Experimental Designs; Randomized Complete Block Design; RCBD; Two-Way ANOVA
TLDRThe script delves into the Randomized Complete Block Design (RCBD), a robust experimental design used in field research to control for extraneous variation. It contrasts RCBD with the simpler Completely Randomized Design (CRD), highlighting the use of blocks to reduce experimental error. The script explains the process of dividing a field into blocks based on known sources of variation, then randomly assigning treatments within each block. It illustrates the application of RCBD with an example involving fertilizer doses and plant growth, demonstrating how RCBD can effectively isolate treatment effects using ANOVA analysis.
Takeaways
- π¬ The script discusses the Randomized Complete Block Design (RCBD), an experimental design used in field research to account for significant sources of variation other than treatment.
- π± RCBD is distinguished from Completely Randomized Design (CRD) by the presence of blocks of equal size, each containing all treatments, to control for environmental heterogeneity in field conditions.
- ποΈ The purpose of blocking in RCBD is to reduce experimental error by eliminating known sources of variation among experimental units, thus enhancing the accuracy of treatment effects.
- π The script provides an example where blocks are created to counteract the gradient effect of a river's influence on soil moisture in a field experiment.
- π§βπ¬ RCBD allows for any number of treatments and blocks, making it a flexible design for field research, but it requires careful consideration of the gradient of known sources of variation.
- π The script explains the process of analyzing RCBD results using ANOVA (Analysis of Variance), which includes calculating Sum of Squares for treatments, blocks, and error.
- π The degrees of freedom for each source of variation (treatments, blocks, and error) are calculated to determine the Mean Sum of Squares, which are then used to calculate F-values.
- π The significance of the treatments and blocks is assessed by comparing calculated F-values with critical F-values from an F-distribution table.
- π The script concludes that there is a highly significant effect of fertilizer dose on the fresh weight of plants, as well as a significant effect of the river gradient on plant weight.
- π The experiment's design effectively isolates the effect of the river gradient, allowing for reliable conclusions about the impact of fertilizer doses on plant growth.
- π The process of RCBD and subsequent ANOVA analysis provides a structured method to control for extraneous variables and assess the impact of treatments in field experiments.
Q & A
What is the Randomized Complete Block Design (RCBD)?
-The Randomized Complete Block Design (RCBD) is an experimental design used in field research to control for extraneous variation by dividing the experimental area into blocks and randomly assigning treatments within each block. Each block contains all the treatments, allowing for the comparison of treatment effects while controlling for block-specific variations.
Why is RCBD different from Completely Randomized Design (CRD)?
-RCBD is different from CRD in that RCBD includes blocks of equal size, each containing all treatments, whereas CRD does not have blocking and treatments are assigned randomly across the entire experimental area without considering any known sources of variation.
What is the purpose of blocking in RCBD?
-The purpose of blocking in RCBD is to reduce experimental error by eliminating known sources of variation among experimental units other than the treatment effects. By grouping experimental units into blocks based on similar characteristics, the design can account for these variations and provide more accurate treatment comparisons.
How does RCBD account for a known source of variation?
-RCBD accounts for a known source of variation by dividing the experimental area into blocks based on the gradient of this source. This allows for the comparison of treatments within more homogeneous conditions within each block while acknowledging and controlling for the varying conditions between blocks.
What is the significance of having all treatments in each block?
-Having all treatments in each block ensures that the comparison of treatments is fair and that any block-specific effects are controlled for. This design allows for the assessment of treatment effects while minimizing the impact of other variables that could confound the results.
How does RCBD differ from Latin Square Design when dealing with multiple sources of variation?
-RCBD is used when there is one significant source of variation, while Latin Square Design is used when there are two or more sources of variation that occur in a gradient. Latin Square Design arranges treatments in such a way that each source of variation is accounted for across rows and columns.
What is the role of randomization within each block in RCBD?
-Randomization within each block in RCBD ensures that the assignment of treatments is done in a way that minimizes bias and allows for the assumption of treatment independence. This random assignment helps in estimating the effect of treatments while controlling for block effects.
How flexible is RCBD in terms of the number of treatments and blocks?
-RCBD is a flexible design that allows for any number of treatments and blocks. Researchers can adapt the design based on the specific needs of their experiment, making it suitable for a wide range of experimental conditions and objectives.
What statistical method is used to analyze the results of an RCBD experiment?
-Analysis of Variance (ANOVA) is used to analyze the results of an RCBD experiment. It helps in determining the significance of the differences between treatments and blocks and assesses the impact of known and unknown sources of variation on the experimental outcomes.
Can you provide an example of a situation where RCBD would be used?
-An example of using RCBD could be a field trial to study the effects of different fertilizer doses on the fresh weight of plants. If there is a known source of variation such as soil moisture gradient due to the proximity of a river, RCBD would be appropriate to ensure that the treatment effects are assessed while controlling for this variation.
How is the significance of treatment effects determined in RCBD using ANOVA?
-The significance of treatment effects in RCBD using ANOVA is determined by calculating the F-value for treatments and comparing it with critical F-values from an F-distribution table at a given level of significance (usually 0.05 or 0.01). If the calculated F-value is greater than the critical value, the effect of treatments is considered significant.
Outlines
π¬ Introduction to Randomized Complete Block Design (RCBD)
The script introduces the concept of Randomized Complete Block Design (RCBD), an experimental design used in field research to control for significant sources of variation other than the treatment itself. Unlike the Completely Randomized Design (CRD), which assumes minimal external variation, RCBD is used when there are known and significant variations in the experimental environment. The design involves dividing the field into blocks of equal size, with each block containing all treatment types applied randomly. This approach helps to reduce experimental error by accounting for known variations, such as soil quality or moisture levels, which can differ across the field.
π± Purpose and Methodology of Blocking in RCBD
This paragraph delves into the purpose of blocking within the RCBD. Blocking is used to minimize experimental error by isolating a known source of variation, such as a gradient effect caused by a river's proximity affecting soil moisture. By creating blocks that correspond to areas with similar levels of this variation, the design ensures that conditions within each block are as homogeneous as possible, while allowing for differences between blocks. This method enables the analysis of both the treatment effects and the effects of the known source of variation, providing a more accurate assessment of the treatments' impact.
π Application of RCBD with an Example
The script provides a practical example of applying RCBD in a field experiment to study the effect of fertilizer doses on plant growth. It illustrates how blocks are created to counteract the gradient effect of a river's influence on soil moisture. Each block, representing a different level of the river's impact, contains all eight fertilizer treatments. This setup allows for a two-way ANOVA analysis to compare the effects of both the blocks (representing the river's gradient) and the treatments (different fertilizer doses) on the fresh weight of plants.
π Analyzing RCBD Results Using ANOVA
The paragraph explains the process of analyzing RCBD results through Analysis of Variance (ANOVA). It outlines the calculation of the correction term, sum of squares for total, treatment, blocks, and error. The script details the steps to calculate degrees of freedom and variances for each source of variation. The significance of the treatments and blocks is assessed using F-values, comparing them against critical F-values to determine the statistical significance of the effects.
π Calculation of Sum of Squares and Variance in RCBD
This section focuses on the detailed calculations for the sum of squares for treatments, blocks, and error within the RCBD framework. It explains how to derive these values from the experimental data and how to use them to calculate the mean sum of squares for each source of variation. The paragraph provides the actual numerical values obtained from the example data, showcasing the process of statistical analysis in RCBD.
π Interpreting F-Values and Concluding the RCBD Analysis
The final paragraph discusses the interpretation of F-values for treatments and blocks derived from the RCBD analysis. It compares the calculated F-values with critical values from an F-distribution table to determine the significance of the effects. The script concludes that there is a highly significant effect of fertilizer treatments on plant weight and a significant effect of the river gradient on the experimental outcomes, validating the effectiveness of RCBD in isolating and analyzing the impact of various sources of variation.
Mindmap
Keywords
π‘Randomized Complete Block Design (RCBD)
π‘Experimental Design
π‘Blocking
π‘Treatments
π‘Variation
π‘Homogeneous
π‘Experimental Error
π‘Degrees of Freedom
π‘Sum of Squares
π‘F-Value
π‘ANOVA
Highlights
Randomized Complete Block Design (RCBD) is a type of experimental design used in field research where local control cannot be exercised.
RCBD is distinguished from Completely Randomized Design (CRD) by the presence of blocks of equal size, each containing all treatments.
The purpose of blocking in RCBD is to reduce experimental error by eliminating known sources of variation among experimental units.
Blocks in RCBD are made according to known or suspected sources of variation, such as gradients in one direction.
Conditions within blocks are kept as homogeneous as possible, while they may differ greatly between blocks.
RCBD allows for complete randomization within each block, maintaining the integrity of the experimental design.
RCBD is a flexible design accommodating any number of treatments and blocks, provided blocks are made according to the gradient of known sources of variation.
The example of effects of fertilizer dose on the fresh weight of plants illustrates the application of RCBD in field trials.
The river gradient serves as a significant source of variation in the field example, necessitating the use of RCBD to control for its effects.
Three blocks are created to eliminate the river gradient effect, each with different levels of moisture from the river.
Each block in the example contains eight treatments, demonstrating the complete randomization of treatments within blocks.
Two-way ANOVA is used to compare the effects of blocks and treatments in RCBD.
The total variability in RCBD is the sum of variability due to treatments, blocks, and experimental error.
The calculated F-values for treatments and blocks indicate significant effects on the fresh weight of plants.
The F-value for treatments is significantly higher than the critical value at the 0.01 level, indicating a highly significant effect.
The F-value for blocks is significant but not highly significant, indicating the river gradient's effect on plant weight is notable but less pronounced.
RCBD results can be effectively analyzed through ANOVA, providing insights into the impact of treatments and known sources of variation.
The design of RCBD allows for reliable conclusions about the effects of treatments by controlling for extraneous variations.
Transcripts
Browse More Related Video
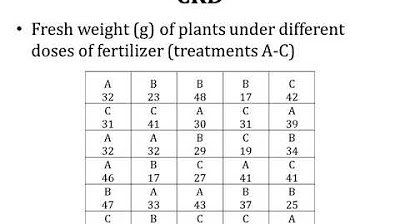
Lecture 18 Experimental Designs; Completely Randomized Design CRD; One Way ANOVA
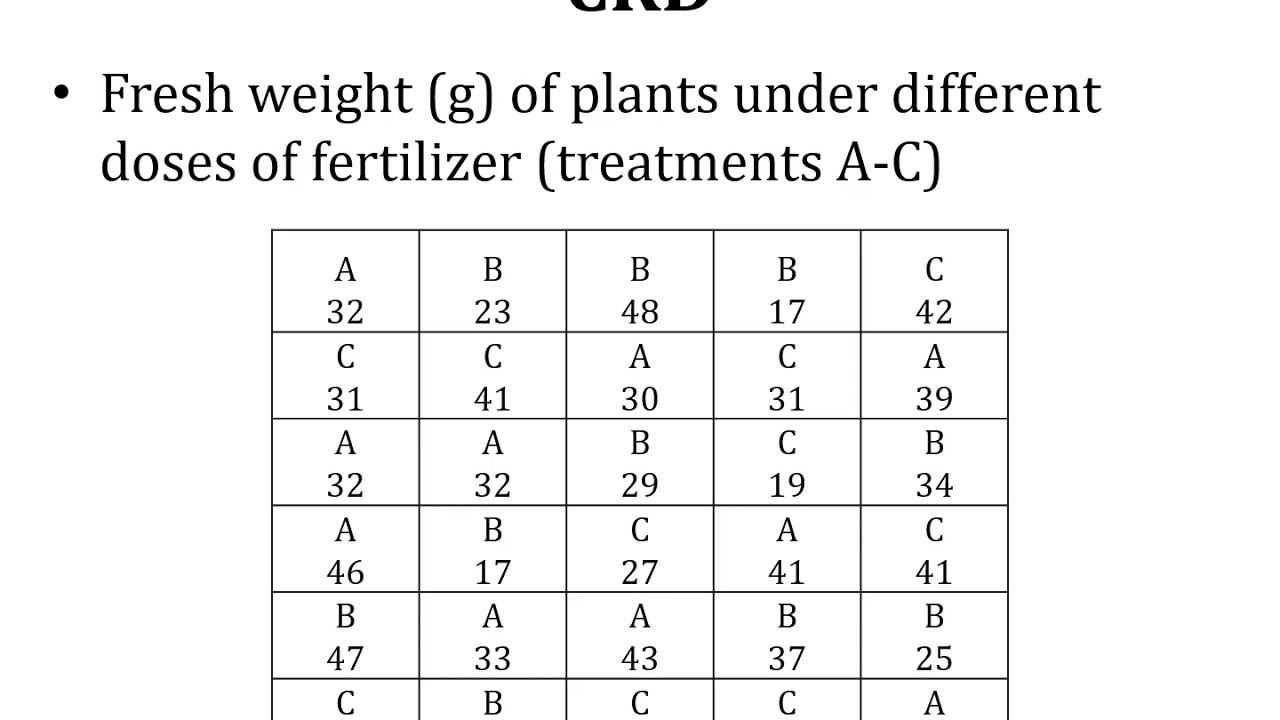
Experimental Designs; CRD; Completely Randomized Design; One-Way ANOVA
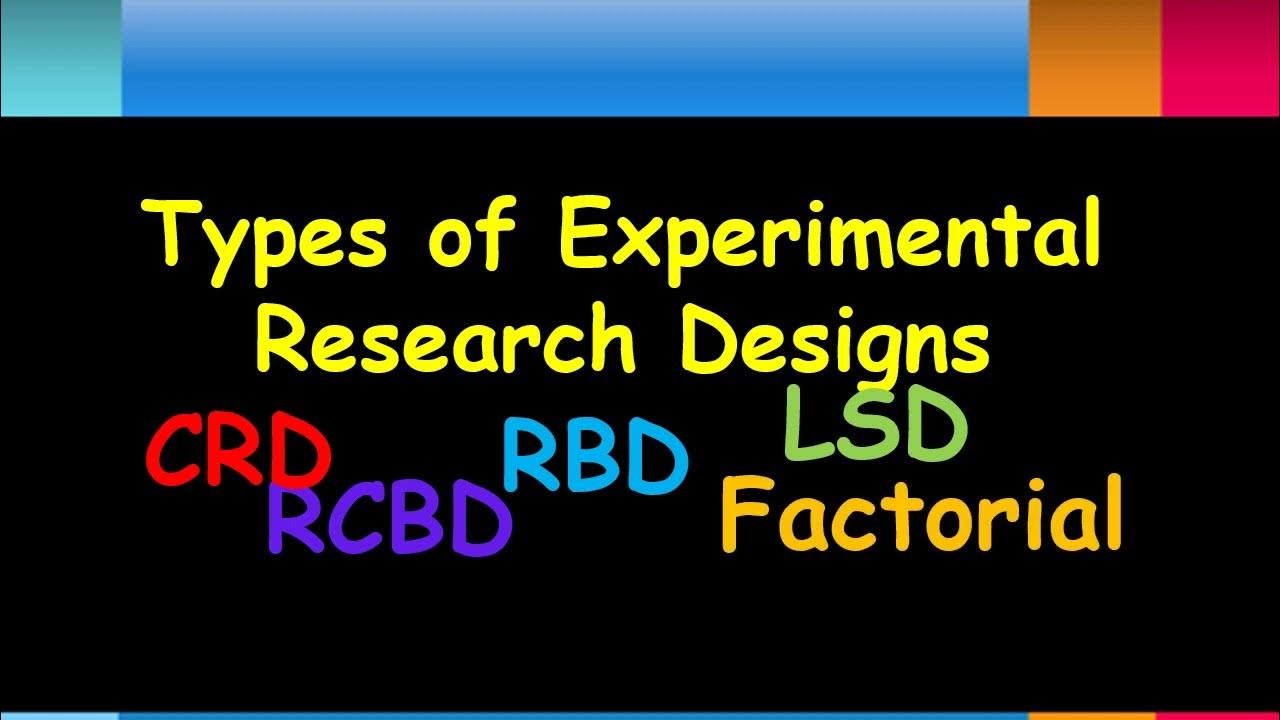
Types of Experimental Research Designs - CRD RBD RCBD LSD FD - Research Methods - Practical Research

Analyse data from experiments with completely randomised design (CRD)
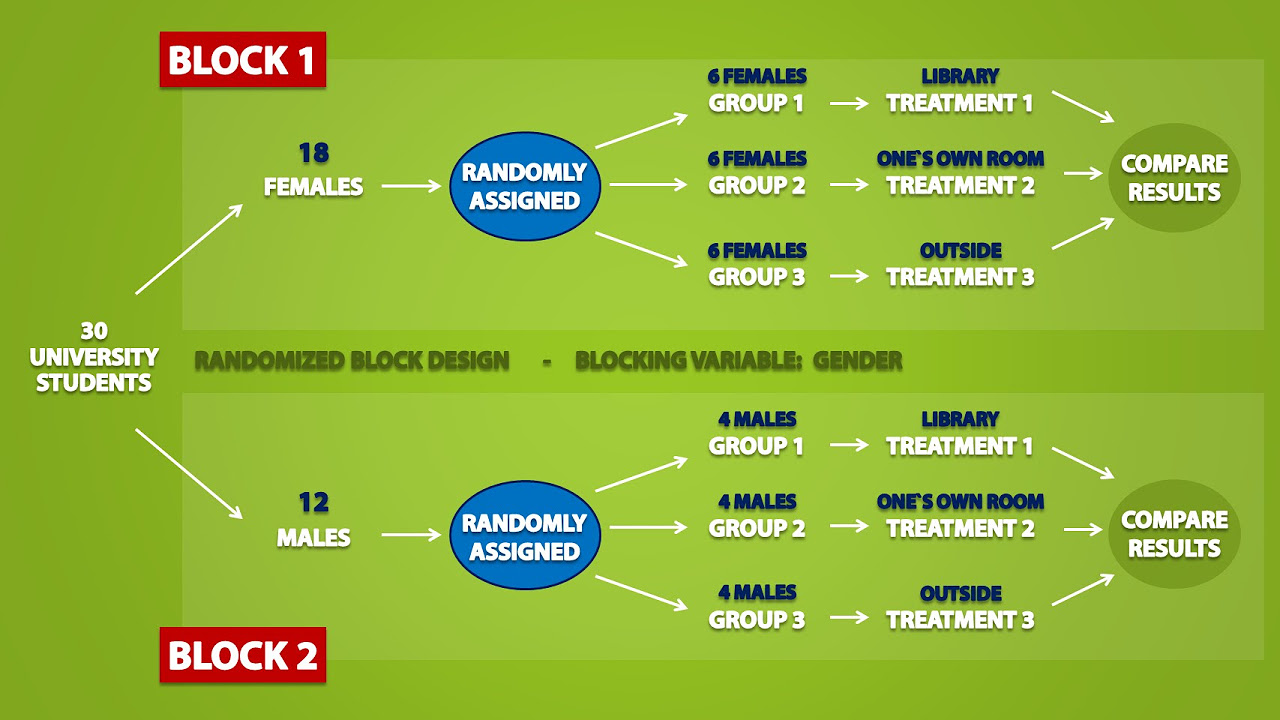
Types of Experimental Designs (3.3)
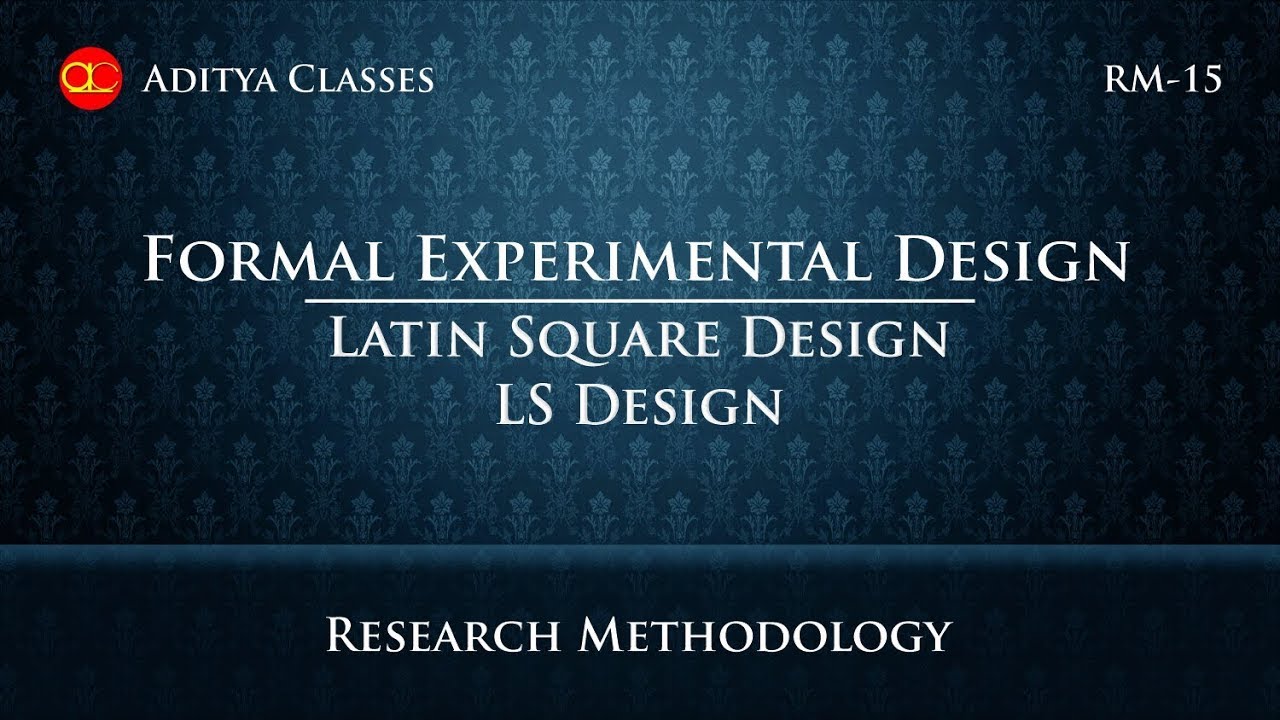
RM-15 | Latin Square Design (in Hindi) | LS Design | Formal Experimental Research Design
5.0 / 5 (0 votes)
Thanks for rating: