Intro to Hypothesis Testing in Statistics - Hypothesis Testing Statistics Problems & Examples
TLDRThis lesson delves into the concept of hypothesis testing, a fundamental aspect of statistics that often challenges students. The instructor aims to simplify the concept by breaking it down into manageable parts, emphasizing that understanding the distinction between the null hypothesis (Hβ) and the alternative hypothesis (Hβ) is key. The lesson explains that hypothesis testing involves making a claim about a population parameter, collecting sample data, and using statistical methods to decide whether to reject or fail to reject the null hypothesis. The process is likened to a legal trial where the null hypothesis represents the presumption of innocence, and the evidence must be strong enough to reject it in favor of the alternative. The importance of statistical significance and the level of confidence in making these decisions is highlighted.
Takeaways
- π Hypothesis testing is a central concept in statistics used to investigate claims or premises.
- π§ The concept can seem complex due to various cases and required different handling, but the overall idea remains the same.
- π Hypothesis testing involves breaking down complex ideas into manageable parts for easier understanding.
- π₯ A hypothesis in statistics is a claim or premise that we aim to test or investigate.
- βοΈ The null hypothesis (Hβ) represents the default or currently accepted value or state of a parameter.
- π Alternative hypothesis (Hβ) is the claim that challenges the null hypothesis, proposing a different state of the parameter.
- π A test statistic is calculated from sample data to help decide whether to reject or fail to reject the null hypothesis.
- π’ The concept of statistical significance helps determine if the data is enough to reject the null hypothesis.
- π Hypothesis testing follows a structured process: from formulating hypotheses to collecting data and making a decision based on the test statistic.
- π The outcomes of a hypothesis test are to either reject the null hypothesis or fail to reject it, based on strong evidence or lack thereof.
- π The level of confidence and level of significance are critical in hypothesis testing, defining how sure we are about our decision to reject or not reject the null hypothesis.
Q & A
What is the main topic of this lesson?
-The main topic of this lesson is hypothesis testing, a central concept in statistics courses that the instructor aims to break down into understandable chunks.
Why does hypothesis testing often seem difficult to students?
-Hypothesis testing seems difficult to students because there are many different cases to consider, each requiring slightly different handling, and most textbooks make the concept more complex than it needs to be.
What is the basic idea behind hypothesis testing?
-The basic idea behind hypothesis testing is to investigate a claim or premise by collecting sample data and then using that information to test the hypothesis, much like how scientific experiments are conducted to test theories.
What is the null hypothesis (H0) in statistics?
-The null hypothesis (H0) is the default premise or claim that is currently accepted as true, which serves as a basis for comparison when testing alternative hypotheses.
What is the alternative hypothesis (Ha), and how is it related to the null hypothesis?
-The alternative hypothesis (Ha) is the claim that challenges the null hypothesis. It is the research hypothesis that the investigator wants to test. It is mathematically opposite to the null hypothesis, representing what the researcher believes to be true as opposed to the null hypothesis.
What are the two possible outcomes of a hypothesis test?
-The two possible outcomes of a hypothesis test are either rejecting the null hypothesis or failing to reject the null hypothesis. Rejection indicates that the data supports the alternative hypothesis, while failure to reject indicates that the data does not provide enough evidence to support the alternative hypothesis over the null hypothesis.
What is the role of a test statistic in hypothesis testing?
-A test statistic is calculated from sample data and is used to help decide whether to reject the null hypothesis or not. It is a numerical value that, when compared to certain thresholds, helps in making a concrete decision in the hypothesis testing process.
What does 'statistically significant' mean in the context of hypothesis testing?
-In the context of hypothesis testing, 'statistically significant' refers to a result that is unlikely to have occurred by chance. It means that the data provides enough evidence to reject the null hypothesis, suggesting that the observed effect or difference is real and not just a random occurrence.
How does the level of confidence relate to hypothesis testing?
-The level of confidence in hypothesis testing indicates the degree of certainty that the decision to reject or fail to reject the null hypothesis is correct. It is expressed as a percentage (e.g., 95%) and represents how confident we are in our conclusion based on the sample data.
What is the relationship between the level of confidence (C) and the level of significance (Ξ±) in hypothesis testing?
-The level of confidence (C) and the level of significance (Ξ±) are complementary; together they add up to 1. The level of confidence represents the percentage of certainty that the decision to reject the null hypothesis is correct, while the level of significance (Ξ±) represents the probability of making a Type I error, which is incorrectly rejecting a true null hypothesis.
How does the process of hypothesis testing relate to the legal system's approach to guilt?
-The process of hypothesis testing is similar to the legal system's approach to guilt in that both start with a presumption of innocence or null hypothesis. In a court, a person is presumed innocent until proven guilty, and in hypothesis testing, the null hypothesis is assumed to be true until the evidence (data) suggests otherwise through statistical analysis.
What is the next step after understanding the basics of hypothesis testing?
-After understanding the basics of hypothesis testing, the next step is to learn how to write down the hypotheses, determine the test statistic, and understand when to reject or fail to reject the null hypothesis based on numerical values and statistical thresholds.
Outlines
π Introduction to Hypothesis Testing
The paragraph introduces the concept of hypothesis testing, emphasizing its importance in statistics education. It acknowledges the common difficulty students face with the topic due to the complexity of textbooks and the variety of cases to consider. The speaker expresses confidence in their ability to break down the concept into manageable parts, ensuring that students understand the process by following the lessons. The paragraph sets the stage for a detailed exploration of hypothesis testing, promising to demystify the concept and make it accessible.
π― Understanding Hypotheses and the Null Hypothesis
This paragraph delves into the specifics of what a hypothesis is in the context of statistics, differentiating it from a general idea or claim. It introduces the null hypothesis (Hβ) as the default or currently accepted hypothesis, which serves as a benchmark for comparison. The speaker uses an example of a candy machine to illustrate how the null hypothesis represents the status quo that an alternative hypothesis (Hβ) aims to challenge. The paragraph explains that the goal is to test the alternative hypothesis against the null, using statistical methods to determine if there's sufficient evidence to reject the null.
π The Process of Hypothesis Testing
The paragraph explains the two possible outcomes of a hypothesis test: rejecting the null hypothesis or failing to reject it. It likens the process to a legal system where the null hypothesis is assumed true until evidence proves otherwise. The speaker introduces the concept of a test statistic, which is calculated from sample data to help decide whether to reject the null hypothesis. The paragraph emphasizes the importance of statistical significance in determining the outcome of the test, highlighting the need for a concrete method to assess the validity of the null hypothesis.
π Real-Life Example of Hypothesis Testing
Using the candy machine example, this paragraph illustrates how hypothesis testing would be applied in a real-world scenario. It describes the process of collecting data by sampling chocolate bars and calculating the average mass to test the null hypothesis that the machine produces 5-gram bars. The speaker discusses different scenarios with varying results, explaining how these outcomes would influence the decision to reject or not reject the null hypothesis. The paragraph underscores the subjectivity of statistical significance and the need for a standardized approach to hypothesis testing.
π’ Statistical Concepts: Confidence and Significance
The paragraph introduces key statistical concepts of confidence level (C) and level of significance (alpha), explaining their roles in hypothesis testing. It clarifies that the confidence level represents the degree of certainty in the decision to reject or not reject the null hypothesis, while the level of significance is the complement to the confidence level. The speaker emphasizes the importance of a high confidence level in ensuring the credibility of the test results, and draws an analogy between hypothesis testing and the legal system, where a high level of certainty is required to prove guilt beyond a reasonable doubt.
Mindmap
Keywords
π‘Hypothesis Testing
π‘Null Hypothesis (H0)
π‘Alternative Hypothesis (Ha or H1)
π‘Statistically Significant
π‘Test Statistic
π‘Level of Confidence
π‘Level of Significance (Alpha)
π‘Reject the Null Hypothesis
π‘Fail to Reject the Null Hypothesis
π‘Sampling
π‘Statistical Opposites
Highlights
The lesson focuses on hypothesis testing, a central topic in statistics courses.
The instructor aims to break down complex concepts into understandable chunks for students.
Hypothesis testing can seem difficult due to various cases and required different handling.
The overall idea of hypothesis testing remains the same across different cases.
A hypothesis in statistics is a claim or premise that is tested or investigated.
The null hypothesis (Hβ) represents the default or currently accepted value for a parameter.
The alternative hypothesis (Hβ) is the claim that challenges the null hypothesis.
The null and alternative hypotheses are mathematical opposites.
There are two possible outcomes in a hypothesis test: rejecting the null hypothesis or failing to reject it.
Rejecting the null hypothesis promotes the alternative hypothesis as more likely to be true.
Hypothesis testing uses a test statistic calculated from sample data to make decisions.
Statistical significance helps determine if the data is enough to reject the null hypothesis.
The level of confidence and level of significance are used to gauge the certainty of the decision made in hypothesis testing.
The process of hypothesis testing is likened to the legal system where innocence is presumed until proven guilty.
Understanding the concepts of null and alternative hypotheses is crucial for conducting hypothesis testing.
The upcoming sections will teach how to write hypotheses, calculate test statistics, and make decisions based on numbers rather than guesses.
The analogy of the legal system helps to clarify the process and outcomes of hypothesis testing.
Transcripts
Browse More Related Video
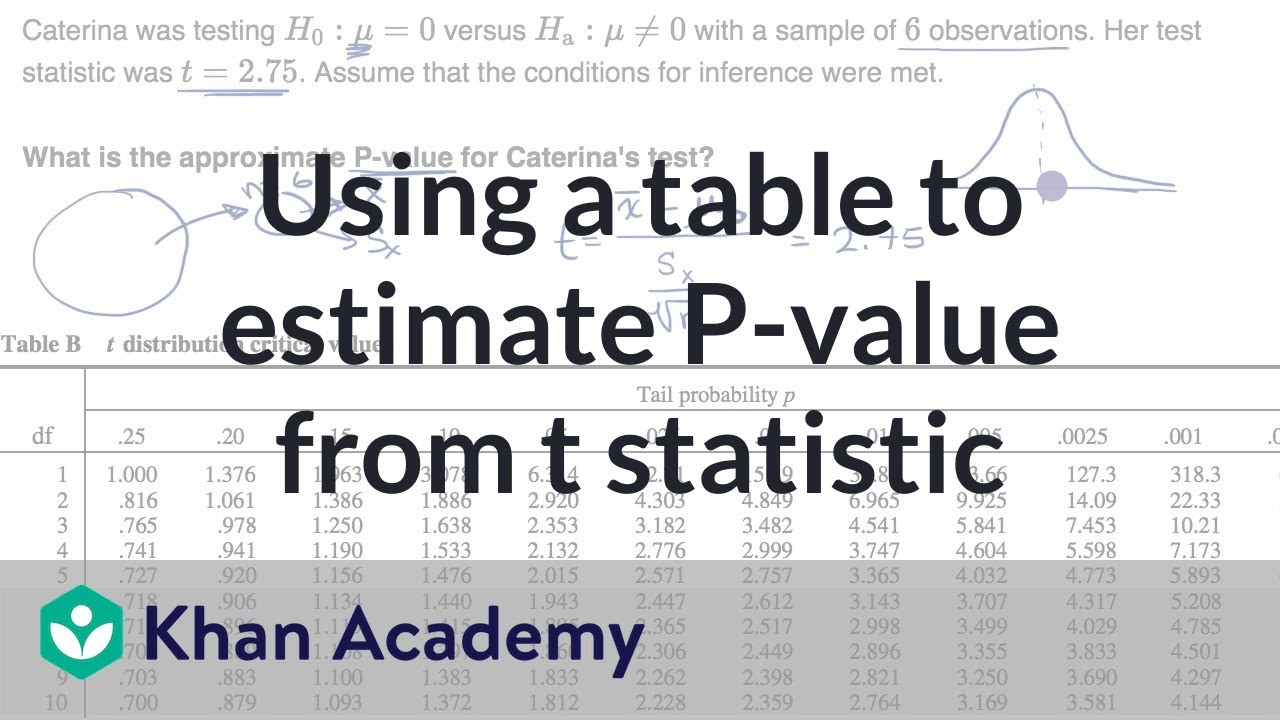
Using a table to estimate P-value from t statistic | AP Statistics | Khan Academy
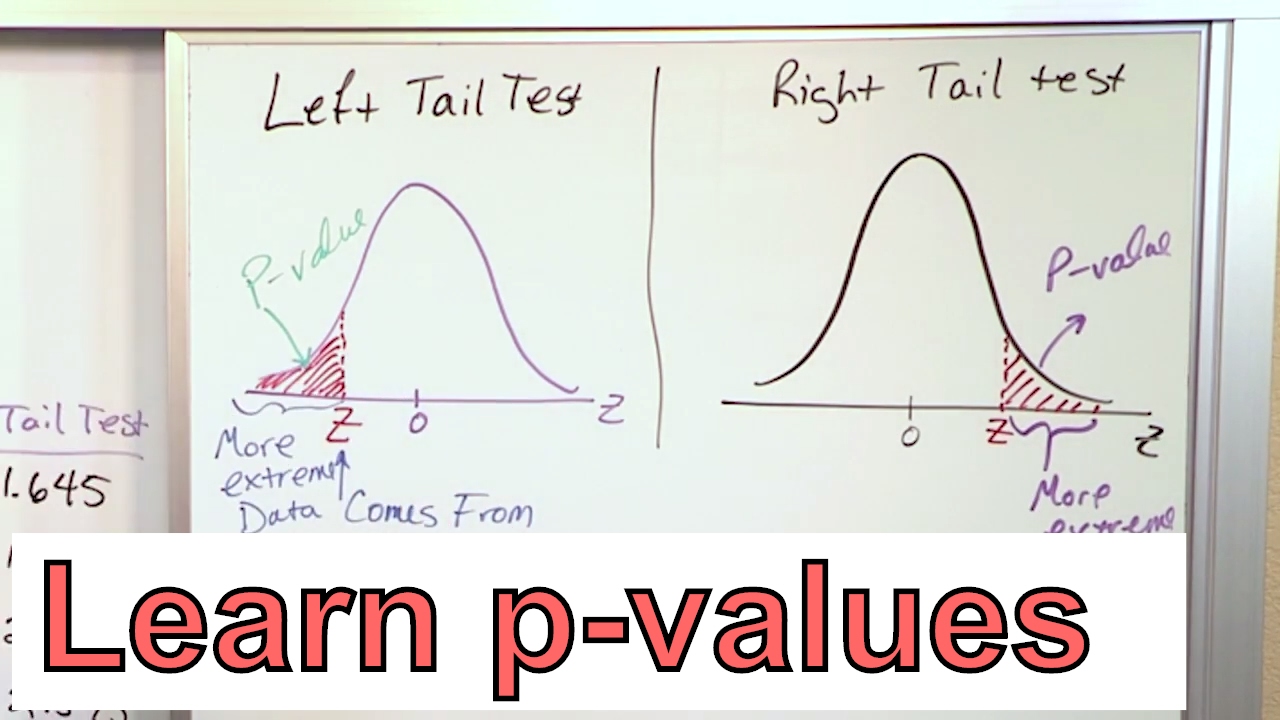
Calculate the P-Value in Statistics - Formula to Find the P-Value in Hypothesis Testing
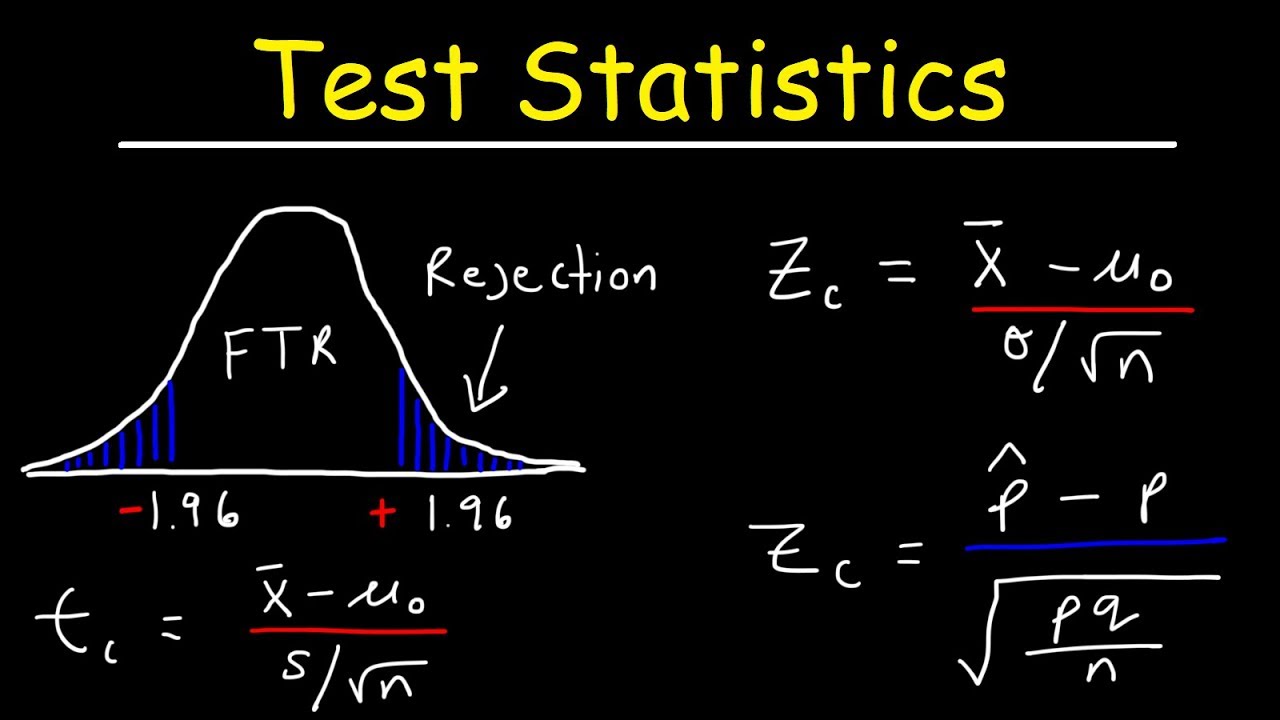
Test Statistic For Means and Population Proportions
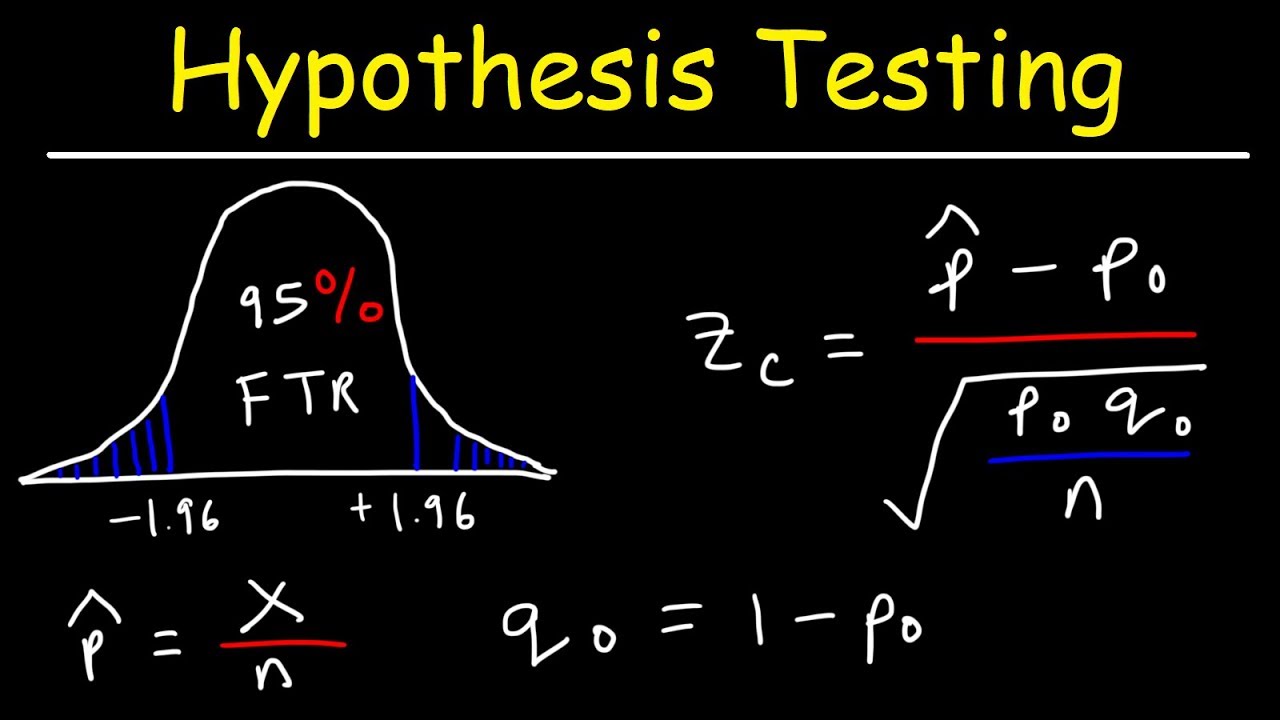
Hypothesis Testing - Solving Problems With Proportions
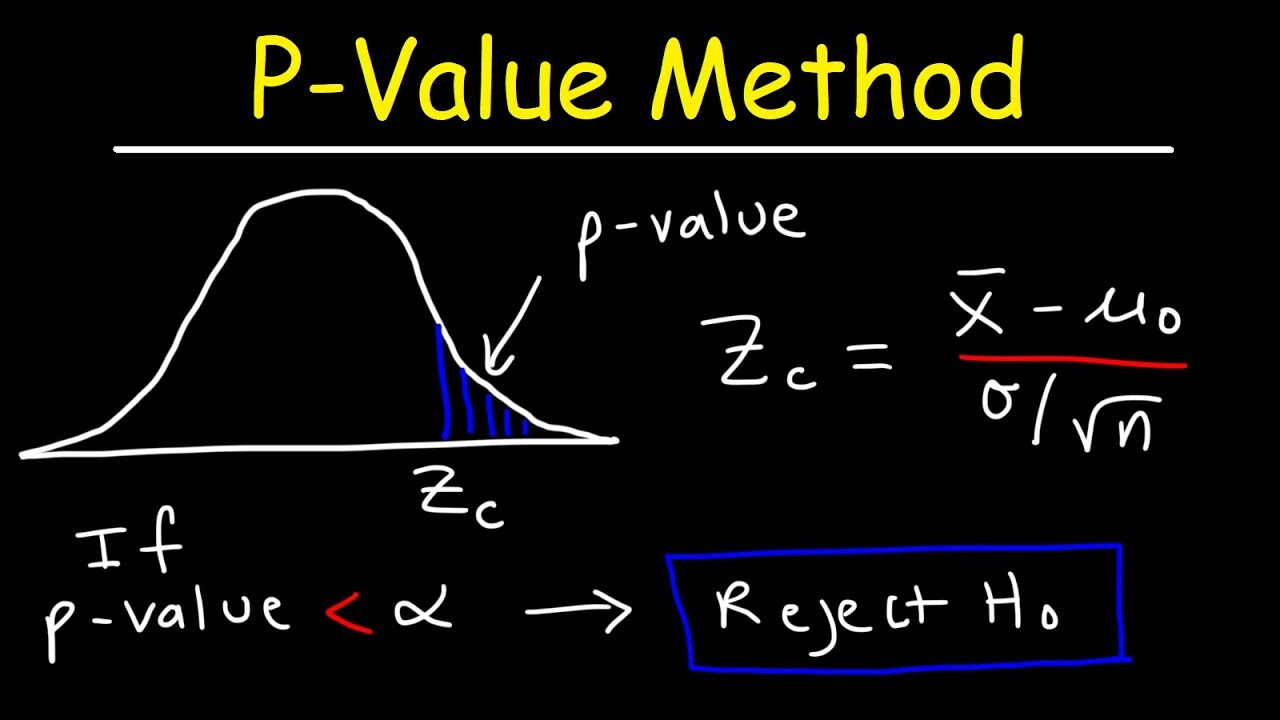
P-Value Method For Hypothesis Testing
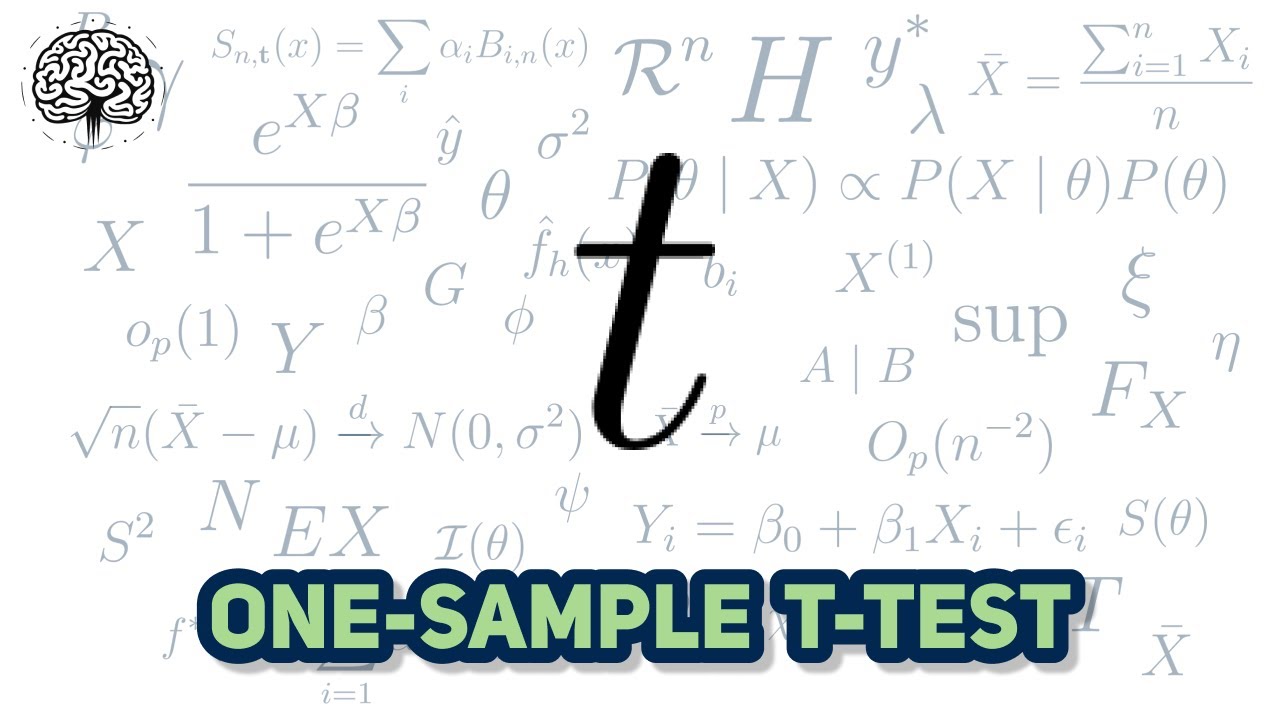
Explaining The One-Sample t-Test
5.0 / 5 (0 votes)
Thanks for rating: