intervention. The video is a call to action for a more nuanced understanding of data, advocating for critical thinking that goes beyond statistical thresholds to assess the true impact and practical implications of findings.
Takeaways
- ๐ **Statistical Significance:** It's a common measure used to determine if an observed result is likely due to chance or a real effect.
- ๐ **Effectiveness vs. Significance:** A new drug or policy needs to show statistical significance to be considered viable, but that doesn't always mean it has meaningful impact.
- ๐ **Meaningful Significance:** Just because a result is statistically significant doesn't mean it's practically important or worth the investment.
- ๐ค **Necessary but Not Sufficient:** Statistical significance is necessary to rule out chance, but it's not enough to justify implementing an intervention.
- ๐ **Effect Size:** This measures the magnitude of an effect and is crucial in determining how impactful an intervention is.
- ๐ฐ **Cost-Benefit Analysis:** Consider the costs versus benefits when evaluating the meaningfulness of an intervention, such as a vaccine or policy change.
- ๐ **Small but Significant:** An intervention can be statistically significant with a small effect size, which may not justify its implementation.
- โ๏ธ **Trade-offs:** Decision-makers should weigh the statistical significance against the practical implications and costs.
- ๐งฎ **Educational Example:** In education, a small improvement in test scores might not justify a large-scale change in teaching methods.
- ๐ค **Policy Decisions:** Policy interventions should not only be statistically proven but also have a meaningful effect size to be considered successful.
- โ **Critical Evaluation:** When assessing results, ask if they are statistically significant and if they are meaningful in a real-world context.
Q & A
What is considered the gold standard in various disciplines to show that something is true?
-Statistical significance is often considered the gold standard in a variety of disciplines to demonstrate that something is true.
What is the primary purpose of statistical significance in the context of a new drug development?
-Statistical significance is used to show that a new drug is effective enough to be considered viable.
What is the difference between statistical significance and meaningful significance?
-Statistical significance determines if a result is likely due to chance or not, while meaningful significance assesses the practical importance or impact of that result.
Why might a statistically significant result not be worth implementing?
-A statistically significant result might not be worth implementing if the effect size is very small and the costs or resources required to implement it are high.
What is an effect size and why is it important?
-Effect size is a measure that indicates the magnitude of an intervention's impact. It is important because it tells us how impactful an intervention is, not just whether it works.
How can effect sizes be used in decision-making?
-Effect sizes can be used to make trade-offs, such as deciding whether to proceed with the distribution of a vaccine, invest in a policy intervention, or change educational strategies.
What is the necessary but not sufficient condition for a result to be considered statistically significant?
-The necessary but not sufficient condition for statistical significance is that the observed difference or relationship between variables is not likely due to chance.
Why is it important to consider both statistical and meaningful significance when evaluating a policy decision?
-Considering both types of significance is important because it helps to determine not just whether a policy will work, but also how effective it is and whether the benefits outweigh the costs.
How might a small but statistically significant increase in wages due to free college tuition be evaluated?
-The increase would be evaluated based on whether the cost of implementing free college tuition is justified by the magnitude of the wage increase, even if it is statistically significant.
null
-null
What is the role of statistical significance in the context of a medical vaccine example?
-Statistical significance in a medical vaccine example helps to confirm that the vaccine's effectiveness in reducing infection rates is not due to chance, but it does not inform about the magnitude of the vaccine's impact.
How can one intuitively compare different types of interventions using effect sizes?
-One can compare different interventions by looking at the effect sizes in a common metric, such as percentage terms, which allows for a direct comparison of the magnitude of impact across different interventions.
What are common language effect sizes and why are they useful?
-Common language effect sizes are a way to express the magnitude of an effect in terms that are easily understood by non-experts. They are useful for communicating the practical significance of results to a broader audience.
Why might a statistically significant result with a tiny difference across groups still be important?
-A statistically significant result with a tiny difference can be important if the context or the condition being measured is such that even a small change has significant practical implications or if the result is part of a cumulative effect over time.
Outlines
๐ Understanding Statistical Significance vs. Meaningful Significance
The first paragraph discusses the concept of statistical significance, which is often used as a benchmark to validate findings in various fields such as pharmaceuticals, policy interventions, and marketing. However, it emphasizes that statistical significance does not necessarily equate to meaningful significance. The speaker, Jeff Gallick, introduces himself and his mission to help viewers navigate the data-rich world. He proposes an intuitive framework for evaluating results based not just on their statistical validity but also on their practical importance or 'effect size,' which measures the magnitude of an intervention's impact. The paragraph also uses the example of a vaccine to illustrate the difference between statistical and meaningful significance, highlighting that even a small reduction in disease risk can be statistically significant but may not be substantial enough to justify the costs associated with the vaccine.
๐ The Importance of Considering Effect Size in Decision Making
The second paragraph expands on the idea that while statistical significance is crucial to establish that a result is not due to chance, it is equally important to consider the effect size to determine the practical implications of an intervention. It uses examples from economics and education to illustrate how a statistically significant result might not translate into a meaningful impact if the effect size is small. The speaker suggests a framework for critical assessment that involves two key questions: whether the result is statistically significant and whether it is meaningful. The paragraph concludes by encouraging viewers to look beyond statistical significance to make informed decisions about the implementation of policies or interventions. It also invites viewers to engage with the content by liking, subscribing, and enabling notifications for new content.
Mindmap
Keywords
๐กStatistical Significance
๐กMeaningful Significance
๐กEffect Size
๐กData Analysis
๐กPolicy Intervention
๐กVaccine Efficacy
๐กRisk Reduction
๐กManufacturing and Distribution
๐กResearch and Development (R&D)
๐กEducational Intervention
๐กTrade-offs
Highlights
Statistical significance is often considered the gold standard in various disciplines to show that something is true.
Statistical significance is a minimum requirement to show the efficacy of a new drug, the effectiveness of a policy intervention, or the choice between different advertisements.
Statistical significance fails to consider meaningful significance, which is the importance of a result beyond just being true.
The speaker, Jeff Gallick, aims to provide an intuitive framework for evaluating the meaningfulness of a result.
Statistical significance determines if an observed difference is likely due to chance or something else.
Having enough data and confidence allows one to claim statistical significance, suggesting the results aren't due to luck.
Effect size measures the impact of an intervention, not just its effectiveness.
Effect sizes are crucial for making trade-offs, such as whether to distribute a vaccine or invest in further research.
Statistical significance can be present even with a very small effect size, such as a 1% reduction in infection rates.
Meaningful significance asks if a statistically significant result is worth the investment or action.
In economics, the cost versus benefit analysis is crucial, and a statistically significant result must also be meaningful to be worth implementing.
Policy interventions should be evaluated not just on their statistical significance but also on their effect size and practical impact.
The framework for assessing statistical results involves asking if the result is statistically significant and if it is meaningful.
Critical evaluation of any policy decision should consider both the effectiveness and the extent of its impact.
Statistical significance can lead to overlooking the practical significance and necessary trade-offs of an intervention.
The video encourages viewers to think beyond statistical significance to judge whether a result is truly meaningful.
The speaker acknowledges that some topics, like computing effect sizes and comparisons between interventions, have been glossed over for the sake of focusing on the core intuition.
The video concludes by encouraging viewers to engage with the content, subscribe to the channel, and stay updated with new content.
Transcripts
Browse More Related Video
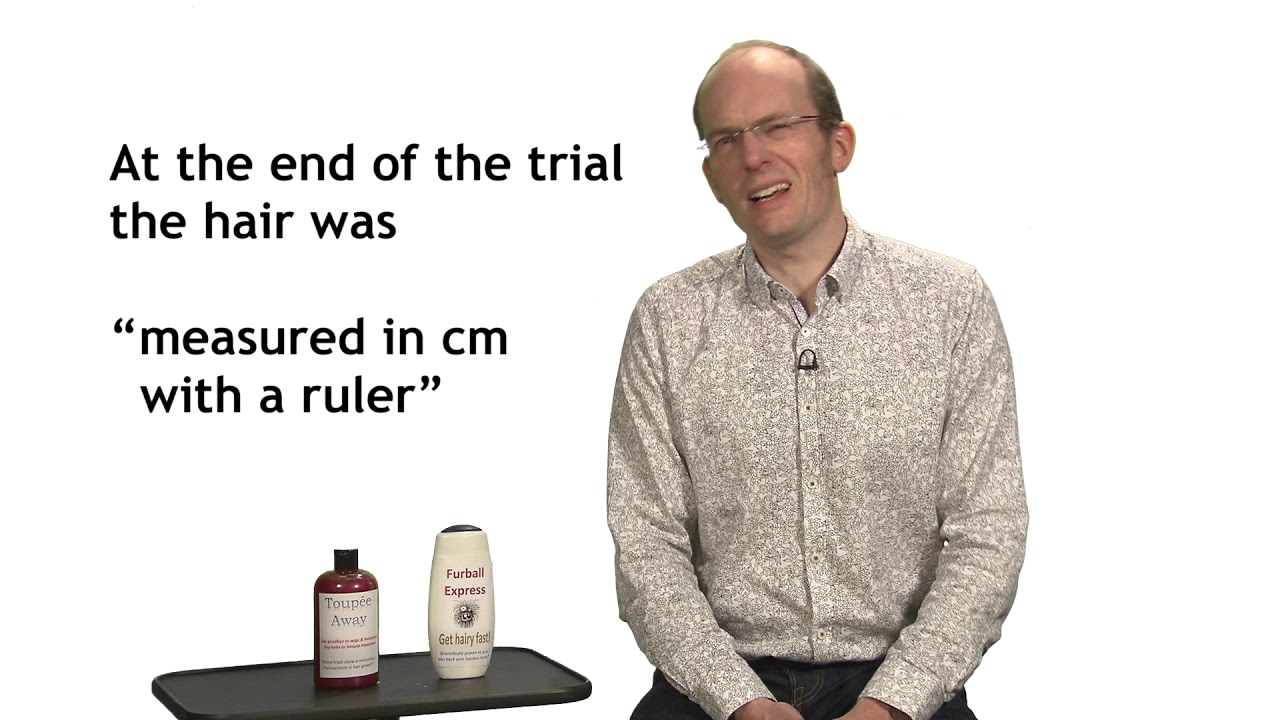
What do my results mean Effect size is not the same as statistical significance. With Tom Reader.
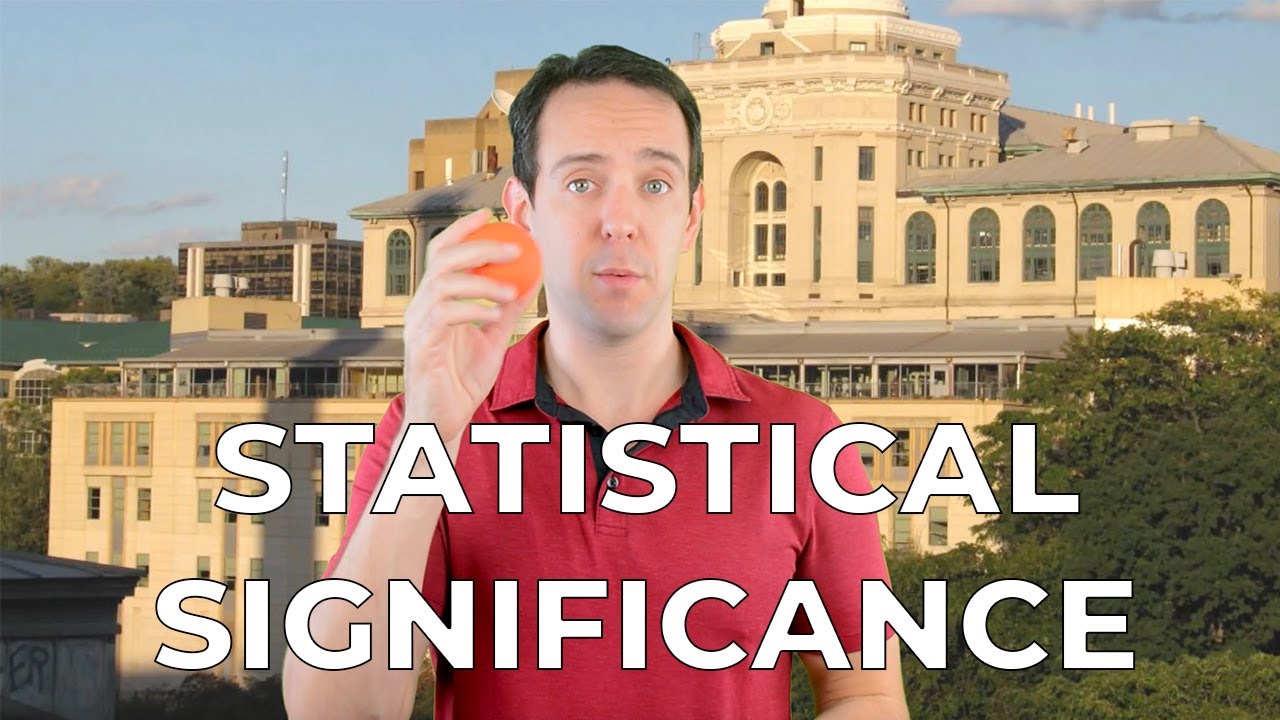
Statistical Significance and p-Values Explained Intuitively

Understanding Statistical Significance - Statistics help
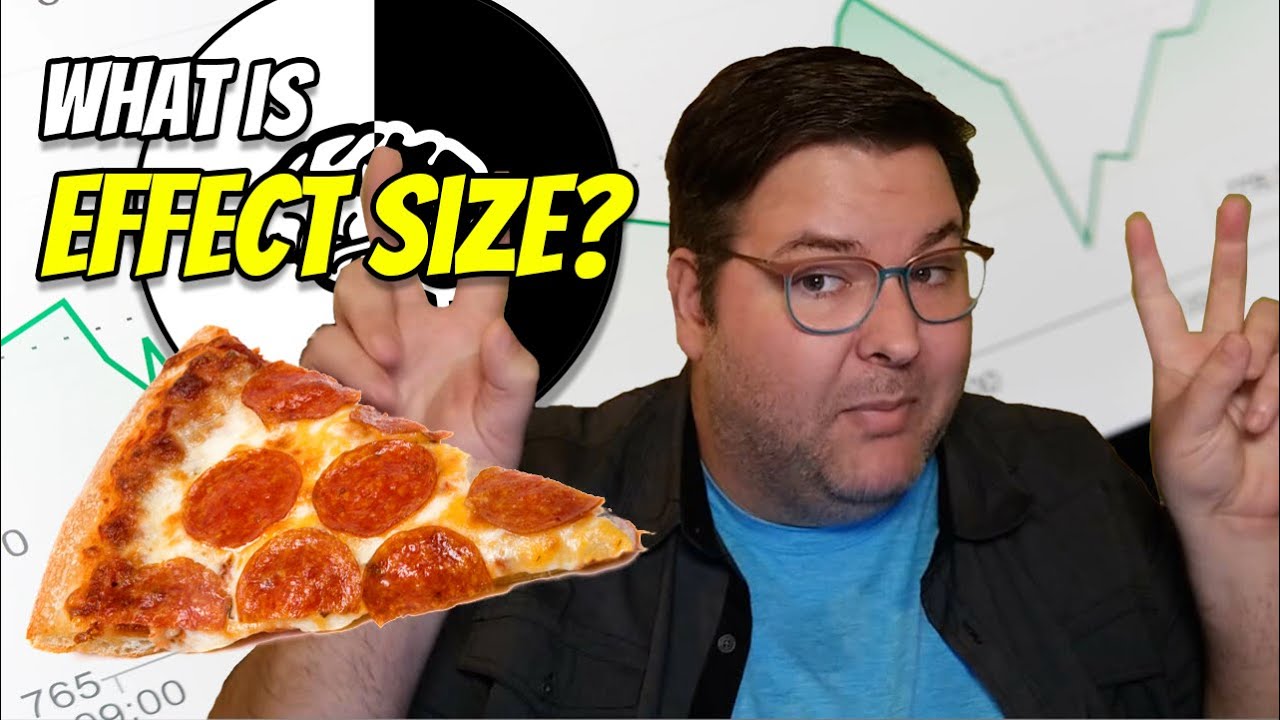
What is Effect Size? Statistical significance vs. Practical significance

P value versus effect size
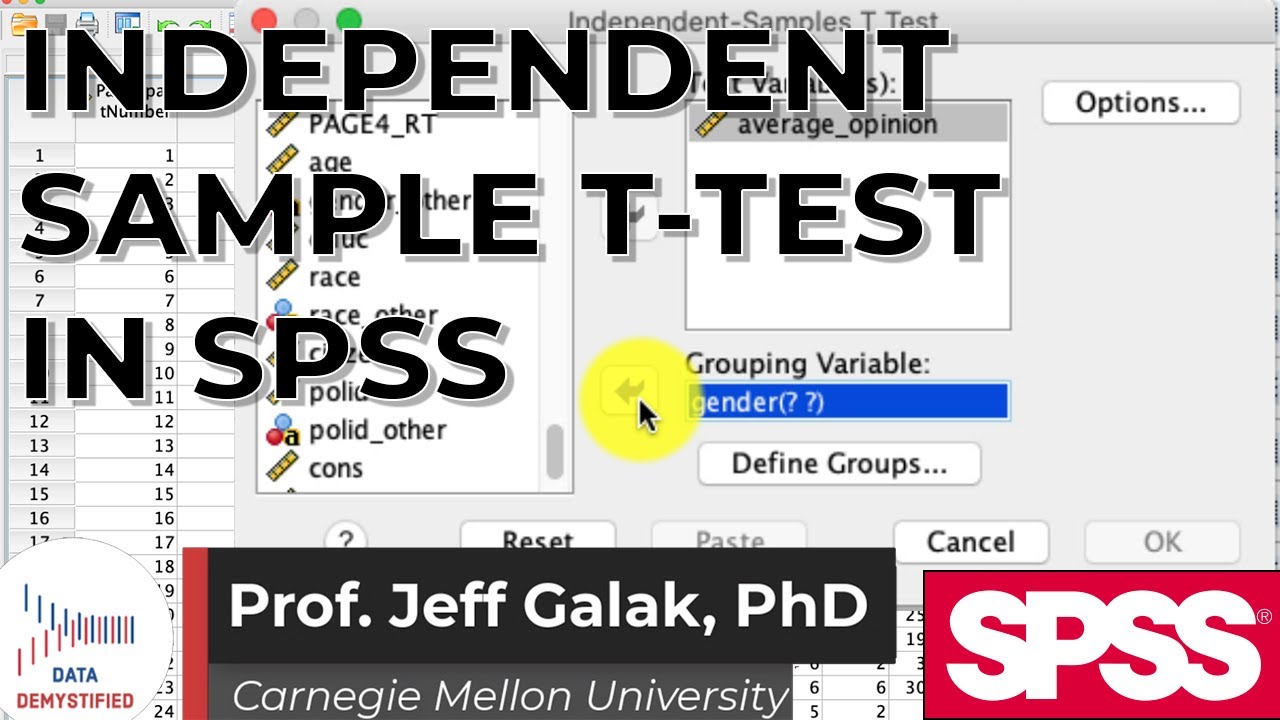
Independent Sample t-Test in SPSS Tutorial (SPSS Tutorial Video #13) - Comparing Two Groups
5.0 / 5 (0 votes)
Thanks for rating: